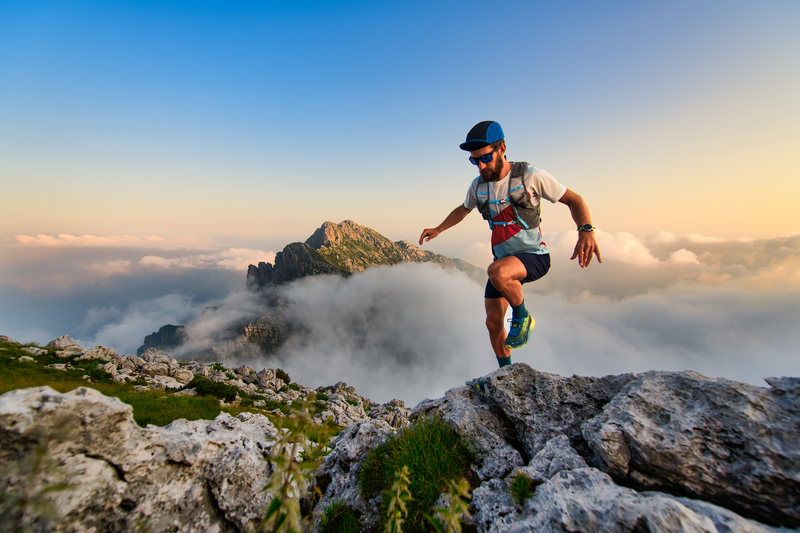
95% of researchers rate our articles as excellent or good
Learn more about the work of our research integrity team to safeguard the quality of each article we publish.
Find out more
TECHNOLOGY AND CODE article
Front. Earth Sci.
Sec. Solid Earth Geophysics
Volume 13 - 2025 | doi: 10.3389/feart.2025.1525363
This article is part of the Research Topic Advanced Materials and Technologies for Sustainable Development of Underground Resources View all 43 articles
The final, formatted version of the article will be published soon.
You have multiple emails registered with Frontiers:
Please enter your email address:
If you already have an account, please login
You don't have a Frontiers account ? You can register here
Based on the measured subsidence data of the 112201 working face in Xiaobaodang Coal Mine of Shaanxi Province, the traditional probability integral method and probability density function method are used to study the curve fitting of the main inclined section under insufficient mining conditions. The analysis results show that the fitting effect of the probability density function method is better than that of the probability integral method. In view of the poor effect of edge fitting, the idea of sub-region fitting is proposed. Two sets of predicted parameters are used to fit the edge and central regions. The fitting results are close to the surface deformation, and the fitting effect is better. The method can improve the accuracy of surface deformation prediction. The research results can provide a reference for the prediction of surface subsidence in Xiaobaodang Coal Mine and its surrounding mining areas.
Keywords: Mining subsidence, probability integral method, Probability density function method, Insufficient mining, Fitting analysis
Received: 09 Nov 2024; Accepted: 01 Apr 2025.
Copyright: © 2025 Du, Guo, Lai, Zhang, Ma, Dun, Jia, Feng and Li. This is an open-access article distributed under the terms of the Creative Commons Attribution License (CC BY). The use, distribution or reproduction in other forums is permitted, provided the original author(s) or licensor are credited and that the original publication in this journal is cited, in accordance with accepted academic practice. No use, distribution or reproduction is permitted which does not comply with these terms.
* Correspondence:
Yafei Ma, Hebei GEO University, Shijiazhuang, China
Disclaimer: All claims expressed in this article are solely those of the authors and do not necessarily represent those of their affiliated organizations, or those of the publisher, the editors and the reviewers. Any product that may be evaluated in this article or claim that may be made by its manufacturer is not guaranteed or endorsed by the publisher.
Research integrity at Frontiers
Learn more about the work of our research integrity team to safeguard the quality of each article we publish.