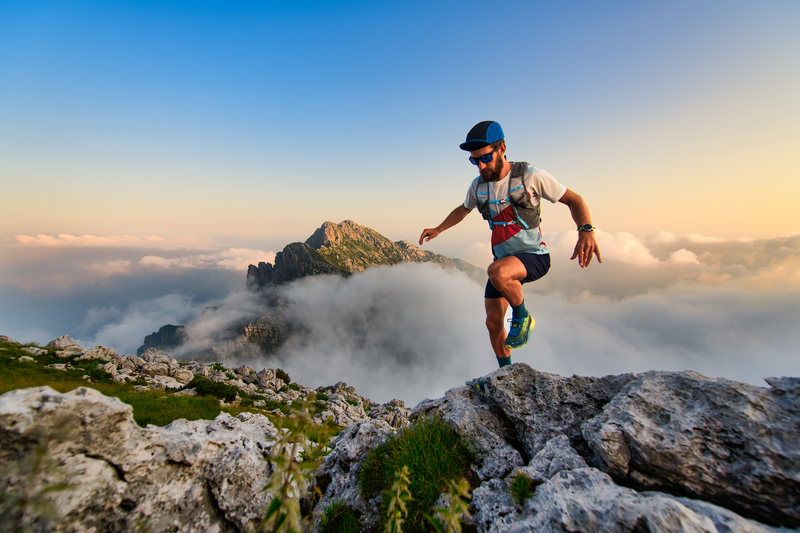
94% of researchers rate our articles as excellent or good
Learn more about the work of our research integrity team to safeguard the quality of each article we publish.
Find out more
ORIGINAL RESEARCH article
Front. Earth Sci.
Sec. Geohazards and Georisks
Volume 13 - 2025 | doi: 10.3389/feart.2025.1546716
This article is part of the Research Topic Failure Analysis and Risk Assessment of Natural Disasters Through Machine Learning and Numerical Simulation: Volume IV View all 21 articles
The final, formatted version of the article will be published soon.
You have multiple emails registered with Frontiers:
Please enter your email address:
If you already have an account, please login
You don't have a Frontiers account ? You can register here
Assessing the lethal resistance levels of buildings during earthquakes is crucial for reducing disaster losses and human casualties. This study proposes a novel model that integrates an improved genetic algorithm (IGA) with an optimized backpropagation neural network (OBPNN) to address data imbalance in classifying building types for lethal resistance levels assessment. The Synthetic Minority Class Oversampling Technique was applied to balance class distributions in the training set by oversampling minority classes. To address overfitting, L2 regularization was combined with a genetic algorithm to optimize the backpropagation neural network (BPNN)'s weights and biases, enhancing global search capability and classification accuracy. Momentum parameters and the Adam optimizer were incorporated to smooth gradient updates, prevent oscillations during training, and accelerate convergence. Additionally, domain adaptation techniques were employed to improve test set performance through feature adaptation, enhancing the model's robustness under varying data distributions and its generalization ability. The experimental results show that the proposed improved model achieves excellent performance in classifying the level of lethal resistance levels of buildings, with an accuracy of 97% and an AUC value of 1, which indicates that the model's generalization and discriminative abilities are more excellent.
Keywords: Genetic algorithm1, Back Propagation2, Synthetic Minority Class Oversampling Technique3, Building Lethality Resistance Levels4, Earthquake5
Received: 17 Dec 2024; Accepted: 24 Feb 2025.
Copyright: © 2025 Jie, Tan, Chaoxu, Yan, Tao and Ma. This is an open-access article distributed under the terms of the Creative Commons Attribution License (CC BY). The use, distribution or reproduction in other forums is permitted, provided the original author(s) or licensor are credited and that the original publication in this journal is cited, in accordance with accepted academic practice. No use, distribution or reproduction is permitted which does not comply with these terms.
* Correspondence:
Bin Tan, Anhui Earthquake Administration, Hefei, China
Disclaimer: All claims expressed in this article are solely those of the authors and do not necessarily represent those of their affiliated organizations, or those of the publisher, the editors and the reviewers. Any product that may be evaluated in this article or claim that may be made by its manufacturer is not guaranteed or endorsed by the publisher.
Research integrity at Frontiers
Learn more about the work of our research integrity team to safeguard the quality of each article we publish.