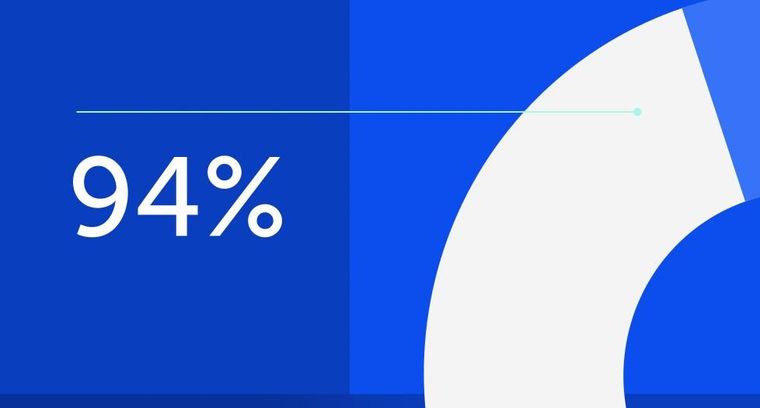
94% of researchers rate our articles as excellent or good
Learn more about the work of our research integrity team to safeguard the quality of each article we publish.
Find out more
ORIGINAL RESEARCH article
Front. Earth Sci.
Sec. Solid Earth Geophysics
Volume 13 - 2025 | doi: 10.3389/feart.2025.1526527
The final, formatted version of the article will be published soon.
Select one of your emails
You have multiple emails registered with Frontiers:
Notify me on publication
Please enter your email address:
If you already have an account, please login
You don't have a Frontiers account ? You can register here
The wide-field electromagnetic (WFEM) method, known for its strengths in deep detection, interference resistance, and adaptability to various surface conditions, has been widely used in geophysical exploration for resources such as shale gas and groundwater in complex geological environments. However, noise interference remains a significant challenge, especially in areas with intense human activity, such as mining regions and urban environments, where electromagnetic noise is complex, non-periodic, and pseudo-random. This interference severely degrades the signal-to-noise ratio (SNR), complicating the interpretation of subsurface structures.In shale gas exploration in the Sichuan Changning Block and groundwater exploration in the Shanxi Xinyuan Coal Mine area, diverse and challenging noise sources further complicate WFEM applications. Traditional denoising methods often struggle to effectively address such complex and non-periodic noise. To solve this problem, we propose an innovative denoising method for WFEM data that integrates Particle Swarm Optimization (PSO) and Grey Wolf Optimization (GWO) to optimize a Convolutional Neural Network (CNN). This method leverages CNN's ability to extract nonlinear features, enabling it to effectively identify and separate multiple noise types (e.g., Gaussian white noise, impulse noise, and attenuation noise), thereby enhancing denoising accuracy. The PSO-GWO hybrid algorithm optimizes CNN hyperparameters, improving the model's adaptability to different noise environments. A sample library was constructed, covering shale gas and groundwater exploration areas, with typical signal features extracted for noise identification and removal using the optimized CNN model. Simulation results demonstrate that this method significantly outperforms traditional techniques in terms of SNR enhancement and its ability to handle complex, non-periodic noise. The proposed denoising approach has been successfully applied in the Sichuan Changning Block and Shanxi Xinyuan Coal Mine area, showing significant improvements in WFEM data quality and the reliability of geological interpretations. This method offers a robust solution to noise challenges in geophysical exploration, enhancing interpretative accuracy across diverse geological blocks.
Keywords: Wide-field electromagnetic, Particle Swarm Optimization, Grey Wolf optimization, Convolutional Neural Networks, Electromagnetic data denoising, geological exploration
Received: 12 Nov 2024; Accepted: 07 Apr 2025.
Copyright: © 2025 yuan, Li, cheng and xian. This is an open-access article distributed under the terms of the Creative Commons Attribution License (CC BY). The use, distribution or reproduction in other forums is permitted, provided the original author(s) or licensor are credited and that the original publication in this journal is cited, in accordance with accepted academic practice. No use, distribution or reproduction is permitted which does not comply with these terms.
* Correspondence: Diquan Li, Central South University, Changsha, China
Disclaimer: All claims expressed in this article are solely those of the authors and do not necessarily represent those of their affiliated organizations, or those of the publisher, the editors and the reviewers. Any product that may be evaluated in this article or claim that may be made by its manufacturer is not guaranteed or endorsed by the publisher.
Supplementary Material
Research integrity at Frontiers
Learn more about the work of our research integrity team to safeguard the quality of each article we publish.