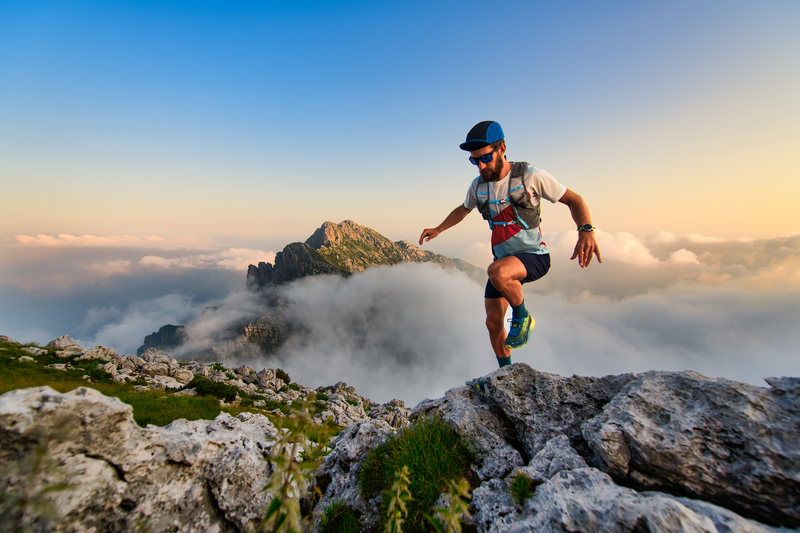
94% of researchers rate our articles as excellent or good
Learn more about the work of our research integrity team to safeguard the quality of each article we publish.
Find out more
ORIGINAL RESEARCH article
Front. Earth Sci.
Sec. Economic Geology
Volume 13 - 2025 | doi: 10.3389/feart.2025.1491334
This article is part of the Research Topic Differences in Shale Oil and Gas Reservoirs across Various Sedimentary Environments: Theories and Applications, Volume II View all 4 articles
The final, formatted version of the article will be published soon.
You have multiple emails registered with Frontiers:
Please enter your email address:
If you already have an account, please login
You don't have a Frontiers account ? You can register here
For lithologic oil reservoirs, lithology identification plays a significant guiding role in exploration targeting, reservoir evaluation, well network adjustment and optimization, and the establishment of reservoir models. Lithology is usually predicted from well log data based on limited core observations. In recent years, machine learning algorithms have been applied to lithology identification to enhance prediction accuracy. In this paper, five algorithms, including Bayes discriminant analysis, Random Forest (RF), Support Vector Machine (SVM), Back Propagation Neural Network (BPNN), and Convolutional Neural Network (CNN) are evaluated for lithology identification using data from the Niuxintuo reservoir. This reservoir is characterized by complex structural and sedimentary features, strong heterogeneity, and intricate lithological properties, all of which present considerable challenges for well logging identification. First, we conducted a detailed observation of the core lithology. Based on the requirements for reservoir evaluation and the principles of logging identification, we reclassify the lithology of the study area into two categories: clastic rocks and dolomite. The clastic rocks are further subdivided into five rock types: fine sandstone, medium-coarse sandstone, conglomerate, mudstone, and transitional rock. The well log series were selected through sensitivity analysis. Then, Bayes discriminant analysis and four machine learning methods were trained to identify the lithology of the study area. The results indicate that except for Bayes discriminant analysis, all the constructed machine learning classifiers demonstrate high prediction accuracy, with the accuracy rate exceeding 85%. Among them, SVM classifier shows the best performance achieving a prediction accuracy as high as 93%. Additionally, the well-trained SVM model was successfully used to predict the lithology profile of blind wells. Our findings provide valuable guidance for predicting the remaining oil distribution and further exploration potential in the Niuxintuo oilfield. Furthermore, this study gains insight into the process and methodology of rapidly predicting lithology of hydrocarbon reservoirs using easily accessible well logging data.
Keywords: Lithology classification, machine learning, Support vector machine, random forest, Convolutional Neural Networks, back propagation neural network, bayes distinguish analysis
Received: 04 Sep 2024; Accepted: 12 Feb 2025.
Copyright: © 2025 Fan, Hu, Jiang, Li, Cai, Jiang, Li and Tian. This is an open-access article distributed under the terms of the Creative Commons Attribution License (CC BY). The use, distribution or reproduction in other forums is permitted, provided the original author(s) or licensor are credited and that the original publication in this journal is cited, in accordance with accepted academic practice. No use, distribution or reproduction is permitted which does not comply with these terms.
* Correspondence:
Yang Li, Research Institute of Oil Exloration and Development, Petrochina Liaohe Oilfield Company, Panjin, China, Panjin, China
Disclaimer: All claims expressed in this article are solely those of the authors and do not necessarily represent those of their affiliated organizations, or those of the publisher, the editors and the reviewers. Any product that may be evaluated in this article or claim that may be made by its manufacturer is not guaranteed or endorsed by the publisher.
Research integrity at Frontiers
Learn more about the work of our research integrity team to safeguard the quality of each article we publish.