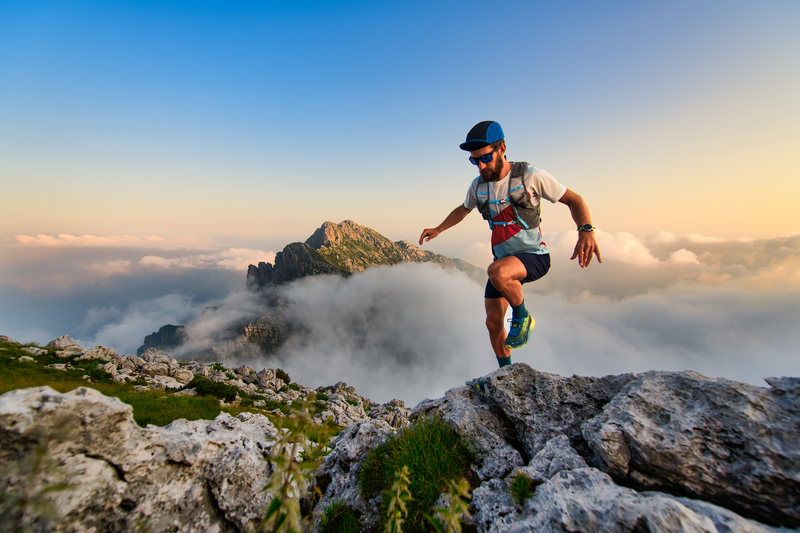
94% of researchers rate our articles as excellent or good
Learn more about the work of our research integrity team to safeguard the quality of each article we publish.
Find out more
ORIGINAL RESEARCH article
Front. Earth Sci.
Sec. Geoinformatics
Volume 13 - 2025 | doi: 10.3389/feart.2025.1554864
The final, formatted version of the article will be published soon.
You have multiple emails registered with Frontiers:
Please enter your email address:
If you already have an account, please login
You don't have a Frontiers account ? You can register here
Tropical cyclones, including surge inundation, are a joint event in the coastal regions of Bangladesh. The surge washes out the life and property within a very short period. Besides, in most cases, the area remains flooded for several days. Prediction of inundation susceptibility due to cyclone surge is one of the key issues in reducing cyclone vulnerability. Surge susceptibility can be analyzed effectively through geospatial techniques and various algorithms. Two geospatial techniques, such as GIS-based Analytical Hierarchy Process (AHP) multi-criteria analysis and bivariate Frequency Ratio (FR) techniques, and three algorithms, i.e., Artificial Neural Network (ANN), k-nearest neighbor (KNN) and Random Forest (RF), were applied to understand the comparative surge inundation susceptibility level between an island, i.e., Sandwip and an area protected by mangrove, i.e., Dacope on the Bangladesh coast. A total of ten criteria were considered influential to surge flooding, i.e., Elevation, Slope, Topographic Wetness Index, Drainage density, Distance from river and sea, Wind flow distance, LULC, NDVI, Precipitation, and Soil types. Among them, distance from river and sea (16.34%) and elevation (15.01%) were found to be crucial to surge inundation susceptibility analysis, according to the AHP expert's opinions. Similarly, precipitation (9.88) and elevation (6.92) in Sandwip and LULC (4.16) and NDVI (4.33) in Dacope were found to be the highest PR values in the FR analysis. The factor maps and final surge susceptibility maps were analyzed through ArcGIS 10.8. The final surge susceptibility maps were categorized into five classes, i.e., very low, low, moderate, high, and very high. Very high and high susceptibility was found around the boundary of Sandwip island and the upper portion of the Dacope upazila. A very high susceptibility area in Sandwip (45.07%) and Dacope (49.41%) was observed by KNN and ANN, respectively. The receiver operating characteristic (ROC) found all techniques acceptable in susceptibility prediction; however, geospatial techniques possessed a better consistent area under the curve (AUC) value than the algorithms for both study sites. Policymakers and professionals can plan to manage disaster reduction activities based on the susceptibility outcomes.
Keywords: AHP, Frequency ratio, ANN, KNN, random forest, Sandwip, Dacope
Received: 08 Jan 2025; Accepted: 25 Feb 2025.
Copyright: © 2025 Mamun, Zhang, Xuzhe, Chen, Zuo, Hasan and Karmakar. This is an open-access article distributed under the terms of the Creative Commons Attribution License (CC BY). The use, distribution or reproduction in other forums is permitted, provided the original author(s) or licensor are credited and that the original publication in this journal is cited, in accordance with accepted academic practice. No use, distribution or reproduction is permitted which does not comply with these terms.
* Correspondence:
Li Zhang, Key Laboratory of Digital Earth Science, Aerospace Information Research Institute, Chinese Academy of Sciences, Beijing, China
Disclaimer: All claims expressed in this article are solely those of the authors and do not necessarily represent those of their affiliated organizations, or those of the publisher, the editors and the reviewers. Any product that may be evaluated in this article or claim that may be made by its manufacturer is not guaranteed or endorsed by the publisher.
Research integrity at Frontiers
Learn more about the work of our research integrity team to safeguard the quality of each article we publish.