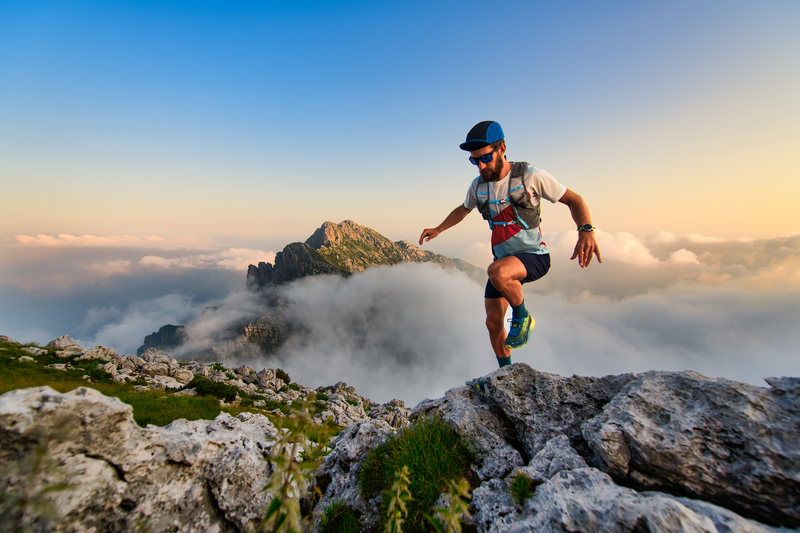
95% of researchers rate our articles as excellent or good
Learn more about the work of our research integrity team to safeguard the quality of each article we publish.
Find out more
EDITORIAL article
Front. Earth Sci.
Sec. Volcanology
Volume 13 - 2025 | doi: 10.3389/feart.2025.1604299
This article is part of the Research Topic Applications of Machine Learning in Volcanology View all 10 articles
The final, formatted version of the article will be published soon.
You have multiple emails registered with Frontiers:
Please enter your email address:
If you already have an account, please login
You don't have a Frontiers account ? You can register here
Machine learning (ML) techniques, capable of learning and adapting through automated or semi-automated algorithms and statistical models, allow us to classify signals and infer insights from patterns “hidden” in data, hence offering deeper understanding when analyzing and correlating large datasets. In recent decades, geosciences have seen a growth of the data available to the scientific community, as denser monitoring networks surround fault structures, volcanoes, geothermal fields and other regions of geological interest.ML techniques are applied to volcanology to improve the understanding of volcano dynamics and forecasting volcanic activity (Carniel & Guzmán, 2021). Recent reviews (Lütjens et al., 2022) highlight how ML’s adaptability addresses key challenges in geosciences, from noisy data to real-time decision-making under uncertainty. These techniques can be applied to early warning systems, significantly enhancing risk assessment and public safety.This research topic aims to be a compendium of the latest advancements in the use of ML techniques within geosciences. It has attracted 8 contributions, whose introductions follow.Titos et al. explored advanced techniques for monitoring volcano-tectonic (VT) earthquakes, by leveraging transfer learning, a procedure capable of adapting ML models to specific regions despite limited data available. As traditional methods often struggle with accurately classifying seismic signals in these settings, by using pre-trained models that have already learned to detect earthquake patterns better identifications and categorizations of VT-events have been found by the authors. The proposed approach represents a significant advance for volcano monitoring systems, allowing researchers to identify early warning signs of eruptions more effectively.Convolutional neural network (CNN) models have been designed to classify first-motion polarities of seismic records in volcanic and tectonic regions, as described in the manuscript by Messuti et al., where the development of the CFM algorithm is reported; this uses CNNs for automated, real-time classification, processing local and regional seismic waveforms and distinguishing between compressional and dilatational first motions. The network was trained on labeled datasets of seismic events: testing results demonstrate how CFM achieves an accuracy >90%, and adaptability across regions with different noise levels and tectonic structures, highlighting its potential to standardize and streamline polarity classification in seismic studies.Noguchi and Shoji explored the use of supervised ML to automate the extraction of stratigraphic features from visible images. Traditional methods for analyzing geological stratigraphy are often labor-intensive and subject to human error, making automated techniques highly valuable. Their approach involves training a CNN on a labeled dataset of stratigraphic images, allowing the model to learn to identify various geological formations with high accuracy. Such a CNN model outperformed conventional methods, effectively classifying stratigraphic features across diverse geological environments.Volcanic eruption forecasting represents an effort valuable for scientists. Rey-Devesa et al. explored this topic by introducing a universal ML approach which uses seismic features. By analyzing seismic data from diverse volcanic settings, the study demonstrates how four different seismic features passed to algorithms like neural networks can effectively predict eruption patterns. Results show the approach's robustness across different volcanoes, highlighting its potential for real-time monitoring and early warning systems, providing a tool for probabilistic short-term volcanic eruption forecasting, easily usable on different volcanic systems.Mitchinson et al., by using two concurrent unsupervised ML clustering algorithms, found anomalous earthquake swarms at Mt. Ruapehu, New Zealand: the article highlights the challenges of distinguishing swarms from background seismicity, using the chosen algorithms to classify patterns in seismic data. By leveraging historical records and real-time monitoring, the study enhances understanding of swarm characteristics and their links to volcanic processes. The findings support more accurate swarm identification, contributing to improved volcanic hazard assessment and mitigation efforts, and correlating their characteristics to seismic swarms related to fluid migration, more likely to happen near fault systems.Volcanic thermal emissions could be indicators of impending or ongoing eruptions. Saunders-Shultz et al. describe a specific CNN, the HotLINK system, for automatic identification and quantification of volcanic hotspots in Alaska: the study automates the detection of thermal anomalies from satellite imagery, improving the monitoring of active volcanic regions. The methodology integrates thermal data and predictive modeling to achieve precise identification of hotspots, offering a scalable solution for volcanic hazard management. The results demonstrate its effectiveness in enhancing real-time surveillance and contribute to advancing geoscientific applications.Giannoulis et al. investigate the drivers of surface temperature changes above geothermal systems by using the deep learning engine DAITAN applied to data recorded at Vulcano island, Italy. By analyzing a combination of surface and subsurface factors, the study identifies patterns and relationships affecting temperature variations. The approach leverages thermal data and environmental parameters to enhance the understanding of geothermal activity and its external influences. The findings provide valuable insights for monitoring and improving geothermal systems, and predicting potential hazards.Di Benedetto et al. introduced a grid-search methodology for optimizing Short-Term Average over Long-Term Average (STA/LTA) parameters in seismological applications, focusing on Stromboli's explosion quakes. By testing different parameter combinations by using a quality parameter, the approach improves signal detection and characterization of volcanic seismicity. The study demonstrates how this method refines STA/LTA settings for greater sensitivity to eruption-related seismic signals. The results highlight the methodology's potential not only for enhancing seismic monitoring in active volcanic regions, contributing to more accurate early warning systems, but also for applying it to a wider typology of signals.Collectively, the contributions proposed in this research topic explore how ML techniques can enhance insights into volcanic activities, achieving results that overwhelm classical methods. ML will assume a transformative role in volcanology -from automating labor-intensive tasks to uncovering hidden patterns in complex datasets. As emphasized by Rouet-Leduc et al. (2023), as the field moves toward operational monitoring, future works must balance innovation with interpretability. With increasing availability of data and the growing of population living near active volcanoes, integrating ML techniques into volcanic studies holds the promise of transforming how researchers will forecast eruptions and respond to volcanic hazards, representing a significant step forward in the future of volcanic research, having the potential to redefine volcanic risk mitigation in the decade ahead.
Keywords: Machine learning in volcanology, Volcanic eruption forecasting, Unsupervised machine learning (UML), Supervised machine learning (SML), Time series automated analysis
Received: 01 Apr 2025; Accepted: 04 Apr 2025.
Copyright: © 2025 Romano, Di Lieto, Scarpetta, Benítez and Aoki. This is an open-access article distributed under the terms of the Creative Commons Attribution License (CC BY). The use, distribution or reproduction in other forums is permitted, provided the original author(s) or licensor are credited and that the original publication in this journal is cited, in accordance with accepted academic practice. No use, distribution or reproduction is permitted which does not comply with these terms.
* Correspondence:
Pierdomenico Romano, Vesuvius Observatory, National Institute of Geophysics and Volcanology (INGV), Napoli, Italy
Disclaimer: All claims expressed in this article are solely those of the authors and do not necessarily represent those of their affiliated organizations, or those of the publisher, the editors and the reviewers. Any product that may be evaluated in this article or claim that may be made by its manufacturer is not guaranteed or endorsed by the publisher.
Research integrity at Frontiers
Learn more about the work of our research integrity team to safeguard the quality of each article we publish.