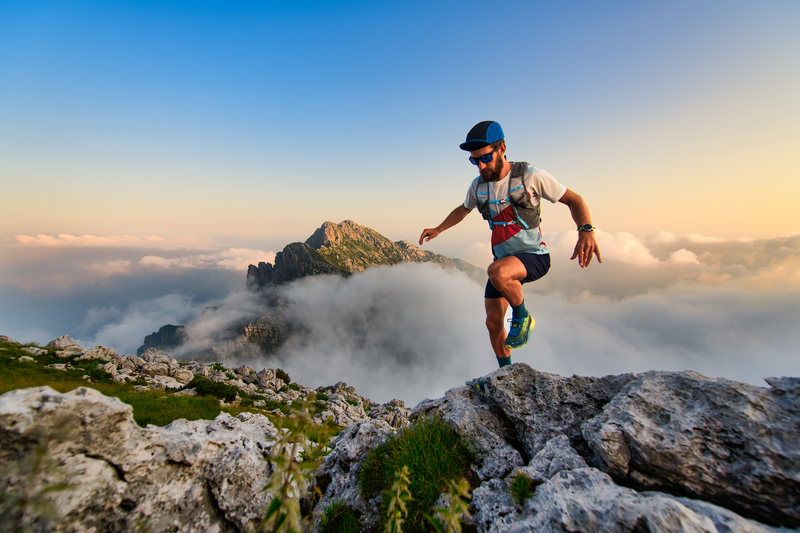
94% of researchers rate our articles as excellent or good
Learn more about the work of our research integrity team to safeguard the quality of each article we publish.
Find out more
METHODS article
Front. Earth Sci.
Sec. Solid Earth Geophysics
Volume 13 - 2025 | doi: 10.3389/feart.2025.1524300
The final, formatted version of the article will be published soon.
You have multiple emails registered with Frontiers:
Please enter your email address:
If you already have an account, please login
You don't have a Frontiers account ? You can register here
Lithology identification is crucial for understanding the characteristics of reservoirs, optimizing development plans, and enhancing development efficiency. For shale oil formations, the mineral composition is complex and the lithological combinations are diverse, which imposes limitations on conventional well logging interpretation for lithology identification.LithoScanner Logging is one of the methods to quantitatively calculate the mineral content, but there is still a discrepancy between the quantitatively calculated mineral content and the mineral content of core experiments in the actual exploration and development process. There are four main problems in LithoScanner Logging data processing: 1. Core data used to calibrate the dry weight of LithoScanner Logging elements are difficult to locate; 2. Changes in sedimentary environments and downhole geological conditions in different regions have a greater impact on the built-in sensitivity parameters of the instrument; 3. The yields of some elements with relatively low dry weight percentages are converted to dry weight curves, and the data are distorted not reflecting the real situation of the stratum; 4. The optimisation algorithm used has poor applicability, lacks better constraints, converges quickly and tends to fall into local optimal solutions. In response to the four questions above. Firstly, density logging and nuclear magnetic logging are used to calculate the rock skeleton density, which is used as the basis for depth normalisation of the core data; secondly, based on the oxide closure model, the offset and scaling factor are added to the calculation of the elemental dry weights, so as to obtain the optimal solution of the logged elemental dry weights; lastly, the elemental dry weights are combined with the differential evolution method, and the density profile and the sum of the mineral dry weights are used as the constraints, so as to carry out the inversion of the mineral dry weight is inverted.The results of mineral inversion have been well applied in different types of shale oil such as Daqing Gulong, Sichuan Liang Gaoshan, Xinjiang Mabei, etc. The average absolute error of the calculated dry weights of all kinds of minerals and the dry weights of core xrd experiments can be less than 5%.
Keywords: Elemental Capture Spectroscopy, LithoScanner logging, Lithology interpretation, mineral dry weight, Shale oil reservoir
Received: 07 Nov 2024; Accepted: 20 Feb 2025.
Copyright: © 2025 Xuan, Zhenlin, Jing, Yang, Xiaoxuan, Peng and LEI. This is an open-access article distributed under the terms of the Creative Commons Attribution License (CC BY). The use, distribution or reproduction in other forums is permitted, provided the original author(s) or licensor are credited and that the original publication in this journal is cited, in accordance with accepted academic practice. No use, distribution or reproduction is permitted which does not comply with these terms.
* Correspondence:
Hu Xuan, PetroChina Xinjiang Oilfield Company, Karamay, China
ZHAO LEI, PetroChina Xinjiang Oilfield Company, Karamay, China
Disclaimer: All claims expressed in this article are solely those of the authors and do not necessarily represent those of their affiliated organizations, or those of the publisher, the editors and the reviewers. Any product that may be evaluated in this article or claim that may be made by its manufacturer is not guaranteed or endorsed by the publisher.
Research integrity at Frontiers
Learn more about the work of our research integrity team to safeguard the quality of each article we publish.