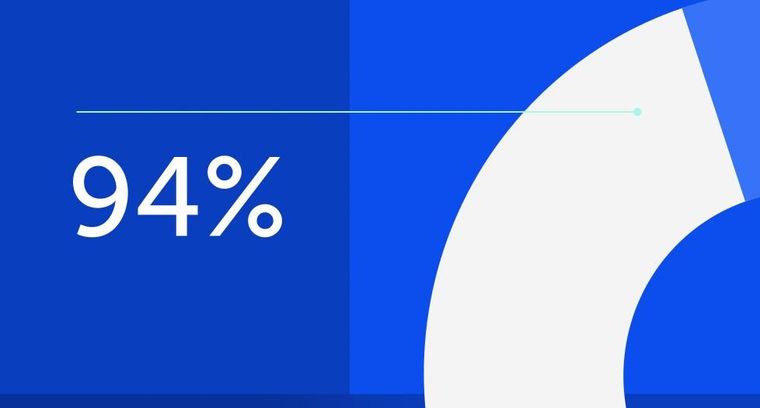
94% of researchers rate our articles as excellent or good
Learn more about the work of our research integrity team to safeguard the quality of each article we publish.
Find out more
ORIGINAL RESEARCH article
Front. Earth Sci.
Sec. Geohazards and Georisks
Volume 13 - 2025 | doi: 10.3389/feart.2025.1590203
The final, formatted version of the article will be published soon.
Select one of your emails
You have multiple emails registered with Frontiers:
Notify me on publication
Please enter your email address:
If you already have an account, please login
You don't have a Frontiers account ? You can register here
Accurate prediction of water inrush volumes is essential for safeguarding tunnel construction operations. This study proposes a method for predicting tunnel water inrush volumes, leveraging the eXtreme Gradient Boosting (XGBoost) model optimized with Bayesian techniques. To maximize the utility of available data, 654 datasets with missing values were imputed and augmented, forming a robust dataset for the training and validation of the Bayesian optimized XGBoost (BO-XGBoost) model. Furthermore, the SHapley Additive explanations (SHAP) method was employed to elucidate the contribution of each input feature to the predictive outcomes. The results indicate that:(1) The constructed BO-XGBoost model exhibited exceptionally high predictive accuracy on the test set, with a root mean square error (RMSE) of 7.5603, mean absolute error (MAE) of 3.2940, mean absolute percentage error (MAPE) of 4.51%, and coefficient of determination (R 2 ) of 0.9755; (2) Compared to the predictive performance of support vector mechine (SVR), decision tree (DT), and random forest (RF) models, the BO-XGBoost model demonstrates the highest R 2 values and the smallest prediction error; (3) The input feature importance yielded by SHAP is groundwater level (h) > water-producing characteristics (W) > tunnel burial depth (H) > rock mass quality index (RQD). The proposed BO-XGBoost model exhibited exceptionally high predictive accuracy on the tunnel water inrush volume prediction dataset, thereby aiding managers in making informed decisions to mitigate water inrush risks and ensuring the safe and efficient advancement of tunnel projects.
Keywords: Tunnel water inflow, XGBoost, Bayesian optimization, Data augmentation, Model interpretation
Received: 09 Mar 2025; Accepted: 08 Apr 2025.
Copyright: © 2025 Ju, Ou, Peng, Wang, Song and Guan. This is an open-access article distributed under the terms of the Creative Commons Attribution License (CC BY). The use, distribution or reproduction in other forums is permitted, provided the original author(s) or licensor are credited and that the original publication in this journal is cited, in accordance with accepted academic practice. No use, distribution or reproduction is permitted which does not comply with these terms.
* Correspondence: Guang-Zhao Ou, Hunan University of Finance and Economics, Changsha, China
Disclaimer: All claims expressed in this article are solely those of the authors and do not necessarily represent those of their affiliated organizations, or those of the publisher, the editors and the reviewers. Any product that may be evaluated in this article or claim that may be made by its manufacturer is not guaranteed or endorsed by the publisher.
Supplementary Material
Research integrity at Frontiers
Learn more about the work of our research integrity team to safeguard the quality of each article we publish.