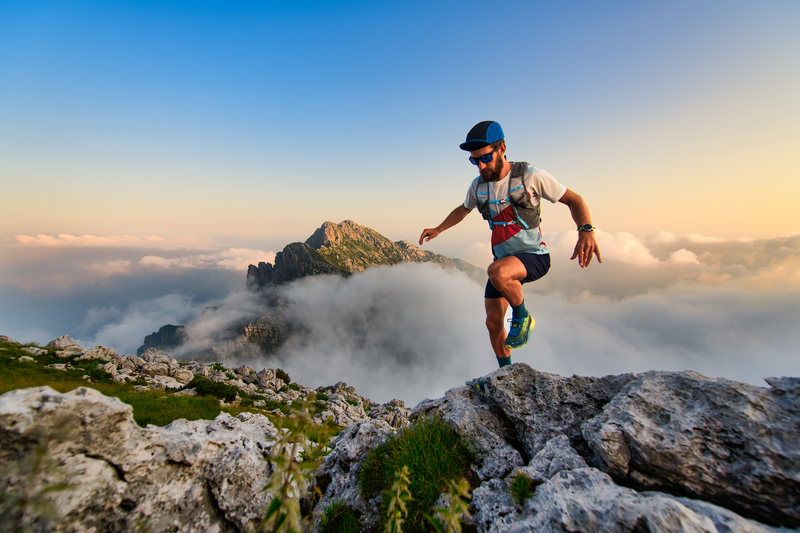
95% of researchers rate our articles as excellent or good
Learn more about the work of our research integrity team to safeguard the quality of each article we publish.
Find out more
ORIGINAL RESEARCH article
Front. Earth Sci. , 02 April 2025
Sec. Geohazards and Georisks
Volume 13 - 2025 | https://doi.org/10.3389/feart.2025.1536412
Introduction: Frequent geohazards along highways in the western Sichuan Plateau gradient zone severely constrain regional development. To clarify the spatial distribution laws and main drivers of highway geohazards is crucial for geohazard prevention and control.
Methods: This study analyzed 2230 geohazard sites within 3 km of 11 highways in the western Sichuan Plateau gradient zone, considering both internal and external geodynamic factors. Additionally, the study further quantified the influence of each conditioning factor on the distribution of geohazards using the Geographical Detector Model (GDM).
Results: The following conclusions were drawn: (1) The density of geohazard sites along highways in the gradient zone is high, averaging 1.16 sites per kilometer. (2) Highway geohazards exhibit spatial clustering, with higher densities observed in the central part of the gradient zone (e.g., Duwen and Wenma highways) and lower densities at the periphery (e.g., Mianjiu and Majiu highways). (3) The distribution of highway geohazards is significantly influenced by distance to fault zones, vertical deformation gradients, and rainfall, with q values of 0.39, 0.37, and 0.31, respectively. (4) The interaction between any two conditioning factors exhibits a higher q value for geohazard distribution than individual factors.
Discussion: Driven by the multi-sphere coupling effects among the tectonic deformation sphere, rock mass relaxation sphere, atmospheric sphere, and engineering disturbance sphere, a geohazard triggering mechanism has been established. This mechanism is primarily controlled by factors such as the distance from the fault zone, vertical deformation gradient, and rainfall, while other factors like elevation and slope serve as secondary controlling factors. The nonlinear synergistic effects between the primary controlling factors and secondary controlling factors significantly amplify the probability of disaster risk. The results quantitatively characterizes the driving factors of geohazards and their nonlinear synergistic effects. It can provide a reference for geological disaster monitoring, preparation of post-disaster emergency measures, and highway planning.
The western Sichuan Plateau gradient zone, located along the eastern edge of the Qinghai-Tibet Plateau, is characterized by highly complex topography and geological conditions, including steep terrain, high earthquake intensity, fragile geological environments, and frequent geohazards (Gong et al., 2022; Wang et al., 2022; Xu et al., 2022; Li et al., 2024). The region has critical transportation corridors, such as the Wenma and Yakang highways, and major national infrastructure projects, including the Sichuan-Tibet Railway, are currently under construction (Peng et al., 2004). However, numerous geohazards, such as the “4.1 Ya’an Landslide” in 2016, the “6.24 Maoxian Landslide” in 2017 (Wang et al., 2018; Yang et al., 2020), and the “8.21 Jinyang Debris Flow” in 2023, pose substantial risks to the normal operation and safety of regional highways (Peng et al., 2020; Lu and Cai, 2019; Yi et al., 2023). Geohazards along highways result in both direct losses, such as casualties and physical damage to infrastructure requiring costly replacement, repairs, or maintenance, and indirect losses, such as reduced productivity across multiple economic sectors (Zhang et al., 2021). According to incomplete statistics (Zhang et al., 2017), geohazards have caused direct and indirect losses amounting to billions of RMB in road infrastructure projects in the region.
Following the “5.12” Wenchuan Earthquake, an increasing number of studies have focused on the western Sichuan Plateau gradient zone. Field investigations and remote sensing techniques have been used to analyze development and distribution at different scales and in different regions. These studies have systematically discussed the development and distribution of geohazards (Huang and Li, 2008; Zhao et al., 2024), with a particular emphasis on the controlling role and sensitivity of different conditioning factors (Yang et al., 2018; Fan et al., 2018; Shu et al., 2022; Gu et al., 2023). For example, studies on landslide distribution along highways and adjacent areas have demonstrated that landslides not only exhibit distinct geomorphic features but also possess specific ground movement characteristics (Chen et al., 2022).
Geohazards arise from interactions among Earth’s deep geological processes, surface systems, atmospheric conditions, and human activities. These interactions form a feedback system involving tectonic deformation, rock mass loosening, atmospheric processes, and engineering disturbances. This feedback profoundly influences regional geological stability and the dynamic mechanisms driving geohazards, as shown in Figure 1 (Peng et al., 2023). The occurrence of geohazards is governed by various geodynamic factors, including slope, rainfall, lithology, and seismic activity, which collectively influence the frequency and severity of these events (Malamud et al., 2004; Xu et al., 2014). Earthquake-induced landslides along highways exhibit notable spatial characteristics, with large-scale landslides typically occurring near watershed outlets and smaller ones concentrated in mid-watershed sections (Liu et al., 2021). These variations in geohazard distribution are primarily driven by natural triggering factors, while human influences play a secondary role (Chen et al., 2021).
Current research has largely focused on topographic, geomorphic, and surface indicators influencing geohazard development and distribution (Convertino et al., 2013; Dandridge et al., 2023). For example, landslide density has been found to increase with higher rainfall levels over various timescales rather than specific rainfall intensities (Mtibaa and Tsunetaka, 2023). Similarly, a study in the Lhasa region of Tibet revealed that landslides are predominantly concentrated at elevations of 4,300–5,300 m and relief ranges of 1,100–1,500 m. The landslide abundance index consistently increases as hillslope gradients increase (Ma et al., 2024). Furthermore, deep geodynamic processes, such as crustal movements and tectonic stress fields, are critical drivers of geohazards (Cloetingh and Willett, 2013; Xie et al., 2021). Despite these advancements, research on the distribution laws of highway geohazards in the western Sichuan Plateau gradient zone remains limited. Additionally, quantitative studies examining the role of deep geodynamic factors are sparse. Further research is required to elucidate the development, controlling factors, and spatial distribution of highway geohazards in this region.
To study the distribution laws of geohazards, commonly employed methods include logistic regression, weight of evidence analysis, frequency ratio, and random forest models (Zhang et al., 2022b; Huang and Chen, 2024). These approaches have been used to analyze the spatial distribution and conditioning factors of various geohazards (Qiu et al., 2019; Ling et al., 2021). However, due to the complex nonlinear characteristics of geohazards, existing methods face challenges in factor selection, spatial correlation analysis, and significance evaluation (Huang et al., 2022; Loche et al., 2022). Established methods are limited in their ability to deal with nonlinear and complex interaction problems, and spatial heterogeneity analysis is weak (Yu et al., 2019; Quevedo et al., 2022). The Geographical Detector Model (GDM) is an innovative statistical tool designed to measure spatial heterogeneity and analyze factor contributions. Compared to traditional methods, this model offers several key advantages: first, it employs stratified heterogeneity testing (q-statistic) for quantitative analysis of factor contributions, overcoming the assumptions of traditional linear regression; second, it utilizes interaction detectors to identify nonlinear coupling effects among multiple factors, clearly distinguishing between synergistic and independent mechanisms; finally, the model accommodates mixed analysis of categorical and continuous variables, preserving the physical significance of geological classification data (Wang et al., 2016; Chen et al., 2023).
Using the GDM, this study investigates the development and distribution laws of highway slope geohazards in the western Sichuan Plateau gradient zone, under the combined influence of internal and external geodynamic factors. Using the factor detector model, key controlling factors of geohazards along highways were identified. By applying the interaction detector to spatially heterogeneous data, the coupling effects between deep-seated dynamic factors and surface processes were quantified, providing a scientific basis for geohazard risk management in regional highway systems.
The western Sichuan Plateau gradient zone extends from the hilly regions on the western edge of the Sichuan Basin to the Longmenshan Mountains in the northwest and the eastern margins of the Tibetan Plateau. This region has an extremely complex and unique geological environment, as illustrated in Figure 2. The total area of the study region is approximately 206,400 km2.The gradient zone spans several geomorphological units, including alluvial plains, eroded low hills, tectonically eroded low-mid mountain landforms, and tectonically eroded medium-high mountain landforms. The terrain gradually ascends from the eastern plains near the Sichuan Basin, with elevations starting at approximately 500 m, to the eastern edge of the Tibetan Plateau, reaching elevations of approximately 4,000 m. This elevation change occurs over a relatively short horizontal distance of 150–200 km, resulting in pronounced topographic elevation differences. The sharp changes in elevation and the distribution of mountain ranges create substantial variability in the region’s local climate, resulting in a diversity of climatic zones. The eastern part of the gradient zone is characterized by a relatively humid monsoon climate, whereas the western part experiences a more arid mountainous climate. Several major rivers, including the Yalong, Dadu, Qingyi, and Min rivers, flow across the region, forming a complex watershed system that supports local ecosystems and human activities.
Figure 2. Location of the study area (a) Map of China. (b) Map of Sichuan Province. (c) Geographic location of the western Sichuan Plateau gradient zone and distribution of highway geohazards.
Geologically, the study area lies at the intersection of the western margin of the Yangtze Paraplatform and the Songpan-Ganzi Orogenic Belt, intersecting with the Longmenshan Orogenic Belt, Xianshuihe Fault Zone, and the north-south tectonic zone of Sichuan-Yunnan. This region is characterized by shallow seismic focal depths, high earthquake magnitudes, and short seismic cycles. Historically, the area has experienced several major earthquakes, including the 1933 Diexi earthquake, the 2008 Wenchuan earthquake, and the 2013 Lushan earthquake. These geological and tectonic conditions, combined with the steep topography, have led to frequent slope-related geohazards such as landslides, collapses, and debris flows. Multiple highways have also been constructed in the region, and the prevalence of geohazards poses significant challenges to transportation safety and engineering construction.
Data were collected for 11 highways, both completed and under construction, within the Plateau gradient zone. For highways that extend beyond the Plateau gradient zone, such as the Yanjiang Highway, only the sections within the gradient zone were analyzed. The total highway mileage within the study area was 1,931 km. Among these, the Yaxi Highway (293 km) and the Lexi Highway (288 km) were the longest routes, while the Guanggan Highway (58.6 km) and the Duwen Highway (72 km) were the shortest routes. A total of 2,230 geohazards located within 3 km of the highways, the zone most affected by engineering activities, were recorded and analyzed. These included 1,213 landslides, 506 collapses, and 511 debris flows.
To explore the spatial distribution of highway geohazards under the influence of internal and external geodynamic factors, four external geodynamic factors—elevation, slope angle, intensity of human engineering activities, and rainfall—and five internal geodynamic factors—distance from fault zones, engineering geological rock groups, Bouguer gravity anomaly gradient, vertical deformation gradient, and horizontal deformation gradient—were selected for analysis.
Data sources included: 30 m-resolution digital elevation model (DEM) data from the Shuttle Radar Topography Mission (SRTM) of NASA; 30 m-resolution Google Maps data; Normalized Difference Vegetation Index (NDVI) data for 2022 derived from the Google Earth Engine; annual rainfall data from the National Climate Center; regional engineering geology and fault zone locations provided by the China Geological Survey; and Bouguer gravity anomaly gradient, vertical deformation gradient, and horizontal deformation gradient data from Yao et al. (2015).
This study employed numerical statistical methods to investigate the spatial distribution of highway geohazards. The influence of individual conditioning factors and combinations of factors on geohazard distribution were quantified using the GDM (Wang et al., 2016). The methodology is illustrated in Figure 3.
The GDM is based on the theory of spatial differentiation. It employs spatial statistical techniques to detect and quantify the interactions among conditioning factors, thereby uncovering their underlying driving mechanisms. The model comprises four sub-models: the factor detector, interaction detector, risk detector, and ecological detector.
The factor detector was used to measure the influence of individual conditioning factors on the spatial distribution of geohazards. The interaction detector was employed to evaluate the combined influence of two conditioning factors. The q value of each factor is calculated as follows:
where q is the explanatory power of the conditioning factor; nh and σh are the sample size and variance of the driving factor in layer h, respectively; n and σ2 are the full sample size and variance, respectively. The q value ranges from 0 to 1, with higher values indicating stronger explanatory power and greater influence on geohazard distribution.
The spatial distribution of geohazards along highways in the western Sichuan Plateau gradient zone is shown in Figure 2. The density of geohazard sites (number of geohazard sites along the highway per kilometer) was 1.16 sites/km, indicating a high overall density along these routes.
Figure 4 illustrates the variation in geohazard density across different highways. The Duwen Highway and Wenma Highway, located in the central Plateau gradient zone, exhibit the highest geohazard densities of 5.15 and 3.01 sites/km, respectively. Conversely, the Mianjiu Highway and Maju Highway, situated at the periphery of the gradient zone, exhibit relatively low densities of 0.69 and 0.34 sites/km, respectively. This is primarily because the central zone, characterized by alpine and valley landscapes, has complex geological formations and fragile environments, providing favorable conditions for large-scale geohazards (Li et al., 2024).
Elevation was divided into six levels: 191–1,000 m, 1,000–1,500 m, 1,500–2,000 m, 2,000–2,500 m, 2,500–3,000 m, and >3,000 m. The spatial distribution of elevation levels is shown in Figure 5. Near the Chengdu Plain, elevation was primarily in the range of 191–1,000 m, while the central gradient zone exhibited elevations of 1,000–3,000 m, and elevations exceeding 2,500 m were predominant in the western part of the gradient zone.
Geohazard densities and counts are also illustrated in Figure 5. Geohazard densities and counts were highest at 1,500–2,000 m, with 481 sites and a density of 219.87 sites/10,000 km2. The 1,000–1,500 m level ranked second, with 480 sites and a density of 200.33 sites/10,000 km2. In contrast, geohazard densities and counts were lowest at elevations >3,000 m, with 212 sites and a density of 26.09 sites/10,000 km2. Geohazard occurrence generally increased with elevation before declining beyond 2,500 m.
Climate and human activities are the fundamental drivers that control the spatial distribution of vegetation and its changes. The ecological system in the western Sichuan Plateau gradient zone is sensitive and fragile. During large-scale engineering construction, activities such as earthwork filling and excavation, construction operations, and the presence of inorganic and heavy metal pollution can create ecological disturbance corridors. These disturbances can lead to a variety of ecological and environmental issues (Sun et al., 2010; Luo et al., 2018). NDVI data obtained via remote sensing can be used to evaluate vegetation growth and can serve as a proxy for the intensity of human engineering activities in the region (Ma et al., 2021). Based on NDVI values, the intensity of human engineering activities were categorized into four levels: extremely high (0–0.25), high (0.25–0.50), medium (0.50–0.75), and low (0.75–1). Areas with NDVI values < 0, characterized by high visible light reflectance, were also categorized as low-intensity zones. The spatial distribution of activity intensity levels is shown in Figure 6. Medium-intensity zones accounted for the largest proportion (60.91%) of the gradient zone area, while high- and extremely high-intensity zones were concentrated near towns and construction sites.
Figure 6. Distribution and numerical statistics of geohazards at different levels of human engineering activity intensity.
Geohazard counts and densities for different levels of human engineering intensity are also shown in Figure 6. Owing to the relatively large proportion of medium- and high-intensity zones in the study area, geohazard numbers were highest in these zones with 1,308 and 767 sites, respectively. In contrast, geohazard numbers were lower in low- and extremely high-intensity zones. However, geohazard density exhibited a marked upward trend as the intensity of human engineering activity increased. Overall, human engineering activities promote geohazard development.
Rainfall is the main factor inducing landslides, such as the Darenyan landslide along the Yakang Highway (Song and Tan, 2021; Liu et al., 2023). Based on annual average values, rainfall was categorized into six levels: 500–800 mm, 800–1,000 mm, 1,000–1,200 mm, 1,200–1,400 mm, 1,400–1,800 mm, and 1,800–2,400 mm (Figure 7). Rainfall distribution was extremely uneven across the gradient zone in the western Sichuan Plateau. The six rainfall levels occupied areas of 91,100 km2, 50,200 km2, 42,600 km2, 12,400 km2, 7,900 km2, 2,200 km2, accounting for 44.1%, 24.3%, 20.7%, 6.0%, 3.8%, and 1.1% of the total area of the gradient zone, respectively.
Geohazard counts and densities for different annual average rainfall levels are also shown in Figure 7. The area occupied by the three lowest rainfall levels (i.e., 500–800 mm, 800–1,000 mm, and 1,000–1,200 mm) accounted for 89.1% of the total area of the gradient zone. The vast majority of highway routes were distributed within this area, leading to the high number of geohazards in these areas. However, the overall geohazard density was low. In contrast, the 1,400–1,800 mm level rainfall area only accounted for 3.8% of the entire gradient zone area. However, the number of geohazards within this area accounted for 21.7% of the total number of geohazards. The geohazard distribution density in this area was 611.73/10,000 km2, far exceeding the geohazard distribution densities of the three lowest rainfall levels. Thus, rainfall has a significant triggering effect on geohazards. The 1,800–2,400 mm level rainfall area accounted for only 1.1% of the entire gradient zone, and this area was primarily uninhabited, with few highways passing through it. This resulted in a low number of geohazards and a lower density of geohazards than that in the 1,400–1,800 mm level.
The slope angle, defined as the degree of steepness of a slope unit, directly affects the gravitational force acting along the slope. An increase in slope angle corresponds to an increase in the gravitational force acting downslope, which in turn raises the probability of slope collapse or sliding of rock and soil masses (Weng et al., 2015; Xie et al., 2019). Slope angles were classified into six categories:0°–10°, 10°–20°, 20°–30°, 30°–40°, 40°–50° and >50°. The spatial distribution of slope angles in the study area is shown in Figure 8. It is evident that the slope angles in the vicinity of the Chengdu Plain and the Songpan and Ruoergai grassland areas predominantly fall in the 0°–10° range, whereas slope angles in other regions primarily fall within the 10°–40° range.
The distribution of geohazards across these slope angle categories is also depicted in Figure 8. Zones with slope angles of 10°–20° exhibited the highest number of geohazards, with 645 sites, followed by zones with slope angles of 20°–30°, with 564 sites. Conversely, the zones with slope angles >50° had the lowest number of geohazards, with only 42 sites. These findings indicate a trend of increasing geohazard occurrence with slope angle, followed by a subsequent decline.
Fault zones, as critical geological structures, play a significant role in inducing geohazards. They disrupt the continuity of rock and soil, reduce slope strength, and create conditions conducive to the sliding of rock and soil masses (Qi et al., 2021). The distance from fault zones was categorized into five levels: 0–10 km, 10–20 km, 20–30 km, 30–40 km and >40 km.
The number of geohazards located at different distances from fault zones is shown in Figure 9. The 0–10 km category contained the highest number of geohazards, with 1,114 sites. This was followed by the 10–20 km category, which contained 395 sites. The 20–30 km category had the lowest number of geohazards, with only 185 sites. These results demonstrate that the number of geohazards is significantly higher as proximity to fault zones increases.
Figure 9. Distribution and numerical statistics of geohazards at different distances from fault zones.
The study area encompasses a wide geographical area and is located in a tectonically active region and is characterized by the exposure of Neoproterozoic to Quaternary strata. Jurassic and Triassic formations exhibit the broadest spatial distribution, collectively accounting for over 30% of the region. Engineering geological rock groups were classified into four levels based on their origin, hardness, degree of intactness and regional tectonic features: poor, fair, good, and excellent (Yang et al., 2018). Figure 10 shows the spatial distribution of these categories. The excellent category covers the largest proportion of the study area, whereas the poor and fair categories occupy relatively smaller areas.
Figure 10. Distribution and numerical statistics of geohazards at different engineering geology rock group levels.
The distribution of geohazards across these rock group categories is presented in Figure 10. The excellent engineering geological rock group contains the highest number of geohazards (1,617 sites) due to its large spatial extent. However, the densities of geohazards is much higher in the poor and fair rock group categories than it is in the good and excellent rock group categories. Notably, the fair rock group category exhibited the highest geohazard density, with 256.99 sites/km2. These findings suggest that as the quality of engineering geological rock groups decreases, the susceptibility to geohazards increases.
Deep tectonic activity is an important factor controlling crustal stability, and changes in the geophysical field serve as indicators of such activity. The Bouguer gravity anomaly gradient, calculated as the rate of change in Bouguer gravity anomaly values within each unit area (20 km2), was used as a measure of regional crustal stability (Fu et al., 2014). The gradient was categorized into four levels: low (0–2), medium (2–5), high (5–10), and extremely high (>10). Figure 11 illustrates the spatial distribution of these categories. The Longmenshan Orogenic Belt, particularly in the Kangding–Xichang, Wenchuan–Lixian, and Pingwu regions, exhibits the steepest gradients, reflecting vertical gravitational imbalances in deep crustal materials. This imbalance will inevitably reach equilibrium through lateral movement. This is consistent with the deep material flow and lateral compression mechanism in the SEE direction of the Longmenshan tectonic belt on the western Sichuan Plateau.
Figure 11. Distribution and numerical statistics of geohazards at different Bouguer gravity anomaly gradient levels.
Geohazard distributions across the gradient levels are shown in Figure 11. The high (5–10) and extremely high (>10) gradient levels exhibited the higher geohazard counts, with 1,083 and 425 sites, respectively. The geohazard densities in these categories were 123.57 and 207.79 sites/10,000 km2, respectively. These results reveal an increasing trend in geohazard occurrence with higher Bouguer gravity anomaly gradients.
Regional crustal deformation includes both horizontal and vertical components, which are closely associated with seismic activity and tectonic stress concentrations (Huang et al., 2018). Regions with large vertical deformation gradient are often unstable or seismic zones, while regions with large horizontal deformation gradient are often zones of tectonic stress concentration.
The vertical deformation gradient, measured as the annual average vertical deformation within an area of 10,000 km2, was categorized into four levels: low (0–1), medium (1–2), high (2–3), and extremely high (3–5.4). Figure 12 illustrates the spatial distribution of these categories, with prominent high-gradient zones observed in the Puge–Xichang and Mianning–Kangding–Lixian regions (near-SN orientation) and the Xichang–Daocheng gradient zone (SE–NW orientation).
Figure 12. Distribution and numerical statistics of geohazards at different vertical deformation gradient levels.
Geohazard counts and densities in the various vertical deformation gradient categories are shown in Figure 12. The high (2–3) and extremely high (3–5.4) gradient levels exhibited the highest geohazard occurrences, number with 821 and 760 respectively, densities with177.33 and 235.76 sites/10,000 km2, respectively. These findings indicate that higher vertical deformation gradients are more conducive to geohazard development.
The horizontal deformation gradient, defined as the annual average horizontal deformation within an area of 3 km2, was classified into four levels: low (0–0.5), medium (0.5–1), high (1–1.5), and extremely high (1.5–7). Figure 13 depicts the spatial distribution of these categories. Low-gradient zones were predominant in the Sichuan Basin, Bayankala region, and Sichuan–Yunnan rhombic region, whereas high-gradient zones were concentrated along the NW-oriented Ganzi–Yushu–Xianshuihe fault zone and the near-SN-oriented Anninghe–Zemuhe fault zone.
Figure 13. Distribution and numerical statistics of geohazards at different horizontal deformation gradient levels.
Geohazard counts and densities across horizontal deformation gradient levels are shown in Figure 13. The low (0–0.5) level exhibited the highest number of geohazards, with 1,053 sites. As the horizontal deformation gradient increased, the overall number of geohazards showed a decreasing trend. The distribution densities for the low (0–0.5), medium (0.5–1), and high (1–1.5) levels did not differ significantly. However, the extremely high (1.5–7) level exhibited the highest density of geohazards, at 166.98 sites/10,000 km2. Overall, compared to other factors, the influence of horizontal deformation gradient on the distribution of geohazards is limited, which is caused by various reasons. The overall horizontal deformation within the western Sichuan Plateau gradient zone is in the southeast direction (Zhang et al., 2022a). When the horizontal deformation direction is opposite to the slope direction, the probability of geohazards will be significantly reduced (Li et al., 2024). Moreover, the horizontal deformation gradient within the western Sichuan Plateau gradient zone is dominated by low to medium levels, with relatively few areas of high to extremely high levels. The deformation magnitude does not reach the critical threshold required for geohazards occurrence. The combination of multiple factors results in a relatively small influence of horizontal deformation gradient on the distribution of geohazards.
The spatial heterogeneity of geohazards was analyzed using a GDM following three main steps: data collection and preprocessing, sample reading and result interpretation. First, data were collected for each conditioning factor and classified according to the requirements of the GDM. These classified factors served as the independent variables X of the model. The density of geohazards in the study area was calculated as the dependent variable Y of the GDM. Classification values corresponding to each geo-environmental factor X and geohazard density Y were statistically combined into a dataset, i.e., [X1n, X2n, X3n, ……X9n, Yn], with n being the number of samples. These samples (X, Y) were input into the GDM software to calculate factor detector and interaction detector results.
In this study, following the principle of prioritizing geological significance and assisting with statistical optimization, various conditional factors were stratified. For the four conditioning factors—engineering geology rock groups, Bouguer gravity anomaly gradient, vertical deformation gradient, and horizontal deformation gradient—their regional tectonic characteristics and geological significance should be fully integrated into the spatial stratification. For the continuous conditioning factors of elevation, slope, rainfall, intensity of human engineering activities, and distance from the fault zone, the equidistant method is employed for stratification. Furthermore, this stratification is adjusted based on regional terrain and landform characteristics to ensure it aligns with actual conditions. The specific stratification results and density calculations have been completed in Section 4.2.
The GDM assessed the importance of nine evaluation factors: elevation (X1), slope angle (X2), rainfall (X3), intensity of human engineering activities (X4), engineering geology rock group (X5), distance from the fault zone (X6), Bouguer gravity anomaly gradient (X7), vertical deformation gradient (X8), and horizontal deformation gradient (X9). The q values for each factor’s influence on geohazard distribution are shown in Figure 14.
Among all the factors, the distance from the fault zone (q = 0.39), vertical deformation gradient (q = 0.37), and rainfall (q = 0.31) had the most significant impacts on the distribution of geohazards along highways in the western Sichuan Plateau gradient zone. Secondary factors included the intensity of human engineering activities (q = 0.30), engineering geology rock groups (q = 0.26), and slope angle (q = 0.23). In contrast, the Bouguer gravity anomaly gradient, horizontal deformation gradient, and elevation exhibited relatively minor effects.
The occurrence of geohazards is governed by the interaction of internal and external factors. Combinations of these geological and environmental factors lead to variations in the spatial distribution of geohazards. The results of interaction detection (Figure 15) revealed that interactions among factors primarily exhibited a nonlinear enhancement effect, with the q value of any two interacting factors surpassing that of individual factors. Specifically, the interactions between rainfall and the vertical deformation gradient (q = 0.60) and between engineering geology rock group and vertical deformation gradient (q = 0.62) had the most pronounced effects on geohazard distribution.
Figure 15. Interaction detection results of different conditioning factors on geohazard distribution.
For the GDM, the primary purpose is to identify and quantify the relationships between spatial variables, rather than to conduct traditional predictive evaluations. In this context, the Bootstrap method is employed to evaluate the model through repeated sampling (Savvides et al., 2023). Assuming a resampling frequency of B = 2000, a total of 2000 Bootstrap samples were generated by randomly resampling (with replacement) 2230 original geohazard datasets. For each Bootstrap sample, the GDM is used to recalculate the q values of all conditional factors. Subsequently, the average q value, standard deviation, and 95% confidence interval for each factor can be derived from the Bootstrap distribution.
Taking the three factors of distance from the fault zone, vertical deformation gradient, and rainfall as examples, which have the highest q value and the greatest impact on the distribution of geohazards. After calculations, the q values obtained through Bootstrap demonstrate strong consistency with the original values. The factors of distance from the fault zone (q = 0.40 ± 0.02), vertical deformation gradient (q = 0.36 ± 0.01), and rainfall (q = 0.32 ± 0.02) remain dominant, all exhibiting narrow confidence intervals (relative error <5%). The nonlinear enhancement effect between rainfall and vertical deformation gradient (q = 0.61 ± 0.03) remains statistically significant. This validation indicates that the distribution law of geohazards on highways, based on GDM, is statistically robust against sampling variability and can provide reliable guidance for geohazard management.
Taking the Lexi Highway within the western Sichuan Plateau gradient zone and the Chengdu-Neijiang section of the Chengyu Highway outside this zone as examples, this study analyzes the regional applicability of geohazard distribution law. As illustrated in Figure 16, 126 geohazard sites were identified within a 3-km buffer zone along the Lexi Highway, compared to 73 geohazard sites along the Chengyu highway (Yang et al., 2023). The GDM was employed to assess the influence of internal and external geodynamic factors on the spatial distribution of geohazards.
Figure 17 shows the q values of conditioning factors on geohazard distribution along the Lexi and Chengyu Highways. Results reveal that distance from the fault zone (q = 0.38), vertical deformation gradient (q = 0.35), and rainfall (q = 0.34) dominate geohazard distribution along the Lexi Highway. Secondary factors include intensity of human engineering activities (q = 0.31), engineering geology rock group (q = 0.28), and slope angle (q = 0.25). Elevation, Bouguer gravity anomaly gradient, and horizontal deformation gradient show minimal impacts. This aligns with the overall geohazard distribution law in the western Sichuan Plateau gradient zone. Based on the distribution law, the distribution range of potential landslide hazards has been further refined. During the construction of the Lexi Highway, four potential unstable landslide hazards were newly discovered, as shown in Figure 18, providing critical support for disaster prevention and mitigation efforts.
Figure 17. Interpretability values (q) of conditioning factors for the Lexi Highway (a) and Chengyu Highway (b).
For the Chengyu Highway region, rainfall (q = 0.36), intensity of human engineering activities (q = 0.34), and distance from the fault zone (q = 0.31) are the dominant factors controlling geohazard distribution. Secondary factors included engineering geology rock group (q = 0.29), vertical deformation gradient (q = 0.26), and slope angle (q = 0.25), while elevation, Bouguer gravity anomaly gradient, and horizontal deformation gradient showed weaker impacts. This is slightly different from the overall distribution law of geohazards on highways in the western Sichuan Plateau gradient zone.
This is mainly due to the unique geological location characteristics of the western Sichuan Plateau gradient zone. As a steep transition zone from the Chengdu Plain to the eastern edge of the Qinghai Tibet Plateau, this region has a significantly different dynamic background from other areas. This region exhibits intense neotectonic activity, high crustal vertical deformation rates, and prominent endogenic-exogenic coupling effects. In such high-energy environments, deep-seated factors (e.g., fault proximity, crustal deformation gradients) exhibit significantly stronger control over geohazards than in stable cratonic regions. Although geohazard distribution law differ along highways outside the study area, the “tectonic-geomorphic-climatic” synergistic control mechanism revealed in this study maintains universal theoretical relevance, providing a scientific foundation for geohazard prevention and mitigation strategies.
Internal dynamic factors such as the distance from the fault zone, Bouguer gravity anomaly gradient, vertical deformation gradient, and horizontal deformation gradient are indicative of regional tectonic deformation intensity. Strong tectonic deformation can lead to deformation and fractures in geological bodies, alter the regional tectonic stress field, and trigger earthquakes along active fault zones, thereby adversely affecting the stability of regional geological structures.
As the Tibetan Plateau continues to uplift, river valleys undergo significant downcutting and erosion, forming alpine canyons, gorges, and wide valleys. This process redistributes or modifies the shallow-surface stress conditions in the mountains, creating unloading rebound zones. Factors such as engineering geology rock groups, elevation, and slope are representative of the rock mass loosening circle. The existence of this loosening circle alters the physical and mechanical properties of rock and soil masses, weakening their structural integrity and modifying stress distribution while reducing their stability. These changes significantly influence slope evolution, promoting sliding and contributing to geohazard occurrence.
Human engineering activities, particularly slope excavation along highways, introduce additional disturbances. Such activities disrupt the original stress equilibrium of slope bodies, intensify the development of shallow-surface unloading zones, and create conditions conducive to geohazard occurrence (Qi et al., 2022).
Rainfall, an indicator of atmospheric feedback effects, further exacerbates geohazard risks. It progressively deteriorates the physical and mechanical properties of rock and soil masses, induces fluctuations in groundwater levels, and alters seepage paths. These processes severely decrease the stability of slope materials, making rainfall a key trigger for geohazards.
Overall, the most conditioning factors determining the distribution of highway geohazards in the western Sichuan Plateau gradient zone are the distance from the fault zone, vertical deformation gradient, and rainfall. For highways outside the study region, although geohazard distribution laws differ, the “tectonic-geomorphic-climatic” synergistic control mechanism revealed in this study retains universal theoretical relevance, providing a scientific basis for geohazard prevention and mitigation.
(1) The distribution of geohazard sites along highways in the western Sichuan Plateau gradient zone is dense, with an average density of 1.16 sites/km.
(2) Geohazard distributions show significant clustering, with higher densities observed in the central portion of the gradient zone and relatively lower densities at its periphery.
(3) The most significant factors influencing highway geohazard distribution in the study area are the distance from the fault zone (q = 0.39), vertical deformation gradient (q = 0.37), and rainfall (q = 0.31). Secondary factors include the intensity of human engineering activities, engineering geology rock groups, and slope angle, while the Bouguer gravity anomaly gradient, horizontal deformation gradient, and elevation have relatively minor effects.
(4) The q value of the interaction between any two conditioning factors on the distribution of geohazards exceeds that of a single factor. Notably, the interactions between rainfall and vertical deformation gradient and between engineering geology rock group and vertical deformation gradient have a greater impact on the distribution of geohazards than the interactions among other conditioning factors.
The raw data supporting the conclusions of this article will be made available by the authors, without undue reservation.
CL: Data curation, Investigation, Writing–original draft. TL: Methodology, Writing–original draft, Data curation. FL: Funding acquisition, Project administration, Writing–review and editing. JW: Data curation, Writing–original draft. YR: Writing–review and editing, Data curation. XK: Supervision, Writing–review and editing.
The author(s) declare that financial support was received for the research and/or publication of this article. This research is supported by the Sichuan Transportation technology project (Grant: No. 2021-ZL-15).
Authors FL and XK were employed by Sichuan Lexi Expressway Co., Ltd.
The remaining authors declare that the research was conducted in the absence of any commercial or financial relationships that could be construed as a potential conflict of interest.
The reviewer JL declared a shared affiliation with the author FL to the handling editor at time of review.
The author(s) declare that no Generative AI was used in the creation of this manuscript.
All claims expressed in this article are solely those of the authors and do not necessarily represent those of their affiliated organizations, or those of the publisher, the editors and the reviewers. Any product that may be evaluated in this article, or claim that may be made by its manufacturer, is not guaranteed or endorsed by the publisher.
Chen, B., Li, Z. H., Zhang, C. L., Ding, M. T., Zhu, W., Zhang, S. C., et al. (2022). Wide area detection and distribution characteristics of landslides along Sichuan expressways. Remote Sens. 14 (14), 3431. doi:10.3390/rs1.4143431
Chen, L. F., Guo, H. X., Gong, P. S., Yang, Y. Y., Zuo, Z. L., and Gu, M. Y. (2021). Landslide susceptibility assessment using weights-of-evidence model and cluster analysis along the highways in the Hubei section of the Three Gorges Reservoir Area. Comput. and Geosciences 156, 104899. doi:10.1016/j.cageo.2021.104899
Chen, Z., Song, D. Q., Du, Y. M., and Dong, L. H. (2023). Investigation on the spatial distribution of landslides in Sichuan Province, southwest China. Geomatics Nat. Hazards Risk 14 (1), 2232085. doi:10.1080/19475705.2.023.2232085
Cloetingh, S., and Willett, S. D. (2013). TOPO-EUROPE:Understanding of the coupling between the deep earth and continental topography. Tectonophysics 602, 1–14. doi:10.1016/j.tecto.2013.05.023
Convertino, M., Troccoli, A., and Catani, F. (2013). Detecting fingerprints of landslide drivers: a MaxEnt model. J. Geophys. Research-earth Surf. 118 (3), 1367–1386. doi:10.1002/jgrf.2.0099
Dandridge, C., Stanley, T. A., Kirschbaum, D. B., and Lakshmi, V. (2023). Spatial and temporal analysis of global landslide reporting using a decade of the global landslide catalog. Sustainability 15 (4), 3323. doi:10.3390/su15043323
Fan, X. M., Scaringi, G., Xu, Q., Zhan, W. W., Dai, L. X., Li, Y. S., et al. (2018). Coseismic landslides triggered by the 8th August 2017 Ms 7.0 Jiuzhaigou earthquake (Sichuan,China):factors controlling their spatial distribution and implications for the seismogenic blind fault identification. Landslides 15 (5), 967–983. doi:10.1007/s10346-018-0960-x
Fu, G. Y., Gao, S. H., Freymueller, J. T., Zhang, G. Q., Zhu, Y. Q., and Yang, G. L. (2014). Bouguer gravity anomaly and isostasy at western Sichuan Basin revealed by new gravity surveys. J. Geophys. Research-Solid Earth 119, 3925–3938. doi:10.1002/2014.JB011033
Gong, Y. F., Yao, A. J., Li, Y. L., Li, Y. Y., and Tian, T. (2022). Classification and distribution of large-scale high-position landslides in southeastern edge of the Qinghai-Tibet Plateau, China. Environ. Earth Sci. 81, 311. doi:10.1007/s12665-022-10433-6
Gu, Z. K., Yao, X., Li, G. G., and Zhu, X. C. (2023). Driving effects of dynamic geomorphologic environments on gravitational erosion hazards: a case of the Baihetan drainage area of the Jinsha River, China. Bull. Eng. Geol. Environ. 82, 49. doi:10.1007/s10064-022-03051-w
Huang, F. M., Shi, Y., Ouyang, W. P., Hong, A. Y., Zeng, Z. Q., and Xu, F. G. (2022). Landslide susceptibility prediction modeling based on weightof evidence and chi-square automatic interactive detection decision tree. J. Civ. Environ. Eng. 44 (5), 16–28. doi:10.11835/.j.issn.2096-6717.2021.254
Huang, F. M., Yin, K. L., Jiang, S. H., Huang, J. S., and Cao, Z. S. (2018). Landslide susceptibility assessment based on clustering analysis and support vector machine. Chin. J. Rock Mech. Eng. 37 (1), 156–167. doi:10.13722/j.cnk.i.jrme.2017.0824
Huang, R. Q., and Li, W. L. (2008). Research on development and distribution rules of geohazards induced by Wenchuan earthquake on 12th may,2008. Chin. J. Rock Mech. Eng. 27 (12), 2585–2592. doi:10.3321/j.issn:1000-6915.2008.12.028
Huang, S. W., and Chen, L. P. (2024). Landslide susceptibility mapping using an integration of different statistical models for the 2015 Nepal earthquake in Tibet. Geomatics Nat. Hazards and Risk 15 (1). doi:10.1080/19475705.2024.2396908
Li, T. B., Wang, J. F., He, C. Y., Meng, L. B., Li, C. F., Ma, J. J., et al. (2024). Distribution law and susceptibility of geohazards across a gradient belt of the Western Sichuan Plateau. J. Mt. Sci. 21 (6), 1849–1867. doi:10.1007/s11629-023-8290-4
Ling, S. X., Sun, C. W., Li, X. N., Ren, Y., Xu, J. X., and Huang, T. (2021). Characterizing the distribution pattern and geologic and geomorphic controls on earthquake-triggered landslide occurrence during the 2017 Ms7.0 Jiuzhaigou earthquake, Sichuan, China. Landslides 18 (4), 1275–1291. doi:10.1007/s10346-020-0.1549-6
Liu, X., Wang, Y., and Leung, A. K. (2023). Numerical investigation of rainfall intensity and duration control of rainfall-induced landslide at a specific slope using slope case histories and actual rainfall records. Bull. Eng. Geol. Environ. 82, 333. doi:10.1007/s10064-023-033.59-1
Liu, X. M., Su, P. C., Li, Y., Xu, R., Zhang, J., Yang, T. Q., et al. (2021). Spatial patterns and scaling distributions of earthquake-induced landslides-A case study of landslides in watersheds along dujiangyan-wenchuan highway. Front. Earth Sci. 9, 659152. doi:10.3389/feart.2021.659152
Loche, M., Alvioli, M., Marchesini, I., Bakka, H., and Lombardo, L. (2022). Landslide susceptibility maps of Italy: lesson learnt from dealing with multiple landslide types and the uneven spatial distribution of the national inventory. Earth-Science Rev. 232, 104125. doi:10.1016/j.earscirev.20.22.104125
Lu, C. F., and Cai, C. X. (2019). Challenges and countermeasures for construction safety during the Sichuan –Tibet railway project. Engineering 5 (5), 833–838. doi:10.1016/j.eng.2019.06.007
Luo, J., Niu, F. J., Liu, M. H., Lin, Z. J., and Yin, G. A. (2018). Field experimental study on long-term cooling and deformation characteristics of crushedrock revetment embankment at the Qinghai-Tibet Railway. Appl. Therm. Eng. 139 (12), 256–263. doi:10.1016/j.applthermaleng.2018.04.138
Ma, C., Cui, P. P., Zhong, G. R., Meng, M., Yang, C., and Ma, W. S. (2021). Impact of climate change and engineering activities on spatiotemporal changes of vegetation index along Qinghai-Tibet Railway. Geogr. Res. 40 (01), 35–51. doi:10.11821/dlyj020.200528
Ma, S. Y., Shao, X. Y., and Xu, C. (2024). Landslide inventory and distribution patterns in Lhasa area, Tibet Plateau. Nat. Hazards. doi:10.1007/s11069-024-07031-z
Malamud, B. D., Turcotte, D. L., Guzzetti, F., and Reichenbach, P. (2004). Landslide inventories and their statistical properties. Landform 29, 687–711. doi:10.1002/e.sp.1064
Mtibaa, S., and Tsunetaka, H. (2023). Revealing the relation between spatial patterns of rainfall return levels and landslide density. Earth Surf. Dyn. 11 (3), 461–474. doi:10.5194/esurf-11-461-2023
Peng, J. B., Cui, P., and Zhuang, J. Q. (2020). Challenges to engineering geology of Sichuan—Tibet railway. Chin. J. Rock Mech. Eng. 39 (12), 2377–2389. doi:10.13722/j.cn.ki.jrme.2020.0446
Peng, J. B., Ma, R. Y., Lu, Q. Z., Li, X. A., and Shao, T. Q. (2004). Geological hazards effects of uplift of Qinghai-Tibet. Adv. Earth Sci. 19 (30), 457–466. doi:10.3321/j.issn:1001-8166.2004.0.3.018
Peng, J. B., Zhang, Y. S., Huang, D., Wang, F. Y., and Wang, Z. P. (2023). Interaction disaster effects of the tectonic deformation sphere, rock mass loosening sphere, surface freeze-thaw sphere and engineering Disturbance Sphere on the Tibetan Plateau. Earth Sci. 48 (8), 3099–3114. doi:10.379.9/dqkx.2023.137
Qi, S. W., Li, Y. C., Song, S. H., Lan, H. X., Ma, F. S., Li, Z. Q., et al. (2022). Regionalization of engineering geological stability and distribution of engineering disturbance disasters in Tibetan Plateau. J. Eng. Geol. 30 (3), 599–608. doi:10.1354.4/j.cnki.jeg.2022-0172
Qi, T. J., Meng, X. M., Qing, F., Zhao, Y., Shi, W., Chen, G., et al. (2021). Distribution and characteristics of large landslides in a fault zone: a case study of the NE Qinghai-Tibet Plateau. Geomorphology 379, 107592. doi:10.101.6/j.geomorph.2021.107592
Qiu, H. J., Cui, Y. F., Hu, S., Yang, D. D., Pei, Y. Q., and Yang, W. L. (2019). Temporal and spatial distributions of landslides in the qinba mountains, shaanxi province, China. Geomatics Nat. Hazards and Risk 10 (1), 599–621. doi:10.1080/19475705.2.018.1536080
Quevedo, R. P., Maciel, D. A., Uehara, T. D. T., Vojtek, M., Rennó, C. D., Pradhan, B. C., et al. (2022). Consideration of spatial heterogeneity in landslide susceptibility mapping using geographical random forest model. Geocarto Int. 37 (25), 8190–8213. doi:10.1080/10106049.2021.1996637
Savvides, R., Mäkelä, J., and Puolamäki, K. (2023). Model selection with bootstrap validation. Stat. Analysis Data Min. 16 (2), 162–186. doi:10.1002/sam.11606
Shu, B., Chen, Y., Amani-Beni, M., and Zhang, R. Z. (2022). Spatial distribution and influencing factors of mountainous geological disasters in southwest China: a fine-scale multi-type assessment. Front. Environ. Sci. 10, 1049333. doi:10.3389/fenvs.2022.1049333
Song, X. H., and Tan, Y. (2021). Experimental investigation on the influences of rainfall patterns on instability of sandy slopes. Enviromental Earth Sci. 80, 803. doi:10.1007/s12665-021-10118-6
Sun, B. X., Yang, L. J., Liu, Q., and Xu, X. Z. (2010). Numerical modelling for crushed rock layer thickness of highway embankments in permafrost regions of the Qinghai-Tibet Plateau. Eng. Geol. 114 (3-4), 181–190. doi:10.1016/j.engge.o.2010.04.014
Wang, J. F., Zhang, T. L., and Fu, B. J. (2016). A measure of spatial stratified heterogeneity. Ecol. Indic. 67, 250–256. doi:10.1016/j.ecolind.2016.02.052
Wang, S. B., Zhuang, J. Q., Mu, J. Q., Zheng, J., Zhan, J. W., Wang, J., et al. (2022). Evaluation of landslide susceptibility of the Ya'an-Linzhi section of the Sichuan-Tibet Railway based on deep learning. Environ. Earth Sci. 81, 250. doi:10.1007/s12665-022-10375-z
Wang, Y. S., Zhao, B., and Li, J. (2018). Mechanism of the catastrophic june 2017 landslide at xinmo village, songping river, sichuan province, China. Landslides 15 (2), 333–345. doi:10.1007/s10346-017-0927-3
Weng, M. C., Lo, C. M., Wu, C. H., and Chuang, T. F. (2015). Gravitational deformation mechanisms of slate slopes revealed by model tests and discrete element analysis. Eng. Geol. 189, 116–132. doi:10.1016/j.enggeo.20.15.01.024
Xie, H. P., Zhang, H. R., Deng, J. H., Gao, M. Z., Li, Y. H., He, Z. L., et al. (2021). A preliminary study on the technical system of deep earth science and geo disaster prevention-control based on the “deep earth–surface” linkage strategy. Adv. Eng. Sci. 53 (04), 1–12. doi:10.15961/j.jsuese.202100493
Xie, J. R., Uchimura, T., Chen, P., Liu, J. P., Xie, C. R., and Shen, Q. (2019). A relationship between displacement and tilting angle of the slope surface in shallow landslides. Landslides 16 (6), 1243–1251. doi:10.1007/s10346-019-01135-5
Xu, C., Xu, X., Yao, X., and Dai, F. (2014). Three (nearly) complete inventories of landslides triggered by the May 12, 2008 Wenchuan Mw 7.9 earthquake of China and their spatial distribution statistical analysis. Landslides 11, 441–461. doi:10.1007/s10346-013-040.4-6
Xu, L. L., Chen, C. L. P., Qing, F., Meng, X. M., Zhao, Y., Qi, T. J., et al. (2022). Graph-represented broad learning system for landslide susceptibility mapping in alpine-canyon region. Remote Sens. 14 (12), 2773. doi:10.3390/rs14122773
Yang, H. Z., Dong, J. Y., and Guo, X. L. (2023). Geohazards and risk assessment along highway in Sichuan Province, China. J. Mt. Sci. 20 (6), 1695–1711. doi:10.1007/s11629-022-7500-9
Yang, L. W., Wang, W. P., Zhang, N., and Wei, Y. J. (2020). Characteristics and numerical runout modeling analysis of the xinmo landslide in sichuan, China. Earth Sci. Res. J. 24 (2), 169–181. doi:10.15446/esrj.v24n2.78990
Yang, Z. H., Zhang, Y. S., Guo, C. B., and Yao, X. (2018). Sensitivity analysis on causative factors of geohazards in eastern margin of Tibetan Plateau. J. Eng. Geol. 26 (3), 673–683. doi:10.13544/j.cnki.jeg.20.17-165
Yao, X., Li, L. J., Zhang, Y. S., Guo, C. B., and Zhou, N. J. (2015). Regional crustal stability assessment of the eastern margin of Tibetan Plateau. Geol. Bull. China 34 (1), 32–44. doi:10.3969/j.issn.1671-2552.2015.0.1.003
Yi, Y. N., Xu, X. W., Xu, G. Y., and Gao, H. R. (2023). Landslide detection using time-series InSAR method along the kangding-batang section of shanghai-nyalam road. Remote Sens. 15 (5), 1452. doi:10.3390/rs15051452
Yu, Y., Xiong, Z. Y., Xiong, Y. S., and Li, W. Z. (2019). Improved logistic regression algorithm based on kernel density estimation for multi-classification with non-equilibrium samples. CMC-Computers Materials& Contin. 61 (1), 103–118. doi:10.32604/cmc.2019.05154
Zhang, J., Lu, M., Zhang, L. L., and Xue, Y. D. (2021). Assessing indirect economic losses of landslides along highways. Nat. Hazards 106 (3), 2775–2796. doi:10.1007/s1106.9-021-04566-3
Zhang, P. Z., Wang, W. T., Gan, W. J., Zhang, Z. Q., Zhang, H. P., Zheng, D. W., et al. (2022a). Present-day deformation and geodynamic process of the Tibetan Plateau. Acta Geol. Sin. 96 (10), 3297–3313. doi:10.19762/j.cnki.dizhixuebao.2022295
Zhang, S. F., Wang, Y. F., Jia, B., and Zhao, S. M. (2017). Spatial-temporal changes and influencing factors of geologic disasters from 2005 to 2016 in China. J. Geo-information Sci. 19 (12), 1567–1574. doi:10.3724/SP.J.1047.2017.01567
Zhang, T. Y., Fu, Q., Li, C., Liu, F. F., Wang, H. Y., Han, L., et al. (2022b). Modeling landslide susceptibility using data mining techniques of kernel logistic regression, fuzzy unordered rule induction algorithm, SysFor and random forest. Nat. Hazards 114 (3), 3327–3358. doi:10.1007/s110.69-022-05520-7
Keywords: western sichuan plateau gradient zone, highway, geohazard, geodynamic factors, distribution law
Citation: Li C, Li T, Lan F, Wang J, Ren Y and Kou X (2025) Research on the distribution law of geohazards along the highways in the Western sichuan plateau gradient zone. Front. Earth Sci. 13:1536412. doi: 10.3389/feart.2025.1536412
Received: 28 November 2024; Accepted: 20 March 2025;
Published: 02 April 2025.
Edited by:
Xiaoping Zhou, Chongqing University, ChinaReviewed by:
Hao Cheng, Wuhan University, ChinaCopyright © 2025 Li, Li, Lan, Wang, Ren and Kou. This is an open-access article distributed under the terms of the Creative Commons Attribution License (CC BY). The use, distribution or reproduction in other forums is permitted, provided the original author(s) and the copyright owner(s) are credited and that the original publication in this journal is cited, in accordance with accepted academic practice. No use, distribution or reproduction is permitted which does not comply with these terms.
*Correspondence: Fuan Lan, MTU1MTM2ODYyNDlAMTYzLmNvbQ==
Disclaimer: All claims expressed in this article are solely those of the authors and do not necessarily represent those of their affiliated organizations, or those of the publisher, the editors and the reviewers. Any product that may be evaluated in this article or claim that may be made by its manufacturer is not guaranteed or endorsed by the publisher.
Research integrity at Frontiers
Learn more about the work of our research integrity team to safeguard the quality of each article we publish.