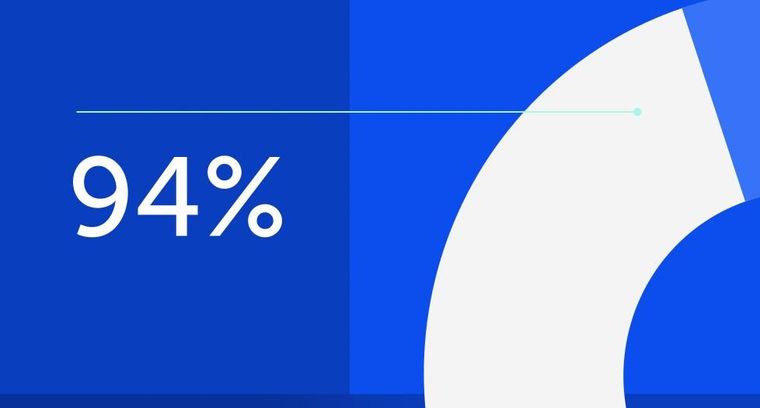
94% of researchers rate our articles as excellent or good
Learn more about the work of our research integrity team to safeguard the quality of each article we publish.
Find out more
ORIGINAL RESEARCH article
Front. Earth Sci.
Sec. Geohazards and Georisks
Volume 13 - 2025 | doi: 10.3389/feart.2025.1564744
This article is part of the Research TopicNatural Disaster Prediction Based on Experimental and Numerical MethodsView all 10 articles
The final, formatted version of the article will be published soon.
Select one of your emails
You have multiple emails registered with Frontiers:
Notify me on publication
Please enter your email address:
If you already have an account, please login
You don't have a Frontiers account ? You can register here
On September 5, 2022, an Ms 6.8 earthquake occurred in Luding County, Sichuan Province, China, triggering numerous landslides and causing extensive damage to buildings and casualties. A comprehensive study of the characteristics of coseismic landslide distribution in this area is highly important for postearthquake emergency response. In this paper, coseismic landslides in highintensity areas were interpreted through remote sensing images, and 5,386 landslides with a total area of 22.2 km 2 were identified. The spatial distribution of coseismic landslides was analyzed in relation to seismic, topographic, and geological factors to assess their susceptibility at the regional scale. The results revealed that the majority of coseismic landslides occurred on both sides of the Xianshuihe fault, which is the causative fault, and the landslides exhibited a linear distribution. These landslides were concentrated mainly at elevations between 1,000 and 1,800 m, with slopes of 30-50°, and they occurred in areas with hard intrusive rock masses. The spatial distribution of coseismic landslides in the study area was predicted using three models: Random Forest (RF), Gradient Boosting Decision Tree (GBDT) and eXtreme Gradient Boosting (XGBoost). Furthermore, SHapley Additive exPlanations (SHAP) theory was used to conduct a quantitative analysis of the main geomorphological factors controlling the landslides. This paper revealed that different topographic factors had varying degrees of nonlinear impacts on landslide formation and that the combined effects of multiple factors, such as the peak ground acceleration (PGA), slope, and lithology, controlled the formation of landslides. This paper highlights the significant advantages of machine learning-based intelligent identification and analytical techniques in landslide disaster emergency surveys and analysis of formation conditions. Rapid prediction of the spatial location and distribution pattern of coseismic landslides provides effective support and guidance for emergency response, risk mitigation, and reconstruction planning.
Keywords: coseismic landslides, 2022 Luding earthquake, spatial distribution, machine learning, SHAP analysis
Received: 22 Jan 2025; Accepted: 10 Apr 2025.
Copyright: © 2025 Ding and Wang. This is an open-access article distributed under the terms of the Creative Commons Attribution License (CC BY). The use, distribution or reproduction in other forums is permitted, provided the original author(s) or licensor are credited and that the original publication in this journal is cited, in accordance with accepted academic practice. No use, distribution or reproduction is permitted which does not comply with these terms.
* Correspondence: Chun Wang, Sichuan Institute of Nuclear Geological Survey, Chengdu, China
Disclaimer: All claims expressed in this article are solely those of the authors and do not necessarily represent those of their affiliated organizations, or those of the publisher, the editors and the reviewers. Any product that may be evaluated in this article or claim that may be made by its manufacturer is not guaranteed or endorsed by the publisher.
Supplementary Material
Research integrity at Frontiers
Learn more about the work of our research integrity team to safeguard the quality of each article we publish.