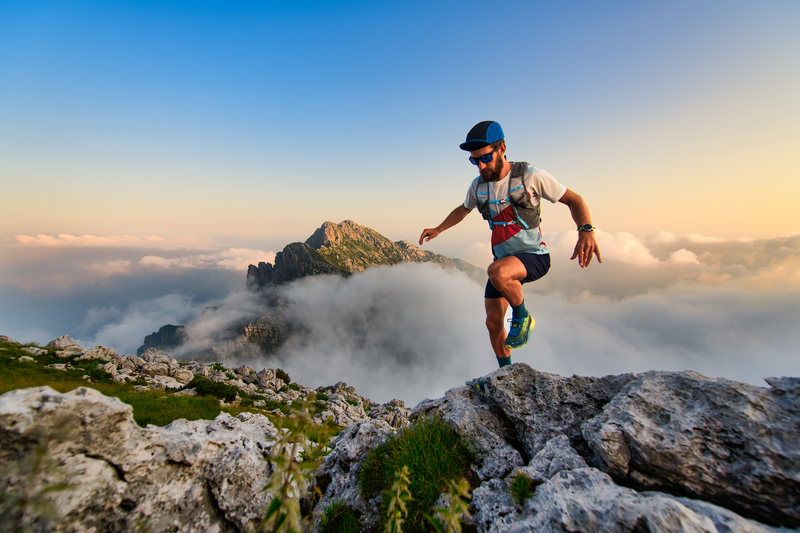
94% of researchers rate our articles as excellent or good
Learn more about the work of our research integrity team to safeguard the quality of each article we publish.
Find out more
METHODS article
Front. Earth Sci.
Sec. Solid Earth Geophysics
Volume 13 - 2025 | doi: 10.3389/feart.2025.1540035
This article is part of the Research Topic Advances in Petrophysics of Unconventional Oil and Gas View all 5 articles
The final, formatted version of the article will be published soon.
You have multiple emails registered with Frontiers:
Please enter your email address:
If you already have an account, please login
You don't have a Frontiers account ? You can register here
Abstract: Lithology identification is a critical task in logging interpretation and reservoir evaluation, with significant implications for recognizing oil and gas reservoirs. The challenge in shale reservoirs lies in the similar logging response characteristics of different lithologies and the imbalanced data scale, leading to fuzzy lithology classification boundaries and increased difficulty in identification.This study focuses on the shale reservoir of the Permian Lucaogou Formation in the Jimusaer Depression for lithology identification. Initially, a comprehensive sampling model -Smote-Tomek(ST) is used to introduce new feature information into the dataset while removing redundant features, effectively addressing the issue of data imbalance. Then, by combining the multi-objective optimization strategy Artificial Rabbit Optimization (ARO) with the Light Gradient Boosting Machine (LightGBM) model, a new intelligent lithology identification model (ST-ARO-LightGBM) is proposed, aimed at solving the problem of non-optimal hyperparameter settings in the model.Finally, the proposed new intelligent lithology identification model is compared and analyzed with six models: K-Nearest Neighbors (KNN), Decision Tree (DT), Gradient Boosting Decision Tree (GBDT), Random Forest (RF), Extreme Gradient Boosting (XGBoost), and LightGBM, all after comprehensive sampling. The experimental results show that the ST-ARO-LightGBM model outperforms other classification models in terms of classification evaluation metrics for different lithologies, with an overall classification accuracy improvement of 9.13%. The method proposed in this paper can solve the problem of non-equilibrium in rock samples, and can further improve the classification performance of traditional machine learning, and provide a method reference for the lithology classification of shale reservoirs.
Keywords: Shale reservoir, Lithology identification, multi-objective optimization, Artificial Rabbit Optimization Model, Integrated learning model, comprehensive sampling
Received: 05 Dec 2024; Accepted: 12 Feb 2025.
Copyright: © 2025 Deng, Li, Chen and Feng. This is an open-access article distributed under the terms of the Creative Commons Attribution License (CC BY). The use, distribution or reproduction in other forums is permitted, provided the original author(s) or licensor are credited and that the original publication in this journal is cited, in accordance with accepted academic practice. No use, distribution or reproduction is permitted which does not comply with these terms.
* Correspondence:
Cheng Feng, China University of Petroleum Beijing, Karamay Campus, Karamay, Xinjiang, China
Disclaimer: All claims expressed in this article are solely those of the authors and do not necessarily represent those of their affiliated organizations, or those of the publisher, the editors and the reviewers. Any product that may be evaluated in this article or claim that may be made by its manufacturer is not guaranteed or endorsed by the publisher.
Research integrity at Frontiers
Learn more about the work of our research integrity team to safeguard the quality of each article we publish.