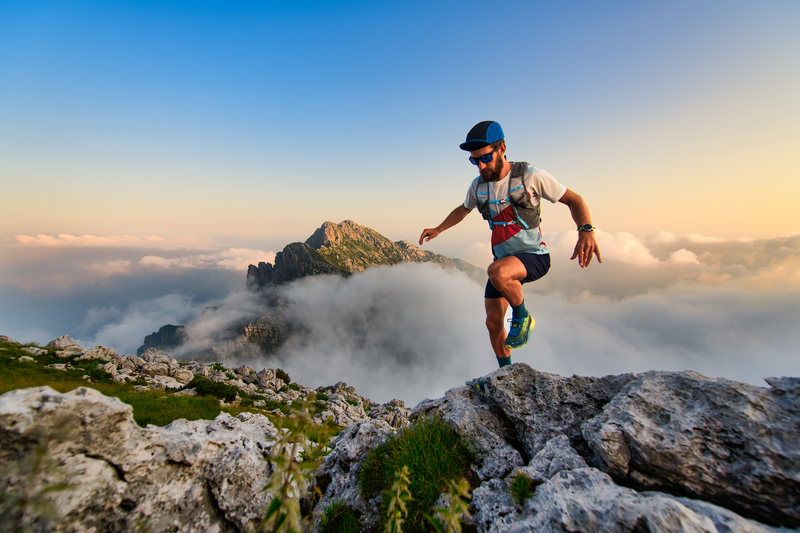
94% of researchers rate our articles as excellent or good
Learn more about the work of our research integrity team to safeguard the quality of each article we publish.
Find out more
ORIGINAL RESEARCH article
Front. Earth Sci.
Sec. Geohazards and Georisks
Volume 13 - 2025 | doi: 10.3389/feart.2025.1526129
This article is part of the Research Topic Failure Analysis and Risk Assessment of Natural Disasters Through Machine Learning and Numerical Simulation: Volume IV View all 20 articles
The final, formatted version of the article will be published soon.
You have multiple emails registered with Frontiers:
Please enter your email address:
If you already have an account, please login
You don't have a Frontiers account ? You can register here
Existing image processing and target recognition algorithms have limitations in complex underwater environments and dynamic changes, making it difficult to ensure real-time and precision.Multiple noise sources interfere with sonar signals, which affects both data precision and clarity. This article studies the dynamic display algorithm of sonar data based on grayscale distribution model and computational intelligence. It proposes to construct a grayscale distribution model for sonar images, analyze the grayscale histogram, determine the threshold selection of the maximum entropy threshold segmentation method, and finally complete the target segmentation. The segmented images can be used to train the convolutional neural network object recognition model constructed in this article. To verify the effectiveness of the proposed method, a test set was used to evaluate the trained target recognition model. The precision of the model recognition was 87.95%, the recall was 87.97%, and the F1 value was 0.8794, which is significantly higher than the traditional model(Such as Otsu and SVM is below 80%). The recognition speed reached 37ms, which is a certain improvement compared to the traditional model.
Keywords: Sonar data, Grayscale Distribution Model, Convolutional Neural Network, Maximum entropy threshold, target recognition
Received: 11 Nov 2024; Accepted: 18 Feb 2025.
Copyright: © 2025 Hongquan, Li and Haidong. This is an open-access article distributed under the terms of the Creative Commons Attribution License (CC BY). The use, distribution or reproduction in other forums is permitted, provided the original author(s) or licensor are credited and that the original publication in this journal is cited, in accordance with accepted academic practice. No use, distribution or reproduction is permitted which does not comply with these terms.
* Correspondence:
Jiang Haidong, School of Geosciences and Info Physics, Central South University, Changsha, 410083, Hunan Province, China
Disclaimer: All claims expressed in this article are solely those of the authors and do not necessarily represent those of their affiliated organizations, or those of the publisher, the editors and the reviewers. Any product that may be evaluated in this article or claim that may be made by its manufacturer is not guaranteed or endorsed by the publisher.
Research integrity at Frontiers
Learn more about the work of our research integrity team to safeguard the quality of each article we publish.