- 1School of Emergency Management Science and Engineering, University of Chinese Academy of Sciences, Beijing, China
- 2National Institute of Natural Hazards, Ministry of Emergency Management of China, Beijing, China
- 3Key Laboratory of Compound and Chained Natural Hazards Dynamics, Ministry of Emergency Management of China, Beijing, China
- 4Research Institute of China Southern Power Grid Co., Ltd., Guangzhou, Guangdong, China
- 5Jiangsu World Group, Danyang, Jiangsu, China
- 6Electric Power Research Institute, Yunnan Power Grid Co., Ltd., Kunming, Yunnan, China
- 7Zhejiang Metallurgical Survey and Design Co., Ltd., Hangzhou, Zhejiang, China
- 8Beijing Engineering Corporation Limited, Beijing, China
- 9Institute of Geology and Geophysics, Chinese Academy of Sciences, Beijing, China
- 10Geological Research Institute, Shougang Geological Exploration Institute, Beijing, China
Introduction: Landslides occur frequently in Zhenxiong County, posing significant threats to residents’ lives and property. A comprehensive understanding of the development patterns of landslide disasters in this region is crucial for disaster prevention, land-use planning, and risk assessment.
Methods: This study utilized high-resolution satellite imagery from the Google Earth Pro platform and employed a human-machine interactive visual interpretation approach to investigate landslide occurrences. A comprehensive landslide inventory comprising 3,979 landslide outlines was established through extensive literature review and data cleaning techniques. The spatial distribution characteristics and statistical patterns of landslides were analyzed.
Results: The total landslide-affected area is 319.20 km2, with the largest landslide covering 4.55 km2 and the smallest measuring 1,779 m2. The average landslide area is 80,215 m2, with the majority (73.54%) classified as medium-sized landslides. The landslide area percentage (LAP) is 8.64%, and the landslide number density (LND) is 1.077 landslides per km2, with the highest recorded landslide density being 3.380 landslides per km2. Landslides are predominantly concentrated in four key areas: the confluence of the Baishui River and Yanxi River, Dashuigou Reservoir, both sides of the valley from Heitang Village to Hongyan Village, and Xiaogou Village. These areas are characterized by well-developed water systems, middle and low mountains, and heavily dissected landscapes.
Discussion: The landslide database established in this study provides essential scientific data for analyzing the spatial distribution of landslide disasters in Zhenxiong County. It offers valuable insights for local governments and relevant authorities in disaster prevention, land-use planning, and risk assessment. The findings highlight the significant impact of complex terrain and developed water systems in middle and low mountain regions on landslide disasters. Future studies should further integrate geological and meteorological factors for deeper analysis.
1 Introduction
Landslides, as a common geological hazard (Huang et al., 2023b; Feng et al., 2024b), are widespread globally and occur with particular frequency in mountainous and hilly regions. These events pose significant threats to human life and property while also adversely impacting transportation, infrastructure, agriculture, and ecological systems. According to the United Global Landslide Database (UGLD), from 1903 to 2020, 37,946 severe landslide events were recorded across 161 countries, resulting in 185,753 fatalities (Gómez et al., 2023). In China alone, landslides claimed 28,139 lives between 1950 and 2016. Notably, in recent decades, the frequency and intensity of landslides have been increasing due to the exacerbation of climate change and intensified human activities (Frodella et al., 2018; Coviello et al., 2024). This rising trend presents significant challenges for disaster forecasting, mitigation, and management (Hwang and Lall, 2024).
Fortunately, the critical issue of landslides has garnered extensive attention over the past decade, leading to a steady growth in landslide research (Xu and Li, 2021; Huang et al., 2022; Chicas et al., 2024; Hosseini et al., 2024; Huu et al., 2024; Jallayu et al., 2024). Landslide inventories have emerged as invaluable resources for advancing our understanding of these hazards. These inventories are crucial for studying landslide processes, types, and triggers, while also providing insights into spatial distribution patterns and risk assessment (McGovern et al., 2024). Many countries have developed detailed landslide inventories (Conforti et al., 2014; Posner and Georgakakos, 2015; Sepúlveda and Petley, 2015; Rosser et al., 2017; Barella et al., 2019; Sultana, 2020). For example, Aristizábal and Sánchez compiled a comprehensive landslide inventory for Colombia, documenting 30,730 landslides between 1900 and 2018 and analyzing their spatiotemporal patterns and socioeconomic impacts (Aristizábal and Sánchez, 2020). Similarly, Bueechi et al. created an inventory of shallow landslides in Peru’s Cordillera Blanca, identifying 254 landslides from 2013 to 2017 using Google Earth imagery and developing a regional-scale susceptibility model (Bueechi et al., 2019). In Nicaragua, the Nicaraguan Institute for Earth Sciences (INETER) documented approximately 17,000 landslides from 1826 to 2003 in mountainous and volcanic terrains. This database has been instrumental for hazard assessment, emergency management, land-use planning, early warning systems, and policy implementation (Devoli et al., 2007). Italy’s national IFFI project, initiated in 1999, has mapped over 620,808 landslides, providing critical data for managing this pervasive hazard (Trigila et al., 2010).
China, with its diverse landforms—including mountains, hills, basins, plains, and plateaus—offers a geological environment highly conducive to landslides. Consequently, substantial research has been dedicated to developing landslide inventories (Li et al., 2021; Cui et al., 2023; Huang et al., 2023a; Li et al., 2024d; Sun et al., 2024b; Wang W. et al., 2024; Zhang et al., 2024; Zhao et al., 2024). For instance, Xu et al. leveraged high-resolution satellite imagery to create a detailed inventory of landslide relics on the Loess Plateau, identifying approximately 80,000 landslides (Xu et al., 2020). Wang et al. mapped 605 landslides covering a total area of 24.53 km2 in Jiyuan City, Henan Province, using Google Earth imagery (Wang et al., 2022). In Shaanxi Province, Chen et al. compiled a comprehensive database of landslide relics in Xianyang, analyzing their spatial distribution (Liu et al., 2023). In the Qinling region, Feng et al. developed an extensive inventory of landslide relics, providing key data for this mountainous area (Feng et al., 2024a; Feng et al., 2024b). Furthermore, Zhao et al. documented 1,073 landslides along the Sichuan-Tibet Engineering Corridor, validating their findings through a two-month field survey (Zhao et al., 2023). Similarly, Shao et al. constructed a database of paleo-landslides for the Wudongde Hydropower Station area, applying the data for hazard assessments (Shao et al., 2024b). These efforts underscore the critical role that landslide inventories play in mitigating risks and enhancing our understanding of this complex geological phenomenon.
Although China has developed numerous landslide inventories, county-level data often lack the necessary detail and comprehensiveness. This shortfall impedes a thorough understanding of regional landslide dynamics, diminishes the accuracy of risk assessments, and undermines the effectiveness of disaster prevention, mitigation, and response efforts. These challenges are particularly pronounced in southwestern China, a region highly prone to geological hazards (Shen et al., 2022; Shu et al., 2022). For instance, on 22 January 2024, a catastrophic landslide in Zhenxiong County, Yunnan Province, resulted in significant casualties and severe economic losses. Addressing this gap, our study employed a human-computer interactive visual interpretation approach to construct a detailed inventory of landslide relics within Zhenxiong County. Furthermore, we conducted a preliminary analysis of their spatial distribution patterns. The findings of this research provide a solid scientific foundation for future investigations and offer valuable data to support disaster prevention, mitigation, and response strategies in the region.
2 Study area
Zhenxiong County is situated in the northeastern part of Yunnan Province, at the junction of Yunnan, Guizhou, and Sichuan Provinces. It borders Xuyong County, Sichuan, along the Chishui River to the east; Bijie and Hezhang in Guizhou to the south; Yiliang to the west; and Weixin to the north. Geographically, the county lies between 104°10′ to 104°45′E and 27°13′ to 27°45′N, characterized by a rugged terrain of intersecting mountain ranges and valleys. The area features significant topographical relief and deep dissection, forming multi-level stepped landforms and deeply incised valleys (Figure 1). Elevation generally increases from northeast to southwest, with typical altitudes ranging between 1,000 and 2,000 m, creating a karst-erosion mid-mountain landscape (Yin et al., 2013; Yin et al., 2015). Geologically, Zhenxiong County features a complex structure as part of the Yunnan-Guizhou Plateau, shaped primarily by the convergence of the Yangtze and Kang-Dian tectonic blocks. Long-term tectonic activity has resulted in multiple stratigraphic overlays and intricate fault systems. The county is dominated by Huaxia-type structural features, characterized by a series of northeast-southwest trending folds of varying scales, accompanied by compressional-shear faults that run nearly parallel to these folds. Additionally, east-west and north-south trending structures, along with smaller torsional features, are present. Key tectonic elements include the Zhenxiong-Tangfang fault, Yuhe-Tanglangba wrench fault, Shanlin fault, and Guanmenshan fault, while seismic activity remains generally low. The region exhibits relatively complete stratigraphic sequences, with the oldest formations dating back to the Lower Cambrian. The most widespread lithologies include terrestrial-dominated, coal-bearing sandstones and shales of the Upper Permian, with interspersed marine layers, as well as Lower Triassic shallow marine sandstones and shales, limited carbonate rocks, and Quaternary deposits (Figure 2). Stratigraphically, the Upper Permian Longtan Formation, comprising shales, siltstones, fine sandstones, and coal seams, is primarily found downstream of the Hekou dam site near Poji Town, with limited outcrops near Tangfang Town at the reservoir tail. Overlying this, the Upper Permian Changxing Formation features shale interbedded with bioclastic limestone. The Lower Triassic Feixianguan Formation includes siltstone, fine sandstone, shale interbedded with limestone, and oolitic limestone, while Quaternary deposits are composed of sand, gravel, angular fragments, silt, and clay. The Upper Permian Emeishan Basalt Formation, extensively distributed in the area, is notable for its weak interlayers, which soften significantly upon water exposure, reducing strength and increasing the likelihood of soil layer slippage. Furthermore, sandstone, mudstone, shale, and coal-bearing strata with transitional marine-terrestrial facies exhibit strong permeability, facilitating water infiltration and softening of interbedded shales and mudstones, which in turn promote landslides. The sand-shale formations, interspersed with coal layers, possess low strength and high weathering susceptibility, further amplifying the region’s vulnerability to landslides (Zheng et al., 2021).
Zhenxiong County has a subtropical plateau monsoon climate, characterized by distinct altitudinal variations. Due to its topography, with higher elevations in the south and lower elevations in the north, the mountain ranges predominantly run north-south or southwest-northeast. Cold air masses from the northwest are forced upward, resulting in frequent fog and fewer sunny days. The average annual temperature is around 15°C, with moderate summers and relatively cold winters. The county’s diverse terrain and significant altitude differences create distinct climate zones: high-altitude areas are cool and humid, while lower elevations are warmer. Rainfall is concentrated during the rainy season from June to August, accounting for 47%–76% of the annual precipitation, and the region experiences an average of 130 rainy days per year, making it one of the wettest areas in China. The combination of complex geology, steep terrain, abundant rainfall, and intense human activities—such as widespread coal mining and rapid infrastructure development—has led to considerable environmental degradation. As a result, Zhenxiong County is highly susceptible to geological hazards.
3 Methods
To construct the landslide disaster database, we primarily utilize human-computer interactive visual interpretation, supplemented by 3S technologies (GIS, RS, GPS) and literature-based validation methods for landslide identification and cataloging. This process involves two key steps: (1) digitizing landslide identification graphical data to establish a graphical database and (2) inputting associated attribute data to form an attribute database. Through calibration, processing, editing, and verification, a comprehensive and accurate landslide catalog database is ultimately created. The method’s workflow is illustrated in Figure 3.
3.1 Construction method of graphic database
The graphical construction method primarily employs human-computer interactive visual interpretation, a technique that combines expert observation with computer-based image processing to enhance accuracy and efficiency in geological hazard identification, particularly for landslides. This approach effectively leverages human expertise alongside the computational power of modern image processing tools. Unlike traditional visual interpretation methods, it integrates real-time analysis software, such as GIS, which provides immediate statistical feedback on identified results. This capability allows operators to monitor overarching landslide trends dynamically during the identification process. Recent advancements in machine learning-based image recognition have further supported landslide detection (Yang and Xu, 2022; Saha et al., 2024; Sharma et al., 2024; Yang et al., 2024). However, compared to these machine learning techniques, human-computer interactive visual interpretation retains a key advantage: the incorporation of expert judgment. This method enables users to interact with the system, guiding it to refine identification parameters for greater accuracy. Additionally, it facilitates deeper insights by allowing experts to interpret and expand on computer-generated data. This iterative feedback loop between expertise and computational analysis significantly enhances both precision and efficiency.
This work primarily utilizes high-resolution, three-dimensional optical remote sensing imagery provided by the Google Earth Pro platform. The satellite imagery is an integration of multisource remote sensing data, including SPOT5 (2.5 m resolution imagery), QuickBird commercial satellite (0.6 m resolution), IKONOS (1 m resolution), Landsat8, WorldView-1 and WorldView-2 satellites (0.5 m resolution), WorldView-3 (0.3 m resolution), WorldView-4 (panchromatic resolution 0.3 m), and GeoEye-1 (0.5 m resolution). Google Earth continuously expands its imagery database and employs advanced data-mining techniques to reduce the effects of cloud cover and atmospheric interference, thereby improving image clarity and usability for analysis. This platform enables multi-angle, comprehensive observation of regional terrain features and landform characteristics (Yu et al., 2024), providing advantageous conditions for human-computer interactive visual interpretation (Yu et al., 2022). In this study, the research area is defined and divided into multiple sub-regions to ensure no areas are missed during the interpretation process. Occasionally, cloud cover obscures some sections; however, Google Earth Pro’s historical imagery function allows us to review these regions over time, enabling more accurate and complete landslide identification across the entire study area.
Landslide identification primarily depends on human visual judgment, requiring personnel to have specialized knowledge of landslide characteristics and assessment criteria. The process relies on identifying discrepancies in color, shape, and texture between the landslide mass and the surrounding geological context, such as landforms and rivers. Key morphological features, including the back scarp, perimeter, and accumulation body, serve as fundamental criteria. Special attention is given to areas with abrupt topographic changes, where regions showing landslide characteristics are accurately delineated using vector polygons. A fully developed landslide should include the following components: the landslide mass, landslide bed, slip surface, back scarp, landslide tongue, landslide steps, and landslide depression, as shown in Table 1. However, not all landslides possess all of these features; nonetheless, the landslide mass and back scarp are present in all cases.
The direct interpretation indicators of landslides primarily focus on the characteristics of the landslide itself in remote sensing images, such as shape, tone, and texture. Shape characteristics: Due to the downward movement of the landslide body, the terrain in the three directions (left, right, and rear) of the landslide tends to be slightly higher, giving it an overall shape resembling a horseshoe, circular chair, bullhorn, or tongue, with the rear wall opening towards the slope base. Tone characteristics: Newly occurred landslides often appear in light tones such as grayish-white or bluish-white due to the destruction of surface vegetation and soil fragmentation. The tone distribution is uneven. Landslide scars tend to appear lighter in tone because they reflect more light, while landslide depressions may appear darker, especially when water accumulates. For older landslides, the recovery of surface vegetation diminishes these color features, but they can still be differentiated from the surrounding tones. Texture characteristics: The original stratigraphic integrity is disrupted, resulting in exposed soil, overturned vegetation, and a fragmented surface. This leads to a rough texture with patchy shadow effects visible in the imagery. The indirect interpretation indicators of landslides primarily focus on environmental factors around the landslide, such as vegetation distribution, topography, geological structure, hydrological information, and ecological landscapes. Vegetation characteristics: For slow-moving or old landslides, the continuous downward movement of the landslide body, combined with the upward growth of trees, results in phenomena like “scythe trees” and “drunken forest” on the landslide surface, which are particularly evident in high-resolution aerial imagery. Hydrological characteristics: Irregular water system patterns on the landslide body, sudden changes in river flow directions at the base of the slope, or narrowing of river channels can indirectly indicate the presence of a landslide. Topographical features: Poor continuity of the landform often results in unique “steep slope + gentle slope” landforms, and the area below the landslide body may exhibit uneven terrain due to the pressure exerted by the sliding mass.
Frequent operations during the identification process may lead to geometric self-intersection issues. Although apparent errors can often be detected manually, smaller discrepancies may evade visual inspection, making algorithmic identification necessary. Unresolved self-intersection issues can hinder the conversion of features into the required GIS format, causing complications in subsequent analyses. Verification is therefore essential after data construction to ensure database integrity and accuracy. This involves using topology checking tools in GIS software to detect self-intersections in polygon features. Identified geometric issues are then corrected to maintain data quality.
3.2 Construction method of attributing database
Collected information is structured into a database, where each landslide point corresponds to a unique attribute record, ensuring precise matching between graphical and attribute data. This includes details such as location, area size, geometric perimeter, and associated geographical factors like elevation, slope, curvature, lithology, and proximity to faults. After data entry, the attribute data undergoes verification and correction to ensure accuracy and completeness. Different experts independently interpret landslide areas using identical satellite imagery and topographic data, recording key characteristics such as location, area size, and boundaries. Their results are compared to calculate consistency indices that quantify the accuracy and reliability of interpretations. Discrepancies are collectively reviewed to identify error sources and refine unified interpretation standards. The final database is stored in shapefile format, comprising the main (.shp), index (.shx), and attribute (.dbf) files, which enable standardized management of geological disaster data. Statistical analysis of attribute data reveals patterns and characteristics of disaster occurrences. For instance, analyzing disaster frequency and regional distribution helps identify high-risk areas and temporal-spatial patterns, providing critical support for disaster early warning and prevention.
4 Results
4.1 The result of landslide identification
Based on the high-resolution optical remote sensing images provided by the Google Earth Pro platform, a detailed interpretation of landslides in the Zhenxiong County area (covering 3,696 km2) was conducted using a human-computer interaction visual interpretation method. A total of 3,979 landslides were identified, encompassing a combined area of 319.20 km2. The largest landslide, measuring 4.55 km2, represents a significant ancient slide that diverted a river by filling a valley. In contrast, the smallest landslide covered just 1,779 m2, while the average landslide area across the study area was 80,215 m2 (Figure 4A). Statistical analysis revealed that there are 72 landslides larger than 0.5 km2, accounting for 1.81% of the total number of landslides, with 15 landslides exceeding 1 km2. Additionally, there are 758 landslides with areas between 0.10 km2 and 0.5 km2, 880 landslides between 0.05 km2 and 0.10 km2, and 2,046 landslides ranging from 0.01 km2 to 0.05 km2. Only 223 landslides have an area smaller than 0.01 km2, as illustrated in Figure 4B. Landslides were categorized by area into small (<10,000 m2), medium (10,000 m2–100,000 m2), and large (>100,000 m2) landslides. It was found that the vast majority (73.54%) of landslides in Zhenxiong County are medium-sized landslides, followed by large landslides, which account for 20.86% of the total number. Small landslides constitute only 5.60% of the total landslide count. Several factors contribute to the prevalence of larger landslides: 1) The morphological features and geomorphology of large landslides are more pronounced, making them easier to identify; 2) Smaller landslides tend to lose their characteristics over time due to erosion, making them difficult or even impossible to recognize; 3) Smaller landslides are more susceptible to vegetation cover, which hampers identification. To gain a deeper understanding of the development of landslide relics in Zhenxiong County, GIS software was utilized to calculate the landslide area percentage (LAP) and landslide point density (LND) across the entire study area. The results showed that LAP and LND were 8.64% and 1.077 landslides per km2, respectively, indicating a significant development of both the number and area of landslides in the county.
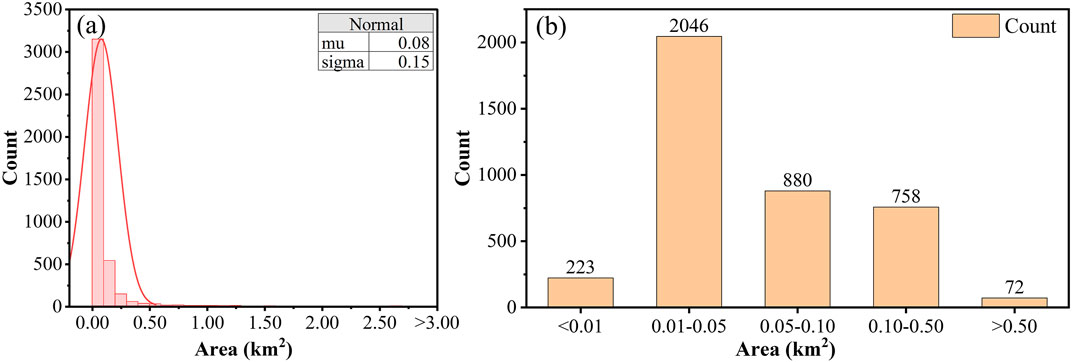
Figure 4. Distribution of landslide area scale, (A) Area histogram, (B) Distribution of landslide area and quantity.
4.2 Spatial distribution of landslide
Overlaying the identified landslides on the elevation map reveals that most are distributed between 1,000 and 2,000 m in elevation, as shown in Figure 5. Statistical analysis of the landslide distribution based on geographic coordinates indicates that landslide density is significantly higher between 104°17′E and 104°47′E compared to other longitudinal areas. Similarly, in the latitudinal range of 27°40′N to 27°25′N, landslide density is notably higher than in other latitudes. Consequently, landslides in Zhenxiong County are primarily concentrated in the northwestern and southwestern regions. To clearly identify areas with higher landslide densities, we used the kernel density tool in GIS software to calculate landslide point density, setting the search radius to 5 km. As shown in Figure 6, the highest density reaches 3.38 landslides per km2. The maximum density is concentrated in four specific regions: the confluence of the Baishui River and Yanxi River in the northwest, the Dahuigou Reservoir, the valleys along both sides of the river from Heitang Village to Hongyan Village, and Xiaogou Village. These areas feature well-developed drainage systems and mid-to-low mountainous terrain, with the western canyons being particularly significant for landslide occurrences. In contrast, the eastern part of Zhenxiong County has relatively flat terrain, with a more uniform landslide distribution. The area around Hongjiayuanzi Village shows a concentrated landslide distribution, where higher elevations and significant topographic variations (with a maximum elevation of 2,300 m) make the terrain more susceptible to geological factors contributing to landslides.
4.3 Typical landslide
This database includes several typical landslide morphologies, such as “hoop chair,” tongue-like, oval, and “shovel” shapes. Geomorphologically, the features typically exhibit dual ditches with a common source, cracks and cliff faces at the back of the landslide, a distinct boundary between the landslide mass and surrounding mountains, steep steps or benches on the landslide body, and landslide deposits that obstruct rivers, causing unusual river diversions. Most of the landslide relics are ancient, having undergone long-term geological evolution that often modifies them, making identification challenging. However, the boundaries of the landslides are usually clearly visible, and the deposits are distinctly marked, with color differences that set them apart from the surrounding vegetation and terrain. As shown in Figure 7, the thick white dashed line indicates the overall boundary of the landslide, the white arrows denote the rear edge where material has slid down from the highest point, the yellow arrow shows the direction of landslide movement, and the thin white dashed line represents the landslide deposits.
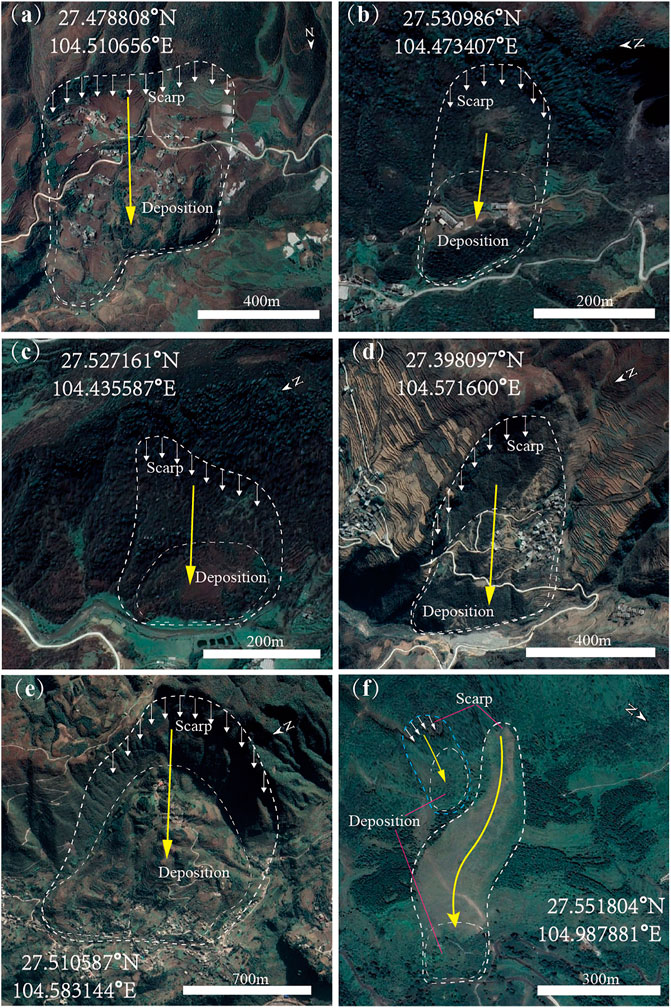
Figure 7. Pictures of typical landslides. (A–F) are images of landslides with relatively clear morphology from the landslide inventory.
Figure 7A illustrates a typical landslide located in Zhangzhai Village, covering an area of 0.31 km2. As shown in the figure, the landslide mass has slid down from the southern mountain, forming deposits below. Upon closer inspection, the length of the deposits on the left side of the landslide is notably greater than on the right side. This discrepancy may be attributed to the heterogeneous nature of the rock masses on the left and right sides during the sliding process. Alternatively, it is possible that the left half of the landslide experienced a secondary sliding event after some time, resulting in a larger deposit area on that side. This has led to a noticeable anomaly in the valley’s orientation. Given the long time since the event, settlements have been constructed on the landslide mass. Figure 7B shows another typical landslide located on the southern mountain of Zhongzhai Village, covering 0.09 km2. The landslide has an elliptical shape, with a clear boundary between the landslide scarp and the landslide body, as well as a distinct demarcation between the landslide deposits and the surrounding environment. Similar to the previous example, this landslide has caused an abnormal valley orientation. Over time, human activity has resulted in the creation of terraced fields on the landslide mass. Figure 7C depicts a typical landslide situated on the south bank of the Huangshui River, with an area of 0.08 km2. This landslide is narrow at the top and widens at the bottom, sliding down the mountain at an inclined angle. Moreover, based on the vegetation on the southern bank of the river below, it can be inferred that a small section on the left side of the landslide deposits has also undergone secondary sliding, indicating the instability of the surrounding mountains and their susceptibility to future landslides. Figure 7D depicts a typical landslide located in Fengyan Village, covering an area of 0.30 km2. This landslide slid northwest from the eastern side of Fengyan Village, with its rear edge still visible. Due to the long time elapsed since the landslide event, settlements have been built on the landslide mass. The landslide has created a significant elevation difference from east to west on the mountain, causing the terraces built by humans to display a discontinuous topography stretching from northeast to southwest. The original southwest-northeast oriented valley was disrupted by the landslide deposits, resulting in the valley shifting approximately 180 m to the northwest. Figure 7E features another typical landslide in Sunjiagou Village, covering an area of 1.21 km2, classified as a large landslide. Erosion gullies have developed on both sides of the landslide, demonstrating the typical dual-ditch morphology with the same source. Due to the considerable size of the landslide, numerous settlements and terraced fields have developed on and around the landslide mass. Lastly, Figure 7F illustrates a landslide that occurred on 11 January 2013, in Zhaojiagou Village, with an area of 0.91 km2. The source, sliding area, and slope morphology of the landslide exhibit a zigzag shape (indicated by the yellow arrows), resembling “boot-shaped terrain.” The overall slope of the rear edge ranges from approximately 50°–90°, with the ridge consisting of steep limestone cliffs at an elevation of about 1800–2000 m. Beneath the cliffs lies a gently sloping “bulge” with an elevation of around 1,690–1800 m. Additionally, a smaller landslide, located southeast of the primary landslide, is enclosed by the blue dashed line in Figure 7F, with a height difference of approximately 151 m between the source and deposit areas.
5 Discussion
5.1 Landslide identification technology
In the past decade, landslide identification technology has advanced from traditional field geological survey methods (Wei et al., 2010) to semi-automatic recognition through human-computer interaction, and more recently, to fully automated recognition using machine learning algorithms (Van Den Eeckhaut et al., 2012; Moosavi et al., 2014; Wang Y. et al., 2024). These new landslide identification methods offer several advantages over traditional techniques, such as faster processing and lower costs (Xun et al., 2019; Pang et al., 2022). However, despite these advances, landslide relic identification still primarily relies on semi-automated methods, particularly human-computer interaction visual interpretation. Machine learning technologies have become widely applied in landslide identification due to continuous improvements in algorithm performance (Wang et al., 2023; Bhuvaneswari et al., 2024; Yang et al., 2024). Nonetheless, the accuracy of identification remains inconsistent (Moosavi et al., 2014). For example, when geological environment data are used as training samples, machine learning algorithms may mistakenly classify non-landslide areas as landslides due to similarities in environmental features, which reduces identification accuracy. Additionally, automatic recognition technologies based on image or pixel comparison may merge multiple adjacent landslides into a single large landslide, compromising the accuracy of area size and impact assessments. Furthermore, landslide recognition methods based on visible remote sensing imagery can erroneously identify cultivated land or deforested areas as landslides due to color differences (Li C. et al., 2024). This issue is especially problematic for ancient landslides, whose characteristics may have gradually faded due to vegetation changes and human engineering activities, significantly reducing the effectiveness of automatic landslide identification.
Human-computer interaction visual interpretation technology can partially compensate for the limitations of automatic identification methods, offering significant advantages in the accuracy and completeness of landslide relic identification (Li et al., 2021; Huang et al., 2023a; Wang W. et al., 2024). However, this technology also faces several challenges. For example, optical remote sensing relies on favorable optical conditions, making it difficult to capture clear surface images in foggy or cloudy weather. Additionally, this method requires human experts to have substantial geological and geomorphological knowledge to effectively guide the system’s analysis and decision-making. Furthermore, current technology cannot identify landslides in the initial sliding stage or those experiencing minor deformations, requiring the integration of other techniques, such as InSAR, for more comprehensive identification and analysis (Antonielli et al., 2019; Li N. et al., 2024). Moreover, this identification method still incurs significant labor and time costs. In terms of objectivity, past experiences often necessitate field surveys for validation. However, the areas accessible to humans and the perspectives available during on-site investigations are frequently limited. To address this, researchers often use small devices like drones for observation (Yavuz et al., 2023), which offers advantages similar to satellite imagery. As a result, the application of human-computer interaction visual interpretation on satellite images is nearly indistinguishable from field surveys, and this method has been validated in other studies (Li et al., 2021; Liu et al., 2023; Wang W. et al., 2024; Zhang et al., 2024), fully meeting the requirements for identifying landslide relics. However, enhancing the precision of landslide recognition while maximizing automation remains an area of ongoing research. With the advancement of deep learning technology, future landslide identification techniques will likely increasingly rely on artificial intelligence algorithms, such as Convolutional Neural Networks (CNN) and Generative Adversarial Networks (GAN), to improve both the automation and accuracy of identification. As the quality of landslide data continues to improve, the accuracy of automatic landslide recognition will also increase. This highlights the importance of high-quality basic landslide data, suggesting that future automatic landslide recognition technologies and the quality of existing data will be mutually reinforcing.
5.2 Application of landslide data
A highly accurate, complete, and detailed landslide data inventory is playing an increasingly important role in the field of landslide geological hazard research. Firstly, the establishment of the landslide inventory will fill the gap in the basic data on landslide disasters in the study area, providing solid data support for disaster prediction and risk assessment. The landslide inventory is a core foundational dataset for landslide disaster management. It includes key information such as the location and size of landslides, providing a reliable basis for governments and relevant departments to develop precise disaster prevention and reduction strategies. For example, the inventory can help identify high-frequency landslide areas and potential hazard zones, supporting disaster risk zonation and management.
Secondly, the landslide inventory provides essential parameter inputs for landslide susceptibility assessment. Based on the landslide inventory, regional landslide susceptibility models can be developed, especially as recent studies increasingly focus on using landslide databases to establish regional landslide susceptibility, hazard assessment, and risk evaluation (Miao et al., 2023; Abdo and Richi, 2024; Chicas et al., 2024; Guo et al., 2024; Kassa, 2024; Kaur et al., 2024). Such studies require substantial data as the foundational basis for model development (Huang et al., 2024; Ma et al., 2024a; Ma et al., 2024b; Shao et al., 2024a; Sun et al., 2024a; Wu et al., 2024), particularly for training samples in machine learning algorithms (Tang et al., 2023; Zhuo et al., 2023; Singh et al., 2024). These data are crucial for determining the reliability and accuracy of the models. For instance, due to the inability to obtain a complete landslide database for the high-altitude regions of the Himalayas, Du et al. developed a quantitative method that combines heuristic and multi-class statistical models to assess landslide susceptibility in areas with incomplete inventory data and high uncertainty in landslide interpretation (Du et al., 2020). While this method somewhat mitigates the issue of sparse landslide data, it still faces challenges in verifying model accuracy. With a relatively complete landslide inventory, there would be enough samples to validate the accuracy of model methods and further enhance model precision. Sahrane et al. found that studying landslide susceptibility in homogeneous and heterogeneous environments requires the use of different datasets (Sahrane et al., 2023). Landslide inventories with limited data may be reliable in monotonous and repetitive areas, but they often prove unreliable in regions with significant geological and geomorphological diversity (Fu et al., 2020). In contrast, this study effectively addresses the issue of inaccurate risk assessment models by conducting detailed identification of landslide geological hazards in Zhenxiong County.
Finally, the establishment of the landslide inventory provides a data foundation for optimizing monitoring and early warning systems. The inventory data enables the identification of key monitoring areas, optimization of monitoring point layouts, and improvement in the accuracy and efficiency of disaster monitoring. For example, deploying comprehensive monitoring equipment such as surface displacement sensors, rain gauges, and groundwater level meters in high-risk landslide zones can significantly enhance early warning capabilities. Moreover, the inventory can serve as calibration data for landslide simulations. By analyzing historical landslide events, it helps improve the accuracy and reliability of numerical simulations, supporting research on dynamic evolution and triggering mechanisms of landslides.
5.3 Compared with previous studies
Research on regional landslide disasters has been increasingly prevalent, leading to the establishment of numerous landslide inventories across various regions (Shen et al., 2023; Rüther et al., 2024; Shi et al., 2024). Most of these inventories have been created for the purpose of training machine-learning models or investigating landslide disasters in specific scenarios (Gao et al., 2024; Li et al., 2024c; Yingze et al., 2024). As a result, the completeness of the landslide data in these inventories may not be fully representative of the areas in question. Table 2 presents previous studies related to landslide disasters in the vicinity of Zhenxiong County, all of which include landslide relic inventories. To evaluate the completeness and detail of these inventories, the authors used landslide density (i.e., the number of landslides per unit area) as a metric. Since Zhenxiong County is located in the northeastern part of Yunnan Province, the sources of these studies were selectively drawn from this region whenever possible. In total, the authors reviewed ten research outcomes, nine of which were conducted within Yunnan Province, with two covering the entire province. Additionally, one study was from Bijie City in neighboring Guizhou Province, which borders Zhenxiong. This approach ensures the comparability of the landslide inventories.
A comparative analysis of landslide inventories from Zhenxiong County and surrounding areas reveals deficiencies in detail and completeness in inventories from other regions. These deficiencies are mainly reflected in the following aspects: (1) Differences in the purposes of landslide inventory compilation have led to varying levels of data completeness. Some landslide inventories were created primarily for machine learning training or specific geological phenomena studies (Gao and Wang, 2016; Ji et al., 2020; Cheng et al., 2021). In such cases, data collection often emphasizes the representativeness of landslide features rather than the comprehensiveness of landslide events. (2) The scope of the study area influences the detail of landslide records. Certain studies encompass broad areas, which limits detailed records of landslide disasters within smaller, specific areas. Compared with Wang et al. and Wu’s research, inventories covering the entire Yunnan Province provide broad coverage but often overlook landslide events in localized areas like Zhenxiong County, thus failing to fully capture landslide distribution and frequency in such regions (Wang et al., 2014; Wu, 2015). (3) The methods of landslide data collection also impact inventory detail. Studies that incorporate high-resolution satellite imagery and drone data tend to achieve more comprehensive landslide information compared to those relying solely on field surveys or historical records. (4) Variations in inventory standards and data processing approaches lead to discrepancies. Some studies apply differing landslide definitions or data filtering methods, resulting in biases in landslide density calculations. For instance, certain inventories record only large-scale or high-impact landslides, omitting smaller or non-lethal events. These landslide lists cannot fully reflect the actual situation of regional landslides, especially in mountainous areas with frequent landslides but small scale. This study aims to achieve a comprehensive identification of historical landslide relics to accurately reflect the landslide hazards in the study area. Consequently, the landslide inventory presented here shows a higher density (1.077 landslides per km2) than those in previous studies.
5.4 Research prospects
Zhenxiong County, located in northeastern Yunnan Province, is characterized by a complex geological environment and frequently experiences landslide disasters. The severe landslide event in Liangshui Village, Tangfang Town, on 22 January 2024, has underscored the urgent need for landslide research and early warning systems in the region. This study primarily focuses on establishing a comprehensive and accurate inventory of landslide relics within Zhenxiong County and provides a preliminary analysis of landslide size and spatial distribution. Moving forward, we plan to conduct a more detailed analysis of landslide distribution in relation to various environmental factors, including elevation, slope, aspect, proximity to rivers, and lithology. Based on this understanding of landslide spatial distribution, the study will then assess landslide susceptibility across Zhenxiong County. Additionally, by incorporating local rainfall and seismic activity data, we will conduct an analysis of landslide hazards to develop a comprehensive risk assessment model. This model aims to evaluate the potential risks of landslides in the study area, providing critical technical support for the prevention and mitigation of regional landslide disasters.
6 Conclusion
This study utilizes a human-computer interactive visual interpretation method on the Google Earth Pro platform to conduct a detailed identification of landslides in Zhenxiong County, Yunnan Province. As a result, the most comprehensive landslide relic inventory to date for Zhenxiong County has been developed. Findings indicate that, within Zhenxiong’s 3,696 km2 area, at least 3,979 landslide relics have occurred. Landslide-affected areas total approximately 319.20 km2, with the largest single landslide covering 4.55 km2 and the smallest extending over 1,779 m2. The average landslide area across the study region is 80,215 m2. Statistical analysis reveals that the majority (73.54%) of landslides in Zhenxiong County are classified as medium-sized landslides, followed by large landslides, accounting for 20.86% of total landslide occurrences, while small landslides constitute only 5.60% of the total. Landslides in Zhenxiong County are primarily concentrated in four areas: the confluence of the Baishui River and Yanxi River in the northwest, Dashuigou Reservoir, the valley along both sides from Heitang Village to Hongyan Village, and the Xiaogou Village area. The water systems in these areas are generally well-developed, and the landforms are mostly middle and low mountains. The landslide relic inventory developed in this study at the county scale for Zhenxiong County provides a reliable dataset for future landslide geological hazard research and offers a scientific basis for comprehensive disaster prevention and mitigation efforts in the region.
Data availability statement
The raw data supporting the conclusions of this article will be made available by the authors, without undue reservation.
Author contributions
ZX: Data curation, Formal Analysis, Investigation, Methodology, Writing–original draft, Writing–review and editing. CX: Project administration, Writing–review and editing. ZZ: Funding acquisition, Writing–review and editing. LF: Data curation, Writing–review and editing. HL: Funding acquisition, Writing–review and editing. HZ: Funding acquisition, Writing–review and editing. DZ: Funding acquisition, Writing–review and editing. JS: Data curation, Writing–review and editing. PW: Data curation, Writing–review and editing. LL: Data curation, Writing–review and editing. JC: Data curation, Writing–review and editing.
Funding
The author(s) declare that financial support was received for the research, authorship, and/or publication of this article. This work was supported by a grant from the Science and Technology Project of China Southern Power Grid (SEPRI-K23A018), Research Institute of China Southern Power Grid Co., Ltd. [1500002024030103SJ000003 (CG1500062001647685-001)], Research Institute of China Southern Power Grid Co., Ltd. [1500002024030103SJ00009 (CG1500062001634723-001)], and the National Institute of Natural Hazards, Ministry of Emergency Management of China (2023-JBKY-57). The authors declare that this study received funding from China Southern Power Grid Co., Ltd. The funder was not involved in the study design, collection, analysis, interpretation of data, the writing of this article, or the decision to submit it for publication.
Conflict of interest
Authors ZZ, HZ, and DZ were Research Institute of China Southern Power Grid Co., Ltd.
Author LF was employed by Jiangsu World Group.
Author HL was employed by Yunnan Power Grid Co., Ltd.
Author JS was employed by Zhejiang Metallurgical Survey and Design Co., Ltd.
Author PW was employed by Beijing Engineering Corporation Limited.
The remaining authors declare that the research was conducted in the absence of any commercial or financial relationships that could be construed as a potential conflict of interest.
The author(s) declared that they were an editorial board member of Frontiers, at the time of submission. This had no impact on the peer review process and the final decision.
Generative AI statement
The author(s) declare that no Generative AI was used in the creation of this manuscript.
Publisher’s note
All claims expressed in this article are solely those of the authors and do not necessarily represent those of their affiliated organizations, or those of the publisher, the editors and the reviewers. Any product that may be evaluated in this article, or claim that may be made by its manufacturer, is not guaranteed or endorsed by the publisher.
References
Abdo, H. G., and Richi, S. M. (2024). Application of machine learning in the assessment of landslide susceptibility: a case study of mountainous eastern Mediterranean region, Syria. J. King Saud University-Science 36, 103174. doi:10.1016/j.jksus.2024.103174
Antonielli, B., Mazzanti, P., Rocca, A., Bozzano, F., and Dei Cas, L. (2019). A-DInSAR performance for updating landslide inventory in mountain areas: an example from Lombardy region (Italy). Geosciences 9, 364. doi:10.3390/geosciences9090364
Aristizábal, E., and Sánchez, O. (2020). Spatial and temporal patterns and the socioeconomic impacts of landslides in the tropical and mountainous Colombian Andes. Disasters 44, 596–618. doi:10.1111/disa.12391
Barella, C. F., Sobreira, F. G., and Zêzere, J. L. (2019). A comparative analysis of statistical landslide susceptibility mapping in the southeast region of Minas Gerais state, Brazil. Bull. Eng. Geol. Environ. 78, 3205–3221. doi:10.1007/s10064-018-1341-3
Bhuvaneswari, T., Sekar, R. C. G., Selvi, M. C., Rubavathi, J. J., and Kaviyaa, V. (2024). Robust deep learning for accurate landslide identification and prediction. Dokl. Earth Sci. 518, 1700–1708. doi:10.1134/S1028334X23602961
Bueechi, E., Klimeš, J., Frey, H., Huggel, C., Strozzi, T., and Cochachin, A. (2019). Regional-scale landslide susceptibility modelling in the Cordillera Blanca, Peru—a comparison of different approaches. Landslides 16, 395–407. doi:10.1007/s10346-018-1090-1
Cheng, L., Li, J., Duan, P., and Wang, M. (2021). A small attentional YOLO model for landslide detection from satellite remote sensing images. Landslides 18, 2751–2765. doi:10.1007/s10346-021-01694-6
Chicas, S. D., Li, H., Mizoue, N., Ota, T., Du, Y., and Somogyvári, M. (2024). Landslide susceptibility mapping core-base factors and models’ performance variability: a systematic review. Nat. Hazards 120, 12573–12593. doi:10.1007/s11069-024-06697-9
Conforti, M., Muto, F., Rago, V., and Critelli, S. (2014). Landslide inventory map of north-eastern Calabria (South Italy). J. Maps 10, 90–102. doi:10.1080/17445647.2013.852142
Coviello, V., Palo, M., Adirosi, E., and Picozzi, M. (2024). Seismic signature of an extreme hydro-meteorological event in Italy. npj Nat. Hazards 1, 17. doi:10.1038/s44304-024-00018-7
Cui, Y., Yang, W., Xu, C., and Wu, S. (2023). Distribution of ancient landslides and landslide hazard assessment in the Western Himalayan Syntaxis area. Front. Earth Sci. 11, 1135018. doi:10.3389/feart.2023.1135018
Devoli, G., Strauch, W., Chávez, G., and Høeg, K. (2007). A landslide database for Nicaragua: a tool for landslide-hazard management. Landslides 4, 163–176. doi:10.1007/s10346-006-0074-8
Du, J., Glade, T., Woldai, T., Chai, B., and Zeng, B. (2020). Landslide susceptibility assessment based on an incomplete landslide inventory in the Jilong Valley, Tibet, Chinese Himalayas. Eng. Geol. 270, 105572. doi:10.1016/j.enggeo.2020.105572
Feng, L., Qi, W., Xu, C., Yang, W., Yang, Z., Xiao, Z., et al. (2024a). Landslide research from the perspectives of qinling mountains in China: a critical review. J. Earth Sci. 35, 1546–1567. doi:10.1007/s12583-023-1935-9
Feng, L., Xu, C., Tian, Y., Li, L., Sun, J., Huang, Y., et al. (2024b). Landslides of China's qinling. Geoscience Data J. 11, 725–741. doi:10.1002/gdj3.246
Frodella, W., Ciampalini, A., Bardi, F., Salvatici, T., Di Traglia, F., Basile, G., et al. (2018). A method for assessing and managing landslide residual hazard in urban areas. Landslides 15, 183–197. doi:10.1007/s10346-017-0875-y
Fu, S., Chen, L., Woldai, T., Yin, K., Gui, L., Li, D., et al. (2020). Landslide hazard probability and risk assessment at the community level: a case of western Hubei, China. Nat. Hazards Earth Syst. Sci. 20, 581–601. doi:10.5194/nhess-20-581-2020
Gao, H., Xu, C., Xie, C., Ma, J., and Xiao, Z. (2024). Landslides triggered by the july 2023 extreme rainstorm in the haihe river basin, China. Landslides 21, 2885–2890. doi:10.1007/s10346-024-02322-9
Gao, J., and Wang, Q. (2016). Application of analytical hierarchy process method for landslide susceptibility mapping using GIS. Electron. J. Geotechnical Eng. 21, 6615–6627.
Gómez, D., García, E. F., and Aristizábal, E. (2023). Spatial and temporal landslide distributions using global and open landslide databases. Nat. Hazards 117, 25–55. doi:10.1007/s11069-023-05848-8
Guo, J., Xi, W., Yang, Z., Shi, Z., Huang, G., Yang, Z., et al. (2024). Landslide hazard susceptibility evaluation based on SBAS-InSAR technology and SSA-BP neural network algorithm: a case study of Baihetan Reservoir Area. J. Mt. Sci. 21, 952–972. doi:10.1007/s11629-023-8083-9
Hosseini, K., Reindl, L., Raffl, L., Wiedemann, W., and Holst, C. (2024). 3D landslide monitoring in high spatial resolution by feature tracking and histogram analyses using laser scanners. Remote Sens. 16, 138. doi:10.3390/rs16010138
Hu, X., Mei, H., Zhang, H., Li, Y., and Li, M. (2021). Performance evaluation of ensemble learning techniques for landslide susceptibility mapping at the Jinping county, Southwest China. Nat. Hazards 105, 1663–1689. doi:10.1007/s11069-020-04371-4
Huang, Y., Xu, C., He, X., Cheng, J., Huang, Y., Wu, L., et al. (2024). Distribution characteristics and cumulative effects of landslides triggered by multiple moderate-magnitude earthquakes: a case study of the comprehensive seismic impact area in Yibin, Sichuan, China. Landslides 21, 2927–2943. doi:10.1007/s10346-024-02351-4
Huang, Y., Xu, C., Li, L., He, X., Cheng, J., Xu, X., et al. (2023a). Inventory and spatial distribution of ancient landslides in Hualong County, China. Land 12, 136. doi:10.3390/land12010136
Huang, Y., Xu, C., Zhang, X., and Li, L. (2022). Bibliometric analysis of landslide research based on the WOS database. Nat. Hazards Res. 2, 49–61. doi:10.1016/j.nhres.2022.02.001
Huang, Y., Xu, C., Zhang, X., Li, L., and Xu, X. (2023b). Research in the field of natural hazards based on bibliometric analysis. Nat. hazards Rev. 24, 04023012. doi:10.1061/nhrefo.nheng-1739
Huu, D. N., Cong, T. V., Bretcan, P., and Petrisor, A.-I. (2024). Assessing the relationship between landslide susceptibility and land cover change using machine learning. Vietnam J. Earth Sci. 46, 339–359. doi:10.15625/2615-9783/20706
Hwang, J., and Lall, U. (2024). Increasing dam failure risk in the USA due to compound rainfall clusters as climate changes. npj Nat. Hazards 1, 27. doi:10.1038/s44304-024-00027-6
Jallayu, P. T., Sharma, A., and Singh, K. (2024). Vulnerability of highways to landslide using landslide susceptibility zonation in GIS: mandi district, India. Innov. Infrastruct. Solutions 9, 354. doi:10.1007/s41062-024-01653-9
Ji, S., Yu, D., Shen, C., Li, W., and Xu, Q. (2020). Landslide detection from an open satellite imagery and digital elevation model dataset using attention boosted convolutional neural networks. Landslides 17, 1337–1352. doi:10.1007/s10346-020-01353-2
Kassa, S. M. (2024). A Systematic review of machine learning based landslide susceptibility mapping. J. Road Traffic Eng. 70, 23–30. doi:10.31075/pis.70.02.03
Kaur, R., Gupta, V., and Chaudhary, B. (2024). Landslide susceptibility mapping and sensitivity analysis using various machine learning models: a case study of Beas valley, Indian Himalaya. Bull. Eng. Geol. Environ. 83, 228. doi:10.1007/s10064-024-03712-y
Li, C., Feng, P., Jiang, X., Zhang, S., Meng, J., and Li, B. (2024a). Extensive identification of landslide boundaries using remote sensing images and deep learning method. China Geol. 7, 277–290. doi:10.31035/cg2023148
Li, L., Xu, C., Xu, X., Zhang, Z., and Cheng, J. (2021). Inventory and distribution characteristics of large-scale landslides in Baoji city, Shaanxi province, China. ISPRS Int. J. Geo-Information 11, 10. doi:10.3390/ijgi11010010
Li, N., Feng, G., Zhao, Y., Xiong, Z., He, L., Wang, X., et al. (2024b). A deep-learning-Based algorithm for landslide detection over wide areas using InSAR images considering topographic features. Sensors 24, 4583. doi:10.3390/s24144583
Li, T., Xie, C., Xu, C., Qi, W., Huang, Y., and Li, L. (2024c). Automated machine learning for rainfall-induced landslide hazard mapping in Luhe County of Guangdong Province, China. China Geol. 7, 315–329. doi:10.31035/cg2024064
Li, T., Xu, C., Li, L., and Xu, J. (2024d). The landslide traces inventory in the transition zone between the Qinghai-Tibet Plateau and the Loess Plateau: a case study of Jianzha County, China. Front. Earth Sci. 12, 1–9. doi:10.3389/feart.2024.1370992
Liu, S., Zhu, J., Yang, D., and Ma, B. (2022). Comparative study of geological hazard evaluation systems using grid units and slope units under different rainfall conditions. Sustainability 14, 16153. doi:10.3390/su142316153
Liu, X., Su, P., Li, Y., Xia, Z., Ma, S., Xu, R., et al. (2023). Spatial distribution of landslide shape induced by Luding Ms 6.8 earthquake, Sichuan, China: case study of the Moxi Town. Landslides 20, 1667–1678. doi:10.1007/s10346-023-02070-2
Ma, S., Shao, X., Li, K., and Xu, C. (2024a). Landslides triggered by the 30th June 2012 Ms6.6 hejing earthquake, xinjiang province, China. Bull. Eng. Geol. Environ. 83, 256. doi:10.1007/s10064-024-03727-5
Ma, S., Shao, X., Xu, C., Chen, X., Lu, Y., Xia, C., et al. (2024b). Distribution pattern, geometric characteristics and tectonic significance of landslides triggered by the strike-slip faulting 2022 Ms 6.8 Luding earthquake. Geomorphology 453, 109138. doi:10.1016/j.geomorph.2024.109138
Mcgovern, A., Demuth, J., Bostrom, A., Wirz, C. D., Tissot, P. E., Cains, M. G., et al. (2024). The value of convergence research for developing trustworthy AI for weather, climate, and ocean hazards. npj Nat. Hazards 1, 13. doi:10.1038/s44304-024-00014-x
Miao, F., Ruan, Q., Wu, Y., Qian, Z., Kong, Z., and Qin, Z. (2023). Landslide dynamic susceptibility mapping base on machine learning and the PS-InSAR coupling model. Remote Sens. 15, 5427. doi:10.3390/rs15225427
Moosavi, V., Talebi, A., and Shirmohammadi, B. (2014). Producing a landslide inventory map using pixel-based and object-oriented approaches optimized by Taguchi method. Geomorphology 204, 646–656. doi:10.1016/j.geomorph.2013.09.012
Pang, D., Liu, G., He, J., Li, W., and Fu, R. (2022). Automatic remote sensing identification of co-seismic landslides using deep learning methods. Forests 13, 1213. doi:10.3390/f13081213
Posner, A. J., and Georgakakos, K. P. (2015). Soil moisture and precipitation thresholds for real-time landslide prediction in El Salvador. Landslides 12, 1179–1196. doi:10.1007/s10346-015-0618-x
Rosser, B., Dellow, S., Haubrock, S., and Glassey, P. (2017). New Zealand’s national landslide database. Landslides 14, 1949–1959. doi:10.1007/s10346-017-0843-6
Rüther, D. C., Lindsay, E., and Slåtten, M. S. (2024). Landslide inventory: ‘Hans’ storm southern Norway, August 7–9, 2023. Landslides 21, 1155–1159. doi:10.1007/s10346-024-02222-y
Saha, A., Tripathi, L., Villuri, V. G. K., and Bhardwaj, A. (2024). Exploring machine learning and statistical approach techniques for landslide susceptibility mapping in Siwalik Himalayan Region using geospatial technology. Environ. Sci. Pollut. Res. 31, 10443–10459. doi:10.1007/s11356-023-31670-7
Sahrane, R., Bounab, A., and El Kharim, Y. (2023). Investigating the effects of landslides inventory completeness on susceptibility mapping and frequency-area distributions: case of Taounate province, Northern Morocco. Catena 220, 106737. doi:10.1016/j.catena.2022.106737
Sepúlveda, S. A., and Petley, D. N. (2015). Regional trends and controlling factors of fatal landslides in Latin America and the Caribbean. Nat. Hazards Earth Syst. Sci. 15, 1821–1833. doi:10.5194/nhess-15-1821-2015
Shao, X., Ma, S., Xu, C., Xie, C., Li, T., Huang, Y., et al. (2024a). Landslides triggered by the 2022 Ms. 6.8 Luding strike-slip earthquake: an update. Eng. Geol. 335, 107536. doi:10.1016/j.enggeo.2024.107536
Shao, X., Xu, C., Li, L., Yang, Z., Yao, X., Shao, B., et al. (2024b). Spatial analysis and hazard assessment of large-scale ancient landslides around the reservoir area of Wudongde hydropower station, China. Nat. Hazards 120, 87–105. doi:10.1007/s11069-023-06201-9
Sharma, N., Saharia, M., and Ramana, G. (2024). High resolution landslide susceptibility mapping using ensemble machine learning and geospatial big data. Catena 235, 107653. doi:10.1016/j.catena.2023.107653
Shen, C., Zhou, S., Luo, X., Zhang, Y., and Liu, H. (2023). Using DInSAR to inventory landslide geological disaster in Bijie, Guizhou, China. Front. Earth Sci. 10, 1–15. doi:10.3389/feart.2022.1024710
Shen, Z., Zhang, Q., Wu, W., and Song, C. (2022). Spatial pattern and attribution analysis of the regions with frequent geological disasters in the Tibetan Plateau and Hengduan Mountains. Acta Geogr. Sin. 77, 1211–1224. doi:10.11821/dlxb202205012
Shi, X., Chen, D., Wang, J., Wang, P., Wu, Y., Zhang, S., et al. (2024). Refined landslide inventory and susceptibility of Weining County, China, inferred from machine learning and Sentinel-1 InSAR analysis. Trans. GIS 28, 1594–1616. doi:10.1111/tgis.13202
Shu, B., Chen, Y., Amani-Beni, M., and Zhang, R. (2022). Spatial distribution and influencing factors of mountainous geological disasters in southwest China: a fine-scale multi-type assessment. Front. Environ. Sci. 10, 1–15. doi:10.3389/fenvs.2022.1049333
Singh, A., Dhiman, N., Kc, N., and Shukla, D. P. (2024). Improving ML-based landslide susceptibility using ensemble method for sample selection: a case study of Kangra district in Himachal Pradesh, India. Environ. Sci. Pollut. Res., 1–24. doi:10.1007/s11356-024-34726-4
Sultana, N. (2020). Analysis of landslide-induced fatalities and injuries in Bangladesh: 2000-2018. Cogent Soc. Sci. 6, 1737402. doi:10.1080/23311886.2020.1737402
Sun, J., Shao, X., Feng, L., Xu, C., Huang, Y., and Yang, W. (2024a). An essential update on the inventory of landslides triggered by the Jiuzhaigou Mw6.5 earthquake in China on 8 August 2017, with their spatial distribution analyses. Heliyon 10, e24787. doi:10.1016/j.heliyon.2024.e24787
Sun, J., Xu, C., Feng, L., Li, L., Zhang, X., and Yang, W. (2024b). The yinshan mountains record over 10,000 landslides. Data 9, 31. doi:10.3390/data9020031
Tang, L., Yu, X., Jiang, W., and Zhou, J. (2023). Comparative study on landslide susceptibility mapping based on unbalanced sample ratio. Sci. Rep. 13, 5823. doi:10.1038/s41598-023-33186-z
Trigila, A., Iadanza, C., and Spizzichino, D. (2010). Quality assessment of the Italian landslide inventory using GIS processing. Landslides 7, 455–470. doi:10.1007/s10346-010-0213-0
Van Den Eeckhaut, M., Kerle, N., Poesen, J., and Hervás, J. (2012). Object-oriented identification of forested landslides with derivatives of single pulse LiDAR data. Geomorphology 173-174, 30–42. doi:10.1016/j.geomorph.2012.05.024
Wang, P., Li, L., Xu, C., Zhang, Z., and Xu, X. (2022). An open source inventory and spatial distribution of landslides in Jiyuan City, Henan Province, China. Nat. Hazards Res. 2, 325–330. doi:10.1016/j.nhres.2022.10.004
Wang, W., Huang, Y., Xu, C., Shao, X., Li, L., Feng, L., et al. (2024a). Identification and distribution of 13003 landslides in the northwest margin of Qinghai-Tibet Plateau based on human-computer interaction remote sensing interpretation. China Geol. 7, 171–187. doi:10.31035/cg2023140
Wang, X., Wang, D., Sun, T., Dong, J., Xu, L., Li, W., et al. (2023). Dual path attention network (DPANet) for intelligent identification of wenchuan landslides. Remote Sens. 15, 5213. doi:10.3390/rs15215213
Wang, X., Zhang, L., Wang, S., and Lari, S. (2014). Regional landslide susceptibility zoning with considering the aggregation of landslide points and the weights of factors. Landslides 11, 399–409. doi:10.1007/s10346-013-0392-6
Wang, Y., Gao, H., Liu, S., Yang, D., Liu, A., and Mei, G. (2024b). Landslide detection based on deep learning and remote sensing imagery: a case study in Linzhi City. Nat. Hazards Res. doi:10.1016/j.nhres.2024.07.001
Wei, F., Chernomorets, S., Aristov, K., Petrakov, D., Tutubalina, O., Su, P., et al. (2010). A Seismically triggered landslide in the Niujuan valley near the epicenter of the 2008 Wenchuan earthquake. J. Earth Sci. 21, 901–909. doi:10.1007/s12583-010-0143-8
Wu, X., Xu, X., Yu, G., Ren, J., Yang, X., Chen, G., et al. (2024). The China active faults database (CAFD) and its web system. Earth Syst. Sci. Data 16, 3391–3417. doi:10.5194/essd-16-3391-2024
Wu, Y., A, F., Yang, C., Yan, S., and Kang, X. (2023). Accuracy improvement of different landslide susceptibility evaluation models through K-Means clustering: a case study on China’s Funing county. Math. Problems Eng. 2023, 2913890. doi:10.1155/2023/2913890
Wu, Z. (2015). Analysis of Cause and Study on liability assessment of landslides and debris flow hazards in Yunnan province. Master Degree. Beijing: China University of Geosciences.
Xu, C., and Li, K. (2021). “Inventory of landslides triggered by the hejing Ms6.6 earthquake, China, on 30 June 2012,” in Understanding and reducing landslide disaster risk: volume 5 catastrophic landslides and Frontiers of landslide science. Editors V. Vilímek, F. Wang, A. Strom, K. Sassa, P. T. Bobrowsky, and K. Takara (Cham: Springer International Publishing), 73–80.
Xu, Y., Allen, M. B., Zhang, W., Li, W., and He, H. (2020). Landslide characteristics in the Loess Plateau, northern China. Geomorphology 359, 107150. doi:10.1016/j.geomorph.2020.107150
Xun, Z., Zhao, C., Liu, X., and Liu, Y. (2019). “Automatic identification of potential landslides by integrating remote sensing, DEM and deformation map,” in Igarss 2019 - 2019 IEEE international geoscience and remote sensing symposium.
Yang, Z., Qi, W., Xu, C., and Shao, X. (2024). Exploring deep learning for landslide mapping: a comprehensive review. China Geol. 7, 330–350. doi:10.31035/cg2024032
Yang, Z., and Xu, C. (2022). Efficient detection of earthquake-triggered landslides based on U-Net++: an example of the 2018 hokkaido eastern iburi (Japan) mw = 6.6 earthquake. Remote Sens. 14, 2826. doi:10.3390/rs14122826
Yavuz, M., Koutalakis, P., Diaconu, D. C., Gkiatas, G., Zaimes, G. N., Tufekcioglu, M., et al. (2023). Identification of streamside landslides with the use of unmanned aerial vehicles (UAVs) in Greece, Romania, and Turkey. Remote Sens. 15, 1006. doi:10.3390/rs15041006
Yin, Y., Liu, C., Chen, H., Ren, J., and Zhu, C. (2013). Investigation on catastrophic landslide of january 11,2013 at Zhaojiagou, Zhenxiong county, yunnan province. J. Eng. Geol. 21, 6–15.
Yin, Z., Xu, Y., and Jiang, X. (2015). The key triggering factor and its mitigation implication of Zhaojiagou catastrophic landslide in Zhenxiong County, Yunnan province. Chin. J. Geol. Hazard Control 26, 36–42. doi:10.16031/j.cnki.issn.1003-8035.2015.02.07
Yingze, S., Yingxu, S., Xin, Z., Jie, Z., and Degang, Y. (2024). Comparative analysis of the TabNet algorithm and traditional machine learning algorithms for landslide susceptibility assessment in the Wanzhou Region of China. Nat. Hazards 120, 7627–7652. doi:10.1007/s11069-024-06521-4
Yu, B., Wang, N., Xu, C., Chen, F., and Wang, L. (2022). A network for landslide detection using large-area remote sensing images with multiple spatial resolutions. Remote Sens. 14, 5759. doi:10.3390/rs14225759
Yu, X., Hu, X., Song, Y., Xu, S., Li, X., Song, X., et al. (2024). Intelligent assessment of building damage of 2023 Turkey-Syria Earthquake by multiple remote sensing approaches. npj Nat. Hazards 1, 3. doi:10.1038/s44304-024-00003-0
Zhang, X., Xu, C., Li, L., Feng, L., and Yang, W. (2024). Inventory of landslides in the northern half of the taihang mountain range, China. Geosciences 14, 74. doi:10.3390/geosciences14030074
Zhao, J., Xu, C., and Huang, X. (2024). Detailed landslide traces database of hancheng county, China, based on high-resolution satellite images available on the Google Earth platform. Data 9, 63. doi:10.3390/data9050063
Zhao, S., Dai, F., Deng, J., Wen, H., Li, H., and Chen, F. (2023). Insights into landslide development and susceptibility in extremely complex alpine geoenvironments along the western Sichuan-Tibet Engineering Corridor, China. Catena 227, 107105. doi:10.1016/j.catena.2023.107105
Zheng, Y., Chen, M., Yang, D., Long, Y., and Luo, J. (2021). Analysis on the causes and early warning and forecasting of frequent geological disasters in Zhenxiong, Yunnan province. Industrial Saf. Environ. 47, 35–38.
Zhu, Z., Yuan, X., Gan, S., Zhang, J., and Zhang, X. (2023). A research on a new mapping method for landslide susceptibility based on SBAS-InSAR technology. Egypt. J. Remote Sens. Space Sci. 26, 1046–1056. doi:10.1016/j.ejrs.2023.11.009
Keywords: geological disasters, landslide inventory, visual interpretation, disaster prevention and control, Zhenxiong County
Citation: Xue Z, Xu C, Zhang Z, Feng L, Li H, Zhang H, Zhu D, Sun J, Wang P, Li L and Chen J (2025) Inventory of landslide relics in Zhenxiong County based on human-machine interactive visual interpretation, Yunnan Province, China. Front. Earth Sci. 12:1518377. doi: 10.3389/feart.2024.1518377
Received: 28 October 2024; Accepted: 26 December 2024;
Published: 10 January 2025.
Edited by:
Rui Yong, Ningbo University, ChinaReviewed by:
Yong Nie, Chinese Academy of Sciences (CAS), ChinaYankun Wang, Yangtze University, China
Copyright © 2025 Xue, Xu, Zhang, Feng, Li, Zhang, Zhu, Sun, Wang, Li and Chen. This is an open-access article distributed under the terms of the Creative Commons Attribution License (CC BY). The use, distribution or reproduction in other forums is permitted, provided the original author(s) and the copyright owner(s) are credited and that the original publication in this journal is cited, in accordance with accepted academic practice. No use, distribution or reproduction is permitted which does not comply with these terms.
*Correspondence: Chong Xu, eGMxMTExMTExMUAxMjYuY29t