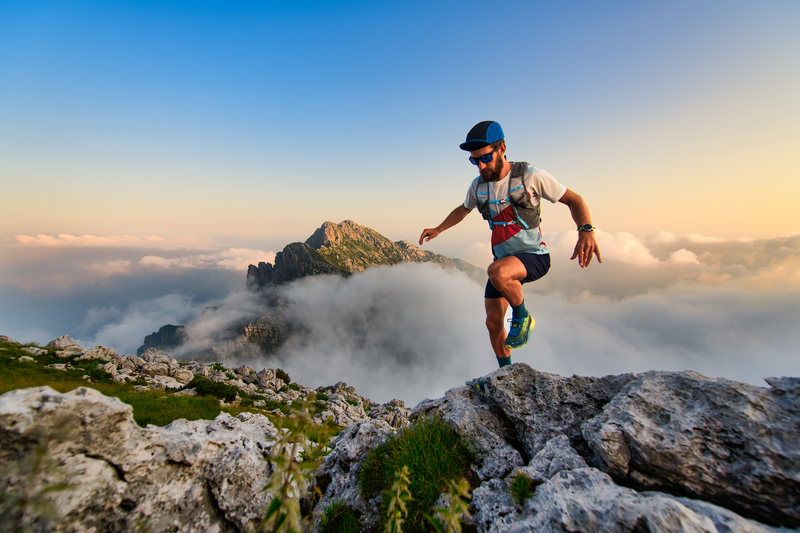
94% of researchers rate our articles as excellent or good
Learn more about the work of our research integrity team to safeguard the quality of each article we publish.
Find out more
ORIGINAL RESEARCH article
Front. Earth Sci.
Sec. Solid Earth Geophysics
Volume 13 - 2025 | doi: 10.3389/feart.2025.1526073
This article is part of the Research Topic The State-of-Art Techniques of Seismic Imaging for the Deep and Ultra-deep Hydrocarbon Reservoirs - Volume III View all articles
The final, formatted version of the article will be published soon.
You have multiple emails registered with Frontiers:
Please enter your email address:
If you already have an account, please login
You don't have a Frontiers account ? You can register here
Full-waveform inversion (FWI) can provide accurate velocity field for fine imaging in depth domain of seismic data. Its mathematics foundation determines that FWI is a strong nonlinearity with the solution being non-unique and the function being difficult to converge. In this paper, adjoint gradient and Hessian operators are introduced into the calculation of FWI objective function to improve the inversion accuracy. Firstly, the adjoint gradient method is used to iteratively optimize the gradient of the model with respect to the residuals of the observed data when solving the objective function. Secondly, in view of the energy inconsistency gradient amplitudes across space, the diagonal elements of Hessian operator are used to scale the gradient, which ensures that the gradient amplitude is inversely proportional to the sensitivity of the synthesized data, thereby the imaging accuracy in deep and weak reflected areas. Finally, the sub-sag model and the overthrust model are used to perform the proposed method in this paper. The inversion results indicated that the FWI method with Hessian operator pre-processing significantly reduced the impact of abnormal amplitude of wave field gradient on structures near the shot detection point and deep structure, and enhanced the accuracy and resolution of FWI modeling. It provides a more accurate velocity model for fine imaging of deep complex structures.
Keywords: Hessian operator, pre-processing, Adjoint gradient, Wave field gradient, FWI
Received: 11 Nov 2024; Accepted: 20 Feb 2025.
Copyright: © 2025 Yang, Zhang, yao, Wang and Zhu. This is an open-access article distributed under the terms of the Creative Commons Attribution License (CC BY). The use, distribution or reproduction in other forums is permitted, provided the original author(s) or licensor are credited and that the original publication in this journal is cited, in accordance with accepted academic practice. No use, distribution or reproduction is permitted which does not comply with these terms.
* Correspondence:
Fei-long Yang, School of Earth Sciences and Engineering, Xi’an Shiyou University, Xi'an, China
Disclaimer: All claims expressed in this article are solely those of the authors and do not necessarily represent those of their affiliated organizations, or those of the publisher, the editors and the reviewers. Any product that may be evaluated in this article or claim that may be made by its manufacturer is not guaranteed or endorsed by the publisher.
Research integrity at Frontiers
Learn more about the work of our research integrity team to safeguard the quality of each article we publish.