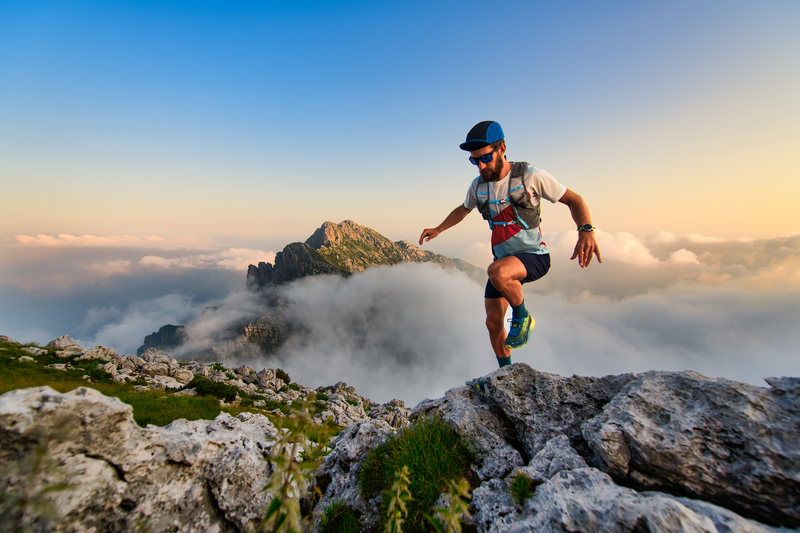
94% of researchers rate our articles as excellent or good
Learn more about the work of our research integrity team to safeguard the quality of each article we publish.
Find out more
ORIGINAL RESEARCH article
Front. Earth Sci.
Sec. Solid Earth Geophysics
Volume 13 - 2025 | doi: 10.3389/feart.2025.1546094
This article is part of the Research Topic Geophysics and Petrophysics Issues Involved in Unconventional Oil and Gas Development Processes View all 8 articles
The final, formatted version of the article will be published soon.
You have multiple emails registered with Frontiers:
Please enter your email address:
If you already have an account, please login
You don't have a Frontiers account ? You can register here
The study area is deep lacustrine shale oil in Mahu, with alkaline lacustrine sediment and strong non-homogeneity, the spatial variation of ROP is fast and cannot be accurately predicted. The selection of drilling parameters and drilling tools are not targeted, especially there is a good correspondence between ROP and the formation resistivity by statistics. In the paper, a new method for well-seismic joint data-driven resistivity-based prediction of 3D spatial ROP based on geologic factors is presented.The advantage of this method is that the geological factors are invariant, and when the engineering factors change and the technological progress is upgraded, only the ROP of the formation needs to be recalibrated with new representative wells. Existing research on ROP prediction mainly focuses on 1D spatial, physical-driven, data-driven, fusion of drilling and logging information, multiple regression, and AI algorithm. The method described in the paper is a new, original, and advanced method. It can be used for accurate prediction of 3D spatial ROP. The specific idea is as follows:Classify the formation hard-to-drill grade based on the unsupervised neural network (UNN) for logging resistivity, construct the resistivity classification template, determine the ROP of each hardto-drill stratigraphy from the ROP of the drilled wells, obtain the 3D spatial resistivity model by using the well-seismic joint data-driven method, classify the resistivity model into hard-to-drill grades and assign the ROP. The geological background of shale oil in Fengcheng formation is summarized, and the current status and difficulties of drilling engineering in the study area are summarized. The method principle and implementation steps are described in detail from five aspects: the relationship between resistivity and ROP, the technical process of predicting 3D spatial ROP, stratigraphy classification based on logging resistivity and calibration of ROP, 3D seismic data processing and facies-controlled resistivity attribute modeling, and prediction of 3D spatial ROP, and an application example of the development well is given. As verified by the example, the predicted ROP of this method is basically consistent with the actual ROP, which effectively guides the selection of drill bits, personalized drill bit design, and optimization of drilling parameters.
Keywords: Well-seismic joint, Data-driven, resistivity, prediction, 3D spatial, rate of penetration, shale
Received: 16 Dec 2024; Accepted: 20 Feb 2025.
Copyright: © 2025 Li, Yu and Tian. This is an open-access article distributed under the terms of the Creative Commons Attribution License (CC BY). The use, distribution or reproduction in other forums is permitted, provided the original author(s) or licensor are credited and that the original publication in this journal is cited, in accordance with accepted academic practice. No use, distribution or reproduction is permitted which does not comply with these terms.
* Correspondence:
Jian-guo Li, CNPC Engineering Technology R & D Company Limited, Beijing, China
Yangfei Yu, Department of Oil and Gas Well Engineering, College of Petroleum Engineering, China University of Petroleum, Beijing, 102249, China
Shouzheng Tian, Department of Oil and Gas Well Engineering, College of Petroleum Engineering, China University of Petroleum, Beijing, 102249, China
Disclaimer: All claims expressed in this article are solely those of the authors and do not necessarily represent those of their affiliated organizations, or those of the publisher, the editors and the reviewers. Any product that may be evaluated in this article or claim that may be made by its manufacturer is not guaranteed or endorsed by the publisher.
Research integrity at Frontiers
Learn more about the work of our research integrity team to safeguard the quality of each article we publish.