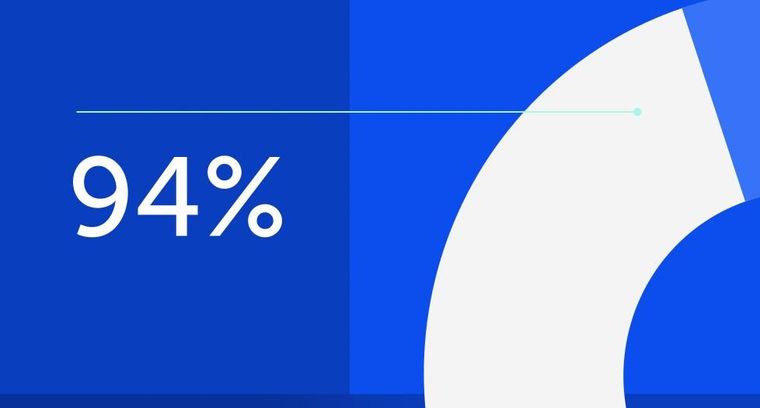
94% of researchers rate our articles as excellent or good
Learn more about the work of our research integrity team to safeguard the quality of each article we publish.
Find out more
ORIGINAL RESEARCH article
Front. Earth Sci.
Sec. Geohazards and Georisks
Volume 13 - 2025 | doi: 10.3389/feart.2025.1577165
The final, formatted version of the article will be published soon.
Select one of your emails
You have multiple emails registered with Frontiers:
Notify me on publication
Please enter your email address:
If you already have an account, please login
You don't have a Frontiers account ? You can register here
This was followed by two additional earthquakes of magnitudes Ms6.0 and Ms5.2. The earthquakes triggered significant geological hazards, impacting Barkam City and surrounding areas. Using Random Forest (RF) and Extreme Gradient Boosting (XGBoost) machine learning models, we assessed landslide susceptibility in Barkam City and identified key influencing factors. The study applied the SHAP method to evaluate the importance of various factors, used UMAP for dimensionality reduction, and employed the HDBSCAN clustering algorithm to classify the data, thereby enhancing the interpretability of the models. The results show that XGBoost outperforms RF in terms of accuracy, precision, recall, F1 score, KC, and MCC. The primary factors influencing landslide occurrence are topographic features, seismic activity, and precipitation intensity. This research not only introduces innovative machine learning techniques and interpretability methods for landslide susceptibility analysis but also provides a scientific foundation for emergency response and post-disaster planning related to landslide risks following the earthquake in Barkam City. keywords:Landslide Susceptibility;Random Forest;XGBoost;UMAP;HDBSCAN
Keywords: Landslide susceptibility, random forest, XGBoost, Umap, HDBSCAN
Received: 15 Feb 2025; Accepted: 24 Mar 2025.
Copyright: © 2025 Xiang Hang, Songhai, Zongqi, Ke, Xiaomin and Ning. This is an open-access article distributed under the terms of the Creative Commons Attribution License (CC BY). The use, distribution or reproduction in other forums is permitted, provided the original author(s) or licensor are credited and that the original publication in this journal is cited, in accordance with accepted academic practice. No use, distribution or reproduction is permitted which does not comply with these terms.
* Correspondence: BU Xiang Hang, Electric Power Research Institute of State Grid Sichuan Electric Power Company, Chengdu, Sichuan Province, China
Disclaimer: All claims expressed in this article are solely those of the authors and do not necessarily represent those of their affiliated organizations, or those of the publisher, the editors and the reviewers. Any product that may be evaluated in this article or claim that may be made by its manufacturer is not guaranteed or endorsed by the publisher.
Supplementary Material
Research integrity at Frontiers
Learn more about the work of our research integrity team to safeguard the quality of each article we publish.