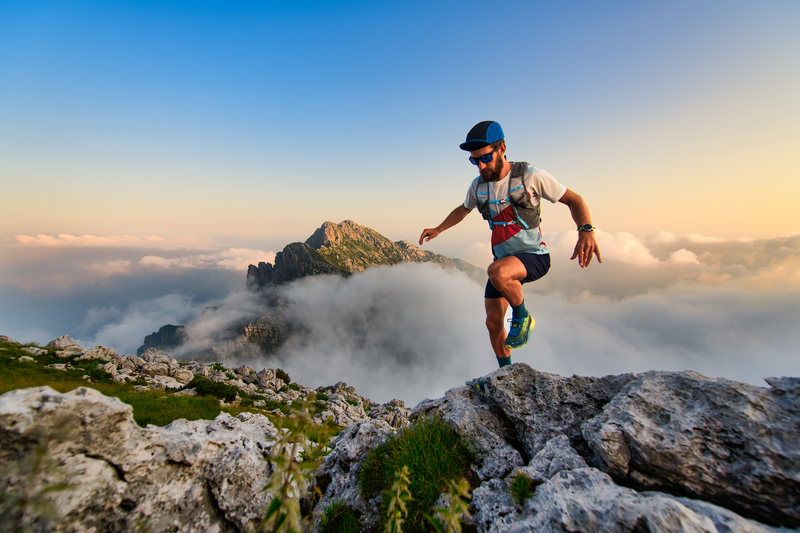
94% of researchers rate our articles as excellent or good
Learn more about the work of our research integrity team to safeguard the quality of each article we publish.
Find out more
ORIGINAL RESEARCH article
Front. Earth Sci.
Sec. Solid Earth Geophysics
Volume 13 - 2025 | doi: 10.3389/feart.2025.1512811
This article is part of the Research Topic Experimental and Numerical Simulations of Rock Physics View all 16 articles
The final, formatted version of the article will be published soon.
You have multiple emails registered with Frontiers:
Please enter your email address:
If you already have an account, please login
You don't have a Frontiers account ? You can register here
The continental deep strata in the Panyu 4 Sag of the Pearl River Mouth Basin in the South China Sea are characterized by complex lithology and tight sandstone reservoirs with low porosity and low permeability. Predicting porosity and lithology in this area has long been a challenge in seismic reservoir prediction. Traditional methods, which rely on linear mapping based on well data or probabilistic mapping through multi-attribute fusion, struggle to capture the complex nonlinear relationships between reservoir parameters and seismic attributes. To address this issue, this paper proposes a method using a convolutional neural network (CNN) for predicting porosity and facies distribution. Based on rock physics analysis and pre-stack elastic impedance inversion data, this approach first takes the effective porosity and shale content(VCL) from well-log interpretation as training targets. It then constructs training samples by simulating different lithologies and extracting the corresponding elastic parameters from well-log data. Through optimal evaluation, the model parameters of the deep learning network are determined, and a nonlinear mapping relationship between elastic parameters and reservoir parameters, such as porosity, is established. Finally, the trained deep learning model is applied to the elastic parameter bodies to obtain predictions of effective porosity and VCL, thereby achieving a quantitative characterization of high-quality deep sandstone reservoirs. The application of this method in the deltaic sediments of the Panyu 4 Sag in the Pearl River Mouth Basin shows that the deep learning-based predictions of facies distribution and porosity are consistent with well data and geological understanding. The fractured well, designed on the basis of the prediction results, achieved significant productivity enhancement following drilling, thereby demonstrating the efficacy of this method as a reservoir parameter prediction tool.
Keywords: Deep learn, Reservoir evaluation, Rock physics, Porosity prediction, shale content prediction
Received: 17 Oct 2024; Accepted: 07 Mar 2025.
Copyright: © 2025 Yingwei, Yanhui, Zhenshen, Xiaozhao and Guofu. This is an open-access article distributed under the terms of the Creative Commons Attribution License (CC BY). The use, distribution or reproduction in other forums is permitted, provided the original author(s) or licensor are credited and that the original publication in this journal is cited, in accordance with accepted academic practice. No use, distribution or reproduction is permitted which does not comply with these terms.
* Correspondence:
Li Yingwei, Shenzhen Branch of CNOOC Ltd., Shenzhen, China
Disclaimer: All claims expressed in this article are solely those of the authors and do not necessarily represent those of their affiliated organizations, or those of the publisher, the editors and the reviewers. Any product that may be evaluated in this article or claim that may be made by its manufacturer is not guaranteed or endorsed by the publisher.
Research integrity at Frontiers
Learn more about the work of our research integrity team to safeguard the quality of each article we publish.