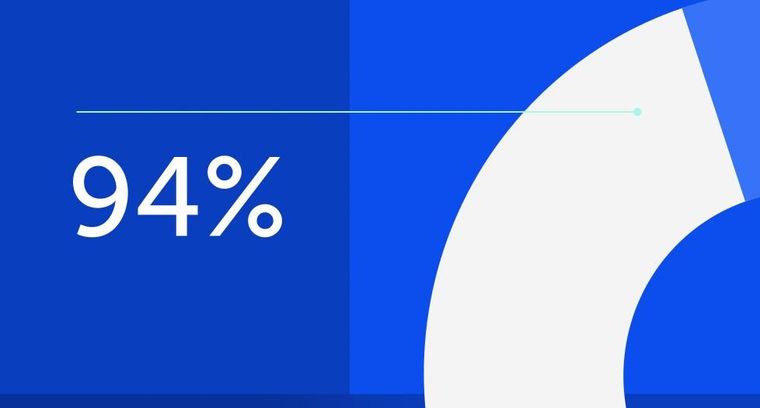
94% of researchers rate our articles as excellent or good
Learn more about the work of our research integrity team to safeguard the quality of each article we publish.
Find out more
ORIGINAL RESEARCH article
Front. Earth Sci., 11 December 2024
Sec. Geoinformatics
Volume 12 - 2024 | https://doi.org/10.3389/feart.2024.1510510
This study analyses the spatiotemporal distribution of land use and land cover (LULC) in the United Arab Emirates (UAE) over the past 50 years (1972–2021) using 72 multi-temporal Landsat satellite images. Three machine learning (ML) classifiers, Classification and Regression Tree (CART), Support Vector Machine (SVM) and Random Forest (RF), were tested, with RF finally chosen for its higher performance. Spectral, spatial, topographic, and object aspect attributes were extracted and used as input for the RF algorithm to enhance the classification accuracy. A dataset comprising 46,146 polygons representing four LULC classes was created, with 80% allocated for training and 20% for testing, ensuring robust model validation. The algorithm was trained to develop a machine learning model that classified the data into four LULC classes namely: built areas, vegetation, water, and desert and mountainous regions, producing eight thematic maps for the years 1972, 1986, 1992, 1997, 2002, 2013, 2017, and 2021. The results reveal the dominance of desert and mountainous regions, with their coverage gradually declining from over 97% in 1972 to nearly 91% in 2021. In contrast, built areas grew from less than 1% to nearly 6%, while vegetation cover increased from 0.71% to 2.85%. Water bodies have exhibited periodic fluctuations between 0.4% and 0.35%. These changes are attributed to extensive urbanization, agricultural expansion, forest plantation programs, land reclamation, and megaprojects. Accuracy assessment of the classified maps showed high overall accuracy, ranging from 85.11% to 98.4%. The study provides a unique long-term analysis of the UAE over 50 years, capturing key developments from the 1970s oil boom through subsequent megaprojects at the onset of the new millennium, leading to reduced reliance on oil. These findings underscore the role of machine learning and geospatial technologies in monitoring LULC distribution in challenging environments, and the results serve as a vital tool for policymakers to manage land resources, urban planning, and environmental conservation.
Producing accurate and timely land use and land cover (LULC) maps is essential for urban planning, disaster risk assessment, and natural resource management (Hasan et al., 2020; Issa et al., 2020a). These maps support informed decision-making by analyzing the spatial distribution and dynamics of LULC classes (Wang et al., 2023). LULC mapping is also significant for monitoring temporal and spatial variations among LULC classes driven by natural or anthropogenic factors (Jain et al., 2021). For instance, it can detect conversions of forests and barren lands to urban areas, track expansion of agricultural land, and observe fluctuations in water bodies. Such monitoring is crucial in rapidly developing regions like the Gulf, which experiences some of the highest rates of urbanization in the world. Urbanization, a global trend impacting all regions, often results in economic challenges, climate variability, and environmental degradation (Almulhim et al., 2022). In the Gulf region, including Saudi Arabia, Kuwait, Qatar, Bahrain, Oman, and the UAE, rapid urbanization fueled by oil exploration has driven a high demand for skilled foreign workers. By 1985, the foreign labor population reached 4.4 million, and by 2010, expatriates outnumbered nationals in Kuwait, Qatar, and the UAE, presenting unique demands on infrastructure and land use (Ramadan, 2015). Planned urban growth is therefore essential to managing these pressures, addressing environmental risks, and sustaining economic growth in the Gulf. Among the Gulf nations, the UAE and Qatar are particularly notable for reaching GDP levels comparable to developed countries, ranking in the top thirty in the World Competitiveness Report 2019 (Schwab, 2019). Saudi Arabia’s Vision 2030 also targets economic diversification and tourism promotion (Khan and Iqbal, 2020). The UAE, with one of the most diversified economies among oil-reliant Arab nations, exemplifies this trend. Drawing on the “Dubai model” inspired by Singapore, the UAE has aimed to attract foreign businesses and establish itself as a regional trade hub. Most other Gulf countries are also reshaping their economies in patterns similar to the Dubai Model (Henderson, 2007).
In the United Arab Emirates (UAE), diverse land cover types include expansive deserts, coastal salt flats (sabkha), urban areas, agricultural land, and water bodies. Understanding the distribution and extent of these land cover types is vital for addressing land use planning and resource management challenges, especially in such a demanding, arid environment (Burt et al., 2023). Multitemporal LULC maps can reveal urbanization patterns to guide planned urban growth (Wang et al., 2020), identify biodiversity hotspots to help preserve unique flora and fauna (Tourenq and Launay, 2008), and assist in planning efficient water use by exploring the potential for non-conventional water resources (Valjarević et al., 2021) for sustainable agriculture and food security (Shahin and Salem, 2015).
Satellite imagery is a valuable source of information for LULC mapping, offering cost-efficiency and broad coverage over long periods (Chen et al., 2021). This makes it particularly useful for studying and mapping LULC classes at different time stations (Gómez et al., 2016). Remotely sensed data is available in various resolutions, from coarse to fine, each suited to different research needs. For regional or local studies, high spatial resolution data, such as GeoEye-1 and Quickbird, may be ideal but often require purchase from commercial providers or specialized access programs. Medium-resolution data, however, provides a valuable balance between spatial detail and accessibility, making it widely used in remote sensing (RS) applications for LULC studies (Macarringue et al., 2022). Among medium-resolution data sources, the Landsat and Sentinel-2 satellites are particularly notable. The Landsat program, a collaboration between NASA and the United States Geological Survey (USGS), has been collecting data since 1972, making it one of the most comprehensive archives for Earth observation. Each Landsat mission has progressively enhanced its imaging capabilities. The early Multispectral Scanner System (MSS) on Landsat 1–5 had an 80 m spatial resolution with 6-bit radiometric depth across four spectral bands (Goward et al., 2022). However, Landsat 7 introduced a 15 m panchromatic band, and more recent missions (Landsat 8 and 9) feature advanced sensors like the Operational Land Imager (OLI) and the Thermal Infrared Sensor (TIRS), which provide higher spectral and radiometric resolutions (12–14 bits) and enhanced spatial accuracy (Goward et al., 2022). This long historical archive makes Landsat particularly valuable for temporal LULC analyses.
The European Union’s Copernicus Program, through its Sentinel-2 constellation (Sentinel-2A and Sentinel-2B), also offers a robust source of medium-resolution data. Launched in 2015, Sentinel-2 captures imagery at a spatial resolution of up to 10 m across 13 spectral bands, with a revisit frequency of 5 days (ESA, 2022). This high temporal resolution is particularly beneficial for contemporary monitoring, although it lacks the historical depth of Landsat. Combining Sentinel-2’s high revisit frequency with Landsat’s extensive temporal archive can provide a comprehensive dataset for monitoring and analyzing LULC changes.
Classifying RS data is challenging, prompting the application of various methods that range from pixel-based to object-oriented classification methods. In recent years, machine learning (ML) classifiers have gained popularity due to their ability to handle high-dimensional data and map complex LULC classes (Dahy et al., 2021). In recent years, machine learning (ML) classifiers have gained huge popularity as an alternative to traditional methods (Aryal et al., 2023). ML is a subtype of artificial intelligence that operates on the fundamental concept of computer systems to make informed decisions with reduced human involvement. ML is known for its reliability in handling high dimensional data and accurately mapping intricate LULC classes. Method based on the idea that computer systems can learn from data to identify patterns and make decisions with minimal human intervention. The strengths of ML include the capacity to handle data of high dimensionality and to map LULC classes with very complex characteristics. There are three main types of ML methods for LULC mapping: supervised learning, unsupervised learning, and semi-supervised learning (Zhao et al., 2023). Supervised algorithms are trained using tagged pixel samples and apply what they have learned to predict the labels of new, unlabeled LULC data (Boori et al., 2018). They use techniques like regression and gradient boosting to predict labels for unlabeled LULC data (Ait Naceur et al., 2024). Supervised algorithms, like random forest (RF) (Adam et al., 2014; Thakur and Panse, 2022), support vector machine (SVM) (Cardoso-Fernandes et al., 2020; Thakur and Panse, 2022), k-nearest neighbor (kNN) (Kalpana and Nandhagopal, 2021; Thakur and Panse, 2022), and the artificial neural network (ANN) (Alshari et al., 2023; Baig et al., 2022) use tagged pixel samples to predict the labels of new, unlabelled LULC data (Khatami et al., 2016; Talukdar et al., 2020). Despite their success in LULC mapping in the UAE, ML classifiers face challenges like atmospheric conditions and spectral confusion (Rogan and Chen, 2004), particularly in desert areas, complicating the differentiation of LULC classes (Stefanov et al., 2001).
The literature features numerous ML algorithms, each with its own strengths and weaknesses. What may work well for one environment or application may not suit others (Sarker, 2021). ML algorithms, like RF and SVM, have also been used for LULC classification and mapping in the UAE (Dahy et al., 2022). Despite the growing interest in LULC classification and mapping using satellite imagery in the UAE, there are still some challenges that need to be addressed. For example, the accuracy of LULC maps can be affected by various factors, such as atmospheric conditions, sensor calibration, and image resolution (Rogan and Chen, 2004). Additionally, spectral confusion of various materials, particularly in desert areas, can make it difficult to distinguish between different LULC classes (Stefanov et al., 2001).
To overcome these challenges, researchers have proposed various approaches, including combining multiple satellite data sources, using spectral indices, and incorporating ancillary data like topographic information and soil data (Mulder et al., 2011). Spectral indices expand training data and improve the classification algorithm’s effectiveness (Deus, 2016; Ghosh and Behera, 2018; Prasad et al., 2022). For instance, indices like the Normalized Difference Built-up Index (NDBI) and Dry Bare-Soil Index (DBSI) are particularly useful for distinguishing LULC classes prone to misclassification, such as built-up areas and desert regions, by addressing their spectral similarities. Additionally, the Normalized Difference Vegetation Index (NDVI) was employed to enhance the identification of vegetation and distinguish it from other land cover types. Similarly, the Normalized Difference Water Index (NDWI) was used to differentiate water bodies from built-up areas and vegetation. These indices, along with other spectral and topographic features, helped refine the model’s ability to classify complex landscapes with high accuracy. Despite challenges, satellite based LULC classification in the UAE offers valuable information for land management, conservation, and climate change monitoring. In the present research, various supervised ML classifiers were assessed, selecting the RF classifier for its superior performance, achieving 95% accuracy and a kappa coefficient of 0.94, consistent with previous studies (Adam et al., 2014; Dahy et al., 2022; Faheem et al., 2024; Thakur and Panse, 2022). The study offers a novel approach through the first long-term analysis in the arid UAE, spanning half a century, capturing key anthropogenic activities from independence, including the 1970s oil boom and the subsequent development of megaprojects at the onset of the new millennium. Our goal is to produce accurate LULC maps, providing insights into land use distribution patterns and identifying urbanization, agricultural, and water distribution hotspots. These maps serve as crucial tools for land registration, urban planning, and environmental management, supporting informed decision-making and promoting sustainable development in the UAE.
The UAE, located in the southeast of the Arabian Peninsula, shares borders with Oman and Saudi Arabia. It boasts an 800 km coastline along the Arabian Gulf and the Gulf of Oman, covering a land area of 83,600 km2. Positioned between 24° 08′ and 26° 04′ N latitude and 51° 35′ and 56° 22′ E longitude (Figure 1). In 2017, the UAE had a total population of 9,304,277, as reported by the Federal Competitiveness and Statistics Authority.
The UAE experiences mild winters and hot, humid summers. Summer temperatures reach an average of 41°C, while the average winter temperature falls up to an average of 21°C (Salameh and Touqan, 2023) Coastal cities of Dubai and Abu Dhabi experience high humidity. The region’s climate, characterized by high temperatures and low precipitation, contributes to its arid nature. This arid climate results in limited freshwater resources and a heavy reliance on desalination for water supply (Bolleter et al., 2021). The topography features mountainous terrain and barren deserts with loose sand and gravel and elevation ranging from 0 to 200 m. Geomorphology features sand dunes, inter-dunal sands, coastal sabkhas, inland sabkhas and exposed rocks (Glennie, 2001). Natural resources include petroleum, natural gas, and marine resources. Main natural hazards are dust storms and common sandstorms. Critical environmental challenges arise from limited freshwater resources, necessitating large-scale desalination facilities. Land conversions, driven by urban expansion, infrastructure development, and desert greening supported by oil revenues, have transformed the UAE into an urbanized state. Numerous modern advancements, including megaprojects, entail reclaiming land from the sea and modifying the LULC especially in the coastal areas (Subraelu et al., 2022). Local authorities have invested in large-scale greening projects, resulting in over 540 afforested areas covering 2.42 km2 by 2017, primarily planted with date palms (Al-Yamani et al., 2019).
For this study, a total of 72 Landsat images were selected based on data availability, image quality, and land cloud cover of less than 10%. These Landsat scenes represent the years 2021, 2017, 2013, 2002, 1997, 1992, 1986, and 1972, totalling eight datasets (Table 1). The Collection 2 and Level 2 (C2, L2) product of Landsat-8 OLI, Landsat-7 ETM+, and Landsat-5 TM images were used; while for Landsat-1 MSS images, the (C2, L1) products were used. All datasets were downloaded in GeoTIFF format from the USGS website (Earth Explorer, 2022).
In addition, 36 panchromatic band scenes (C2, L1) were used for the years 2021, 2017, 2013, and 2017 to sharpen the 30-m multispectral bands to 15 m. Since panchromatic bands were unavailable for the MSS images and TM imagery prior to 1999, these images were resampled to maintain consistent spatial resolution. The study further utilized a Shapefile of the UAE boundary, which includes maritime borders, and a 30 m spatial resolution Digital Elevation Model (DEM) – ALOS DSM covering the entire UAE. The processing tasks were conducted using ERDAS Imagine 2020 software package, and the resulting images were saved in (*.img) format of ERDAS.
Our study involves different steps leading to the creation of eight high-quality LULC maps. Based on the visual investigation of the eight colour composite images created (see 2.3.1 and Supplementary Appendix A), knowledge of the researchers, and previous studies, it was determined that our study area has four major LULC classes: desert and mountainous areas, built areas, vegetation, and water. Although the number of classes could be increased for a deeper understanding (e.g., distinguishing between sand and sabkha, or different types of vegetation-like palms, dates, and agriculture); however, it was decided, at this stage of the research, to focus on these four major classes for producing significant LULC maps of the study area. The methodological processes used in this study from data preparation to analysis are presented in Figure 2, while the details of these processes are given in the subsequent sections.
The acquired datasets underwent three pre-processing procedures: stacking, pan-sharpening, and resampling. Firstly, stacking involved the selection and combination of four equivalent bands, namely, green, red, near infrared (NIR), and shortwave infrared 1 (SWIR1) for each year from 1986 (TM) to 2021 (OLI). For the year 1972 (MSS), the stacked bands were green, red, NIR1, and NIR2. While relying on Landsat’s 30-m resolution for consistency, pan-sharpening was used to achieve 15-m resolution for selected years. Pan-sharpening was conducted by merging the panchromatic band (band 8 at 15-m resolution) and the stacked multispectral images using the hyperspectral color space (HCS) resolution merge technique and the nearest neighbourhood (NN) algorithm (Padwick et al., 2010). This procedure was only applied to OLI and ETM+ scenes from 2002, 2013, 2017, and 2021 to produce an enhanced image at 15 m spatial resolution. Finally, resampling involved the use of the NN algorithm to resample all scenes to a final pixel size of 15m × 15 m for consistency across all years, including 1997 (TM), 1992 (TM), 1986 (TM), and 1972 (MSS). After pan-sharpening and resampling, the scenes for each year were merged to create mosaics covering the entire study area (Supplementary Appendix A). Visual interpretation of these mosaics enabled the identification of LULC classes for the study area (see 2.3).
To match the four main classes identified in the study (built areas, vegetation, water, and desert and mountains), four layers of indices were generated for each scene. These indices include the Normalized Difference Vegetation Index (NDVI) (Equation 1) simplifies complex spectral information by creating a single band using the normalized ratio of near-infrared (NIR) and red-sensitive bands of the electromagnetic spectrum. NDVI is particularly effective in distinguishing healthy vegetation from stressed vegetation and differentiating vegetation from other land cover classes (Huang et al., 2021). The Normalized Difference Built-up Index (NDBI) (Equation 2) highlights urban and built-up areas by using the difference between the SWIR1 and NIR bands (He et al., 2010). The Modified Normalized Difference Water Index (MNDWI) (Equation 3) enhances the detection of water bodies by suppressing noise from built-up and bare-soil areas. Unlike the original NDWI, MNDWI uses the green and shortwave infrared (SWIR1) bands instead of the NIR band, making it more effective in urban and arid regions (Wang et al., 2018), and the Dry bare-soil index (DBSI) (Equation 4) differentiates barren land from other classes by emphasizing the spectral characteristics of dry soil and sand, incorporating both the SWIR1 and green bands (Rasul et al., 2018).
For each image, the derived indices’ layers, and the DEM were added and stacked with their corresponding multispectral image bands. This resulted in nine raster layers for each year’s dataset: green, red, NIR, SWIR1, NDVI, NDBI, MNDWI, DBSI, and DEM (except for MSS 1972, which had six raster layers: green, red, NIR1, NIR2, DEM, and NDVI). These time-series (TS) images were used as input to train the datasets and generate the relevant attributes.
Each image (72 images) was classified into four classes: built areas, vegetation, water, and desert and mountainous areas. A full description of each class is highlighted in Table 2 below.
Careful consideration was given to the selection and collection of extensive, representative training sets in the form of polygons to accurately represent the four LULC classes. The number of samples per scene varied as function of the abundance of the four main classes in the scene as well as the percentage of the scene within the boundary of the UAE. Thorough cleaning of the training data was conducted to remove errors and outliers, resulting in a total of 46,146 polygons (Figure 3). The training sets were then divided into two groups: training samples (80%, equal to 36,900 samples) to classify the images and testing samples (20%, equal to 9,264 samples) to be used in the accuracy assessment stage (see 2.3.7 and Supplementary Appendix B). The geostatistical Analyst Tool in ArcGIS was utilized to randomly split the samples. Lastly, the testing sample polygons were converted to points by creating polygon centroids using the Data Management Tools in ArcGIS.
To extract the object aspect (shape attribute) of the classes, “compactness” layers were generated in the vector data model and added to the datasets prior to conducting the classification. The compactness layer represents the ratio of the perimeter of a circle whose area is equal to that of the feature, to the perimeter of the feature. By incorporating this aspect into our datasets prior to conducting the classification, we aimed to improve the discrimination between built areas and desert regions. This resulted in ten layers used for the classification process, with seven layers used for the 1972 MSS datasets. These layers were used to extract four relevant attributes: spectral, spatial (textural), topographic (elevation), and object aspects (compactness).
In this study, the Classification and Regression Trees (CART), SVM, and RF classifiers were tested. Ultimately, the RF classifier was chosen for its higher accuracy and simpler implementation (Issa and Sultan, 2024). The initial step involved using the training samples to extract spectral (mean of Digital Number (DN) value), spatial (variance of DN value), topographic (elevation), and object aspect (compactness) attributes, which were then saved as shapefiles. The RF algorithm was then trained on this data to create the machine intellect before generalizing and performing the actual classification. The final step involved conducting the actual classification to produce thematic maps with the four defined classes. The spatial model used for the classification was developed using the ERDAS Imagine Spatial Modeler tool. Finally, the encoding depth of each thematic map was reduced from 64 bit to 4 bit to facilitate further processing steps such as accuracy assessment, mosaicking, and subset analysis.
After performing RF classification on each scene, we conducted individual accuracy assessments for the scene. For this purpose, a confusion matrix, using testing samples (see 2.3.4) was created, allowing us to visualize the performance of the classification algorithm by comparing predicted classes against actual classes (Foody, 2002). Next, we created separate masks for each class; by doing so, it became easier to identify and address the misclassified pixels within that specific class. This step allows for a more targeted and efficient manual correction process, as the focus is narrowed down to one class at a time. The key factors contributing to misclassifications were identified as spectral similarities between built areas and desert, as well as between hill shade and water class, leading to inaccuracies in the classification results. Subsequently, overall LULC maps were generated for all the selected years based on the classification outcomes. To create these maps, the classified scenes generated for each year were mosaicked and cropped with the UAE land borders, excluding the sea, to show the LULC classes within the land borders.
Eight thematic maps spanning 50 years, from 1972 to 2021, covering the entire UAE mainland has been generated (Figure 4). These TS maps correspond to the years 1972, 1986, 1992, 1997, 2002, 2013, 2017, and 2021, illustrating the spatial distribution of key LULC classes: built areas, vegetation, water, and desert and mountainous areas. These maps provide a visual representation of LULC patterns in the region, which can be valuable for applications such as informed spatial planning projects.
Accuracy assessment is a crucial step in LULC classification to validate results. A detailed description of accuracy results, including the overall accuracy and Kappa statistic for Landsat images across the study area over the years, is presented in Supplementary Appendix C. The results show high overall accuracy percentages ranging from 88.11% to 98.46% and corresponding Kappa statistic ranging from 0.24 to 0.98, indicating the reliability of the classification outcomes. Highest overall accuracy and kappa statistic were observed in 2021 while lowest in 1972.
Visual interpretation of LULC maps displays desert and mountainous areas as the most prevalent LULC throughout the study period. The maps also reveal significant spread of built areas in the emirates of Abu Dhabi, Dubai, Ajman, and Sharjah due to economic opportunities and land development. Green areas have expanded in Dubai and Abu Dhabi, reflecting urban greening initiatives and agricultural reforms. For instance, in Al Awir, Dubai, a project was implemented to reduce water consumption by up to 70% during cucumber production (Al-Qaydi, 2016). Similarly, in Al Dahara, Abu Dhabi drip irrigation system introduced, which decreased the water usage by 75% and fertilizer application by 50% compared to previous practices (Al-Qaydi, 2016). The water class shows a decline in coastal areas resulting from coastal development and megaprojects.
The charts presented in Figure 5 give a glance at the distribution of LULC classes over the study period (1972–2021), showing the land cover by class in the mapped years. The data reveals that desert and mountainous areas have consistently covered more than 90% of the area, although there has been a persistent decline in this LULC class over the years. Concurrently, the built area has shown continuous growth, along with the vegetation class. However, the water class, which has remained consistently low at less than 1% throughout the study period, is experiencing a reduction over time.
Despite being the largest class, desert and mountainous areas have shown significant variations throughout the study period. Initially covering nearly 97.81% of the total area in 1972, the distribution has decreased to 97.13%, 96.48%, 95.30%, 93.46%, 92.14%, 91.53%, and 91.06% in 1986, 1992, 1997, 2002, 2013, 2017, and 2021 respectively. The conversion of desert areas into built areas and vegetation is rapid primarily due to the availability of land; typically, these conversions do not significantly harm the desert ecosystem. However, threats such as excessive grazing lead to loss of desert rangelands, soil erosion and compaction in certain regions of the country (Abed and Hellyer, 2001; El-Keblawy and Alsharhan, 2003). The conversion of deserts to built areas is a common trend among Gulf countries, as seen in Saudi Arabia’s Riyadh (Alshammari et al., 2019) and Ibri in Oman (Mansour et al., 2022), where the built environment has expanded at the cost of desert, barren soil, and vegetation, raising concerns over water resource depletion and environmental degradation. Excessive urbanization also leads to the urban heat island phenomenon, observed in cities like Dubai. Conversely, Al Ain, known as a garden city in the UAE, maintains green spaces and parks to mitigate urban heat. However, the villa-style housing, unlike taller buildings in other urban centers, along with the city’s agricultural demands, has led to high water consumption and significant groundwater depletion (Mohamed et al., 2018).
The initial force behind the conversion of the desert into other land covers and subsequent development began with the discovery of oil reserves, which opened the doors for economic opportunities and work. This was later followed by economic diversification policies and the need to accommodate a rising population through large numbers of immigrants, along with their food, water, and living needs (M. Khan, 1981; Shihab, 2001).
The second most dominant class is the built area, which initially occupied only 0.97% of the total area within the UAE’s land borders in 1972, reaching 1.35% in 1986, 1.69% in 1992, 2.57% in 1997, 3.35% in 2002, 4.78% in 2013, 5.46% in 2017, and finally 5.8% in 2021. The distribution of built areas has varied over the years. The remarkable pace of development is attributed to oil revenue, providing significant financial resources for infrastructure and urbanization, making UAE one of the fastest growing nations in mid 20th century (Shihab, 2001). This financial influx has enabled the country to not only keep pace with but also outstrip the development seen in many other nations. Urban growth, driven by planned activities, was evident from 1972 to 1986, reflecting the early stages of development and infrastructure expansion to accommodate a growing population. By 1975, this percentage had risen to 69.5 percent, the highest among the GCC countries (Winckler, 1997). Between 1986 and 1992, the UAE faced a decline in oil revenues due to the fall in oil prices and the Gulf War, which affected its economic growth and development plans. The UAE had to diversify its economy and reduce its dependence on oil (Winckler, 1997). The creation of free zones, designated economic zones with relaxed administrative requirements and diminished conventional trade impediments, has been another major driver of rapid development in the UAE. One prominent example is Jebel Ali, which was established in the 1980s and expanded in 1996 (Shayah and Qifeng, 2015). At the beginning of the new millennium, notable mega-projects in Dubai, including the “Palm Islands” and “World Islands,” alongside the development of waterfront cities, rose. The major development projects of the UAE like construction and opening of Burj Khalifa, Dubai, in 2010, also led to extensive development projects in the surroundings too. The mega cities of the UAE have been a source of attraction to economic opportunity seekers and tourists, such Al Maryah Central, the Expo 2020 Dubai, the Museum of the Future, and the Al Qana.
The extensive coverage of desert and mountainous areas has made it challenging to observe and visualize the distribution of other classes. Therefore, specific hotspots (Figure 6) within the study area have been selected, as example, to illustrate the distribution of different LULC classes over the years.
Figure 6. Distribution of LULC classes at key locations, including (A) Dubai City, (B) the Liwa area, and (C) Al Wathba Lake and their surroundings. The subfigures correspond to subsequent years.
A closer view of multitemporal maps shows that the distribution of built areas in Dubai and Abu Dhabi becomes more prominent over the years. For example, Dubai’s increase in built area distribution has been fuelled by the political decision aimed at economic diversification through real estate investments, recognising finite nature of oil reserves. Unlike other cities, Dubai has not encountered constraints related to physical boundaries or land ownership issues facilitating unrestricted urban growth (Mishrif and Kapetanovic, 2018). This unique combination of factors makes Dubai an intriguing and significant location for studying the distribution of built area over the mapping period, Figure 6A shows the selected areas of Dubai city over the years.
Despite covering a small area, vegetation is considered a prominent LULC class in the UAE. The area covered by vegetation was 0.7% in 1972, 1.1% in 1986, 1.51% in 1992, 1.78% in 1997, 2.81% in 2002, 2.76% in 2013, 2.66% in 2017, and ultimately reached 2.85% in 2021. While the vegetation class shows an overall rise in its distribution throughout the study area, some fluctuations occurred in certain years, mainly caused by the seasonal fluctuations in some vegetation classes. Since its inception, the UAE has embarked on a journey to enhance greenery. The rise in vegetation cover from 1972 to 1986 can be attributed to governmental initiatives promoting agriculture and forestry, including land allocation for vegetation and camping, and the provision of incentives (Khan, 1981). With its early development and economic growth, the green areas of the UAE started facing pressure mainly because of the increasing number of grazing animals, vehicle disturbance, camping, beside other factors. Hence threatening the rangeland. To deal with such issues, the Five-Year National Plan (1981–85) included desertification control and resource management (Khan, 1981). This led to a visible increase in vegetation for the Baynunah rangeland in Abu Dhabi (Oatham et al., 1995). Additionally, the UAE’s participation in international environmental agreements, such as the 1989 Vienna Convention and the Montreal Protocol, underscores its commitment to environmental conservation (Cooperation, 2020).
Sheikh Zayed bin Sultan Al Nahyan, the founder of UAE, was known for his strong connection with the people, as reflected in his policies, including granting three parcels of land to each citizen for residential, commercial, and industrial purposes. His vision extended beyond economic growth; during his reign over Al Ain city in 1946, he established an irrigation system and emphasized the creation of large green spaces within cities (Hashim, 2018). Recognizing the need to protect the environment and enhance dependence on local food, the UAE authorities have implemented programs like establishing of the Federal Environmental Agency in 1993 and creating several protected areas and wildlife reserves. Success in mangrove planting initiatives has been notable despite previous reduction due to clearance and disease (MOCCAE, 2013). The increase in vegetation area in the UAE between 1997 and 2004 can be attributed to the establishment of the Dubai Desert Conservation Reserve (DDCR) and the expansion of urban greenery projects (Khafaga and Officer, 2009). The DDCR aims to restore the desert ecosystem, while urban greenery projects enhance the quality of urban areas. Both initiatives contribute to vegetation increase and natural resources conservation. Amid global food security concerns, UAE’s agricultural limitations prompt focus on imports and local farming to address growing population needs (Al-Qaydi, 2016; Sadiku et al., 2024). Besides enhancing aesthetics, the overall increase in country’s vegetation cover aids biodiversity conservation, soil protection, and climate regulation. Vegetation plays a crucial role in supporting ecosystem functions including carbon capture, water regulation, and habitat provision (Issa et al., 2020b; Issa and Saleous, 2014).
Despite being a predominantly arid region, the UAE has notable vegetation hotspots, such as the Liwa Oasis. The Liwa Oasis is shaped like a crescent and sits on top of a freshwater hill at the edge of the Empty Quarter. It is one of the two shallow and high-quality groundwater systems in Abu Dhabi Emirate. The area surrounding the oasis is the main farmland of Abu Dhabi’s Western Region (Fragaszy and McDonnell, 2016). Over the years, vegetation cover has increased gradually in the Liwa Oasis. However, this growth raises concerns about groundwater usage, as intensive farming and forest plantation programs may strain existing water resources. Figure 6B shows the temporal distribution of LULC classes in the Liwa area and its surroundings. To ensure a balance between local needs for food, economy, and culture, and the preservation of the delicate natural resources, careful planning and management are necessary to protect and sustain the groundwater in Liwa.
Given the arid nature of the region, water coverage in the UAE, coastal and inland waters within the land borders, is relatively limited. The initial coverage recorded in 1972 was 0.42%, which fluctuated over the year: 0.41% in 1986, 0.32% in 1992, 0.35% in 1997, 0.38% in 2002, 0.32% in 2012, 0.35% in 2017, and 0.30% in 2021, reflecting a mixed trend. These fluctuations can be attributed to factors such as land reclamation along the coast and the emergence of artificial lakes. Some minor increases in water coverage have been observed in years with heavy rainfall and flooding events such as in 1997 (Yagoub and Al Yammahi, 2022). The UAE’s coastal landscape shows both expansion and retreat due to ongoing reclamation projects and natural processes (Subraelu et al., 2022). assessed three decades of coastal dynamics, observing retreat in Ajman and Umm Al Quwain, while other emirates show extensive coastal reclamation. Overall, this contributes to a gradual decline in the UAE’s natural coastline. Changes in coastal waters are associated with the construction of new islands such as Palm Jumeirah, which is an artificial island in the shape of a palm tree, completed in 2006. The development of coastal cities and land reclamation projects have led to the loss of coastal waters within the land borders of the UAE (Dahy et al., 2024; Sherif et al., 2023).
The inland water resources in the UAE are very small in number and their replenishment depends on flash floods from heavy rains (Sherif et al., 2023). While the UAE depends on rain for both surface and groundwater recharge, the scarcity and irregularity of rainfall pose significant challenges to water availability and sustainability (Murad et al., 2007). In response, the UAE has prioritized both conventional and unconventional water sources to support sustainability. Unconventional sources like dew harvesting, for instance, are being explored as alternatives to supplement the water supply (Valjarević et al., 2021). assessed potential sites in the UAE’s mountainous regions where dew collectors could capture water for agriculture, offering a limited yet innovative source of water for arid areas.
Considering the alarming water scarcity, the government of the UAE has initiated multiple projects to ensure optimal use and reduce water losses during irrigation. Farmers are being trained in this respect. Besides, considerable investment has been made in desalination projects to meet the rising water requirements (Murad et al., 2007).
Additionally, artificial lakes, such as Al Wathba Lake, Zakher Lake, and Al Qudra Lake, have been created in the UAE. Figure 6C shows the LULC distribution of Al Wathba lakes and its surroundings over the years. Al Wathba Lake, a watershed area which was seen dry during the initial years of mapping, while in later years, multiple small lakes were created, which provide a home to various species of birds. Due to its ecological significance, Al Wathba Lake was declared the first Ramsar site of the UAE (Saji et al., 2018). These lakes not only serve as recreational areas but also provide habitats for various bird species. These lakes are recharged from treated wastewater and desalinated water. Through these approaches, the UAE is moving towards achieving sustainable development and improving natural habitats (Saji et al., 2018).
It has been observed that the UAE has desert and mountainous areas as prevalent land cover, which naturally hinders vegetation growth and lacks water resources. Despite rapid urbanization, land degradation, is primarily caused by over grazing and salinity from excessive groundwater drilling (Abdelfattah, 2009). While the expansion of built areas signifies economic progress, it also presents risks to biodiversity, natural habitats, and exacerbates the strain on already limited water resources. Conversely, the positive trend of vegetation growth offers benefits such as enhancing food security, reducing the reliance on food imports, and improving the overall environmental quality of the country. However, the rise in vegetation may introduce its own challenges, including increased water demand, degradation of groundwater resources, and soil quality issues. In terms of managing inland water resources, the UAE has been proactive in creating artificial lakes that are sustained with treated wastewater and seawater. Ensuring the sustainable management of these artificial lakes is paramount to preventing water pollution and upholding ecological equilibrium within the region. Overall, the study findings can contribute to planned development activities.
The study aimed to map the main LULC classes of the UAE since its independence in 1971, covering a fifty-year study period, using multi-temporal medium resolution Landsat satellite imagery. This comprehensive timeframe allowed us to capture and analyze the evolution of LULC patterns over nearly five decades. Our study covers all anthropogenic activities carried out over the study area, from the period of independence to significant events such as the discovery of oil reserves and major development projects.
The desert area has gradually decreased over time, although it remains the largest land cover class, covering more than 90% of the total area. This decrease in desert areas is accompanied by a simultaneous increase in the built areas, which is the second largest land cover class in the UAE. This rise in urbanization reflects the rapid development and transformation of urban centres in the country, driven by factors such as rapid economic growth led by the oil boom, attracting more migration, and the effect of globalization. Vegetation has consistently increased, reflecting the UAE’s commitment to greening, food security, and sustainable practices. Water bodies within the UAE’s land borders have fluctuated due to coastal reclamation, artificial island construction, and water conservation measures. These findings underscore the UAE’s balancing of development and environmental sustainability.
While the use of medium-resolution Landsat data posed limitations, it was the only freely available source spanning the entire study period, making it a crucial baseline for future research. Higher-resolution datasets, such as Sentinel-2, could further refine these findings.
The generated LULC maps serve as important documentation for various purposes, including land registration, inheritance, urban planning, and environmental management. They provide critical information for decision-makers and stakeholders, promoting informed decision-making and sustainable development practices in the UAE. The findings provide valuable information for policymakers and planners to effectively manage the country’s resources while balancing development with environmental sustainability. Continuous monitoring and conservation efforts are essential to ensure the long-term wellbeing of the UAE.
The original contributions presented in the study are included in the article/Supplementary Material, further inquiries can be directed to the corresponding author.
MuS: Formal Analysis, Investigation, Visualization, Writing–original draft. SI: Conceptualization, Project administration, Supervision, Writing–review and editing. BD: Investigation, Methodology, Writing–review and editing. NS: Supervision, Validation, Writing–review and editing. MaS: Supervision, Writing–review and editing.
The author(s) declare that financial support was received for the research, authorship, and/or publication of this article. This study was supported by the United Arab Emirates University through Grant Number 12S155.
The authors express their sincere gratitude to the United Arab Emirates University for financial support under Grant Number 12S155.
The authors declare that the research was conducted in the absence of any commercial or financial relationships that could be construed as a potential conflict of interest.
The author(s) declare that Generative AI was used in the creation of this manuscript. The author(s) verify and take full responsibility for the use of generative AI in the preparation of this manuscript. The authors declare that Grammarly and ChatGPT (free versions) were used to improve sentence structure and language clarity in the manuscript.
All claims expressed in this article are solely those of the authors and do not necessarily represent those of their affiliated organizations, or those of the publisher, the editors and the reviewers. Any product that may be evaluated in this article, or claim that may be made by its manufacturer, is not guaranteed or endorsed by the publisher.
The Supplementary Material for this article can be found online at: https://www.frontiersin.org/articles/10.3389/feart.2024.1510510/full#supplementary-material
Abdelfattah, M. A. (2009). Land degradation indicators and management options in the desert environment of Abu Dhabi, United Arab Emirates. Soil Surv. horizons 50 (1), 3–10. doi:10.2136/sh2009.1.0003
Adam, E., Mutanga, O., Odindi, J., and Abdel-Rahman, E. M. (2014). Land-use/cover classification in a heterogeneous coastal landscape using RapidEye imagery: evaluating the performance of random forest and support vector machines classifiers. Int. J. Remote Sens. 35 (10), 3440–3458. doi:10.1080/01431161.2014.903435
Ait Naceur, H., Abdo, H. G., Igmoullan, B., Namous, M., Alshehri, F., and A Albanai, J. (2024). Implementation of random forest, adaptive boosting, and gradient boosting decision trees algorithms for gully erosion susceptibility mapping using remote sensing and GIS. Environ. Earth Sci. 83 (3), 121. doi:10.1007/s12665-024-11424-5
Almulhim, A. I., Bibri, S. E., Sharifi, A., Ahmad, S., and Almatar, K. M. (2022). Emerging trends and knowledge structures of urbanization and environmental sustainability: a regional perspective. Sustainability 14 (20), 13195. doi:10.3390/su142013195
Al-Qaydi, S. (2016). The status and prospects for agriculture in the United Arab Emirates (UAE) and their potential to contribute to food security. J. Basic Appl. Sci. 12, 155–163. doi:10.6000/1927-5129.2016.12.23
Alshammari, T. (2019). Sustainable urbanisation in desert cities: case study Riyadh city. Sheffield, United Kingdom: University of Sheffield Ph.D. Thesis.
Alshari, E. A., Abdulkareem, M. B., and Gawali, B. W. (2023). Classification of land use/land cover using artificial intelligence (ANN-RF). Front. Artif. Intell. 5, 964279. doi:10.3389/frai.2022.964279
Al-Yamani, W., Kennedy, L., Green, S., Kemp, P., and Clothier, B. (2019). The historical basis and future options for native plant-species in the hyper-arid forests of Abu Dhabi. Land Use Policy 88, 104186. doi:10.1016/j.landusepol.2019.104186
Aryal, J., Sitaula, C., and Frery, A. C. (2023). Land use and land cover (LULC) performance modeling using machine learning algorithms: a case study of the city of Melbourne, Australia. Sci. Rep. 13 (1), 13510. doi:10.1038/s41598-023-40564-0
Baig, M. F., Mustafa, M. R. U., Baig, I., Takaijudin, H. B., and Zeshan, M. T. (2022). Assessment of land use land cover changes and future predictions using CA-ANN simulation for selangor, Malaysia. Water 14 (3), 402. doi:10.3390/w14030402
Bolleter, J., Grace, B., Hooper, P., and Foster, S. (2021). Wet-bulb temperature and sea-level rise in the United Arab Emirates–planning responses. Plan. Pract. Res. 36 (4), 408–429. doi:10.1080/02697459.2020.1859199
Boori, M., Paringer, R., Choudhary, K., and Kupriyanov, A. (2018). “Supervised and unsupervised classification for obtaining land use/cover classes from hyperspectral and multi-spectral imagery,” in Paper presented at the Sixth International Conference on Remote Sensing and Geoinformation of the Environment (RSCy2018), 4. doi:10.1117/12.2322624
Burt, J. A., Al-Mansoori, N., Bejarano, I., Brown, G., Campbell, O., Els, J., et al. (2023). “The emirates at 2050: balancing development and environmental stewardship,” in A natural history of the emirates (Springer), 735–748.
Cardoso-Fernandes, J., Teodoro, A. C., Lima, A., and Roda-Robles, E. (2020). Semi-automatization of support vector machines to map lithium (Li) bearing pegmatites. Remote Sens. 12 (14), 2319. doi:10.3390/rs12142319
Chen, B., Tu, Y., Song, Y., Theobald, D. M., Zhang, T., Ren, Z., et al. (2021). Mapping essential urban land use categories with open big data: results for five metropolitan areas in the United States of America. ISPRS J. Photogrammetry Remote Sens. 178, 203–218. doi:10.1016/j.isprsjprs.2021.06.010
Dahy, B., Al-Memari, M., Al-Gergawi, A., and Burt, J. A. (2024). Remote sensing of 50 years of coastal urbanization and environmental change in the Arabian Gulf: a systematic review. A Syst. Rev. 5, 1422910. doi:10.3389/frsen.2024.1422910
Dahy, B., Issa, S., and Saleous, N. (2021). “A review of land change modelling techniques using Remote sensing and GIS,” in Paper presented at the 42nd Asian Conference on Remote Sensing, ACRS 2021.
Dahy, B., Issa, S., and Saleous, N. (2022). Random forest for classifying and monitoring 50 Years of vegetation dynamics in three desert cities of the uae. Int. Archives Photogrammetry, Remote Sens. Spatial Inf. Sci. 43, 69–76. doi:10.5194/isprs-archives-xliii-b3-2022-69-2022
Deus, D. (2016). Integration of ALOS PALSAR and Landsat data for land cover and forest mapping in Northern Tanzania. Land 5 (4), 43. doi:10.3390/land5040043
Earth Explorer (2022). U.S. Geological Survey. Retrieved 2022, from earth explorer. Available at: https://earthexplorer.usgs.gov.
El-Keblawy, A., and Alsharhan, A. (2003) “Effects of protection from grazing on species diversity, abundance and productivity in two regions of Abu Dhabi, United Arab Emirates,”. Lisse, Netherlands: Swets Zeitlinger Publishers, 217–226. Desertification in the third millennium.
ESA (2022). Sentinel-1-missions-Sentinel online-Sentinel online. Eur. Sp. Agency. Available at: https://sentinel.esa.int/web/sentinel/missions/sentinel-1
Faheem, Z., Kazmi, J. H., Shaikh, S., Arshad, S., and Mohammed, S. J. E. I. (2024). Random forest-based analysis of land cover/land use LCLU dynamics associated with meteorological droughts in the desert ecosystem of Pakistan. Ecol. Indic. 159, 111670. doi:10.1016/j.ecolind.2024.111670
Foody, G. M. (2002). Status of land cover classification accuracy assessment. Remote Sens. Environ. 80 (1), 185–201. doi:10.1016/s0034-4257(01)00295-4
Fragaszy, S., and McDonnell, R. (2016). Oasis at a crossroads: agriculture and groundwater in Liwa, United Arab Emirates. IWMI project publication–Groundwater governance in the Arab World–Taking stock and addressing the challenges, USAID. IWMI.
Ghosh, S. M., and Behera, M. D. (2018). Aboveground biomass estimation using multi-sensor data synergy and machine learning algorithms in a dense tropical forest. Appl. Geogr. 96, 29–40. doi:10.1016/j.apgeog.2018.05.011
Glennie, K. W. (2001). Evolution of the Emirates’ land surface: an introduction. London: Trident Press Ltd, 9–27.
Gómez, C., White, J. C., and Wulder, M. A. (2016). Optical remotely sensed time series data for land cover classification: a review. ISPRS J. Photogrammetry Remote Sens. 116, 55–72. doi:10.1016/j.isprsjprs.2016.03.008
Goward, S. N., Williams, D. L., Arvidson, T., Rocchio, L. E., Irons, J. R., Russell, C. A., et al. (2022). Landsat's enduring legacy: pioneering global land observations from space photogrammetric engineering remote sensing. Photogramm. Eng. remote Sens. 88 (6), 357–358. doi:10.14358/pers.88.6.357
Hasan, S. S., Zhen, L., Miah, M. G., Ahamed, T., and Samie, A. (2020). Impact of land use change on ecosystem services: a review. Environ. Dev. 34, 100527. doi:10.1016/j.envdev.2020.100527
He, C., Shi, P., Xie, D., and Zhao, Y. (2010). Improving the normalized difference built-up index to map urban built-up areas using a semiautomatic segmentation approach. Remote Sens. Lett. 1 (4), 213–221. doi:10.1080/01431161.2010.481681
Henderson, J. C. (2007). Destination development: Singapore and Dubai compared. J. travel Tour. Mark. 20 (3-4), 33–45. doi:10.1300/j073v20n03_03
Huang, S., Tang, L., Hupy, J. P., Wang, Y., and Shao, G. (2021). A commentary review on the use of normalized difference vegetation index (NDVI) in the era of popular remote sensing. J. For. Res. 32 (1), 1–6. doi:10.1007/s11676-020-01155-1
Issa, S., Alshamsi, W., and Saleous, N. (2020a). “Analysis of urban growth and sprawl of Musaffah industrial zone (Abu Dhabi) since its establishment in 1984 until today: a remote sensing and geographical information systems-based study,” in Paper presented at the Fifth international conference on engineering geophysics (ICEG), 252–255. doi:10.1190/iceg2019-064.1
Issa, S., Dahy, B., Ksiksi, T., and Saleous, N. (2020b). A review of terrestrial carbon assessment methods using geo-spatial technologies with emphasis on arid lands. Remote Sens. 12 (12), 2008. doi:10.3390/rs12122008
Issa, S., and Saleous, N. (2014). Satellite image-based analysis of the greening impact on the formation of an urban Heat Island (UHI) in Abu Dhabi City. Arab World Geogr. 17 (1), 91–101. doi:10.5555/arwg.17.1.q540p55865h1jr04
Issa, S., and Sultan, M. (2024). “Land use and land cover mapping using Google Earth Engine: a comparative analysis of machine learning algorithms,” in Seventh International Conference on Engineering Geophysics, Al Ain, UAE, October 16–19 2023 (Houston, TX: Society of Exploration Geophysicists Houston), 147–151.
Jain, S., Roy, S. B., Panda, J., and Rath, S. S. (2021). Modeling of land-use and land-cover change impact on summertime near-surface temperature variability over the Delhi–Mumbai Industrial Corridor. Model. Earth Syst. Environ. Dev. 7, 1309–1319. doi:10.1007/s40808-020-00959-8
Kalpana, Y. B., and Nandhagopal, S. (2021). Lulc image classifications using k-means clustering and knn algorithm. Dyn. Syst. Appl. 30 (10), 1640–1652. doi:10.46719/dsa202130.10.07
Khafaga, T., and Officer, C. (2009). A comparative study of vegetation structure and regeneration between two monitoring surveys in the Dubai Desert Conservation Reserve: initial assessment and baseline data. DDCR Intern. Rep., 66.
Khan, M. (1981). Management, conservation and development of agricultural resources in the United Arab Emirates. Pak. J. For. 31 (4), 137–150.
Khan, M. B., and Iqbal, S. (2020). “Vision 2030 and the national transformation program,” in Research, innovation and entrepreneurship in Saudi Arabia (London, United Kingdom: Routledge), 146–166.
Khatami, R., Mountrakis, G., and Stehman, S. V. (2016). A meta-analysis of remote sensing research on supervised pixel-based land-cover image classification processes: general guidelines for practitioners and future research. Remote Sens. Environ. 177, 89–100. doi:10.1016/j.rse.2016.02.028
Macarringue, L. S., Bolfe, É. L., and Pereira, P. R. M. (2022). Developments in land use and land cover classification techniques in remote sensing: a review. J. Geogr. Inf. Syst. 14 (1), 1–28. doi:10.4236/jgis.2022.141001
Mansour, S., Alahmadi, M., Atkinson, P. M., and Dewan, A. (2022). Forecasting of built-up land expansion in a desert urban environment. Remote Sens. 14 (9), 2037. doi:10.3390/rs14092037
Mishrif, A., and Kapetanovic, H. (2018). Dubai’s model of economic diversification. Econ. Diversif. Gulf Region, Volume II Comp. Glob. Challenges, 89–111. doi:10.1007/978-981-10-5786-1_5
MOCCAE (2013). Mangrove resources of the United Arab Emirates. UAE Ministry of Climate Change and Environment: UAE.
Mohamed, E. A., Paleologos, E. K., Caratelli, P., and Mohamed, E. (2018). Are garden cities in the desert sustainable? the oasis city of Al Ain in the emirate of Abu Dhabi. Kanazawa, Japan: Kanazawa University Ph.D. Thesis.
Mulder, V., De Bruin, S., Schaepman, M. E., and Mayr, T. (2011). The use of remote sensing in soil and terrain mapping—a review. Geoderma 162 (1-2), 1–19. doi:10.1016/j.geoderma.2010.12.018
Murad, A. A., Al Nuaimi, H., and Al Hammadi, M. (2007). Comprehensive assessment of water resources in the United Arab Emirates (UAE). Water Resour. Manag. 21 (9), 1449–1463. doi:10.1007/s11269-006-9093-4
Oatham, M., Nicholls, M., and Swingland, I. (1995). Manipulation of vegetation communities on the Abu Dhabi rangelands. I. The effects of irrigation and release from longterm grazing. Biodivers. Conserv. 4, 696–709. doi:10.1007/bf00158863
Padwick, C., Deskevich, M., Pacifici, F., and Smallwood, S. (2010). “WorldView-2 pan-sharpening,” in Paper presented at the Proceedings of the ASPRS 2010 Annual Conference, San Diego, CA, USA.
Prasad, P., Loveson, V. J., Chandra, P., and Kotha, M. (2022). Evaluation and comparison of the earth observing sensors in land cover/land use studies using machine learning algorithms. Ecol. Inf. 68, 101522. doi:10.1016/j.ecoinf.2021.101522
Ramadan, E. (2015). Sustainable urbanization in the arabian Gulf region: problems and challenges. Arts Soc. Sci. J. 6 (2), 1–4. doi:10.4172/2151-6200.1000109
Rasul, A., Balzter, H., Ibrahim, G. R. F., Hameed, H. M., Wheeler, J., Adamu, B., et al. (2018). Applying built-up and bare-soil indices from Landsat 8 to cities in dry climates. Land 7 (3), 81. doi:10.3390/land7030081
Rogan, J., and Chen, D. (2004). Remote sensing technology for mapping and monitoring land-cover and land-use change. Prog. Plan. 61 (4), 301–325. doi:10.1016/s0305-9006(03)00066-7
Sadiku, M. N., Chukwu, U. C., and Sadiku, J. O. (2024). “Agriculture in United Arab Emirates,” in Paper presented at the International Conference on Agriculture Sciences (Morocco: Environment, Urban and Rural Development) 24–32.
Saji, A., Mischke, S., Soorae, P. S., Ahmed, S., and Al Dhaheri, S. (2018). The alwathba wetland reserve lake in Abu Dhabi, United Arab Emirates and its ostracod (seed shrimp) fauna. Int. J. Aquatic Biol. 6 (5), 265–273. doi:10.22034/ijab.v6i5.483
Salameh, M., and Touqan, B. (2023). From heritage to sustainability: the future of the past in the hot arid climate of the UAE. Buildings 13 (2), 418. doi:10.3390/buildings13020418
Sarker, I. H. (2021). Machine learning: algorithms, real-world applications and research directions. SN Comput. Sci. 2 (3), 160. doi:10.1007/s42979-021-00592-x
Shahin, S. M., and Salem, M. A. (2015). The challenges of water scarcity and the future of food security in the United Arab Emirates (UAE). Nat. Resour. Conserv. 3 (1), 1–6. doi:10.13189/nrc.2015.030101
Shayah, M. H., and Qifeng, Y. (2015). Development of free zones in United Arab Emirates. Int. Rev. Res. Emerg. Mark. Glob. Econ. 1 (2), 286–294.
Sherif, M., Liaqat, M. U., Baig, F., and Al-Rashed, M. (2023). Water resources availability, sustainability and challenges in the GCC countries: an overview. Heliyon 9, e20543. doi:10.1016/j.heliyon.2023.e20543
Shihab, M., Abed, I., and Hellyer, P. (2001). Economic development in the UAE. United Arab. Emir. a new Perspect. (London, United Kingdom: Trident Press Ltd.) 2, 249–258.
Stefanov, W. L., Ramsey, M. S., and Christensen, P. R. (2001). Monitoring urban land cover change: an expert system approach to land cover classification of semiarid to arid urban centers. Remote Sens. Environ. 77 (2), 173–185. doi:10.1016/s0034-4257(01)00204-8
Subraelu, P., Ebraheem, A. A., Sherif, M., Sefelnasr, A., Yagoub, M., and Rao, K. N. (2022). Land in water: the study of land reclamation and artificial islands formation in the uae coastal zone: a remote sensing and gis perspective. Land 11 (11), 2024. doi:10.3390/land11112024
Talukdar, S., Singha, P., Mahato, S., Pal, S., Liou, Y.-A., Rahman, A., et al. (2020). Land-use land-cover classification by machine learning classifiers for satellite observations—a review. Remote Sens. 12 (7), 1135. doi:10.3390/rs12071135
Thakur, R., and Panse, P. (2022). Classification performance of land use from multispectral remote sensing images using decision tree, K-nearest neighbor, random forest and support vector machine using EuroSAT data. Int. J. Intelligent Syst. Appl. Eng. 10 (1s), 67–77.
Tourenq, C., and Launay, F. (2008). Challenges facing biodiversity in the United Arab Emirates. Manag. Environ. Qual. An Int. J. 19 (3), 283–304. doi:10.1108/14777830810866428
Valjarević, A., Milanović, M., Valjarević, D., Basarin, B., Gribb, W., and Lukić, T. (2021). Geographical information systems and remote sensing methods in the estimation of potential dew volume and its utilization in the United Arab Emirates. Arabian J. Geosciences 14, 1430–1515. doi:10.1007/s12517-021-07771-3
Wang, S. W., Gebru, B. M., Lamchin, M., Kayastha, R. B., and Lee, W.-K. (2020). Land use and land cover change detection and prediction in the Kathmandu district of Nepal using remote sensing and GIS. Sustainability 12 (9), 3925. doi:10.3390/su12093925
Wang, Y., Sun, Y., Cao, X., Wang, Y., Zhang, W., and Cheng, X. (2023). A review of regional and Global scale Land Use/Land Cover (LULC) mapping products generated from satellite remote sensing. ISPRS J. Photogrammetry Remote Sens. 206, 311–334. doi:10.1016/j.isprsjprs.2023.11.014
Wang, Z., Liu, J., Li, J., and Zhang, D. D. (2018). Multi-spectral water index (MuWI): a native 10-m multi-spectral water index for accurate water mapping on Sentinel-2. Remote Sens. 10 (10), 1643. doi:10.3390/rs10101643
Winckler, O. (1997). The immigration policy of the Gulf Cooperation Council (GCC) states. Middle East. Stud. 33 (3), 480–493. doi:10.1080/00263209708701165
Yagoub, M., and Al Yammahi, A. A. (2022). Spatial distribution of natural hazards and their proximity to heritage sites: case of the United Arab Emirates. Int. J. Disaster Risk Reduct. 71, 102827. doi:10.1016/j.ijdrr.2022.102827
Keywords: remote sensing, multispectral, classification, random forest, accuracy assessment, arid environment, multitemporal, urban expansion
Citation: Sultan M, Issa S, Dahy B, Saleous N and Sami M (2024) Fifty years of land use and land cover mapping in the United Arab Emirates: a machine learning approach using Landsat satellite data. Front. Earth Sci. 12:1510510. doi: 10.3389/feart.2024.1510510
Received: 13 October 2024; Accepted: 25 November 2024;
Published: 11 December 2024.
Edited by:
Sheng Nie, Chinese Academy of Sciences (CAS), ChinaReviewed by:
Aleksandar Valjarević, University of Belgrade, SerbiaCopyright © 2024 Sultan, Issa, Dahy, Saleous and Sami. This is an open-access article distributed under the terms of the Creative Commons Attribution License (CC BY). The use, distribution or reproduction in other forums is permitted, provided the original author(s) and the copyright owner(s) are credited and that the original publication in this journal is cited, in accordance with accepted academic practice. No use, distribution or reproduction is permitted which does not comply with these terms.
*Correspondence: Nazmi Saleous, bmF6bWkuc2FsZW91c0B1YWV1LmFjLmFl
Disclaimer: All claims expressed in this article are solely those of the authors and do not necessarily represent those of their affiliated organizations, or those of the publisher, the editors and the reviewers. Any product that may be evaluated in this article or claim that may be made by its manufacturer is not guaranteed or endorsed by the publisher.
Research integrity at Frontiers
Learn more about the work of our research integrity team to safeguard the quality of each article we publish.