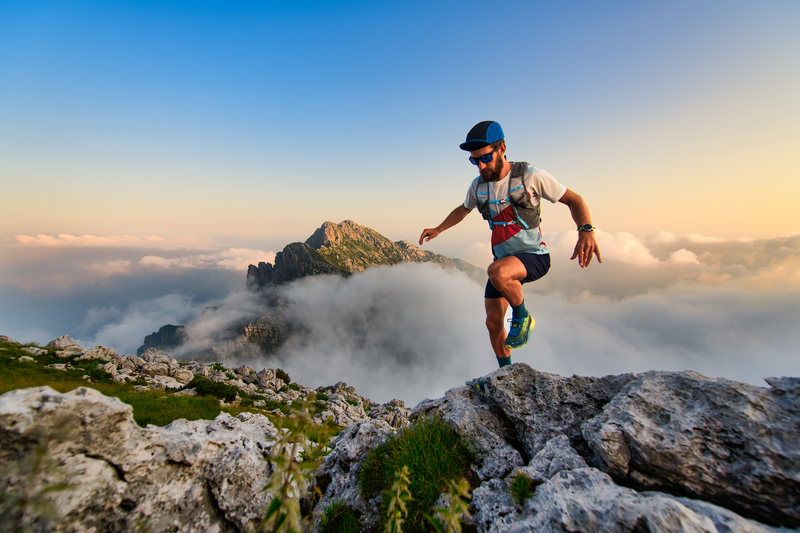
95% of researchers rate our articles as excellent or good
Learn more about the work of our research integrity team to safeguard the quality of each article we publish.
Find out more
ORIGINAL RESEARCH article
Front. Earth Sci.
Sec. Economic Geology
Volume 13 - 2025 | doi: 10.3389/feart.2025.1534598
This article is part of the Research Topic Differences in Shale Oil and Gas Reservoirs across Various Sedimentary Environments: Theories and Applications, Volume II View all 9 articles
The final, formatted version of the article will be published soon.
You have multiple emails registered with Frontiers:
Please enter your email address:
If you already have an account, please login
You don't have a Frontiers account ? You can register here
With the gradual reduction in conventional exploration and the gradual improvement of hydraulic fracturing technology, the exploration of tight oil and gas has gradually become general.Marine carbonate rocks are developed in the lower Paleozoic Ordovician in the eastern margin of Ordos Basin. According to the drilling results, the minerals in this area are mainly calcite and dolomite, and the secondary minerals are halite, anhydrite, quartz, feldspar and clay minerals.Core experiment reveals that the reservoir is tight, with porosity between 2 and 6% and permeability less than 2mD.Relationship between lithology and porosity established by conventional logging interpretation is not consistent with the results of gas logging, so it is impossible to accurately determine the reservoir location.The research in this paper is to interpret lithologic profile based on mineral X-ray diffraction data, to accurately evaluate pore type and permeability based on borehole electrical imaging and multipole array acoustic logging, and to interpret gas saturation by using the Archie formula with variable cementation index.This method improves the interpretation accuracy of geophysical logging methods for tight reservoirs in this block, and plays a key role in favorable evaluation of reservoir sweet spots.
Keywords: Carbonate rock, Logging evaluation, Imaging logging, Tight gas, Unconventional exploration
Received: 26 Nov 2024; Accepted: 18 Mar 2025.
Copyright: © 2025 Dan, Kui, Yu and Shanshan. This is an open-access article distributed under the terms of the Creative Commons Attribution License (CC BY). The use, distribution or reproduction in other forums is permitted, provided the original author(s) or licensor are credited and that the original publication in this journal is cited, in accordance with accepted academic practice. No use, distribution or reproduction is permitted which does not comply with these terms.
* Correspondence:
Li Dan, CNOOC Research Institute Ltd, Beijing, China
Disclaimer: All claims expressed in this article are solely those of the authors and do not necessarily represent those of their affiliated organizations, or those of the publisher, the editors and the reviewers. Any product that may be evaluated in this article or claim that may be made by its manufacturer is not guaranteed or endorsed by the publisher.
Research integrity at Frontiers
Learn more about the work of our research integrity team to safeguard the quality of each article we publish.