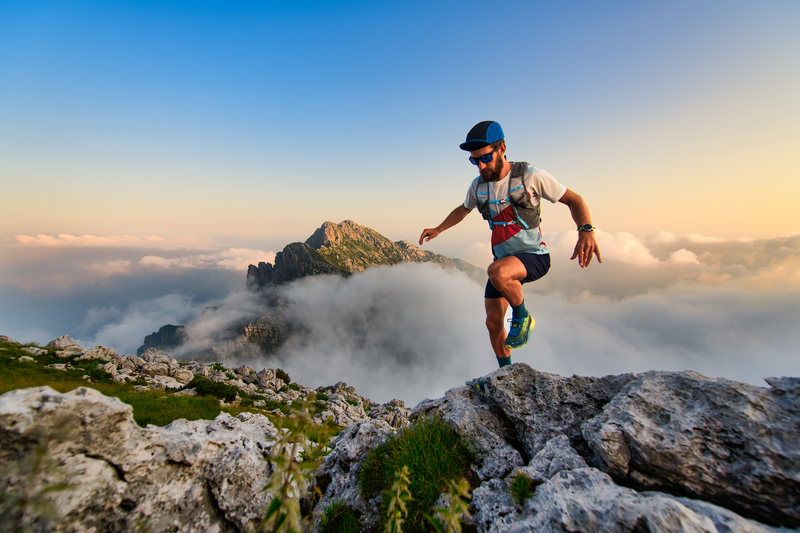
94% of researchers rate our articles as excellent or good
Learn more about the work of our research integrity team to safeguard the quality of each article we publish.
Find out more
ORIGINAL RESEARCH article
Front. Earth Sci.
Sec. Geohazards and Georisks
Volume 13 - 2025 | doi: 10.3389/feart.2025.1549911
This article is part of the Research Topic High-Altitude and Long-Runout Landslide Dynamics: Theory, Methods, and Applications View all 4 articles
The final, formatted version of the article will be published soon.
You have multiple emails registered with Frontiers:
Please enter your email address:
If you already have an account, please login
You don't have a Frontiers account ? You can register here
Driven by rainfall and excavation, frequent landslides in southwestern China pose a serious threat to lives and property. This study uses advanced small baselines subset-interferometric synthetic aperture radar (SBAS-InSAR) time series analysis method and Sentinel-1 satellite data, combined with numerical simulation analysis, to study the deformation and stability characteristics of the Laoyingpan Village landslide under different rainfall and support measures conditions. The study results indicate that the Laoyingpan Village landslide has a 24° average slope, covering approximately 3.8×10 5 m² with a volume of about 1.3×10 7 m³ and an "arm-chair" shape oriented at 106°. Excavation and rainfall are key factors impacting stability, causing significant deformation, especially on the left side with translational failure. SBAS-InSAR analysis indicates a maximum subsidence rate of 25 mm/month in lower slope sections. Under extreme rainfall, the stability coefficient dropped from 1.160 to 0.871, transitioning from stable to unstable before stabilizing after 60 hours due to submergence. The implementation of anti-slide piles and anchor cables improved stability, reaching a coefficient of 1.745 with optimal parameters, recommending a combined support approach for effective landslide management. The study results can provide useful reference and guidance for the stability analysis and engineering prevention of the landslides.
Keywords: landslide, Deformation analysis, SBAS-InSAR, stability, rainfall, Support measures
Received: 22 Dec 2024; Accepted: 11 Mar 2025.
Copyright: © 2025 Zhang, Hu, Zheng, Yu, Guo and Gao. This is an open-access article distributed under the terms of the Creative Commons Attribution License (CC BY). The use, distribution or reproduction in other forums is permitted, provided the original author(s) or licensor are credited and that the original publication in this journal is cited, in accordance with accepted academic practice. No use, distribution or reproduction is permitted which does not comply with these terms.
* Correspondence:
Yitao Hu, Chengdu University of Technology, Chengdu, China
Disclaimer: All claims expressed in this article are solely those of the authors and do not necessarily represent those of their affiliated organizations, or those of the publisher, the editors and the reviewers. Any product that may be evaluated in this article or claim that may be made by its manufacturer is not guaranteed or endorsed by the publisher.
Research integrity at Frontiers
Learn more about the work of our research integrity team to safeguard the quality of each article we publish.