- 1School of Biological, Earth and Environmental Sciences, University College Cork, Cork, Ireland
- 2Centre for Ecology and Hydrology, Bush Estate, Edinburgh, UK
Climate change may have demographic consequences for marine top predators if it leads to altered rates of skipped breeding. Here we examine variation in skipping propensity at both the population and individual levels in common guillemots Uria aalge in relation to climate and oceanographic variables and explore the extent to which skipping may be adaptive or an unavoidable consequence of ecological or social constraints. We assumed a detection probability for birds present in the colony of 1.00 and skipping events were defined to include both resightings of non-breeders and failures to resight individuals known to be alive (not present at the colony but resighted in future years). Skipping frequency was higher in years where sea surface temperatures (SST) were higher in winter (both in the current and previous year), when guillemots from our study colony disperse widely across the southern North Sea. Individuals differed consistently in their average skipping propensity and their responses to SST. Males and females were equally likely to skip on average and the frequency of skipping increased in the oldest age classes. Birds that skipped in year t had lower breeding success in year t + 1 if they laid an egg, compared to birds that did not skip in year t. Lifetime reproductive output was negatively related to individual skipping frequency. These results imply that skipping is driven by individual-specific constraints, although we cannot rule out the possibility that birds benefit from skipping when environmental (or internal) signals indicate that breeding in poor years could be detrimental to their residual reproductive value. While future climate change might lead to guillemots skipping more often due to carry-over effects from wintering to breeding periods, the net demographic impacts may be subtle as the absolute frequency of skipping may remain low and individuals will not be equally affected.
Introduction
A central premise of life history theory is that reproduction is costly (Stearns, 1989). At a proximate level, animals may only be able to breed if exogenous food and nutrient intake, or endogenous reserves, surpass a critical threshold (Erikstad et al., 1998; Meijer and Drent, 1999), while investment in breeding entails potential physiological costs such as reduced immune function or increased susceptibility to oxidative stress (Harshman and Zera, 2007; Monaghan et al., 2009). At an ultimate level, parents face a fitness trade-off between current and future reproduction: increased allocation of resources to current offspring production may come at the expense of reduced parental survival chances and hence reduced residual reproductive value (Williams, 1966; Charlesworth, 1994).
Annual reproductive investment in iteroparous species involves a series of sequential “decisions,” chief among which is the decision of whether to breed or not (Clutton-Brock, 1988; Newton, 1989). For some vertebrate species living in seasonal environments, the norm is for mature individuals to breed in alternate years or less often (e.g., obligate biennial breeding in albatrosses, Jouventin and Dobson, 2002; and in many fish, amphibians and reptiles: Bull and Shine, 1979). However, more typically annual breeding is the norm but in such situations a variable (often substantial) fraction of individuals that have already bred before may skip in some years, i.e., facultative annual breeding (Aebischer and Wanless, 1992; Chastel, 1995; Harris and Wanless, 1995; Cam et al., 1998; Nur and Sydeman, 1999; Reed et al., 2004; Johnston and Post, 2009; Rideout and Tomkiewicz, 2011). Given its pervasive direct and indirect ecological effects, climate likely plays an overarching role in determining both how often individuals skip (proximate influence of climate via cues or constraints) and when skipping would be profitable from a fitness-maximization perspective (ultimate influence of climate).
Hypotheses to explain intermittent breeding can be grouped into adaptive and non-adaptive explanations. In temporally varying environments, skipping may be adaptive when the costs of reproduction are higher during unfavorable seasons (Schaffer, 1974; Bull and Shine, 1979; Erikstad et al., 1998; McNamara and Houston, 2008). Long-lived species, in particular, are expected to exhibit restrained reproductive investment under poor conditions because even small reductions in adult survival will reduce the number of subsequent breeding seasons and hence depress lifetime reproductive success substantially (Curio, 1988; Linden and Møller, 1989). For example, Coulson (1984) reasoned that common eider (Somateria mollissima) refrain from breeding in years of low adult survival in order not to incur further survival costs or reductions in future reproductive success, while Aebischer and Wanless (1992) made similar adaptive arguments for skipped breeding in European shags (Phalacrocorax aristotelis). The capacity to regulate breeding effort by skipping in certain years (i.e., adaptive phenotypic plasticity in breeding propensity) would presumably only evolve if cues are available to individuals prior to the breeding season that reliably correlate with environmental conditions later in the year (including potentially the following winter) that actually determine the fitness costs of reproduction (Erikstad et al., 1998; Bårdsen et al., 2011).
Non-adaptive explanations suggest that skipping breeding in some years is itself not advantageous, but rather an unavoidable outcome of other events. For example, Danchin and Cam (2002) found that black-legged kittiwakes (Rissa tridactyla) that changed breeding areas between years were 1.7 times more likely to skip breeding than those that remained in the same area. They argued that adult non-breeding may thus be a cost of breeding dispersal, given that dispersers often struggle to find a new breeding site or mate (Danchin and Cam, 2002). Similarly, experienced adults may have no option but to skip breeding following forced eviction from their previous breeding site or divorce from their previous mate (Harris and Wanless, 1995; Kokko et al., 2004; Jeschke et al., 2007). Skipping may therefore be non-adaptive in the sense that breeding propensity itself is not the direct target of selection, although it may be affected by other behaviors and traits (e.g., breeding dispersal, mate fidelity, resource gathering abilities) which are potential targets of selection. In reality, adaptive and non-adaptive explanations may both apply and skipping may be linked to future survival or reproductive success through both causal and non-causal mechanisms (Cam et al., 1998; Danchin and Cam, 2002). Moreover, individuals may vary in resource acquisition abilities or other traits that influence their physiological state, which in turn affects their ability to breed (Mills, 1989; Harris and Wanless, 1995; Cam et al., 1998; Cubaynes et al., 2011). While such individual heterogeneity may obscure trade-offs between current breeding and survival/future reproduction, it does not mean they do not exist: the fitness consequences of skipping may simply be different for different classes of individuals (Cam et al., 1998) and some may be better able to bear the costs of reproduction than others (Robert et al., 2012).
Among marine top predators, which tend to be long-lived, intermittent breeding is relatively common and variation in breeding propensity has been shown to have a strong influence on population numbers (Aebischer and Wanless, 1992; Jenouvrier et al., 2005a; Forcada et al., 2008). Climate may influence breeding propensity of marine top predators directly, for example if climate variables are used as cues to regulate breeding effort or if the survival benefits of non-breeding depend on weather conditions (Erikstad et al., 1998; Robert et al., 2012), or indirectly via bottom-up or top-down effects on marine ecosystems (Frederiksen et al., 2006). Few studies, however, have tested for associations between climate variables and breeding propensity and most have done so only at the population level (e.g., Jenouvrier et al., 2005a; Mills et al., 2008; Cubaynes et al., 2011). Given the global trend in climate warming (Solomon, 2007) coupled with increased climate variability (Rahmstorf and Coumou, 2011; Seager et al., 2012) there is a need to better characterize and understand such associations in wild populations and the conservation implications. Moreover, very little is known about individual-level patterns or fitness consequences of skipped breeding in marine top predators (Cam et al., 1998; Danchin and Cam, 2002), information that is important to predicting the evolutionary and demographic consequences of climate change (Forcada et al., 2008).
The aim of the current analysis was to shed light on these issues using long-term, individual-based data on common guillemots (Uria aalge) from a well-studied colony in Scotland. Common guillemots are long-lived and lay a single-egg clutch. Species with such a life history are good candidates for investigating potentially adaptive adult non-breeding because they (a) are expected to be prudent parents, given the importance of longevity to their lifetime reproductive success (Moreno, 2003; Reed et al., 2008), and (b) have fewer options than species that lay multiple eggs when it comes to adjusting reproductive effort (Cubaynes et al., 2011). While they could adjust investment in the single egg or chick in response to changes in the environment (e.g., Erikstad et al., 1997), not breeding in the first place may be less costly in terms of future fitness. Common guillemots from our study colony spend the winter months in the shallow southern North Sea (Reynolds et al., 2011), an area that has experienced extreme local warming rates over the past 30 years (Rayner et al., 2003). Previous studies of common guillemots have established links between demographic rates or phenology and marine climate experienced both locally around the breeding colony and further afield in the wintering area (e.g., Frederiksen et al., 2004a; Sandvik et al., 2005; Votier et al., 2008; Reed et al., 2009). We therefore (1) tested for population-level associations between breeding propensity and climate-related variables measured at different temporal and spatial scales, (2) investigated whether individuals varied in their climate responses, (3) tested for effects of age and sex on breeding propensity, and (4) explored the individual-level fitness consequences of skipping.
Materials and Methods
Study Species, Site, and Data Collection Methods
Common guillemots (hereafter guillemots) are medium-sized seabirds with a circumpolar distribution in temperate and sub-arctic waters of the North Atlantic and North Pacific. They breed on cliffs from the age of 5 or 6 years and can live for 30 or more years (for general information, see Harris and Birkhead, 1985; Gaston et al., 1998). The data used here were collected as part of a long-term study on the Isle of May, Firth of Forth, Scotland (56°11′ N, 2°33′ W). Since 1982, breeding guillemots of unknown age in five study areas of varying cliff topography and bird density have been caught and fitted with unique metal and color leg-rings under license from the British Trust for Ornithology. Every year additional breeding adults are ringed to replace those disappearing from the study population to maintain sample size. Average age of birds ringed in the earliest cohorts was assumed to be older than that of later cohorts, as new birds caught after the first few years of the study were likely to be (although could not be confirmed as) first-time breeders (Harris and Wanless, 1995; Crespin et al., 2006). The study areas were viewed from permanent hides 10–20 m away. All breeding sites were numbered on large photographs and the identities of both adults at all sites were ascertained several times every season. Intense searches were carried out at least daily throughout the breeding season to determine whether ringed birds (1) were present in the colony, (2) held a breeding site, (3) laid an egg, and (4) successfully fledged a chick, i.e., left the colony when aged at least 14 days old (Harris and Wanless, 1988). Particular attention was paid to adjacent areas to ensure that birds resident outside the main study plots were not overlooked. At this colony guillemot pairs typically persist across multiple years (Jeschke et al., 2007) and birds were sexed by observations of copulations. The dataset comprised 9741 records (bird-year combinations) over 33 years from 891 ringed individuals (412 females, 442 males, 37 sex unknown). For further details on the study population see Harris and Wanless (1988).
Defining and Measuring Skipped Breeding
High-quality breeding sites are limited in this colony and fiercely contested (Kokko et al., 2004). Across all breeding seasons (1982–2014), an annual average of 7.1% (696/9741) of individuals that had bred at least once did not hold a site. The majority (86.1%, 599/696) of these siteless birds were recorded non-breeders in the colony, while the remainder (97/696) were not seen in that year (Figure 1). Observer effort remained very high over the study period and resighting probabilities (i.e., the probability that an alive bird was seen in a given breeding season on the island) of birds ringed as adults, as estimated from an integrated Bayesian analysis of mark–recapture–recovery data (Reynolds et al., 2009), were also very high and consistent across years (always >93%). Non-breeding guillemots spend much time in the colony near their last breeding site. Although it is not possible to say with 100% certainty that an unrecorded bird was not present somewhere in the colony, this seems very unlikely and we assume a detection probability for birds present in the colony (as breeders or non-breeders) of 1.00. Thus, we are confident that any ringed birds not seen in a given year did not actually return to the colony, but rather spent the summer somewhere away from the island (e.g., offshore). We defined skipped breeding as where an individual which bred previously was either seen somewhere in the colony during the breeding season without a breeding site, or was not seen in that breeding season but was seen in a subsequent season. This measure of skipping excluded cases where a bird was not seen that year and then never seen again (and therefore presumed to be dead), but it includes consecutive skipping events so long as the bird was seen again. The proportion of birds skipping could not be calculated for the 2014 breeding season, as birds not seen in this year may still be alive (confirming this requires resighting them in future years). The estimate for 2013 might also be an underestimate for the same reason. Birds observed without a site in their last year of being seen were defined as having skipped.
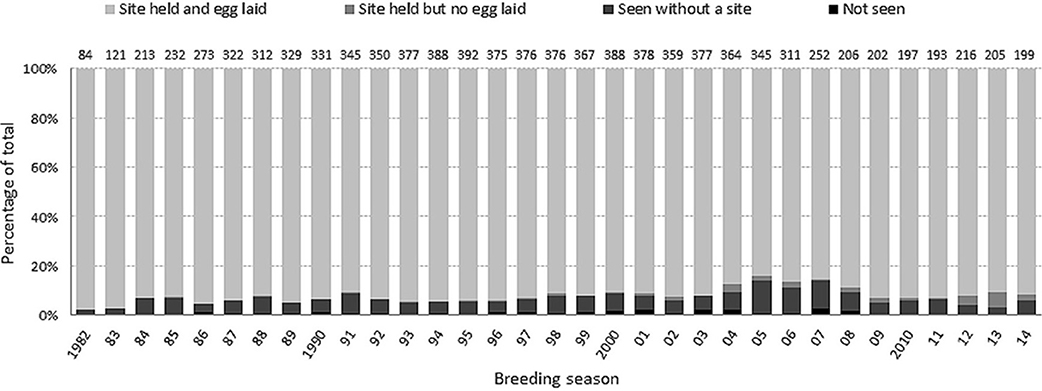
Figure 1. Breakdown by breeding season of the percentage of birds in each category. Non-skipping encompasses the first two categories (site holders) and skipping encompasses the last two (seen without a site or not seen but known to be alive).
By this definition, “breeders” or “non-skippers” were adults that held a site during the breeding season, and an annual average of 92.9% (9045/9741) of known individuals fell into this category (Figure 1). The majority (99.1%, 8962/9045) that held a site bred i.e., an egg was recorded. Birds holding a site where no egg was recorded were included in the non-skippers category as we considered that they had made a substantially larger commitment than had those without a site. However, the results and conclusions were unchanged when they were included in the skippers category (results not presented).
Statistical Analyses
For the population-level analysis, the annual incidence of skipping was quantified by summing the number of birds that skipped each year and dividing by the total number of birds (i.e., skippers + non-skippers). Similarly, for the individual-level analyses the incidence of skipping was a binary variable with a value of one assigned to birds that skipped, and a value of zero assigned to birds that did not skip. The data were then analyzed via logistic regression using either generalized linear models (GLMs, population-level analyses) or generalized linear mixed models (GLMMs, individual-level analyses). Some previous studies (e.g., Cam et al., 1998; Forcada et al., 2008; Cubaynes et al., 2011; Sanz-Aguilar et al., 2011) used multi-state capture–recapture (or multi-event) models to estimate rates of adult non-breeding when non-breeders are unobservable (e.g., because they remain at sea during the breeding season). In our study, however, average resighting probability was extremely high (~96%; Reynolds et al., 2009) so our estimates of breeding propensity were not unduly biased by recapture probabilities less than one (see also Danchin and Cam, 2002 for a similar justification for using logistic regression, rather than a multi-state mark recapture approach, to analyze factors influencing breeding status).
Population-level analyses
The population-level analyses tested for significant relationships between annual breeding propensity and candidate annual covariates linked directly or indirectly to climate. Guillemots disperse widely outside of the breeding season and ringing recoveries and data from geolocators indicate that in December and January adult guillemots from the Isle of May are concentrated in the southern North Sea (Reynolds et al., 2011; Harris et al., in press), although birds are also known to attend the breeding colony intermittently throughout the non-breeding season (Harris and Wanless, 1989, 1990). Weather or oceanographic conditions experienced in the winter range may therefore have a direct or indirect effect on annual breeding propensity via cues or constraints. Sea surface temperature (SST) is a good candidate variable in this regard, given that (a) birds may be able to directly sense SST or other closely correlated atmospheric/oceanographic variables and use this as a predictive cue for breeding decisions, and (b) SST correlates with various demographic rates in seabirds in the North Sea/Northeast Atlantic region (e.g., Frederiksen et al., 2004b; Sandvik et al., 2005). Weekly SST data were obtained from NOAA (Optimum Interpolation SST V2 obtained from http://www.esrl.noaa.gov/psd/data/gridded/data.noaa.oisst.v2.html) for two regions of the North Sea. The first corresponded to the winter range (51–56°N, 1–5°E) and values were averaged for the months November, December and January (hereafter “winter range SST” or wSST). The second was an area in the immediate vicinity of the breeding colony (55–57°N, 3–0°W) and values were averaged for February and March (hereafter “local spring SST,” locSST). The latter was included to capture environmental conditions that might affect breeding propensity prior to the breeding season, when birds are likely to forage closer to the breeding colony. We tested for lagged effects (1 year lag) of both SST variables, given that SST may affect seabird vital rates indirectly through the food chain (Frederiksen et al., 2006; Lahoz-Monfort et al., 2013). We also considered the winter North Atlantic Oscillation Index (wNAO, Hurrell, 2014), a broad-scale proxy measure that correlates well with life history traits and population dynamics of North Atlantic seabirds (Reid et al., 1999; Durant et al., 2004; Grosbois and Thompson, 2005; Reed et al., 2006; Sandvik et al., 2008, 2012). The wNAO index is the mean December–March value for year t, where December is in year t-1. Lagged effects of NAO have been documented for seabirds (Lewis et al., 2009; Sandvik et al., 2012) so we also considered a lag of 1 year. Finally, we considered an effect of population size (the annual count of breeding guillemots on the Isle of May) to test for density dependence.
As a preliminary step, we first tested for time trends in the annual frequency of skipping and each of the explanatory variables. The plot of annual skipping frequency vs. year (Figure 2A) suggested a non-linear trend, while exploratory analyses indicated temporal autocorrelation in the raw values at a lag of 1 year. A generalized additive model was therefore fitted to the time series, allowing for an auto-regressive serial correlation structure, using the gamm function in the R package mgcv (Wood, 2006). This uses cross-validation to estimate the optimal amount of smoothing for the continuous variable “year” and a normal error distribution was assumed. Three different models were compared: one with no residual correlation structure (i.e., residuals assumed to be independent) and models with serial correlation structures of orders one and two (i.e., lagged residual correlations of one and two years), respectively. The model with the lowest AIC was chosen as the best descriptor of the time series (Zuur et al., 2009). The same process was repeated for each of the candidate explanatory variables to test for temporal trends in each (Figures 2B–E). Pair-wise Pearson correlations among all explanatory variables were also tested for.
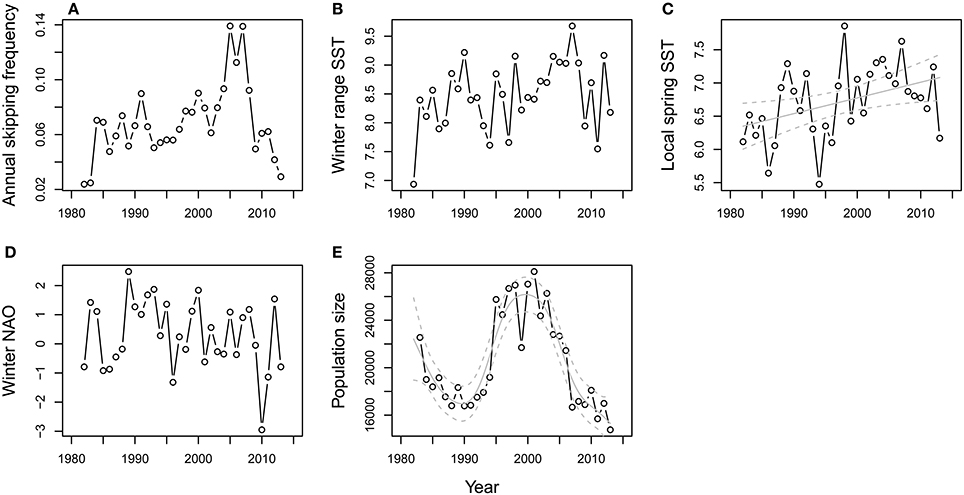
Figure 2. Temporal trends in annual skipping frequency and the covariates considered in the population-level analyses. Trend in (A) annual skipping frequency, (B) winter range SST, (C) local spring SST, (D) winter NAO, and (E) population size. Significant GAM fits (± confidence bands) are included in gray for local spring SST and population size.
Next, a series of nested GLMs with logit link functions and binomial errors were fitted where the response variable was a concatenated vector of the number of birds that skipped breeding each year and the number of birds that did not skip. The full model consisted of additive effects of each annual covariate. and this was simplified using backwards selection, where each explanatory variable was dropped, in turn, and each time an analysis of deviance test applied (with the difference in deviance between nested models assumed to follow a chi-squared distribution with one degree of freedom). The variable with the highest P-value was removed, and then the process was repeated until all remaining variables were significant at the 5% level (Zuur et al., 2009). Interactions were not tested for as there were too few data points.
Individual-level analyses: explaining variation in skipping
A population-level relationship between breeding propensity and the environment could occur without necessarily existing within individuals (Figure 3). To characterize individual-level patterns, a GLMM was fitted where the binary response variable indicated whether the individual skipped or not that year. The population-level analysis revealed significant effects of wSST and lagged wSST (see Results). For the individual-level analysis, we focussed on the unlagged effect of wSST, as GLMMs including wSST, lagged wSST and their interactions with age and sex did not converge. To separate within- and between-individual effects of wSST, a technique known as “within-subject centering” (van de Pol and Wright, 2009) was used, which involved first calculating the mean value of wSST (wSST_mean) for each individual and then expressing annual values as deviations from this mean (wSST_dev). Both wSST_mean and wSST_dev were then included as fixed effects in the GLMM, with the former corresponding to the between-individual effect and the latter to the within-individual effect (see Figure 3). Fixed effects of sex (two-level factor: males and females; unknowns excluded) and years since ringing (as a proxy for age, see Crespin et al., 2006) and years since ringing squared were included. Models including two-way interactions did not converge so we only considered main effects. A random effect of year was included to account for interannual variation in skipping not captured by the fixed effects. A random effect of individual was included to account for non-independence of multiple measures of skipping on the same individual (and to quantify the magnitude of between-individual differences in average skipping propensity). Three random effects structures were compared: (1) random intercepts, (2) random intercepts and random slopes for the wSST deviation effect, correlation between intercept and slope constrained to zero; and (3) random intercepts and random slopes with the correlation estimated. The best random effects structure was chosen on the basis of likelihood ratio tests, including all fixed effects in each case regardless of the their statistical significance (Zuur et al., 2009, p. 121). Once the best random effects structure was found, non-significant fixed effects were then dropped sequentially from the model (using analysis of deviance tests) until all remaining terms were significant at the 5% level.
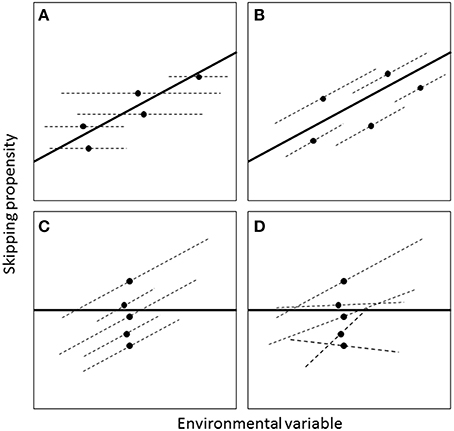
Figure 3. Four different scenarios for how the effect of an environmental variable on skipping propensity may differ at the between- and within-individual levels (adapted from van de Pol and Wright, 2009). The relationships are assumed to be linear on the transformed (e.g., logit) scale. Each panel depicts five hypothetical individuals that vary both in their mean skipping propensity (black dots) and in the environmental conditions they experience (e.g., because they are born in different years or vary in lifespan). The dark lines are regressions fitted to the black dots and represent the between-individual effect. The dashed lines are within-individuals effects. In A, there is no relationship within individuals but an effect across individuals; in B, the within- and between-individual effects are the same; in C and D, the effect is present within but not across individuals. In A–C, there is no variation in within-individual slopes, but in D there is.
Individual-level analyses: fitness consequences of skipping
A GLMM was used to test the effect of skipping in year t on breeding success in year t + 1. The response variable was binary 1 = chick raised successfully in year t + 1, 0 = failure. This analysis was restricted to cases where an egg was laid in year t + 1. “Previous status” was defined as a factor with three levels: 1 = skipped breeding in year t; 2 = bred unsuccessfully in year t; 3 = bred successfully in year t. The fixed effects were previous status, sex, years since ringing and years since ringing squared. Random effects of individual and year were included. A similar GLMM was fitted with probability of skipping in year t + 1 as the response.
Finally, we calculated a proxy for lifetime reproductive success (LRS) for individuals that had disappeared (presumed dead) before 2013. Although it is rare for individuals not to be seen for two consecutive years, individuals not seen in 2013 or 2014 were excluded from this analysis since they could still have been alive. The first 3 years of the dataset were also excluded as individuals first caught at the start of the study were likely to have bred before. This gave relatively complete breeding life histories of 660 individuals (6841 bird-year combinations). For each individual, LRS was calculated by summing the number of chicks produced over their breeding lifespan. A generalized additive model (GAM) with LRS as the Poisson response variable (log link function) was then fitted, with smoothers for the effects of breeding lifespan (number of years from first capture to disappearance) and mean skipping propensity (proportion of years skipped) using cross validation (Wood, 2006), as exploratory analyses indicated non-linear effects. A GAM was also used to test for a relationship between breeding lifespan (response variable, Poisson error and log link function) and skipping frequency. To avoid over-fitting, these GAMs were constrained to have fixed degrees of freedom and three knots (Wood, 2006). All models were fitted in R version 3.1.1 (R Core Team, 2014). GLMMs were fit using the glmer function from the lme4 package (Bates et al., 2012).
Results
Temporal Trends
The annual covariates included as candidate explanatory variables in the population-level analysis were correlated to some degree; for example, wSST and locSST were correlated with r = 0.69 (Supplementary information, Table A1). The time series for annual skipping propensity and each explanatory variable are shown in Figure 2. The best GAM for annual skipping propensity indicated there was temporal autocorrelation with a lag of 1 year (i.e., years of high skipping propensity were followed by years of high skipping propensity). When this residual autocorrelation was taken into account, the smoother for the year effect was effectively linear and not significantly different from zero (estimated df = 1, residual df = 1, F = 0.43, P = 0.52), i.e., there was no evidence for a directional trend over time. The best model for wSST indicated no temporal autocorrelation in the residuals and a marginally non-significant positive effect of year (GAM smoother: estimated df = 1, residual df = 1, F = 4.00, P = 0.054). Similarly, the best model for locSST indicated no temporal autocorrelation in the residuals and a positive effect of year (GAM smoother: estimated df = 1, residual df = 1, F = 6.06, P = 0.02). No trend (GAM smoother: estimated df = 1, residual df = 1, F = 1.20, P = 0.28) or temporal autocorrelation in the residuals was apparent for wNAO. A significant non-linear trend in population size was present over the study period (GAM smoother: estimated df = 6.33, residual df = 6.33, F = 23.38, P < 0.001) with no temporal autocorrelation in the residuals after accounting for this trend.
Population-Level Analysis
The mean annual frequency of skipping was 0.07 and ranged from 0.02 to 0.14. The annual frequency of skipping was positively associated with wSST in the same year (Figure 4A, Table 1) and in the previous year (Figure 4B, Table 1). None of the other annual covariates were associated with annual skipping frequency (Table 1).
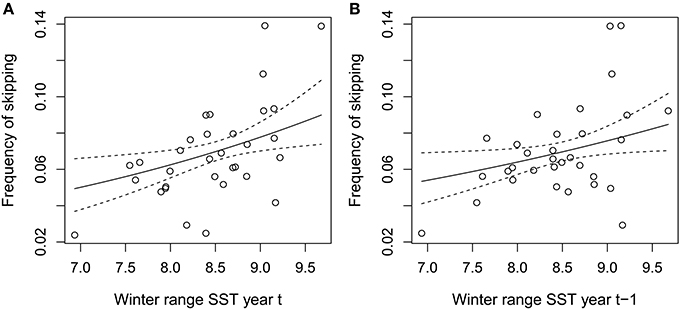
Figure 4. Annual skipping frequency as a function of (A) winter range SST in year t and (B) winter range SST in year t-1. Regression fits with confidence bands are shown (back-transformed from logit scale).
Individual-Level Analyses
The GLMM with skipping as the binary response indicated that the effect of wSST was present within individuals (effect of wSST_dev: χ2 = 9.66, df = 1, P = 0.002) but not across individuals (effect of wSST_mean: χ2 = 2.25, df = 1, P = 0.13). The estimate from the GLMM for the term wSST_dev was 0.38 ± 0.12, which compares with an overall effect of winter range SST in the population-level analysis of 0.37 ± 0.08 (Table 1), indicating that the cross-sectional relationship mirrors the underlying within-individual effect. The best random effects structure was one with uncorrelated random intercepts and slopes. A likelihood ratio test indicated that this model was better than one with just random intercepts (χ2 = 4.98, df = 1, P = 0.026), while a model with correlated random intercepts and slopes was not supported (χ2 = 1.08, df = 1, P = 0.30). Sex did not have an effect on skipping propensity (χ2 = 1.75, df = 1, P = 0.18). The overall quadratic effect of years since ringing (Figure 5) was highly significant (χ2 = 81.89, df = 2, P < 0.001), but the linear component was not significantly different from zero (χ2 = 0.002, df = 1, P = 0.96). The between-individual variance in skipping propensity (variance in intercepts) was much larger than the between-year variance (2.86 vs. 0.02, both on logit scale; variance in slopes = 0.49). These results indicate that the individual-level patterns are most consistent with scenario D in Figure 3.
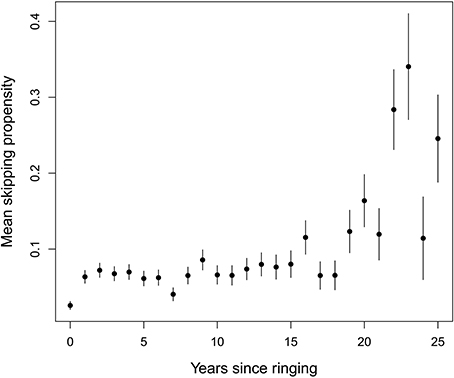
Figure 5. Mean skipping propensity as a function of years since ringing (proxy for age). Data points are means ± standard errors for each age class.
Individuals that skipped breeding in year t had lower breeding success in year t + 1 than individuals that bred in year t but failed, which in turn had lower breeding success in year t + 1 than individuals that bred successfully in year t (Figure 6A, effect of previous status: χ2 = 406.47, df = 2, P < 0.001). Sex had no effect on breeding success (χ2 = 0.15, df = 1, P = 0.70). The quadratic effect of years since ringing (our proxy for bird age) on breeding success in year t + 1 was highly significant (χ2 = 54.05, df = 2, P < 0.001), with breeding success being lower at older minimum inferred ages (Figure S1). Individuals that skipped breeding in year t had a higher probability to skip in year t + 1 than individuals that bred but failed, which in turn had a higher probability to skip in year t + 1 than individuals that bred successfully in year t (Figure 6B, effect of previous status: χ2 = 379.98, df = 2, P < 0.001), controlling for the significant quadratic effect of minimum inferred age (χ2 = 36.94, df = 2, P < 0.001).
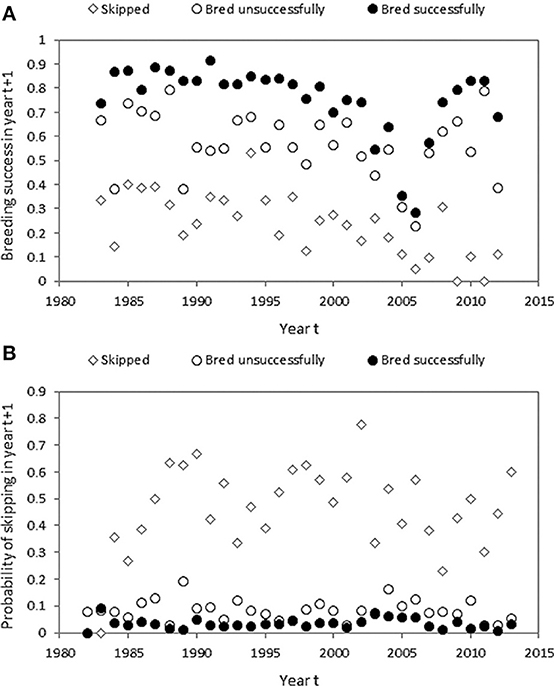
Figure 6. (A) Breeding success in year t + 1 conditional on previous status in year t. (B) Probability of skipping breeding in year t + 1 conditional on previous status in year t.
Among those individuals with complete breeding histories, those that skipped breeding more frequently produced fewer chicks on average across their breeding lifespans (Figure 7A), controlling for the fact that individuals with longer breeding lifespans had higher LRS (Figure 7B). The GAMs indicated that these effects were slightly non-linear (effect of skipping frequency: estimated df = 2.55, residual df = 3.19, χ2 = 285.6, P < 0.001; effect of breeding lifespan: estimated df = 6.11, residual df = 7.27, χ2 = 2899.9, P < 0.001; overall deviance explained = 85.1%). An asymmetric non-linear relationship between skipping frequency and breeding lifespan was found (Figure S2; estimated df = 2, residual df = 2, χ2 = 30.9, P < 0.001; deviance explained = 5.0%): individuals that skipped approximately 30% of the time had the longest breeding lifespans, while both individuals that skipped very infrequently and those skipping more frequently lived less long.
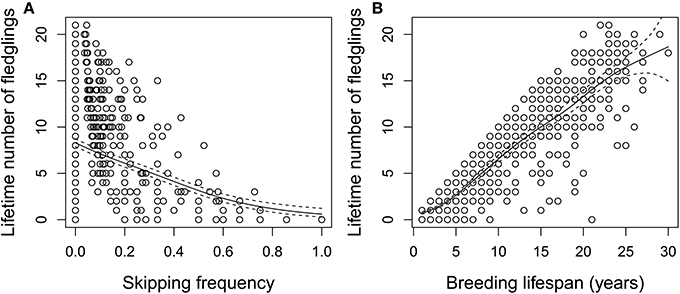
Figure 7. (A) Lifetime number of fledglings as a function of skipping frequency (fraction of breeding lifespan where adult did not breed) for individuals with complete life histories (n = 660). (B) Lifetime number of fledglings as a function of breeding lifespan for same individuals. Predicted fits with confidence bands from generalized additive model are included.
Discussion
A relatively low absolute rate of skipping was found for guillemots on the Isle of May, with only 7% of birds skipping on average across the study period. Nonetheless, skipping was more frequent in years where SST in their wintering area in the southern/western North Sea were high the preceding winter and also the one before i.e., ca.15 months prior to the breeding season. Ringing recoveries and tracking studies indicate that guillemots from the Isle of May disperse widely throughout the North Sea during the winter months, with the core of the winter distribution centering on the southern and western North Sea (Reynolds et al., 2011; Harris et al., in press). North Sea temperatures have increased gradually over the past several decades in line with rising global air temperatures, but the rates of warming observed in the southern North Sea (most of which is <50 m deep) are among the highest documented anywhere in the world (Rayner et al., 2003; Heath et al., 2012). A direct effect of higher SSTs on breeding propensity seems less likely than indirect effects mediated via changes in the food web, particularly given that lagged effects of wSST were also found. The little information available on the winter diet of guillemots indicates that many fish species are taken, in particular clupeids, gadids, and sandeels (e.g., Blake et al., 1985; Harris and Bailey, 1992; Sonntag and Hüppop, 2005). The mechanisms by which increases in wSST might filter through the marine food web to affect the breeding propensity of guillemots are unclear but recruitment rates of lesser sandeels (Ammodytes marinus), which are an important prey species for guillemots during the breeding season but may also be taken during winter months, correlate negatively with winter SSTs in the North Sea (Arnott and Ruxton, 2002). Herring (Clupea harengus) are another oil-rich small pelagic fish taken by guillemots during winter months (Blake et al., 1985; Harris and Bailey, 1992; Sonntag and Hüppop, 2005) and positive correlations between SSTs and herring recruitment and stock biomass have been reported in the Barents Sea (Hjermann et al., 2004). These findings and others indicate that changes in SST and other physical oceanographic variables can have complex food web effects (Durant et al., 2004; Frederiksen et al., 2007). The net impacts on the body condition and hence breeding propensity of piscivorous seabirds may in general be difficult to predict.
In long-lived species with low annual reproductive output population growth rate is often most sensitive to changes in adult survival and these species are expected to favor self-maintenance over reproduction when conditions are poor (Curio, 1988; Linden and Møller, 1989; Sæther and Bakke, 2000). Fitness in marine top predators may therefore be maximized by minimizing interannual variance in adult survival: so-called life-history buffering against environmental variability (Morris and Doak, 2004; Forcada et al., 2008). Recent work on Antarctic mammals and seabirds has shown that variation in annual breeding propensity can also strongly influence individual fitness and population growth (Jenouvrier et al., 2005b; Forcada et al., 2008). In the case of Antarctic fur seals Arctocephalus gazella, increased ecosystem fluctuations associated with increased climate variability since 1990 seems to have reduced their capacity for life-history buffering, as evidenced by an increasing impact of SST variation (which affects abundance and predictability of krill, a key food source for fur seals) on adult survival and breeding propensity (Forcada et al., 2008). Cubaynes et al. (2011) found that a higher fraction of red-footed boobies (Sula sula) skipped breeding in El Niño years (associated with high SSTs), which are predicted to become more frequent or intense as result of global warming (Jackson, 2008). Similarly, increases in the mean or variance of pre-breeding SSTs could lead to a loss of life-history buffering abilities in guillemots with possible consequences for population numbers and structure. On the other hand, annual rates of skipping are relatively low in this population and the breeding propensity of guillemots appears to be less sensitive to environmental fluctuations than that of European shags on the Isle of May, where up to 60% of adults may skip breeding in extremely poor years when crashes in population size also occur (Aebischer and Wanless, 1992). Cormorant species in general are prone to such periodic population crashes and rates of non-breeding are also typically highly variable (Duffy, 1983; Boekelheide and Ainley, 1989; Nur and Sydeman, 1999). Thus, population impacts of climate change mediated via changes in breeding propensity will depend on the life history strategy and ecological constraints of the species in question; some species likely have greater scope for regulating breeding effort than others.
A relationship between climate and skipping frequency was present within individuals, as evidenced by a significant effect of wSST_dev in the individual-level GLMM. No across-individual relationship was found between mean skipping propensity and wSST_mean; such a relationship could have arisen if individuals consistently spent the winter in different areas, or lived through different periods, and hence experienced warmer or cooler temperatures on average. Individuals varied in their sensitivity to wSST (as in Figure 3D) in that variation among individuals in the slope of their skipping-wSST relationship was greater than expected by chance. Such a pattern could reflect individual variation in cue sensitivity, individual differences in the extent to which the environment imposes constraints on breeding propensity, or both. We cannot distinguish definitively in this observational study whether cues or constraints are more important, but the constraints hypothesis seems more likely. First, some individuals were consistently more likely to skip breeding than others and were also more likely to breed unsuccessfully if they did breed the year after having skipped (Figure 6). Skipping tends to occur after a bird loses its breeding site to another individual and hence may be socially-induced (Harris and Wanless, 1995), but our analysis indicates that environmental factors (i.e., wSST) also play a role. One possibility is that certain individuals may be in poorer body condition following a difficult winter than others and hence be less capable of defending their nest site against incursions (fights are energetically costly) and so be evicted by a competitor. Non-breeding adult black-legged kittiwakes at a colony in Brittany (France) were shown to have lower survival rates than breeders (Cam et al., 1998) and to be more likely to skip again the following year. Our guillemot studies and the studies of Cam et al. (1998) thus suggest persistent between-individual phenotypic differences that influence a range of fitness components such as breeding propensity, breeding success and adult survival (see also Le Bohec et al., 2007; Robert et al., 2012). Understanding how such “quality” effects arise in the first place, are maintained throughout the lives of individuals and whether they are transmitted from parents to offspring constitutes a major challenge for evolutionary ecology in general (Wilson and Nussey, 2010; Bergeron et al., 2011).
Second, if individuals base their decision of whether to breed or not on predictive cues then one would expect some fitness advantage to skipping in poor years. The most obvious such advantage would be an increase in survival probability from one breeding season to the next compared to individuals that did breed, as predicted by the life history buffering hypothesis. A multi-year study of king penguins Aptenodytes patagonicus found that non-breeders and breeders had similar survival rates, but breeders were less likely to breed again the following year than non-breeders, suggesting the existence of reproductive costs and hence benefits of prudent skipping (Le Bohec et al., 2007). Cam et al. (1998), on the other hand, found that non-breeding kittiwakes survived less well than breeders, as did previous work on Isle of May guillemots (Harris and Wanless, 1995). In the current study we did not test for survival rate differences between individuals skipping vs. those breeding, but examined overall lifetime breeding success. Lifetime fledgling production declined monotonically with skipping frequency, controlling for the positive effect of breeding lifespan (Figure 7). If skipping when conditions are poor is advantageous, then one would expect a hump-shaped relationship between individual-level skipping frequency and lifetime number of fledglings, because individuals that never skip might suffer survival or future reproductive costs, while those that skip too frequently (“over-responders”) would likely also raise fewer chicks on average. We found no such optimum. Again, this points toward the constraints rather than restraints (adaptive life history buffering) hypothesis.
Third, it is difficult to imagine that environmental cues are available to individuals during the winter or early spring (when guillemots may be far away from the breeding colony) that reliably correlate with environmental factors determining the fitness consequences of breeding decisions. A string of years of poor breeding success occurred at this colony from 2004 to 2008 (Figure S3), associated with poor feeding conditions around the breeding colony (Ashbrook et al., 2010). Skipping frequency was also noticeably higher during this period (Figure 2A), which would be consistent with the restraint hypothesis, i.e., birds “chose” to skip when breeding conditions were perceived in advance to be poor. Indeed, wSST (the putative cue) is correlated negatively with annual breeding success (r = −0.51, P = 0.003) and annual skipping frequency and breeding success are also negatively correlated (r = −0.69, P < 0.001). However, correlations between wSST, skipping propensity and breeding success do not constitute strong evidence that wSST acts as a cue for adaptive restraint and could instead reflect carry-over effects of winter conditions on both skipping propensity and breeding performance of individuals that do lay an egg (e.g., mediated via changes in body condition). The costs, in terms of residual reproductive value, of breeding under poor conditions are paid after the breeding season, not during it. If these future costs depend on the environment (e.g., survival chances of breeders are lower than non-breeders in harsh winters) then one would expect flexible skipping rules to evolve, but only if environmental cues perceived prior to the breeding season predict post-breeding survival chances, or future breeding success. Rephrased in the language of adaptive plasticity theory, the environment of decision making must predict the environment of selection (Gavrilets and Scheiner, 1993). If such cues are not available or reproduction costs are independent of the environment, then selection would instead favor a fixed, environmentally-insensitive rate of skipping (i.e., flat reaction norms). Nonetheless, the notional cost of responding to a weakly predictive cue by not breeding (in terms of foregone offspring production when conditions turned out to be good) may be considerably less than the cost of making a bad decision, i.e., breeding under circumstances that turn out to be bad and hence jeopardizing one's own survival. Such asymmetry in fitness outcomes under good vs. bad conditions may favor the evolution of risk-averse strategies (Bårdsen et al., 2008), and hence flexibility in breeding propensity may be adaptive even when environmental cues are only weakly informative of future costs. Given the lack of any discernible fitness benefits of skipping in our case, however, we tentatively infer that the observed relationship with winter SST reflects (individual-specific) constraints on breeding condition, rather than adaptive restraint in response to a cue. An interesting alternative, which we cannot fully exclude, is that individuals use their own body condition (which may be affected by environmental variation) as a cue and skip breeding if they are below a certain threshold (i.e., condition-dependent decision making). If inter-individual variation in condition is large, a positive relationship between current breeding and future breeding (and hence LRS) may be found, because those individuals that are in good condition breed now and are successful again later. Those skipping, however, may still have made an adaptive choice under their specific circumstances (particularly if poor body condition is associated with higher post-breeding mortality risk) and might have had even lower LRS if they had instead bred. The fact that individuals that never skipped had shorter lifespans (see Figure S2; i.e., breeding lifespan was maximal at a skipping frequency of approximately 30%) is consistent with this hypothesis. It remains possible, therefore, that differences between studies in the apparent adaptive benefit of skipping could reflect differing amounts of inter-individual heterogeneity in body condition (or other physiological cues) in different study systems.
Finally, skipping became more frequent in very old individuals (those with a minimum inferred age of circa 15 or more, Figure 5). Increased frequency of skipping in the oldest age classes has also been reported in great skuas Catharacta skua (Catry et al., 1998), European shags (Harris et al., 1998) and short-tailed shearwaters Puffinus tenuirostris (Bradley et al., 2000). These patterns collectively point toward reproductive senescence effects in long-lived seabirds and clearly warrant further investigation.
Limitations of the Approach
In this study we made a number of simplifying assumptions that allowed us to analyze skipping events as a binary variable in a GLMM framework. First, we assumed that known individuals were detected during the breeding season in our study area (if present) with a probability of 1.00. That is, if a ringed bird returned to the breeding colony in a given year we were 100% confident that we would indeed detect it as a breeder or a non-breeder. All breeding pairs are monitored intensively in our study plots throughout the breeding season and non-breeding individuals are easy to resight from the hides as they simply linger around the study area without a breeding site. While it is remains possible that birds could come ashore elsewhere in the colony (and go undetected) and then return to the study area in the future, we have no record of this in 30 years of intensive searching for ringed birds. Moreover, it is extremely unlikely that any of our ringed birds not resighted in a given year bred on a different island or on the mainland since, as far as we are aware, there is no record of a guillemot changing colony once it has bred. Thus, we can be confident that our estimates of skipping frequency are not biased substantially by true detection probabilities that could be slightly lower than 1.00. Nevertheless, we acknowledge that our study system may be unusual in this regard (in that both breeders and non-breeders present at the colony can be resighted easily) and in many other systems (e.g., nocturnal burrow-nesters) detection probabilities will be much lower. In such cases, our GLMM approach should not be applied as failing to account for variation in detection probability (and possible effects of covariates on this) could result in severely biased estimates of skipping frequencies and incorrect inferences regarding the effects of covariates. A multi-event modeling framework (e.g., Cam et al., 1998; Forcada et al., 2008; Cubaynes et al., 2011; Sanz-Aguilar et al., 2011) would be more appropriate in these circumstances, although it would be interesting to test the relative performance of both techniques in a simulation study to elucidate the extent of such biases.
A second major assumption we made was that skipping events were defined conditional on future resighting: if an individual was missing in year t and never seen again, this was not classed as a skipping event. This means that skipping frequency may have been slightly under-estimated, especially in the last 2 years of the study, as some of these individuals might have actually been alive in year t but did not return to breed. Adult survival rates would then also be slightly underestimated, depending on the prevalence of skipping in the final year of life, for the same reason. In this study we used resighting histories to estimate skipping rates but not adult survival rates, but we acknowledge the above biases. While these should not have had a strong influence on our findings, such biases could be larger in other study systems and we again advise caution in applying the GLMM approach in cases where detection probabilities are lower or where additional information is unavailable (e.g., non-breeders cannot be observed or the occupancy of previous nests of non-breeders cannot be confirmed). Statistical inference and model selection with GLMMs are constantly being refined (e.g., Bolker et al., 2009; Link and Barker, 2009). As always, GLMMs should be applied cautiously, particularly when P-values are marginal and further confidence in findings will be instilled when different approaches (e.g., forwards vs. backwards selection) produce the same answers.
Finally, we acknowledge that correlative studies are by their nature limited and experimental studies are required to rigorously test whether skipped breeding is adaptive or not, i.e., individuals must be “moved” experimentally from their hypothesized adaptive optimum to quantify the fitness consequences of breeding decisions. This is very challenging in the case of skipped breeding as skippers would need to be forced to breed without altering other aspects of phenotype (or environment) that might influence the fitness outcome. Correlative studies, especially those that use long-term and information-rich datasets, nonetheless provide good information on possible trade-offs because in some years, or for some groups of individuals, extreme environmental conditions might indeed push individuals to make non-optimal decisions.
In conclusion, we have documented a clear link between skipped breeding in common guillemots and climate that suggests that skipping may become more frequent in a warmer, or more climatically variable, future. While we cannot definitely say whether skipping reflects an adaptive response to predictive cues or environmental constraints on breeding propensity, our results collectively point more toward the latter, particularly given that the lifetime reproductive output of individuals declined as a function of the fraction of years skipped.
Conflict of Interest Statement
The authors declare that the research was conducted in the absence of any commercial or financial relationships that could be construed as a potential conflict of interest.
Acknowledgments
We thank Sarah Burthe for advice on obtaining and extracting SST data, Francis Daunt for useful discussions, the many people who have helped collect the guillemot data, particularly Mark Newell and Carrie Gunn, Scottish Natural Heritage for permission to work on the Isle of May National Nature Reserve and our two reviewers for helpful comments. Funding for Sarah Wanless and Mike P. Harris was provided by the Natural Environment Research Council and the Joint Nature Conservancy Council. Thomas E. Reed was supported by the Beaufort Marine Research Award in Fish Population Genetics funded by the Irish Government under the Sea Change Programme.
Supplementary Material
The Supplementary Material for this article can be found online at: http://www.frontiersin.org/journal/10.3389/fevo.2015.00001/abstract
Figure S1. Breeding success in year t + 1 as a function of years since ringing (proxy for age) in year t.
Figure S2. Breeding lifespan as function of mean skipping frequency at the individual level. Predicted fits with confidence bands from generalized additive model are included. Data were grouped for ease of plotting into 14 classes along the x-axis, but the GAM fit is based on the raw (unbinned) data.
Figure S3. Annual mean breeding success (fraction of egg layers that successfully raised a chick) as a function of year.
References
Aebischer, N. J., and Wanless, S. (1992). Relationships between colony size, adult non-breeding and environmental conditions for Shags Phalacrocorax aristotelis on the Isle of May, Scotland. Bird Study 39, 43–52. doi: 10.1080/00063659209477098
Arnott, S. A., and Ruxton, G. D. (2002). Sandeel recruitment in the North Sea: demographic, climatic and trophic effects. Mar. Ecol. Prog. Ser. 238, 199–210. doi: 10.3354/meps238199
Ashbrook, K., Wanless, S., Harris, M. P., and Hamer, K. C. (2010). Impacts of poor food availability on positive density dependence in a highly colonial seabird. Proc. R. Soc. B Biol. Sci. 277, 2355–2360. doi: 10.1098/rspb.2010.0352
Pubmed Abstract | Pubmed Full Text | CrossRef Full Text | Google Scholar
Bårdsen, B. J., Fauchald, P., Tveraa, T., Langeland, K., Yoccoz, N. G., and Ims, R. A. (2008). Experimental evidence of a risk-sensitive reproductive allocation in a long-lived mammal. Ecology 89, 829–837. doi: 10.1890/07-0414.1
Pubmed Abstract | Pubmed Full Text | CrossRef Full Text | Google Scholar
Bårdsen, B. J., Henden, J. A., Fauchald, P., Tveraa, T., and Stien, A. (2011). Plastic reproductive allocation as a buffer against environmental stochasticity–linking life history and population dynamics to climate. Oikos 120, 245–257. doi: 10.1111/j.1600-0706.2010.18597.x
Bates, D., Maechler, M., and Bolker, B. (2012). Lme4: Linear Mixed-Effects Models Using S4 Classes. R Package Version 0.999999-0. Available online at: http://CRANR-project.org/package=lme4
Bergeron, P., Baeta, R., Pelletier, F., Réale, D., and Garant, D. (2011). Individual quality: tautology or biological reality? J. Anim. Ecol. 80, 361–364. doi: 10.1111/j.1365-2656.2010.01770.x
Pubmed Abstract | Pubmed Full Text | CrossRef Full Text | Google Scholar
Blake, B. F., Dixon, T. J., Jones, P. H., and Tasker, M. L. (1985). Seasonal changes in the feeding ecology of guillemots Uria aalge off north and east Scotland. Estuar. Coast. Shelf Sci. 20, 559–568. doi: 10.1016/0272-7714(85)90107-6
Boekelheide, R. J., and Ainley, D. G. (1989). Age, resource availability, and breeding effort in Brandt's Cormorant. Auk 106, 389–401.
Bolker, B. M., Brooks, M. E., Clark, C. J., Geange, S. W., Poulsen, J. R., Stevens, M. H. H., et al. (2009). Generalized linear mixed models: a practical guide for ecology and evolution. Trends Ecol. Evol. 24, 127–135. doi: 10.1016/j.tree.2008.10.008
Pubmed Abstract | Pubmed Full Text | CrossRef Full Text | Google Scholar
Bradley, J. S., Wooller, R. D., and Skira, I. J. (2000). Intermittent breeding in the short-tailed shearwater Puffinus tenuirostris. J. Anim. Ecol. 69, 639–650. doi: 10.1046/j.1365-2656.2000.00422.x
Bull, J. J., and Shine, R. (1979). Iteroparous animals that skip opportunities for reproduction. Am. Nat. 114, 296–303. doi: 10.1086/283476
Cam, E., Hines, J. E., Monnat, J.-Y., Nichols, J. D., and Danchin, E. (1998). Are adult nonbreeders prudent parents? The kittiwake model. Ecology 79, 2917–2930. doi: 10.1890/0012-9658(1998)079[2917:AANPPT]2.0.CO;2
Catry, P., Phillips, R. A., Hamer, K. C., Ratcliffe, N., and Furness, R. W. (1998). The incidence of nonbreeding by adult great skuas and parasitic jaegers from Foula, Shetland. Condor 100, 448–455. doi: 10.2307/1369710
Charlesworth, B. (1994). Evolution in Age-Structured Populations. Cambridge: Cambridge University Press. doi: 10.1017/CBO9780511525711
Chastel, O. (1995). Influence of reproductive success on breeding frequency in four southern petrels. Ibis 137, 360–363. doi: 10.1111/j.1474-919X.1995.tb08033.x
Clutton-Brock, T. H. (1988). Reproductive Success: Studies of Individual Variation in Contrasting Breeding Systems. Chicago: University of Chicago Press.
Coulson, J. C. (1984). The population dynamics of the eider duck Somateria mollissima and evidence of extensive non-breeding by adult ducks. Ibis 126, 525–543. doi: 10.1111/j.1474-919X.1984.tb02078.x
Crespin, L., Harris, M. P., Lebreton, J.-D., and Wanless, S. (2006). Increased adult mortality and reduced breeding success with age in a population of common guillemot Uria aalge using marked birds of unknown age. J. Avian Biol. 37, 273–282. doi: 10.1111/j.0908-8857.2006.03495.x
Cubaynes, S., Doherty, P. F. Jr., Schreiber, E. A., and Gimenez, O. (2011). To breed or not to breed: a seabird's response to extreme climatic events. Biol. Lett. 7, 303–306. doi: 10.1098/rsbl.2010.0778
Pubmed Abstract | Pubmed Full Text | CrossRef Full Text | Google Scholar
Curio, E. (1988). Relative realized life span and delayed cost of parental care. Am. Nat. 131, 825–836. doi: 10.1086/284824
Danchin, E., and Cam, E. (2002). Can non-breeding be a cost of breeding dispersal? Behav. Ecol. Sociobiol. 51, 153–163. doi: 10.1007/s00265-001-0423-5
Duffy, D. C. (1983). Environmental uncertainty and commercial fishing: effects on Peruvian guano birds. Biol. Conserv. 26, 227–238. doi: 10.1016/0006-3207(83)90075-7
Durant, J. M., Stenseth, N. C., Anker-Nilssen, T., Harris, M. P., Thompson, P. M., and Wanless, S. (2004). Marine birds and climate fluctuation in the North Atlantic. Mar. Ecosyst. Clim. Var. N. Atl. 95–105. doi: 10.1093/acprof:oso/9780198507499.003.0007
Pubmed Abstract | Pubmed Full Text | CrossRef Full Text | Google Scholar
Erikstad, K. E., Asheim, M., Fauchald, P., Dahlhaug, L., Tveraa, T., and Dahlhaug, P. (1997). Adjustment of parental effort in the puffin; the roles of adult body condition and chick size. Behav. Ecol. Sociobiol. 40, 95–100. doi: 10.1007/s002650050320
Erikstad, K. E., Fauchald, P., Tveraa, T., and Steen, H. (1998). On the cost of reproduction in long-lived birds: the influence of environmental variability. Ecology 79, 1781–1788. doi: 10.1890/0012-9658(1998)079[1781:OTCORI]2.0.CO;2
Forcada, J., Trathan, P. N., and Murphy, E. J. (2008). Life history buffering in Antarctic mammals and birds against changing patterns of climate and environmental variation. Glob. Change Biol. 14, 2473–2488. doi: 10.1111/j.1365-2486.2008.01678.x
Frederiksen, M., Edwards, M., Richardson, A. J., Halliday, N. C., and Wanless, S. (2006). From plankton to top predators: bottom-up control of a marine food web across four trophic levels. J. Anim. Ecol. 75, 1259–1268. doi: 10.1111/j.1365-2656.2006.01148.x
Pubmed Abstract | Pubmed Full Text | CrossRef Full Text | Google Scholar
Frederiksen, M., Furness, R. W., and Wanless, S. (2007). Regional variation in the role of bottom-up and top-down processes in controlling sandeel abundance in the North Sea. Mar. Ecol. Prog. Ser. 337, 279–286. doi: 10.3354/meps337279
Frederiksen, M., Harris, M. P., Daunt, F., Rothery, P., and Wanless, S. (2004a). Scale-dependent climate signals drive breeding phenology of three seabird species. Glob. Change Biol. 10, 1214–1221. doi: 10.1111/j.1529-8817.2003.00794.x
Frederiksen, M., Wanless, S., Harris, M. P., Rothery, P., and Wilson, L. J. (2004b). The role of industrial fisheries and oceanographic change in the decline of North Sea black-legged kittiwakes. J. Appl. Ecol. 41, 1129–1139. doi: 10.1111/j.0021-8901.2004.00966.x
Gaston, A. J., Jones, I. L., and Lewington, I. (1998). The Auks: Alcidae. Oxford: Oxford University Press.
Gavrilets, S., and Scheiner, S. M. (1993). The genetics of phenotypic plasticity. V. Evolution of reaction norm shape. J. Evol. Biol. 6, 31–48. doi: 10.1046/j.1420-9101.1993.6010031.x
Grosbois, V., and Thompson, P. M. (2005). North Atlantic climate variation influences survival in adult fulmars. Oikos 109, 273–290. doi: 10.1111/j.0030-1299.2005.13774.x
Harris, M. P., and Bailey, R. S. (1992). Mortality rates of puffin Fratercula arctica and guillemot Uria aalge and fish abundance in the North Sea. Biol. Conserv. 60, 39–46. doi: 10.1016/0006-3207(92)90797-Q
Harris, M. P., and Birkhead, T. R. (1985). “Breeding ecology of the Atlantic Alcidae,” in The Atlantic Alcidae, eds D. N. Nettleship and T. R. Birkhead (London: Academic Press), 155–204.
Harris, M. P., and Wanless, S. (1988). The breeding biology of guillemots Uria aalge on the Isle of May over a six year period. Ibis 130, 172–192. doi: 10.1111/j.1474-919X.1988.tb00969.x
Harris, M. P., and Wanless, S. (1989). Fall colony attendance and breeding success in the common murre. Condor 91, 139–146. doi: 10.2307/1368157
Harris, M. P., and Wanless, S. (1990). Breeding status and sex of common murres (Uria aalge) at a colony in autumn. Auk 107, 603–605.
Harris, M. P., and Wanless, S. (1995). Survival and non-breeding of adult Common Guillemots Uria aalge. Ibis 137, 192–197. doi: 10.1111/j.1474-919X.1995.tb03239.x
Pubmed Abstract | Pubmed Full Text | CrossRef Full Text | Google Scholar
Harris, M. P., Wanless, S., Ballesteros, M., Moe, B., Daunt, F., and Erikstad, K. E. (in press). Geolocators reveal an unsuspected moulting area for Isle of May Common Guillemots Uria aalge. Bird Study.
Harris, M. P., Wanless, S., and Elston, D. A. (1998). Age-related effects of a nonbreeding event and a winter wreck on the survival of Shags Phalacrocorax aristotelis. Ibis 140, 310–314. doi: 10.1111/j.1474-919X.1998.tb04394.x
Harshman, L. G., and Zera, A. J. (2007). The cost of reproduction: the devil in the details. Trends Ecol. Evol. 22, 80–86. doi: 10.1016/j.tree.2006.10.008
Pubmed Abstract | Pubmed Full Text | CrossRef Full Text | Google Scholar
Heath, M. R., Neat, F. C., Pinnegar, J. K., Reid, D. G., Sims, D. W., and Wright, P. J. (2012). Review of climate change impacts on marine fish and shellfish around the UK and Ireland. Aquat. Conserv. Mar. Freshw. Ecosyst. 22, 337–367. doi: 10.1002/aqc.2244
Hjermann, D. Ø., Stenseth, N. C., and Ottersen, G. (2004). Indirect climatic forcing of the Barents Sea capelin: a cohort effect. Mar. Ecol. Prog. Ser. 273, 229–238. doi: 10.3354/meps273229
Hurrell, J. W. (2014). The Climate Data Guide: Hurrell North Atlantic Oscillation (NAO) Index (station-based). Available online at: https://climatedataguide.ucar.edu/climate-data/hurrell-north-atlantic-oscillation-nao-index-station-based (Accessed July 1, 2014).
Jackson, J. B. (2008). Ecological extinction and evolution in the brave new ocean. Proc. Natl. Acad. Sci. U.S.A. 105, 11458–11465. doi: 10.1073/pnas.0802812105
Pubmed Abstract | Pubmed Full Text | CrossRef Full Text | Google Scholar
Jenouvrier, S., Barbraud, C., Cazelles, B., and Weimerskirch, H. (2005a). Modelling population dynamics of seabirds: importance of the effects of climate fluctuations on breeding proportions. Oikos 108, 511–522. doi: 10.1111/j.0030-1299.2005.13351.x
Jenouvrier, S., Barbraud, C., and Weimerskirch, H. (2005b). Long-term contrasted responses to climate of two Antarctic seabird species. Ecology 86, 2889–2903. doi: 10.1890/05-0514
Pubmed Abstract | Pubmed Full Text | CrossRef Full Text | Google Scholar
Jeschke, J. M., Wanless, S., Harris, M. P., and Kokko, H. (2007). How partnerships end in guillemots Uria aalge: chance events, adaptive change, or forced divorce? Behav. Ecol. 18, 460–466. doi: 10.1093/beheco/arl109
Johnston, F. D., and Post, J. R. (2009). Density-dependent life-history compensation of an iteroparous salmonid. Ecol. Appl. 19, 449–467. doi: 10.1890/07-1507.1
Pubmed Abstract | Pubmed Full Text | CrossRef Full Text | Google Scholar
Jouventin, P., and Dobson, F. S. (2002). Why breed every other year? The case of albatrosses. Proc. R. Soc. Lond. B Biol. Sci. 269, 1955–1961. doi: 10.1098/rspb.2002.2080
Pubmed Abstract | Pubmed Full Text | CrossRef Full Text | Google Scholar
Kokko, H., Harris, M. P., and Wanless, S. (2004). Competition for breeding sites and site-dependent population regulation in a highly colonial seabird, the common guillemot Uria aalge. J. Anim. Ecol. 73, 367–376. doi: 10.1111/j.0021-8790.2004.00813.x
Lahoz-Monfort, J. J., Morgan, B. J., Harris, M. P., Daunt, F., Wanless, S., and Freeman, S. N. (2013). Breeding together: modeling synchrony in productivity in a seabird community. Ecology 94, 3–10. doi: 10.1890/12-0500.1
Pubmed Abstract | Pubmed Full Text | CrossRef Full Text | Google Scholar
Le Bohec, C. L., Gauthier-Clerc, M., Grémillet, D., Pradel, R., Béchet, A., Gendner, J. P., et al. (2007). Population dynamics in a long-lived seabird: I. Impact of breeding activity on survival and breeding probability in unbanded king penguins. J. Anim. Ecol. 76, 1149–1160. doi: 10.1111/j.1365-2656.2007.01268.x
Pubmed Abstract | Pubmed Full Text | CrossRef Full Text | Google Scholar
Lewis, S., Elston, D. A., Daunt, F., Cheney, B., and Thompson, P. M. (2009). Effects of extrinsic and intrinsic factors on breeding success in a long lived seabird. Oikos 118, 521–528. doi: 10.1111/j.1600-0706.2008.17308.x
Linden, M., and Møller, A. P. (1989). Cost of reproduction and covariation of life history traits in birds. Trends Ecol. Evol. 4, 367–371. doi: 10.1016/0169-5347(89)90101-8
Pubmed Abstract | Pubmed Full Text | CrossRef Full Text | Google Scholar
Link, W. A., and Barker, R. J. (2009). Bayesian Inference: With Ecological Applications. London: Academic Press.
McNamara, J. M., and Houston, A. I. (2008). Optimal annual routines: behaviour in the context of physiology and ecology. Philos. Trans. R. Soc. B Biol. Sci. 363, 301–319. doi: 10.1098/rstb.2007.2141
Pubmed Abstract | Pubmed Full Text | CrossRef Full Text | Google Scholar
Meijer, T., and Drent, R. (1999). Re-examination of the capital and income dichotomy in breeding birds. Ibis 141, 399–414. doi: 10.1111/j.1474-919X.1999.tb04409.x
Mills, J. A. (1989). “Red-billed gull,” in Lifetime Reproduction in Birds, ed I. Newton (London: Academic Press), 387–404.
Mills, J. A., Yarrall, J. W., Bradford-Grieve, J. M., Uddstrom, M. J., Renwick, J. A., and Merilä, J. (2008). The impact of climate fluctuation on food availability and reproductive performance of the planktivorous red-billed gull Larus novaehollandiae scopulinus. J. Anim. Ecol. 77, 1129–1142. doi: 10.1111/j.1365-2656.2008.01383.x
Pubmed Abstract | Pubmed Full Text | CrossRef Full Text | Google Scholar
Monaghan, P., Metcalfe, N. B., and Torres, R. (2009). Oxidative stress as a mediator of life history trade-offs: mechanisms, measurements and interpretation. Ecol. Lett. 12, 75–92. doi: 10.1111/j.1461-0248.2008.01258.x
Pubmed Abstract | Pubmed Full Text | CrossRef Full Text | Google Scholar
Moreno, J. (2003). Lifetime reproductive success in seabirds: interindividual differences and implications for conservation. Sci. Mar. 67, 7–12.
Morris, W. F., and Doak, D. F. (2004). Buffering of life histories against environmental stochasticity: accounting for a spurious correlation between the variabilities of vital rates and their contributions to fitness. Am. Nat. 163, 579–590. doi: 10.1086/382550
Pubmed Abstract | Pubmed Full Text | CrossRef Full Text | Google Scholar
Nur, N., and Sydeman, W. J. (1999). Survival, breeding probability and reproductive success in relation to population dynamics of Brandt's Cormorants Phalacrocorax penicillatus. Bird Study 46, S92–S103. doi: 10.1080/00063659909477236
Rahmstorf, S., and Coumou, D. (2011). Increase of extreme events in a warming world. Proc. Natl. Acad. Sci. U.S.A. 108, 17905–17909. doi: 10.1073/pnas.1101766108
Pubmed Abstract | Pubmed Full Text | CrossRef Full Text | Google Scholar
Rayner, N. A., Parker, D. E., Horton, E. B., Folland, C. K., Alexander, L. V., Rowell, D. P., et al. (2003). Global analyses of sea surface temperature, sea ice, and night marine air temperature since the late nineteenth century. J. Geophys. Res. Atmospheres 108, 1984–2012. doi: 10.1029/2002JD002670
R Core Team. (2014). R: A Language and Environment for Statistical Computing. Vienna, Austria: R Foundation for Statistical Computing. Available online at: http://www.R-project.org/
Reed, E. T., Gauthier, G., and Giroux, F. (2004). Effects of spring conditions on breeding propensity of greater snow goose females. Anim. Biodivers. Conserv. 27, 35–46.
Reed, T. E., Kruuk, L. E., Wanless, S., Frederiksen, M., Cunningham, E. J., and Harris, M. P. (2008). Reproductive senescence in a long-lived seabird: rates of decline in late-life performance are associated with varying costs of early reproduction. Am. Nat. 171, E89–E101. doi: 10.1086/524957
Pubmed Abstract | Pubmed Full Text | CrossRef Full Text | Google Scholar
Reed, T. E., Wanless, S., Harris, M. P., Frederiksen, M., Kruuk, L. E., and Cunningham, E. J. (2006). Responding to environmental change: plastic responses vary little in a synchronous breeder. Proc. R. Soc. B Biol. Sci. 273, 2713–2719. doi: 10.1098/rspb.2006.3631
Pubmed Abstract | Pubmed Full Text | CrossRef Full Text | Google Scholar
Reed, T. E., Warzybok, P., Wilson, A. J., Bradley, R. W., Wanless, S., and Sydeman, W. J. (2009). Timing is everything: flexible phenology and shifting selection in a colonial seabird. J. Anim. Ecol. 78, 376–387. doi: 10.1111/j.1365-2656.2008.01503.x
Pubmed Abstract | Pubmed Full Text | CrossRef Full Text | Google Scholar
Reid, J. B., Becker, P. H., and Furness, R. W. (1999). “Evidence for decadal scale variations in seabird population ecology and links with the North Atlantic Oscillation,” in Diets of Seabirds and Consequences of Changes in Food Supply, eds R. W. Furness and M. L. Tasker (København: International Council for the Exploration of the Sea), 47–50.
Reynolds, T. J., Harris, M. P., King, R., Swann, R. L., Jardine, D. C., Frederiksen, M., et al. (2011). Among-colony synchrony in the survival of common guillemots Uria aalge reflects shared wintering areas. Ibis 153, 818–831. doi: 10.1111/j.1474-919X.2011.01149.x
Reynolds, T. J., King, R., Harwood, J., Frederiksen, M., Harris, M. P., and Wanless, S. (2009). Integrated data analysis in the presence of emigration and mark loss. J. Agric. Biol. Environ. Stat. 14, 411–431. doi: 10.1198/jabes.2009.08008
Rideout, R. M., and Tomkiewicz, J. (2011). Skipped spawning in fishes: more common than you might think. Mar. Coast. Fish. 3, 176–189. doi: 10.1080/19425120.2011.556943
Robert, A., Paiva, V. H., Bolton, M., Jiguet, F., and Bried, J. (2012). The interaction between reproductive cost and individual quality is mediated by oceanic conditions in a long-lived bird. Ecology 93, 1944–1952. doi: 10.1890/11-1840.1
Pubmed Abstract | Pubmed Full Text | CrossRef Full Text | Google Scholar
Sæther, B.-E., and Bakke, Ø. (2000). Avian life history variation and contribution of demographic traits to the population growth rate. Ecology 81, 642–653. doi: 10.1890/0012-9658(2000)081[0642:ALHVAC]2.0.CO;2
Sandvik, H., Coulson, T. I. M., and Sæther, B.-E. (2008). A latitudinal gradient in climate effects on seabird demography: results from interspecific analyses. Glob. Change Biol. 14, 703–713. doi: 10.1111/j.1365-2486.2007.01533.x
Sandvik, H., Erikstad, K. E., Barrett, R. T., and Yoccoz, N. G. (2005). The effect of climate on adult survival in five species of North Atlantic seabirds. J. Anim. Ecol. 74, 817–831. doi: 10.1111/j.1365-2656.2005.00981.x
Sandvik, H., Erikstad, K. E., and Sæther, B.-E. (2012). Climate affects seabird population dynamics both via reproduction and adult survival. Mar. Ecol. Prog. Ser. 454, 273–284. doi: 10.3354/meps09558
Sanz-Aguilar, A., Tavecchia, G., Genovart, M., Igual, J. M., Oro, D., Rouan, L., et al. (2011). Studying the reproductive skipping behavior in long-lived birds by adding nest inspection to individual-based data. Ecol. Appl. 21, 555–564. doi: 10.1890/09-2339.1
Pubmed Abstract | Pubmed Full Text | CrossRef Full Text | Google Scholar
Schaffer, W. M. (1974). Optimal reproductive effort in fluctuating environments. Am. Nat. 108, 783–790. doi: 10.1086/282954
Pubmed Abstract | Pubmed Full Text | CrossRef Full Text | Google Scholar
Seager, R., Naik, N., and Vogel, L. (2012). Does global warming cause intensified interannual hydroclimate variability? J. Clim. 25, 3355–3372. doi: 10.1175/JCLI-D-11-00363.1
Solomon, S. (2007). Climate Change 2007-The Physical Science Basis: Working group I Contribution to the Fourth Assessment Report of the IPCC. Cambridge: Cambridge University Press.
Sonntag, N., and Hüppop, O. (2005). Snacks from the depth: summer and winter diet of common guillemots Uria aalge around the Island of Helgoland. Atl. Seab. 7, 1–14.
Stearns, S. C. (1989). Trade-offs in life-history evolution. Funct. Ecol. 3, 259–268. doi: 10.2307/2389364
van de Pol, M., and Wright, J. (2009). A simple method for distinguishing within-versus between-subject effects using mixed models. Anim. Behav. 77, 753–758. doi: 10.1016/j.anbehav.2008.11.006
Votier, S. C., Birkhead, T. R., Oro, D., Trinder, M., Grantham, M. J., Clark, J. A., et al. (2008). Recruitment and survival of immature seabirds in relation to oil spills and climate variability. J. Anim. Ecol. 77, 974–983. doi: 10.1111/j.1365-2656.2008.01421.x
Pubmed Abstract | Pubmed Full Text | CrossRef Full Text | Google Scholar
Williams, G. C. (1966). Natural selection, the costs of reproduction, and a refinement of Lack's principle. Am. Nat. 100, 687–690. doi: 10.1086/282461
Wilson, A. J., and Nussey, D. H. (2010). What is individual quality? An evolutionary perspective. Trends Ecol. Evol. 25, 207–214. doi: 10.1016/j.tree.2009.10.002
Pubmed Abstract | Pubmed Full Text | CrossRef Full Text | Google Scholar
Keywords: phenotypic plasticity, intermittent breeding, non-breeding, non-breeders, life history buffering, environmental cue
Citation: Reed TE, Harris MP and Wanless S (2015) Skipped breeding in common guillemots in a changing climate: restraint or constraint? Front. Ecol. Evol. 3:1. doi: 10.3389/fevo.2015.00001
Received: 13 November 2014; Accepted: 03 January 2015;
Published online: 29 January 2015.
Edited by:
Morten Frederiksen, Aarhus University, DenmarkReviewed by:
Res Altwegg, Univerity of Cape Town, South AfricaGiacomo Tavecchia, Consejo Superior de Investigaciones Científicas, Spain
Copyright © 2015 Reed, Harris and Wanless. This is an open-access article distributed under the terms of the Creative Commons Attribution License (CC BY). The use, distribution or reproduction in other forums is permitted, provided the original author(s) or licensor are credited and that the original publication in this journal is cited, in accordance with accepted academic practice. No use, distribution or reproduction is permitted which does not comply with these terms.
*Correspondence: Thomas E. Reed, School of Biological, Earth and Environmental Sciences, University College Cork, Western Road, Cork, Ireland e-mail:dHJlZWRAdWNjLmll