- Área de Botánica, Departamento de Biología, Centro Andaluz Superior de Estudios Marinos, Universidad de Cádiz, Cadiz, Spain
A commentary on
Bayesian state-space modeling of metapopulation dynamics in the Glanville fritillary butterfly
by Harrison, P. J., Hanski, I., and Ovaskainen, O. (2011). Ecol. Monog. 81, 581–598. doi: 10.1890/11-0192.1
“Our truth is the intersection of independent lies”
The strategy of scientific model building is the strategy of abstraction. An abstraction is devised to explain the workings of certain mechanistic principles and, as subjective constructs they are neither right nor wrong. Rather, abstractions obscure or enlighten the process under study, yielding useless or useful models (Levins, 2006). Two opposing approaches are usually followed in the process of ecological model construction as a route to understanding (Evans et al., 2013). In the first one, a model is worked out from theoretical principles and a set of testable predictions are then confronted with data from real systems. In the second approach, it is the detailed knowledge of the natural history of a particular system what drives the level of complexity of a mechanistic model used to fit empirical data, and this model is further used to reproduce the dynamics of the original system and, ideally, of other systems. Given the current challenges in the study of population dynamics (Oro, 2013), which abstraction is more useful?
The paper by Harrison et al. (2011) is a salient example of the second approach. Using 17-year time series data from a metapopulation of the Glanville fritillary butterfly (Melitaea cinxia Figure 1) inhabiting 72 meadows in Finland, they fit a mechanistic Individual-Based Model (IBM) to spatial biannual counts of larval groups. With the aim of assessing the desirability of complex models with respect to simpler ones in explaining the observed metapopulation dynamics, they also derived a simpler, Stochastic Patch Occupancy Model (SPOM), and fit it to the presence or absence of local populations across time and space. Interestingly, the overall fitting of both models was rather similar, and the bold result is the same with either model: variation in habitat quality influenced patch occupancy mainly through the effects on movement behavior at patch edges. The question immediately arises: does it pay to have expensive, individual-based data if cheap, qualitative population data does a great job with respect to the original question?
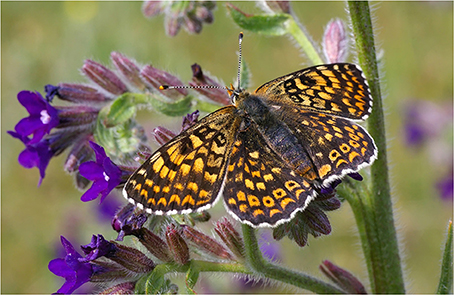
Figure 1. The Glanville fritillary butterfly (Melitaea cinxia). Author: Christian Fischer (CC BY-SA 3.0).
Building models of increasing complexity invariably involve a tradeoff between generality, precision and realism (Levins, 1966; Reiners and Lockwood, 2010; Evans et al., 2013). What the modeling strategy of Harrison et al. (2011) demonstrates, however, is that clear-cut definitions of simplicity or generality does not really exist (Evans et al., 2013). Their modeling strategy simultaneously holds several layers of simplicity and complexity: although the ecological mechanism of dispersal as a factor connecting a set of spatially distributed populations is relatively simple (Hanski, 1999), the mathematics underpinning models construction is rather complex. Furthermore, in line with state-of-the-art ecological modeling strategies (Lavine, 2010) their Bayesian state-space formulation involved the simultaneous statistical estimation of hundreds of parameters, some of them unidentifiable, and the authors acknowledged that a considerable amount of time was invested in the refining and fine-tuning of the fitting scheme.
So, where does this effort led them? Well, surprisingly, even though their approach is based on a sound knowledge on the biology and natural history of the Glanville fritillary butterfly accumulated during the past two decades, using independent data both the IBM and the SPOM consistently overestimated the probability of patch occupancy across the landscape. There's another significant lesson here. The Popperian mantra “We learn from our errors” is at the core of current philosophy of science (e.g., Mayo, 1996; Reiners and Lockwood, 2010). In simple terms, it means that more knowledge can be obtained from the failures of a perspective than from its success. The fact that both the IBM and the SPOM agree not only on their predictions but also on their mispredictions is an important one, as it suggests a fundamental mismatch between model architectures and reality: key ecological ingredients are yet missing. The reasons for this mismatch are not clear, but it points to areas were further model improvements are evident. Framed in the strategy of learning by failing, the models by Harrison et al. (2011) are thus neither right nor wrong: they are fundamentally useful.
In summary, the paper by Harrison et al. (2011) is a brave work. It is a leading exercise on the development of a sophisticated mathematical language firmly rooted in a sound ecological knowledge. For more than 20 years now, the Glanville fritillary butterfly project has been yielding fundamental lessons on how to construct a particular ecological narrative through the use of an elegant blend of mathematical modeling and question-oriented fieldwork (Hanski, 1999). It is a nice example of how the strategy of building complex models in ecology can be seen as the first step in the strategy of constructing simpler, yet effective ones. This journey from simple to complex models and back again to simpler ones, driven by bold questions, is likely the way to go for ecological research in the future, and it shows, once again, that false models should be regarded as means to truer theories (Wimsatt, 2007).
Conflict of Interest Statement
The author declares that the research was conducted in the absence of any commercial or financial relationships that could be construed as a potential conflict of interest.
References
Evans, M. R., Grimm, V., Johst, K., Knuuttila, T., de Langhe, R., Lessells, C. M., et al. (2013). Do simple models lead to generality in ecology? Trend. Ecol. Evol. 28, 578–583. doi: 10.1016/j.tree.2013.05.022
Harrison, P. J., Hanski, I., and Ovaskainen, O. (2011). Bayesian state-space modeling of metapopulation dynamics in the Glanville fritillary butterfly. Ecol. Monog. 81, 581–598. doi: 10.1890/11-0192.1
Lavine, M. (Ed.). (2010). Living dangerously with big fancy models. Ecology 91, 3487–3514. doi: 10.1890/10-1124.1
Levins, R. (2006). Strategies of abstraction. Biol. Philos. 21, 741–755. doi: 10.1007/s10539-006-9052-8
Mayo, D. (1996). Error and the Growth of Experimental Knowledge. Chicago, IL: The University of Chicago Press.
Oro, D. (2013). Grand challenges in population dynamics. Front. Ecol. Evol. 1:2. doi: 10.3389/fevo.2013.00002
Keywords: modeling and simulation, Bayesian inference, metapopulation dynamics, evolutionary ecology, philosophy of science
Citation: Almaraz P (2014) Simple models, complex models, useful models: can we tell them from the flap of a butterfly's wings? Front. Ecol. Evol. 2:54. doi: 10.3389/fevo.2014.00054
Received: 29 June 2014; Paper pending published: 18 July 2014;
Accepted: 13 August 2014; Published online: 29 August 2014.
Edited by:
Mauricio Lima, Pontificia Universidad Catolica de Chile, ChileReviewed by:
Sergio Andrés Estay, Universidad Austral de Chile, ChileDaniel Ernesto Naya, Universidad de la Republica, Uruguay
Rodrigo Ramos-Jiliberto, Universidad de Chile, Chile
Copyright © 2014 Almaraz. This is an open-access article distributed under the terms of the Creative Commons Attribution License (CC BY). The use, distribution or reproduction in other forums is permitted, provided the original author(s) or licensor are credited and that the original publication in this journal is cited, in accordance with accepted academic practice. No use, distribution or reproduction is permitted which does not comply with these terms.
*Correspondence:cGFsbWFyYXpAZ21haWwuY29t