- School of Mine Safety, North China Institute of Science and Technology, Langfang, Hebei, China
The analysis of historical coal mine safety events and the accurate identification of disaster factors are essential for effective mine safety management. Based on the KeyBERT network model, conducts a coupling analysis of six typical coal mine disaster cases in China between 2013 and 2023: gas explosions, water disasters, fires, roof collapses, coal dust incidents, and rockbursts. It utilizes the 24Model (a theoretical model of accident causation) to systematically analyze the mechanisms of each causative factor. The research reveals that causative factors of coal mine accidents can be classified into three categories: geological factors representing hazardous conditions of materials, serving as prerequisites for disaster occurrences; behavioral and managerial factors reflecting unsafe human behaviors, crucial as trigger conditions for disasters. Moreover, deeply explored the disaster-causing characteristics and action mechanisms of key geological factors such as faults, folds, goafs and overburden structures, and divided behavioral factors into two levels: psychological and executive. It was found that psychological factors play a leading role in accident induction. When psychological factors are superimposed on problems at the executive level, major safety hazards will be formed, seriously threatening coal mine safety production. Based on these findings, we have developed a dual-prevention mechanism integrating hidden danger investigation with safety risk classification control, and proposed an innovative “3LA” coal mine disaster management system, revealing that the inevitability of mine disasters stems from simultaneous failures at three management levels.
1 Introduction
Coal is projected to remain China’s primary energy source for the foreseeable future (Li et al., 2024). As the scale, speed, and depth of coal mining operations increase, the geological conditions and engineering challenges encountered during mining are becoming increasingly complex. According to recent data from the National Mine Safety Administration (2024), 1,128 coal mines in China are still facing geological disasters such as gas explosions, coal and gas outbursts, hydrogeological issues, and rockbursts. These problems pose significant safety risks in these mines.
Statistical analysis of coal mine accidents is essential for summarizing incident characteristics, elucidating underlying mechanisms, and promptly identifying factors that contribute to disasters, all crucial for safety management in mining operations. For example, data on coal mine safety accidents in China from 2010 to 2021 were compiled and analyzed by Zhao Yajun et al., who based their analysis on accident type, severity and location, recommending enhanced management of second and third-category hazard sources (Zhao et al., 2023). Zhao et al. conducted a statistical analysis of major coal accidents (involving three or more deaths) in mainland China from 2017 to 2022, comprehensively studying regional, temporal, and distribution characteristics of mining methods in the accident database (Zhao et al., 2024). Wang et al. utilized the Safety Entropy Model and Safety Degree Analysis to probe various aspects of coal mine accidents, such as accident types and regional distribution, offering recommended prevention and control measures (Wang et al., 2023). Liu et al. extracted key hazard terms from coal mine gas accident investigation reports and employed association rule analysis to identify root causes of these incidents, highlighting non-compliant operations by coal mining companies as a primary contributing factor (Liu et al., 2023). Tan et al. employed text analysis techniques to identify high-frequency terms related to coal mine accident hazards through preprocessing textual information (Tan et al., 2017). Zhao and Tian introduced a method for hazard identification in coal mines based on the naive Bayes algorithm, addressing limitations of traditional methods such as low recognition rates and extended recognition times (Zhao and Tian, 2021). Cheng Lei and colleagues applied mathematical statistics and systematic cluster tree analysis to focus on casualty numbers, accident types, and timing of coal mine accidents, categorizing accident risk levels (Cheng et al., 2023). Yuhong Qian utilized data mining techniques and the Apriori and FP-growth algorithms to analyze intrinsic relationships between methane, geological structures, and coal composition (Qian, 2018). Based on their analysis of coal mine accidents, Li Shuang et al. argued that inadequacies in management, education and supervision represent the primary causes of coal mine accidents compared to environmental and equipment risks (Li et al., 2022). Rulin Liu et al. gathered statistical data on 362 major coal mine accidents in China from 2000 to 2016, developing the Human Factors Analysis and Classification System for Chinese Mines (HFACS-CM) based on the results. Through the Analytic Hierarchy Process (AHP) method, and the HFACS-CM model, they conducted qualitative and quantitative evaluations of major coal mine accidents (Liu et al., 2018). Yuxin Wang et al. utilized a modeled accident prevention framework combined with coal mine gas explosion accident case data to construct a targeted data mining network for obtaining accident cause networks and enhancing control measures (Yuxin et al., 2024). Additionally, scholars like Li et al. (2023), Fa et al. (2021), and You et al. (2021) conducted text mining on accident cases to extract main risk factors and associated factors in coal mine safety accidents.
In conclusion, domestic and international scholars have systematically analyzed the types, spatiotemporal distribution characteristics, and disaster-causing mechanisms of coal mine accidents using historical data. Through the application of mathematical statistics, system safety evaluation, and data mining methods, they have investigated the complex relationships among various disaster-causing factors, thereby establishing a robust theoretical foundation for understanding coal mine disaster features. However, as coal mining operations intensify and extend to greater depths, the causes of disasters are becoming increasingly dynamic. Traditional analysis methods, which rely heavily on personal experience, are often subjective and insufficient, underscoring the need for more objective analytical approaches. Although computer technology has made significant progress in accident hazard text mining, it remains in its early stages, with considerable potential for enhancing the accuracy and depth of analysis. To address these challenges, this study employs computer technology and the Python programming language to develop a KeyBERT-based model. It conducts a coupling analysis of six typical coal mine disasters in China—gas explosions, water disasters, fires, roof collapses, coal dust incidents, and rockbursts—from 2013 to 2023. The study aims to objectively identify disaster-causing factors across different events, elucidate their geological foundations, and analyze the interrelationships among these factors using the 24Model of accident causation. This research provides a theoretical framework and practical insights for preventing and controlling disaster sources and implementing classification management in coal mine safety.
2 Data and methods
2.1 Technical route
In the study, pre-processed data, statistical methodologies, accident causation analysis models, and advanced deep learning techniques are utilized to classify and elucidate the contributory factors of diverse coal mine accidents. The technical methodology is depicted in Figure 1.
2.2 Data set source and preprocessing
Accident investigation reports play a crucial role in hazard identification and accident prevention (Liu et al., 2018; Tian et al., 2024). These reports offer a more truthful, accurate, and comprehensive depiction of incidents compared to other document types. In this study, a total of 332 typical coal mine accident investigation reports from the period 2013 to 2023 have been collected from reputable sources such as the Emergency Management Department, the National Mine Safety Administration, and the Coal Safety Network (https://www.mkaq.org/), as outlined in Table 1.
To prepare the accident text corpus for model input, preprocessing of the accident text dataset is conducted:
(a) Remove invalid data. In the original data set, remove the data with unknown accident time, location and cause as invalid data.
(b) Accident document consolidation. Trigger accidents within compound disasters, such as floods caused by rockburst, are integrated into the rock burst accident dataset.
(c) Data format conversion. After removing invalid entries, the valid data is summarized and organized into TXT format files categorized by accident type to streamline computer processing.
(d) Dataset segmentation. Text within reports is divided into datasets of direct and indirect causes based on coal mine accident types and investigative report segments. Some reports may lack indirect cause data due to diverse data sources, as outlined in Table 1.
(e) Text tokenization. The “Jieba” tokenizer in exact mode is utilized to process the text within each dataset, ensuring data efficiency.
(f) Text de-noising and punctuation removal. A stopword list for coal mine accident analysis, comprising 2,011 stopwords/phrases from the Harbin Institute of Technology supplemented with specialized vocabulary from safety and mining engineering, is constructed. This list is employed to eliminate stopwords and special punctuation from the text datasets, thereby reducing vector dimensions and enhancing model performance.
(g) Report text consolidation. Coal mine accident reports often vary in their descriptions of accident causes, leading to different yet synonymous terms like “burial depth” and “depth”, or “safety supervision and management” and “safety regulation.” To address this, a synonym consolidation lexicon is developed to unify expressions of the same cause, preventing oversight of similar accident causes due to diverse terminology.
3 Results
3.1 Disaster-causing keyword extraction
The TF-IDF (Term Frequency-Inverse Document Frequency) algorithm (Zhang et al., 2011) is utilized as a statistical weighting measure in information retrieval and text mining fields. Its purpose is to determine the importance of words in documents, particularly effective in situations where text length is consistent, and term complexity is low. A higher TF-IDF score generally signifies increased textual significance. Equations 1–3 detail the computation of TF-IDF.
In the formula: TF represents the frequency of the keywords within the accident dataset texts; IDF represents the inverse document frequency; ni,j denotes how many times the keywords appear in the document; N corresponds to the overall document count in the corpus; dfi signifies the quantity of documents containing the keywords.
The TF-IDF algorithm was employed to analyze the importance of each word in the datasets for direct and indirect causes. The results were visualized using the WordCloud package in Python, as shown in Figure 2. In Figure 2, the size of the words is proportional to their frequency.
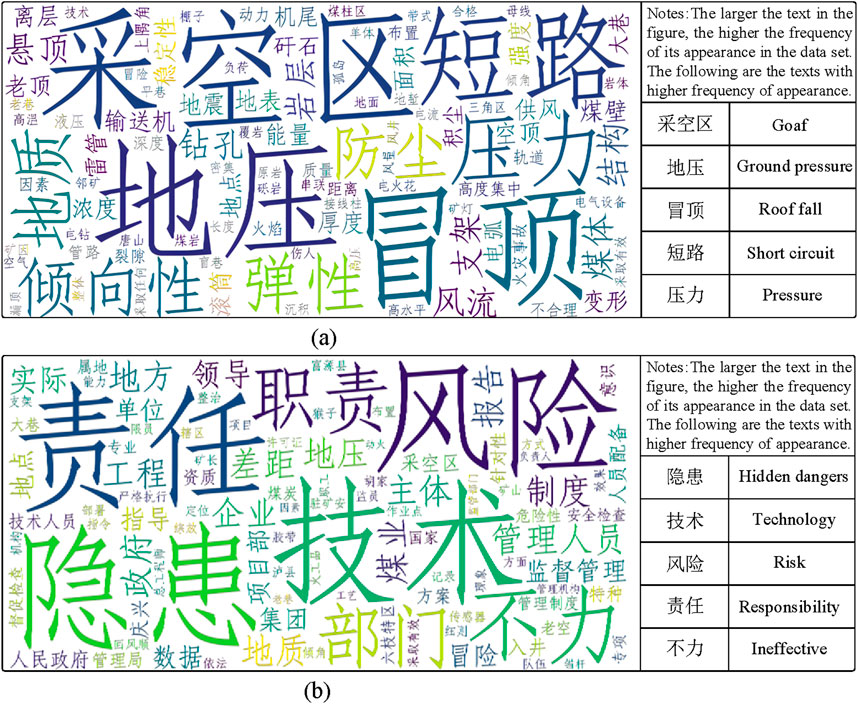
Figure 2. Visualization of disaster-causing keywords. (a) Direct cause dataset. (b) Indirect cause dataset.
As depicted in Figure 2, the disaster-causing factors of coal mine accidents are categorized as geological, behavioral, and managerial factors. The direct cause dataset predominantly extracts disaster-causing geological and behavioral factors, while the indirect cause dataset focuses on disaster-causing managerial factors. However, TF-IDF, a statistical weighting algorithm, measures the significance of words based solely on frequency, overlooking word semantics, and presents the following shortcomings:
(1) Due to variations in the number of documents across different coal mine accident reports and the differing lengths of each report, it is possible that some relatively important words that do not appear frequently may be overlooked.
(2) After text segmentation and the removal of stopwords, the documents extracted by the TF-IDF algorithm consist only of individual nouns or verbs, which may not fully reflect the causes of accidents, such as coal thickness, ventilation, accumulation, personnel, etc.
3.2 Disaster factor identification
BERT (Bidirectional Encoder Representations from Transformers) is a deep learning-based pre-trained language model that utilizes the Transformer Encoder architecture (Devlin et al., 2019). During its pre-training phase, multi-layer bidirectional Transformer encoders are employed to capture bidirectional relationships within a large-scale corpus, significantly enhancing the model’s ability to recognize semantic similarities. The application of BERT in text extraction is primarily dependent on fine-tuning, which involves making subtle adjustments to the pre-trained model for specific tasks. The significance of BERT in short text classification is underscored by its bidirectional encoding capabilities and contextual understanding (Qin and Zhang, 2024), addressing the limitations of the TF-IDF algorithm, such as insufficient accuracy, incapacity to reflect word position, and incapacity to represent sequence information.
KeyBERT is a keyword/phrase extraction model based on the BERT architecture, which utilizes text embeddings and word-document matrices to identify document-relevant keywords while minimizing semantic similarity between them. In this section, disaster-related keywords extracted using TF-IDF are further refined using the KeyBERT model by adjusting parameters such as “keyphrase_length” and “n_gram_range.” Cosine similarity is used to identify the most relevant keywords/phrases, and the Maximal Marginal Relevance (MMR) algorithm is employed to address issues arising from similar word groups, extracting key geological, behavioral, and managerial factors contributing to various types of coal mine disasters as seen in Tables 2, 3. KeyBERT’s architecture is illustrated in Figure 3. Note: Since the dataset originates from a Chinese website, except for some specific terminology in English, all others are in Chinese. Thus, the BERT module used in this study refers to the Bert-base-chinese version.
From Table 4, it is evident that the factors that lead to coal mine disasters are complex and multifaceted. According to accident investigations and statistical analyses (Fu et al., 2020), it is understood that coal mine disasters are the result of interactions between hazardous physical conditions and unsafe human behaviors. Geological factors represent these hazardous conditions and are prerequisites for the occurrence of disasters, while behavioral and managerial factors are triggers due to unsafe human actions.
From the perspective of hazardous physical conditions, geological factors such as deep coal seams, susceptibility to impacts, complex geological structures, unclear mined-out areas, and poor stability of coal layers are considered the prerequisites for coal mine disasters. These geological factors that cause disasters are primarily characterized by their geogenic nature and concealment. In terms of disaster factor analysis, mined-out areas and coal layer stability emerge as common factors contributing to all types of coal mine accidents. Effective detection technologies should be utilized in practical mining engineering to accurately pinpoint the location, size and water content of mined-out areas. Efforts should also be directed towards assessing and monitoring the thickness, occurrence and structure of coal layers to ensure mining safety. Analysis of disaster types reveals that water disasters, roof collapses, and rockbursts are associated with a higher number of contributing factors, whereas fires, dust explosions, and gas disasters involve fewer. Empirical evidence suggests that water disasters, roof collapses, and rockbursts pose significantly greater challenges in terms of control during mining operations, thereby indirectly corroborating the accuracy of these research findings (Cao et al., 2022; He et al., 2018; Miao et al., 2024).
Compared to hazardous physical conditions, human unsafe behaviors are more complex, and effectively controlling these behaviors is crucial for improving the severe situation of coal mine safety production (An et al., 2020). Inadequate dust management, incomplete “ventilation and three prevention” systems, unauthorized use of open flames, violation in using welding machines, and “naked-eye” blasting are unsafe behaviors that trigger coal mine disasters. In individual behavior analysis, workers” unfamiliarity with safety regulations, lack of safety knowledge, low safety awareness, and presence of wishful thinking are factors that trigger disasters. In management system analysis, flaws in the safety management organizations, loopholes in safety regulations, lack of safety training and routine emergency drills, failure in administrative approvals, lax inspection at resumption of work, ineffective supervision, and oversight of significant hazards trigger disasters.
3.3 Analysis of causes of coal mine disasters
The “2–4” Accident Causation Model (24Model) has been proposed by Fu Gui and others, drawing on foundations such as the Domino and Swiss Cheese models (Fu et al., 2022). This model classifies the causes of accidents into two primary factors: organizational and individual. Organizational factors are further subdivided into safety culture (root causes) and safety management systems (fundamental causes), whereas individual factors are segmented into human safety capabilities (indirect causes) and the safety actions of individuals and objects (direct causes). The sixth edition of the 24Model is depicted in Figure 4. The building-like structure of the “2–4” model is widely utilized across diverse industries to assist enterprises in identifying risks and preventing accidents (Fu et al., 2020; Fu et al., 2013; Sun et al., 2023).
Deriving from the identified disaster causation factors, a 24Model is utilized in this section for the coupling analysis of coal mine accident causation factors. The detailed results of this analysis are depicted in Figure 5. As demonstrated in Figure 5, the causation pathways of coal mine disasters encompass organizational and individual levels. Organizational pathways consist of root causes and fundamental causes, while individual pathways entail indirect and direct causes. Often, the occurrence of coal mine disasters is rooted in simultaneous deficiencies at both organizational and individual levels.
Coal mining enterprises should establish a dual prevention mechanism involving safety risk classification control and hidden danger investigation and management. The risk situation of various hidden danger factors should be analyzed, evaluated, and tracked to ensure the effective implementation of various control measures and proper risk management. Hidden dangers resulting from inadequate control measures should be addressed through hidden danger investigation and management to eradicate potential accident hazards and ensure safety at the root. This two-tier control approach not only offers a structured method for risk mitigation but also integrates the organization’s safety procedures with individual responsibilities, creating a comprehensive safety network that spans all levels of the mine.
3.4 Connotation of coal mine disaster-causing factors
Coal mine disasters exhibit a predisposition to calamity, sudden onset, and manageability. A comprehensive grasp of the implications of factors that lead to disasters in coal mines is pivotal in effectively mitigating such occurrences. Building upon the identification outcomes of disaster-inducing factors, this section delves into the implications of each catalyst of disaster. Delving into the core of each factor facilitates a profound understanding of their respective contributions to the overall risk and the efficacy of specific preventive measures. This process aids not only in pinpointing critical areas that demand safety interventions but also in tailoring responses to align with the intricacy and uniqueness of each factor. The ultimate objective is to establish a resilient framework that reduces disaster risk by addressing systemic vulnerabilities and specific triggers within the mining environment.
3.4.1 Geological factors
3.4.1.1 Coal seam depth
The burial depth of a coal seam denotes its vertical distance from the surface and is categorized as either shallow or deep. Shallowly buried coal mines are situated at depths of less than 300 m, while deeply buried coal mines extend beyond 300 m. The depth of burial influences the geostress conditions of the coal seam and serves as a concealed factor that triggers disasters, representing a critical hidden geological element in the occurrence of rockbursts and roof falls in coal mines.
Research indicates a positive correlation between the level of geostress and the depth of coal burial. With increasing depth, the vertical stress on deep coal seams escalates significantly, heightening the likelihood of rockbursts and roof falls. According to the theory of elasticity, in the triaxial stress state unaffected by mining activities, the calculation for the vertical stress on the coal body is defined by Equation 4:
In the formula:
3.4.1.2 Coal seam stability
Coal seam stability is defined by the variations in thickness, occurrence, structure and coal quality within a working area. The key factors determining coal seam stability are the fluctuations in thickness and occurrence. By considering the characteristics of occurrence, thickness, structure and variation coefficients, coal seam stability can be classified into four types: stable, relatively stable, unstable, and unstable. The stability of coal seams impacts not just the arrangement of mining tunnels and workface recovery but also has the potential to trigger coal and gas outbursts, water disasters, roof falls, and rockbursts.
(a) Coal seam stability is considered a crucial factor in gas accumulation. Compared to surrounding rock, coal seams demonstrate lower permeability and enhanced gas retention capacity, increasing susceptibility to coal and gas outburst accidents, particularly in regions with stress concentration. Research by Yang et al. indicates that both the frequency and severity of outbursts notably rise with coal seam thickness (Yang et al., 2024). For instance, a coal and gas outburst incident transpired in 2020 at a mine operated by Ziliang Qiao Coal Industry Co., Ltd. in Tongchuan, Shaanxi Province, China, coinciding with a sudden thickening of the coal seam. Failure to implement timely drainage measures resulted in eight fatalities and 13 injuries.
(b) Fluctuations in coal seam thickness can establish connections with water-bearing pathways. The existence of coal washout zones and burned coal regions may foster links between the coal seam and the underlying aquifers, triggering roof water inrush incidents and posing potential hazards to workface recovery. Thorough geological and hydrological analyses are imperative to mitigate risks during mining operations.
(c) Anomalous geostress is observed in areas where coal thickness varies. Regions experiencing fluctuations in coal seam thickness exhibit heightened gradients in elastic energy alterations. The more pronounced the variations in coal seam thickness, the greater the mining-induced pressure, escalating the likelihood of dynamic disasters like roof collapses and rockbursts (Zhu et al., 2016), as depicted in Figure 6.
3.4.1.3 Overburden structure
The rock layers above and below coal seams are known as the overlying and underlying strata, respectively. The overlying rock, or “roof,” and the underlying rock, or “floor,” are crucial for structural integrity in mining operations (Zhu et al., 2024). The overlying strata typically consist of bedrock, which can be divided into hard and soft rock layers. The hard roof above coal seams plays a crucial role in influencing dynamic pressure impacts, roof stability, and water disaster accidents.
(a) Roof accidents occur when the immediate roof fails to collapse adequately to fill the mined-out void, leading the remaining hanging roof to shift its weight and that of the overlying rock layers onto the coal surrounding the mining face, potentially causing roof accidents.
(b) In high static load environments, intense mining operations can induce differential settlement between layers within the overlying strata, resulting in the creation of separation spaces (Chen et al., 2023). If these voids become filled with water from an aquifer, they can transform into water bodies. Should fracture conducive to water flow intersect with these water bodies, a significant inrush incident may transpire (Qiao et al., 2021), as demonstrated in Figure 7.
(c) As the mining face progresses backward, the fracturing and initial collapse of the thick old roof, along with cyclic rotational instabilities, exert a significant influence on the mining pressure encountered at the work front. This influence serves as a catalyst for the emergence of impact ground pressures, which present a substantial hazard. If a full-layer fracture occurs, it could pose a considerable shock hazard to the mining face, escalating the complexities and risks involved in overseeing mine safety under these dynamic circumstances.
3.4.1.4 Goaf
Mining voids, or goafs, are empty spaces left underground after the extraction of coal or gangue, including abandoned tunnels and old workings. These voids can be categorized into three types based on the continuity of the physical parameters of the surrounding rock post-mining: intact, damaged, and small coal pit voids. Due to the vast number and widespread distribution of these voids, along with severely inadequate or missing historical mining records, their exact locations and internal conditions are often unclear, posing significant threats to nearby coal seam mining (Xu et al., 2025). Mining voids are critical factors in causing water hazards, fires, gas outbursts, and rock burst accidents.
(a) Coal seam extraction results in the destruction, collapse, and surface fissures of overlying rock layers, potentially establishing connections with overlying aquifers or surface water bodies, leading to water pooling within the goaf. As underground mining activities approach the water-filled goaf, influenced by hydrostatic pressure, water can suddenly flood into the underground mine workings, posing risks of inundation or flooding accidents.
(b) Goafs that remain uncollapsed or incompletely collapsed may suddenly collapse over time due to mining disturbances and other influencing factors. Such collapses can cause damage to surface personnel and critical structures. Furthermore, the ensuing high-speed air blasts and shockwaves can result in injuries and equipment damage for personnel working in neighboring underground areas near the aged goafs.
(c) In coal seams with short natural ignition periods, spontaneous combustion of residual coal within goafs can occur easily (Qin and Ma, 2024), especially under conditions of ample oxygen supply. If left unchecked, fires may spread to lower coal seams, necessitating production halts in adjacent mine workfaces and endangering the safety of mining personnel.
(d) Old goafs tend to accumulate methane gas, posing threats to safe mining operations as gas may infiltrate lower mining areas through mining-induced fissures during the extraction process, jeopardizing the safety of mine workfaces.
(e) After coal extraction, the fracturing and displacement of overlying rock layers lead to the creation of complex three-dimensional rock structures above the mine workings. The evolution of these structures, driven by roof fractures, subsidence, and rotational movements, triggers the migration of high-stress fields within the mining area, consequently elevating the risk of rockbursts at the workface (Liu et al., 2024).
3.4.1.5 Fault
Faults refer to brittle deformations of rocks that occur when the forces applied surpass the rocks' strength limits, resulting in distinct displacement and misalignment that compromise the continuity and integrity of the rocks (Ma et al., 2025). Faults are classified by the relative direction of movement between their walls into normal faults, reverse faults, and strike-slip faults (Due to the predominantly horizontal sliding nature of strike-slip faults, their vertical displacement is minimal, resulting in a lesser direct impact on the stability of coal seams. Moreover, strike-slip faults typically belong to major fault systems associated with seismic activity, whereas coal mining disasters are more closely linked to variations in local geological conditions during the extraction process. Consequently, this section primarily focuses on the causative implications of normal and reverse faults.). These geological features alter the depositional conditions of coal-bearing rock layers and are crucial, concealed geological factors triggering coal mine water inrushes, coal and gas outbursts, impact ground pressures, and roof accidents.
(a) Faults directly connect coal rock layers with strong aquifers or establish a conductive connection between fault zones and strong aquifers, forming the most prevalent type of water inrush channel in mines, as illustrated in Figure 8. Additionally, faults also act as aquifer sources due to the disruption of strata continuity and stability, with fractures commonly developing, making it easy to accumulate and store water. Under the influence of mining activities, this can lead to sudden water inrush accidents (Qiao et al., 2024).
(b) Faults cause fragmentation of the coal seam roof and its adjacent rock layers. As the mining face advances near the fault due to mining dynamics and mining pressure, the coal seam roof slides along the sides of the fault plane, enhancing rock fragmentation near the fault and increasing the risk of roof falls (Chen et al., 2018).
(c) Elevated stresses near faults induce the compression and fracturing of coal layers, creating numerous pores and free spaces that enhance the coal’s adsorption surface area and expand its storage capacity for free gas (Shen et al., 2008). As the mining face crosses a fault, the permeability of the coal rock mass on either side or in the vicinity changes instantaneously, leading to substantial variations in gas pressure. Additionally, disturbances in the mining stress field result in stress concentration and the release of structural stress, thereby triggering rockbursts.
(d) Faults compromise the continuity and integrity of coal rock layers, transforming the uniformly distributed stress environment. Furthermore, stress accumulation near faults, in conjunction with pre-existing residual structural stresses, generates highly concentrated local stresses in the coal rock mass surrounding the faults, as depicted in Figure 9. Mining activities exacerbate the disturbance of this non-uniform stress field near the fault, inducing destabilization. This destabilization may result in fault slippage, activation, or instability (including sudden misalignment), potentially initiating fault-induced rockbursts. For example, during mining operations at the Lucky Friday mine in the United States of America, Williams and Whyatt, utilizing seismic analysis and direct observations, recorded significant fault misalignments that caused tunnel damage (Whyatt et al., 2002).
3.4.1.6 Folds
Folds in rock layers, or their combinations, develop through slow deformation induced by horizontal compressive forces acting along bedding planes. Empirical studies have shown that rockburst-related hazards predominantly occur on the anti-dip side of synclinal axes, especially in regions of structural change, near faults, within zones of coal seam inclination variation, at coal seam folds, and within structural stress zones (Pan et al., 2024). As tunnels approach the vicinity of a fold axis, local stresses escalate significantly, markedly increasing both the likelihood and intensity of rockbursts and roof falls. The spatial distribution of hazards in fold areas is depicted in Figure 10, emphasizing high-risk zones and delineating targeted areas for monitoring and intervention to effectively mitigate these risks.
3.4.1.7 Collapse column
A collapse column is a geological feature formed when voids resulting from the dissolution of soluble rock layers beneath coal seams are filled and consolidated by overlying coal-bearing strata. Collapse columns are classified into non-conductive, weakly conductive, conductive, and strongly conductive categories based on their hydraulic conductivity.
The presence of collapse columns disrupts the continuity of coal seams and surrounding rock, rendering specific coal seam sections unmineable. This disruption often necessitates redesigning mine shafts and tunnels or may lead to their complete abandonment. The functionality and efficiency of mechanized coal mining equipment are significantly compromised, severely disrupting standard mining operations. Collapse columns frequently create direct hydraulic connections between multiple water-bearing strata, serving as primary conduits for karst groundwater flow and covert pathways for catastrophic water inrush events. These phenomena are marked by sudden, high-volume water inflows, unpredictability, substantial economic losses, and elevated management costs, representing significant hazards to mine safety.
3.4.2 Management factors
3.4.2.1 Coal mine “3LA” management system
The management system of coal mines is characterized by its high systematic nature and extensive content (Yang and He, 2022). Analysis of accident investigation reports allows for the classification of coal mine management into a “3LA” framework, as depicted in Table 3. “3LA” encompasses three levels—government, enterprises, and mines—as well as three aspects: people, materials, and the environment.
Distinct roles and focuses are characterized by each management tier within the coal mining sector. The government, situated at the first tier, assumes responsibilities for top-level design and macroeconomic regulation. Enterprises constitute the second tier, serving as intermediaries between the government and the mines, applying governmental designs by integrating corporate interests and operational data to guide normative mine management. The mines, representing the third tier, directly implement the strategic designs and balance corporate and mining interests.
3.4.2.2 Connotation analysis of management factors
Based on the “3LA” management system of coal mines and combined with the results of coal mine disaster-causing factors identification, it can be seen that the occurrence of a certain coal mine disaster is inevitable and is the result of simultaneous mistakes at three management levels. From the environmental management standpoint, detrimental influences on disaster occurrence include a deficient societal safety culture, fragile safety development ideologies, gaps in safety production regulations, and an adverse safety culture within enterprises and mines. In terms of material management, secondary factors leading to disasters include inadequate material provisions, irrational material logistics, obsolete production equipment, non-compliant flammability specifications, insufficient structural support, and subpar performance of personal safety devices. Regarding personnel management, primary factors entail multiple failures in administrative oversight, inadequate scrutiny by inspectors, fraudulent activities by institutional appraisers, and negligence towards significant risks by workers.
3.4.3 Behavioral factors
The analysis in Section 3 concludes that behavioral factors encompass both psychological and operational dimensions. In coal mining disasters, predominant psychological elements—such as low safety awareness, poor safety attitudes, wishful thinking, complacency, and risk-taking tendencies—often lead workers to engage in unsafe actions, such as unauthorized extensions of mine shafts or the improper use of oxygen or liquefied petroleum gas for cutting. If corrective measures are not promptly implemented at both psychological and operational levels, significant hazards can arise, posing severe threats to the safety of coal mining operations. The disaster-inducing nature of behavioral factors is illustrated in Figure 11.
4 Conclusion and limitations
1) Drawing on 332 typical coal mine accident investigation reports occurring between 2013 and 2023 as the data foundation, the causative factors in coal mine accidents are accurately identified utilizing the TF-IDF algorithm and the KeyBERT model. The results indicate that the factors leading to coal mine accidents can be broadly categorized as geological, behavioral, and managerial factors.
2) Based on the statistical analysis of accident investigations, coal mine disasters result from a combination of hazardous conditions and unsafe behaviors of individuals. In this regard, geological factors, serving as hazardous conditions, constitute the foundational prerequisites for disaster occurrences, while behavioral and managerial factors, representing unsafe behaviors, act as triggering conditions for disasters. Leveraging the insights from the “2–4” accident causation model, a dual preventive mechanism is proposed.
3) An analysis is conducted on the pathways through which common geological factors in coal mines, such as geological structures, goafs, coal seam depth, stability, and overlying strata, lead to disasters. The disaster-causing implications of behavioral factors in coal mine accidents are examined from the perspectives of psychology and implementation. Furthermore, a coal mine “three-vertical and three-horizontal” management system is proposed, encompassing analyses of the connotations of disaster management factors in coal mines from the aspects of environmental management, material management, and personnel management.
This paper uses computer technology to identify the causative factors of the six major disasters in coal mines, analyzes the meaning and characteristics of each causative factor, provides a reference for the source prevention and classification management of coal mine disasters, and can guide the development of coal mine disaster factor detection technology, and provide valuable reference for solving on-site engineering problems. However, this paper only discusses the causative factors of a single disaster, and does not conduct an in-depth discussion on the identification or analysis of compound causative factors. In addition, since this paper was written in 2024, to ensure the timeliness of the data, this study only selected data from 2013 to 2023 as the analysis object. In fact, with the increase in mining depth and the complexity of coal seam conditions, coal mine disasters are changing from single disasters to compound disasters, such as gas-dust compound explosion, rock burst-gas outburst compound disaster, coal spontaneous combustion-gas explosion compound disaster, etc. According to the list of national disaster-prone coal mines released by the China National Mine Safety Supervision Bureau in April 2024, 145 mines were affected by two or more types of coal mine disasters. In the past, most coal mines have established corresponding prevention and control systems for various single disasters. However, due to differences in construction time, construction units and data standards, there are data islands, inconsistent standards, and difficulty in integration, which leads to the independent operation of various disaster prevention and control systems. The comprehensive prevention and control capabilities for multiple disasters are weak, and there is no linkage with emergency broadcasting and disaster prevention facilities and equipment. Therefore, in view of the above-mentioned shortcomings, we will further explore the compound disasters that have occurred in recent years in subsequent work.
Data availability statement
The original contributions presented in the study are included in the article/supplementary material, further inquiries can be directed to the corresponding author.
Author contributions
ZO: Validation, Methodology, Investigation, Writing – review and editing. QX: Writing – original draft, Validation, Methodology, Investigation, Formal analysis. TZ: Writing – review and editing, Investigation. HY: Writing – review and editing, Conceptualization. NZ: Writing – review and editing, Investigation, Conceptualization. MX: Writing – review and editing, Visualization. CJ: Writing – review and editing, Methodology.
Funding
The author(s) declare that financial support was received for the research and/or publication of this article. This work was supported by the National Natural Science Foundation of China (52274120) and supported by Key Program of Hebei Natural Science Foundation (E2024508004).
Conflict of interest
The authors declare that the research was conducted in the absence of any commercial or financial relationships that could be construed as a potential conflict of interest.
Generative AI statement
The author(s) declare that no Generative AI was used in the creation of this manuscript.
Publisher’s note
All claims expressed in this article are solely those of the authors and do not necessarily represent those of their affiliated organizations, or those of the publisher, the editors and the reviewers. Any product that may be evaluated in this article, or claim that may be made by its manufacturer, is not guaranteed or endorsed by the publisher.
References
An, Y., Wang, H., Li, Z., Zhang, J., and Tong, R. (2020). Research on formation mechanism of miners' unsafe behaviors based on TPB. China Saf. Sci. J. 30 (10), 20–26. doi:10.16265/j.cnki.issn1003-3033.2020.10.003
Cao, Z., Gu, Q., Huang, Z., and Fu, J. (2022). Risk assessment of fault water inrush during deep mining. Int. J. Min. Sci. Technol. 32 (2), 423–434. doi:10.1016/j.ijmst.2022.01.005
Chen, S., Liu, R., Xu, Z., A, K. A., Shao, H., and Feng, F. (2023). Surface subsidence laws of footwall coal seam mining of normal fault under different overburden strata. J. Shandong Univ. Sci. Technol. Sci. 42 (01), 38–48. doi:10.16452/j.cnki.sdkjzk.2023.01.005
Chen, S., Xia, Z., Guo, W., and Shen, B. (2018). Research status and prospect of mining catastrophic response of rock mass under the influence of fault. Coal Sci. Technol. 46 (01), 20–27. doi:10.13199/j.cnki.cst.2018.01.003
Cheng, L., Xu, Y., Jing, G., Cheng, Z., and Liu, Y. (2023). Statistical analysis of national coal mine accidents and classification of accident type risk. Coal Technol. 42 (06), 128–132. doi:10.13301/j.cnki.ct.2023.06.030
Devlin, J., Chang, M.-W., Lee, K., and Toutanova, K. (2019). BERT: pre-training of deep bidirectional Transformers for language understanding, in Proceedings of the 2019 conference of the North American chapter of the association for computational linguistics: human language technologies, volume 1 (long and short papers) Minneapolis, Minnesota. Editors J. Burstein, C. Doran, and T. Solorio doi:10.48550/arXiv.1810.04805
Fa, Z., Li, X., Qiu, Z., Liu, Q., and Zhai, Z. (2021). From correlation to causality: path analysis of accident-causing factors in coal mines from the perspective of human, machinery, environment and management. Resour. Policy 73, 102157. doi:10.1016/j.resourpol.2021.102157
Fu, G., Chen, Y., Xu, S., Chen, P., Yuan, C., and Wu, Y. (2022). Detailed explanations of 24Model and development of its 6th version. China Saf. Sci. J. 32 (01), 12–19. doi:10.16265/j.cnki.issn1003-3033.2022.01.002
Fu, G., Xie, X., Jia, Q., Li, Z., Chen, P., and Ge, Y. (2020). The development history of accident causation models in the past 100 years: 24Model, a more modern accident causation model. Process Saf. Environ. Prot. 134, 47–82. doi:10.1016/j.psep.2019.11.027
Fu, G., Yin, W., Dong, J., Di, F., and Cherrie, J. Z. (2013). Behavior-based accident causation:the “2-4”model and its safety implications in coal mines. J. China Coal Soc. 38 (07), 1123–1129. doi:10.13225/j.cnki.jccs.2013.07.032
He, M., Ren, F., and Liu, D. (2018). Rockburst mechanism research and its control. Int. J. Min. Sci. Technol. 28 (5), 829–837. doi:10.1016/j.ijmst.2018.09.002
Li, F., Duan, B., Sun, Y., He, X., Li, Z., and Wang, B. (2024). Quantitative risk assessment model of working positions for roof accidents in coal mine. Saf. Sci. 178, 106628. doi:10.1016/j.ssci.2024.106628
Li, S., You, M., Li, D., and Liu, J. (2022). Identifying coal mine safety production risk factors by employing text mining and Bayesian network techniques. Process Saf. Environ. Prot. 162, 1067–1081. doi:10.1016/j.psep.2022.04.054
Li, Z., Yao, M., Luo, Z., Wang, X., Huang, Q., and Su, C. (2023). Analysis of risk factors of coal chemical enterprises based on text mining. J. Environ. Public Health 2023 (1), 1–12. doi:10.1155/2023/4181159
Liu, R., Cheng, W., Yu, Y., and Xu, Q. (2018). Human factors analysis of major coal mine accidents in China based on the HFACS-CM model and AHP method. Int. J. Industrial Ergonomics 68, 270–279. doi:10.1016/j.ergon.2018.08.009
Liu, W.-j., Hou, M.-j., Dong, S.-s., Xiao, Y., Wang, H.-n., Deng, Z.-g., et al. (2024). Rock burst prevention and control of multifield coupling in longwall working face. Appl. Geophys. 21 (1), 119–132. doi:10.1007/s11770-023-1013-3
Liu, Y., Liang, Y., and Li, Q. (2023). Cause analysis of coal mine gas accidents in China based on association rules. Appl. Sci. 13 (16), 9266. doi:10.3390/app13169266
Ma, Q., Bai, S., Zhang, R., Li, X., Xie, C., and Liu, X. (2025). Research on the deformation and failure law and control technology of surrounding rock in the dense roadway area in footwall of normal fault. Front. Earth Sci. 13. doi:10.3389/feart.2025.1517598
Miao, D., Wang, W., Liu, L., Yao, K., and Sui, X. (2024). Coal mine roof accident causation modeling and system reliability research based on directed weighted network. Process Saf. Environ. Prot. 183, 653–664. doi:10.1016/j.psep.2024.01.053
Pan, Y., Song, Y., Luo, H., and Xiao, Y. (2024). Coalbursts in China: theory, practice and management. J. Rock Mech. Geotechnical Eng. 16 (1), 1–25. doi:10.1016/j.jrmge.2023.11.003
Qian, Y. (2018). Application of data mining algorithm in gas safety prediction. Coal Technol. 37 (05), 207–209. doi:10.13301/j.cnki.ct.2018.05.079
Qiao, W., Liu, M., Meng, X., Cheng, X., Feng, P., Li, X., et al. (2024). Exploration and prediction evaluation on causative factors of water inrush from separation layers of mining overburden in coal mines. J. China Coal Soc. 49 (04), 2031–2044. doi:10.13225/j.cnki.jccs.XH23.1691
Qiao, W., Wang, Z., Li, W., Lu, Y., Li, L., Huang, Y., et al. (2021). Formation mechanism, disaster-causing mechanism and prevention technology of roof bed separation water disaster in coal mines. J. China Coal Soc. 46 (02), 507–522. doi:10.13225/j.cnki.jccs.XR20.1972
Qin, B., and Ma, D. (2024). Research progress and challenges in prevention and control of combined disas-ters of coal spontaneous combustion and methane in coal mine goaf. J. China Coal Soc., 1–18. doi:10.13225/j.cnki.jccs.ST23.1624
Qin, S., and Zhang, M. (2024). Boosting generalization of fine-tuning BERT for fake news detection. Inf. Process. & Manag. 61 (4), 103745. doi:10.1016/j.ipm.2024.103745
Shen, B., King, A., and Guo, H. (2008). Displacement, stress and seismicity in roadway roofs during mining-induced failure. Int. J. Rock Mech. Min. Sci. 45 (5), 672–688. doi:10.1016/j.ijrmms.2007.08.011
Sun, S., Sun, Z., Feng, Z., and Fu, G. (2023). Behavioral causes analysis of construction accidents based on behavior-based accident causation 24Model theory. China Saf. Sci. J. 33 (11), 30–37. doi:10.16265/j.cnki.issn1003-3033.2023.11.2450
Tan, Z., Chen, X., Song, Q., and Chen, X. (2017). Analysis for the potential hazardous risks of the coal mines based on the socalled text mining. J. Saf. Environ. 17 (04), 1262–1266. doi:10.13637/j.issn.1009-6094.2017.04.009
Tian, S., Wang, Y., Li, H., Ma, T., Mao, J., and Ma, L. (2024). Analysis of the causes and safety countermeasures of coal mine accidents: a case study of coal mine accidents in China from 2018 to 2022. Process Saf. Environ. Prot. 187, 864–875. doi:10.1016/j.psep.2024.04.137
Wang, Y., Zhang, X., Huang, C., Qi, W., Wang, J., Yang, X., et al. (2023). Spatial-convolution spectral-transformer interactive network for large-scale fast refined land cover classification and mapping based on ZY1-02D satellite hyperspectral imagery. Remote Sens. 15 (13), 3269. doi:10.3390/rs15133269
Whyatt, J. K., Blake, W., Williams, T. R. N., and White, B. G. (2002). 60 Years of rockbursting in the coeur D’alene district of Northern Idaho, USA: lessons learned and remaining issues.
Xu, K., Chen, Y., Li, M., Zhang, Y., Zhang, P., and Chen, T. (2025). Research on the mechanism of deformation and instability of surrounding rock in goaf under dynamic loading and the mechanism of active coordinated control. Front. Earth Sci. 13. doi:10.3389/feart.2025.1535868
Yang, G., Song, D., Wang, M., Qiu, L., He, X., Khan, M., et al. (2024). New insights into dynamic disaster monitoring through asynchronous deformation induced coal-gas outburst mechanism of tectonic and raw coal seams. Energy 295, 131063. doi:10.1016/j.energy.2024.131063
Yang, W., and He, S. (2022). Coal mine safety management index system and environmental risk model based on sustainable operation. Sustain. Energy Technol. Assessments 53, 102721. doi:10.1016/j.seta.2022.102721
You, M., Li, S., Li, D., and Xu, S. (2021). Applications of artificial intelligence for coal mine gas risk assessment. Saf. Sci. 143, 105420. doi:10.1016/j.ssci.2021.105420
Yuxin, W., Gui, F., Qian, L., Jingru, W., Yali, W., Meng, H., et al. (2024). Accident case-driven study on the causal modeling and prevention strategies of coal-mine gas-explosion accidents: a systematic analysis of coal-mine accidents in China. Resour. Policy 88, 104425. doi:10.1016/j.resourpol.2023.104425
Zhang, W., Yoshida, T., and Tang, X. (2011). A comparative study of TF*IDF, LSI and multi-words for text classification. Expert Syst. Appl. 38 (3), 2758–2765. doi:10.1016/j.eswa.2010.08.066
Zhao, Y., and Tian, S. (2021). Identification of hidden disaster causing factors in coal mine based on Naive Bayes algorithm. J. Intelligent & Fuzzy Syst. 41, 2823–2831. doi:10.3233/JIFS-202726
Zhao, Y., Yan, Y., Liu, K., Zhao, X., Li, H., Cao, J., et al. (2024). Analysis of coal mine safety accident features in China, 2017–2022. Geohazard Mech. 2 (2), 108–120. doi:10.1016/j.ghm.2024.03.002
Zhao, Y., Zhang, Z., and Jia, T. (2023). Analysis of coal mine safety accidents and research on safety countermeasures in China from 2010 to 2021. Coal Technol. 42 (08), 128–131. doi:10.13301/j.cnki.ct.2023.08.027
Zhu, G.-a., Dou, L.-m., Li, Z.-l., Cai, W., Kong, Y., and Li, J. (2016). Mining-induced stress changes and rock burst control in a variable-thickness coal seam. Arabian J. Geosciences 9 5, 365. doi:10.1007/s12517-016-2356-3
Keywords: coal mine disasters, accident investigation, risk analysis, process safety, safety engineering
Citation: Ouyang Z, Xu Q, Zhang T, Yi H, Zhang N, Xiao M and Ju C (2025) Coupling analysis of disaster-causing factors in coal mines and dual prevention mechanism based on the KeyBERT model and accident-causation theory model. Front. Earth Sci. 13:1586785. doi: 10.3389/feart.2025.1586785
Received: 03 March 2025; Accepted: 26 March 2025;
Published: 09 April 2025.
Edited by:
Xiaojun Feng, China University of Mining and Technology, ChinaReviewed by:
Guojun Liu, Hunan City University, ChinaBin Xiao, Liaoning Technical University, China
Xiangshang Li, China Coal Research Institute, China
Copyright © 2025 Ouyang, Xu, Zhang, Yi, Zhang, Xiao and Ju. This is an open-access article distributed under the terms of the Creative Commons Attribution License (CC BY). The use, distribution or reproduction in other forums is permitted, provided the original author(s) and the copyright owner(s) are credited and that the original publication in this journal is cited, in accordance with accepted academic practice. No use, distribution or reproduction is permitted which does not comply with these terms.
*Correspondence: Qianhai Xu, YnNlYTg4OEAxNjMuY29t