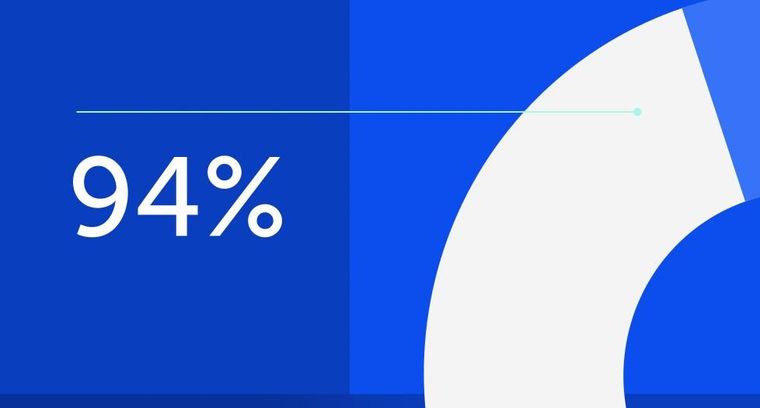
94% of researchers rate our articles as excellent or good
Learn more about the work of our research integrity team to safeguard the quality of each article we publish.
Find out more
METHODS article
Front. Earth Sci.
Sec. Solid Earth Geophysics
Volume 13 - 2025 | doi: 10.3389/feart.2025.1535990
This article is part of the Research TopicThe State-of-Art Techniques of Seismic Imaging for the Deep and Ultra-deep Hydrocarbon Reservoirs - Volume IIIView all 5 articles
The final, formatted version of the article will be published soon.
Select one of your emails
You have multiple emails registered with Frontiers:
Notify me on publication
Please enter your email address:
If you already have an account, please login
You don't have a Frontiers account ? You can register here
Seismic data acquisition inevitably faces disruptions from various environmental sources, such as factories, machinery, and highways. These disturbances introduce anomalous amplitudes in seismic data, significantly compromising the signal-to-noise ratio (SNR). At present, the primary approach for denoising such noise involves direct attenuation when amplitudes surpass a predefined threshold. However, determining this threshold relies on the window size of the selected data. Small windows are unable to suppress continuous anomalous noise traces, while using larger windows to calculate thresholds results in inaccurate outcomes. Furthermore, the window-based threshold computation can inadvertently damage non-noise strong amplitude signals, like near-offset traces or surface waves. In this study, we take advantage of the distinctive characteristics of seismic data acquired by nodal instruments. These instruments not only record seismic data but also preserve pure environmental signals which are recorded ahead of the shooting time. Combining the statistical distribution of the amplitudes of pure environmental signals and deep learning techniques, we identify data samples potentially contaminated by anomalous amplitude noise in the seismic data. Based on noise identification, we propose a novel pointwise adaptive threshold (PAT) method. This entails calculating an individual threshold value for each noise sample and subsequently applying noise attenuation. The proposed method offers several benefits, including more accurate threshold computation, preservation of effective signals with strong amplitudes, reducing the dependence on picking precise first-break, and simultaneously addressing multi-trace and single-trace anomalous amplitudes. Moreover, this approach has high robustness, as misidentification in local sample points does not influence the outcomes of noise attenuation.
Keywords: Anomalous Amplitude Noises, attenuation, Nodal Land Seismic Data, denoising, deep learning
Received: 28 Nov 2024; Accepted: 08 Apr 2025.
Copyright: © 2025 Mao, Yang, Pang, Zhou, Mao, Zhang, Wang and Pan. This is an open-access article distributed under the terms of the Creative Commons Attribution License (CC BY). The use, distribution or reproduction in other forums is permitted, provided the original author(s) or licensor are credited and that the original publication in this journal is cited, in accordance with accepted academic practice. No use, distribution or reproduction is permitted which does not comply with these terms.
* Correspondence: Wen Yang, BGP Inc., China National Petroleum Corporation, Zhuozhou, China
Disclaimer: All claims expressed in this article are solely those of the authors and do not necessarily represent those of their affiliated organizations, or those of the publisher, the editors and the reviewers. Any product that may be evaluated in this article or claim that may be made by its manufacturer is not guaranteed or endorsed by the publisher.
Supplementary Material
Research integrity at Frontiers
Learn more about the work of our research integrity team to safeguard the quality of each article we publish.