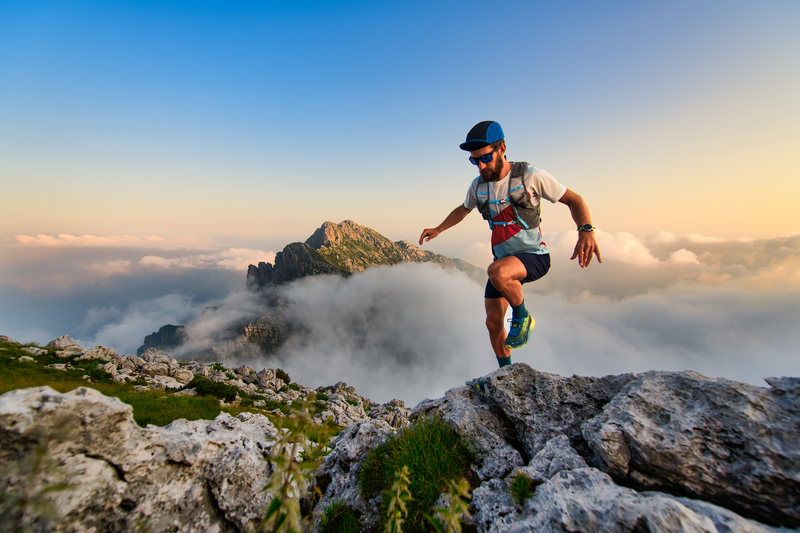
94% of researchers rate our articles as excellent or good
Learn more about the work of our research integrity team to safeguard the quality of each article we publish.
Find out more
ORIGINAL RESEARCH article
Front. Earth Sci.
Sec. Solid Earth Geophysics
Volume 13 - 2025 | doi: 10.3389/feart.2025.1582129
This article is part of the Research Topic Advances in Petrophysics of Unconventional Oil and Gas View all 8 articles
The final, formatted version of the article will be published soon.
You have multiple emails registered with Frontiers:
Please enter your email address:
If you already have an account, please login
You don't have a Frontiers account ? You can register here
Gas hydrate saturation is a critical parameter for quantitative assessment of hydrate reservoirs. To accurately determine the saturation of gas hydrate saturation, it is essential to consider various morphological occurrences of hydrates and the coexistence of hydrates with free gas. In this study, we devised a method for estimating the saturations of gas hydrate and free gas as well as the hydrate morphologies by employing the generalized effective medium model based on the constrained least squares and trust region method. Four hydrate occurrence morphologies, namely contact-cementing, grain-coating, load-bearing, and pore-filling, were integrated into the rock physics model, with the coexistence of hydrates and free gas also being considered. We formulated the inversion as a multi-parameter constrained least squares inversion problem and subsequently adopted then used the trust region algorithm to obtain find the optimal solution for the least least-squares solution. The saturations of gas hydrate and free gas, along with the percentages of hydrate morphologies, were computed using the sonic logs at sites GMGS3-W11 and SH-W01-2017 in the Shenhu Area. The predicted hydrate saturation exhibited comparability to the saturation calculated from resistivity log, NMR log, and chloride data. The gas hydrate saturations predicted for these sites using the rock physics model were found to reach maximum values of 54% and 72%, respectively, while the saturation of free gas could attain up to 22%. The quantitative calculation results of hydrate morphologies reveal that in the Shenhu Area, load-bearing hydrates and pore-filling hydrates predominantly coexist, whereas contact-cementing and grain-coatinghybrid-cementing hydrates are relatively
Keywords: Rock physics, Gas hydrate saturation, Hydrate morphology, Trust region, Least squares
Received: 24 Feb 2025; Accepted: 20 Mar 2025.
Copyright: © 2025 Meng, Zhang, Gu, Zhang, Lu, Lin and Wang. This is an open-access article distributed under the terms of the Creative Commons Attribution License (CC BY). The use, distribution or reproduction in other forums is permitted, provided the original author(s) or licensor are credited and that the original publication in this journal is cited, in accordance with accepted academic practice. No use, distribution or reproduction is permitted which does not comply with these terms.
* Correspondence:
Dajiang Meng, Guangzhou Marine Geological Survey, Guangzhou, China
Disclaimer: All claims expressed in this article are solely those of the authors and do not necessarily represent those of their affiliated organizations, or those of the publisher, the editors and the reviewers. Any product that may be evaluated in this article or claim that may be made by its manufacturer is not guaranteed or endorsed by the publisher.
Research integrity at Frontiers
Learn more about the work of our research integrity team to safeguard the quality of each article we publish.