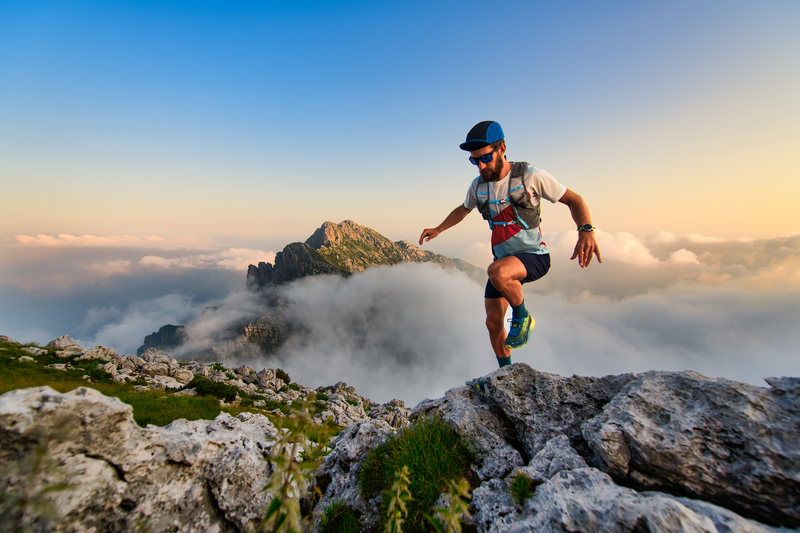
94% of researchers rate our articles as excellent or good
Learn more about the work of our research integrity team to safeguard the quality of each article we publish.
Find out more
ORIGINAL RESEARCH article
Front. Earth Sci. , 12 December 2024
Sec. Geoscience and Society
Volume 12 - 2024 | https://doi.org/10.3389/feart.2024.1490811
This article is part of the Research Topic Exploration, Development, and Protection of Earth’s Resources and Environment: Methods, Techniques, Applications, Prospects, Insights, and Problems View all 42 articles
Niobium, an irreplaceable raw material in high-tech industries, boasts a complex global trade network across its industrial chain. This study, leveraging 2022 trade data and inter-linkage dependencies within the niobium industry, constructs a multi-layer network model and simulates the impact and propagation of supply disruptions under two realistic scenarios: Restrictions on primary product exports and total export bans. Our findings reveal that: (1) Supply constraints on primary products cascade through the middle and downstream trade networks, impacting the final product layer more severely, especially for countries with limited ability to cope with disruptions. (2) China, Malaysia, Brazil, and the United States emerge as crucial risk sources, with their export restrictions potentially triggering a complete collapse of trade networks across all layers. (3) Hidden risk sources include Asian countries like Japan and Korea in the primary product layer, while European nations like the United Kingdom and Germany, despite playing a limited role in upstream disruptions, significantly influence the intermediate and final product layers. These findings underscore the importance of developing feasible systemic risk mitigation strategies, optimizing industrial structures, fostering global cooperation, and ultimately bolstering the robustness and resilience of the global niobium industry chain.
The global surge in high-tech industries has intensified international competition for critical raw materials, including niobium (Bigerna et al., 2021; David et al., 2022; Wickramaratne and Mazin, 2022). Niobium plays a crucial role in various high-tech applications, such as superconductors, superalloys, and advanced manufacturing (Zhou et al., 2024). Recognizing its strategic importance, many countries, including China, the United States and European Union have designated niobium as a critical mineral(Silveira and Resende, 2020; Williams-Jones and Vasyukova, 2023; Burton et al., 2024; Bhamra et al., 2024). However, despite growing demand, global niobium reserves are highly concentrated, with Brazil and Canada accounting for 98% (Zeng et al., 2024). This has resulted in a high import dependency for most countries, including a 100% reliance on net imports for China and the United States (McCaffrey et al., 2023). Consequently, ensuring a stable and secure supply of niobium has become a pressing concern, especially given the increasing demand from emerging technologies and rising geopolitical tensions. The niobium industry chain spans from primary products (refined ores, niobium oxides) to intermediate products (metals, alloys), and downstream final products (oil and gas pipelines, auto parts, infrastructure) (Gómez et al., 2024). The interconnected nature of this global trade network, coupled with various risks like economic fluctuations, supply disruptions, geopolitical events, and pandemics, creates potential for cascading failures. Disruptions affecting a single entity can rapidly spread across the trade network, exacerbated by the vertical dependencies within the industry chain (Hu et al., 2021a; Kalubanga and Gudergan, 2022). Even seemingly minor local shocks can potentially trigger widespread disruptions, increasing national vulnerabilities and deepening supply security concerns (Chen and Su, 2020; Li et al., 2021). Therefore, identifying potential risk sources within the niobium trade network is crucial for ensuring a stable and sustainable supply and further optimize the overall network structure.
Understanding the resilience of critical mineral supply chains is crucial for ensuring the sustainable development of high-tech industries. While previous studies have employed complex network theory to analyze supply chain risks in various sectors (Bier et al., 2020; Dong et al., 2020; Pacini et al., 2021), research specifically focusing on the niobium industry and its vulnerability to cascading failures within a multi-layered trade network remains limited. Existing studies on critical mineral supply chains often focus on single-layer network analysis (Chen C. Y et al., 2020; Zhu et al., 2022; Bridge and Faigen, 2022; Li et al., 2023a; Shuai et al., 2023), which may not fully capture the interconnectedness and cross-layer dependencies within complex industrial systems. Multi-layer complex networks offer a more comprehensive framework for analyzing supply chain risks compared to traditional single-layer network approaches. By incorporating multiple layers to represent different stages of the industry chain and their interdependencies, multi-layer networks can capture the cascading effects of disruptions across different levels of the system (Naqvi and Monasterolo, 2021; Wang et al., 2022a). This approach provides a more realistic and nuanced understanding of supply chain vulnerability and resilience. The industry chain itself is a complex multi-layer network structure, and the supply risk lurks in each industry chain link (McNulty and Jowitt, 2021). The analysis of multi-layer trade network from the perspective of mineral resources industry chain focuses on revealing the structural features of the network (Shao et al., 2022), such as (Wang et al., 2022a), who studies the characteristics of multi-layer trade network in the nickel industry chain, Kang et al. (2022) calculate the national competitiveness based on the study of the characteristics of the multi-layer network of the copper industrial chain, and Shi et al. (2022) reveals the key nodes in the multi-layer network of the global cobalt industrial chain. Although the analysis of static network structure features has important reference value for understanding the structural defects of trade networks (Wang et al., 2022), the impact of trade risk on individual or marginal countries is likely to affect the supply security of other countries (Hu et al., 2023; Yin et al., 2022), the impact propagation model of complex networks combined with cascade failure model and epidemic model takes into account the complex trade relations in networks (Hu et al., 2021b; Wang et al., 2021; Wang et al., 2020; Wang et al., 2022b), and quantifies the impact scale under different impact scenarios, it has an incomparable advantage in characterizing the impact of such systemic risk (Li et al., 2023b), which has been proved in the industrial chain trade network of nickel, copper, cobalt and other mineral resources (Kang et al., 2023; Li C et al., 2023; Sun et al., 2022).
While existing research has explored supply chain risks in various industries, including critical minerals (see Table 1 for a comparison with existing studies), a comprehensive analysis of the global niobium supply chain and its vulnerability to cascading disruptions within a multi-layered trade network remains limited. Specifically, previous studies have not fully addressed the cross-layer impacts of supply constraints across the upstream, midstream, and downstream segments of the niobium industry. To address these limitations, this study develops a multi-layered trade network model of the global niobium industry chain, explicitly considering the interdependencies between upstream, midstream, and downstream sectors. We then simulate the propagation of supply disruptions under two distinct scenarios to inform strategic planning and development efforts. The first scenario analyzes the impact of constraints on primary product supply, wherein a country’s capacity to provide refined ores and niobium oxides is restricted. This scenario investigates the vulnerability of the niobium supply chain to disruptions originating at the upstream level. The second scenario explores a more extreme circumstance: a complete disruption of a country’s ability to supply any niobium products across the entire industry chain. This allows us to evaluate the network’s resilience to more severe and widespread disruptions, providing insights for policies promoting diversification and redundancy within the supply chain. By analyzing these two scenarios, we gain a deeper understanding of the potential consequences arising from supply disruptions at different stages of the niobium value chain, ultimately contributing to the development of effective mitigation strategies.
The rest of this study is organized as follows. Section 2 provides the data and methods of this paper, including the construction of the network model for the niobium industry chain and the dynamic risk propagation model. Section 3 reveals the findings and discussions from three aspects: the overall impact of the supply crisis, key risk countries, and transmission pathways. Finally, Section 4 is the conclusion.
The whole life cycle of niobium includes four stages: mining, processing, consumption, and waste management. Based on the main products of each stage, the niobium industry chain is divided into three stages: upstream, middle, and downstream. Upstream products include primary products such as niobium ore and concentrate; midstream products mainly consist of intermediate products containing niobium; and downstream products represent the final consumer products made with niobium. Table 2 presents the six-digit HS codes for all relevant products, with niobium coefficients referenced from McCaffrey et al. (2023) and Zhou et al. (2024). We obtained the raw trade data for 2022 from the UN Comtrade database for all commodities related to the niobium industry chain, including trade value, trade volume and import/export data (in kilograms) for participating countries. For missing values in trade flows, there are no cases where both trade value and trade volume are missing. For cases where trade volume is missing, we sum up all the trade values and trade volumes in that year and divide them to obtain the average price. If the trade flow between two countries is missing, the trade value of that flow is divided by the average price to approximate the missing trade volume (Liu et al., 2022). Furthermore, we consolidated trade flows for mainland China with those of Hong Kong, Macao, and Taiwan, resulting in a dataset encompassing 231 major countries. Additionally, to address potential inconsistencies in statistical calibrations between importing and exporting countries, we utilized data from exporting countries (Gao et al., 2022).
To reflect the interconnections among the upstream, midstream, and downstream of the niobium supply chain, and further compare the robustness of each stage, this study constructs a global niobium industry chain network (GNICN) for 2022 based on the framework of multi-layer complex networks theory. As shown in Figure 1, the GNICN consists of three single-layer networks, denoted as L1, L2, L3, L1 representing the upstream primary product layer, L2 representing the intermediate product layer in the middle, and L3 representing the downstream final product layer. For example, the trade network formed by upstream primary products is a single-layer network
Based on constructing three single-layer networks, GNICN is constructed as
where
Building upon the multi-layer trade network of the niobium industry chain and the overload model, this study constructs a dynamic risk propagation model (Artime et al., 2024; Chen G et al., 2020). This model simulates a scenario where a country’s state shifts from normal to a state of avalanche when its risk level exceeds a predefined threshold. By simulating the impact of supply crises on each stage of the niobium industry chain under two realistic risk scenarios–primary product supply restriction and complete product supply obstruction–the model reveals hidden key risk sources and delineates the detailed pathways of risk propagation (Shao et al., 2022).
In the scenario of primary product supply constraints, all nodes within the multi-layer network are initialized to a normal state at iteration 0, and the initial load of nodes is set to
Figure 2. Cascade failure in primary niobium product for layer node interruption. (A) Lateral propagation of supply risk caused by wind source node Country A within L1 through affected partner nodes. (B) Vertical propagation of supply shortage risk from L1 along the industry chain along inter-layer supply relationships. (C) Supply risk originating from Country A has spread in the multi-layer network of the niobium industry chain.
It is worth noting that each country’s risk threshold is set as the total import volume multiplied by the threshold parameter
The niobium industry chain is a complex system with numerous countries participating at each stage. Governing bodies strive to ensure the sustainable development of the industry through strategic planning of supply channel. However, analyzing the impact of primary product supply constraints within the context of overall strategic development can be challenging. The second scenario presents a more extreme situation where a country experiences a complete disruption in its ability to supply all products. This could arise from events such as large-scale natural disasters and COVID-19 (Chowdhury et al., 2021; El Baz and Ruel, 2021). Different from the single product supply restriction scenario, in this case, the supply shortage originating from the risk source node A (at iteration 1) simultaneously affects all layers (L1, L2, and L3). This means that
COVID-19 has increased global awareness of the fragility and resilience of supply chains. In the niobium industry, emergencies such as extreme weather, geopolitical events, public health crises, and economic downturns can suddenly disrupt supply chains. These disruptions can spread rapidly through international trade, affecting all parts of the global industry, potentially leading to significant economic consequences such as price volatility, production delays, and decreased availability of essential goods. Similar disruptions observed in other critical mineral markets, such as the rare earth element market in 2010, have demonstrated the potential for widespread economic impact. To build a more secure and resilient niobium supply chain, it's crucial to identify the countries that pose the greatest risk and understand how disruptions affect different parts of industry. This study employs a multi-layer network model to simulate two realistic risk scenarios, reflecting potential disruptions observed in other critical mineral markets: (1) one country restricting exports of raw niobium materials, analogous to a complete export ban, drawing upon extreme analogies such as Kazakhstan’s chromium ore export restrictions; and (2) a total cessation of exports from one country, similar to disruptions experienced during global-scale public health crises like the COVID-19 pandemic or widespread geopolitical events. We then analyze how these disruptions spread through the niobium supply chain and affect different industry sectors (primary, intermediate, and final product).
Table 3 shows the ten countries whose export restrictions would cause the most disruption (“avalanches”) in the niobium supply chain under the three resilience scenarios (β = 0.1, 0.5, and 0.9). We consider three levels of risk tolerance (“resilience”) for other countries, represented by a parameter ranging from 0.1 (low resilience) to 0.9 (high resilience). The sensitivity analysis reveals that when global resilience is low (β = 0.1), restricting exports from any of the top ten countries causes avalanches across all parts of the niobium industry. The final product sector is most affected, followed by the intermediate product sector. Interestingly, the raw material sector is affected less than the other two, even though it’s the direct source of the disruption. As resilience increases (0.5), the overall level of disruption decreases significantly. Only China and Malaysia still cause major disruptions to the final product sector. For the intermediate and raw material sectors, most disruptions become much smaller. When resilience is high (0.9), the niobium supply chain becomes very stable. Disruptions are minimal across all sectors, with the final product sector experiencing almost no disruptions. These results show that the final product sector is most sensitive to disruptions, followed by the intermediate product sector and lastly, the raw material sector. This vulnerability of downstream industries highlights the potential for significant economic consequences, including increased prices for steel used in construction, automotive, and energy industries, potential performance degradation or shortages of specialized steel used in aerospace and energy applications, and potential disruptions to the supply chain of certain medical devices. This heightened vulnerability of the final product sector can be attributed to several factors. For example, disruptions upstream, such as restrictions on raw niobium exports, are magnified as they move down the value chain. Furthermore, final products generally have a much higher value-added than raw materials or intermediate goods. Therefore, improving the resilience of countries, especially those involved in the final product stage, is crucial for ensuring the stability and robustness of the entire niobium supply chain and mitigating potential economic damage. The disproportionate vulnerability of downstream sectors to supply disruptions observed here is consistent with experiences in other critical mineral markets, most notably the rare earth element market. The 2010–2011 crisis, driven by China’s export restrictions, demonstrated this vulnerability, significantly impacting downstream industries like electronics, renewable energy, and defense, which experienced price spikes and production bottlenecks due to their reliance on REEs.
China, the United States, Malaysia, Spain, and Turkey consistently rank among the top ten countries whose export restrictions would cause the most disruption, regardless of the resilience level of other countries. This highlights their importance in the global niobium industry. China and the United States are major consumers of niobium, while Malaysia is a key processor and exporter of niobium products. Spain and Turkey are important trading partners in the raw material sector. Interestingly, China and Malaysia are less affected by disruptions than other countries. This suggests they have larger domestic reserves and production, making them less reliant on imports. In contrast, Brazil, the main supplier of raw niobium, and Canada, another important supplier, are less likely to cause major disruptions when other countries have higher resilience. This indicates that diversifying the sources of raw niobium and building up reserves could help reduce the overall risk to the supply chain.
Table 3 demonstrates that a scenario where niobium-rich countries disrupt the industry chain by restricting supply is not a foregone conclusion. Therefore, to gain a deeper understanding of the potential consequences, we consider both the severe repercussions of node-level supply constraints and the characteristics of the network structure at the node level, such as weighted out-degree and betweenness centrality. Focusing on China, the United States, Brazil, and Malaysia as case studies, this paper analyzes the impact of supply constraints imposed by these four countries on the three-tier trade network of the niobium industry chain.
China is widely recognized as the largest consumer of niobium, heavily reliant on imports. Its trade volume consistently ranks among the top three across primary, intermediate and final product sectors. However, an often-overlooked aspect is China’s role as the largest exporter of primary products and the second-largest exporter in the other two sectors. This suggests that China is deeply involved in all stages of the niobium industry chain. Therefore, a chain-wide avalanche triggered by China’s restrictions of primary product supply becomes a plausible scenario. When China experiences supply disruptions at the primary product level, it leads to significant losses for most countries. This situation is further exacerbated at the intermediate and final product levels, as the number of affected countries increases along the industry chain. In terms of import losses, the primary product layer suffers the most significant impact, followed by the final product layer. Analyzing geographical proximity, we find that five of the top 10 countries experiencing upstream import losses are located in Asia (India, China, Korea, Singapore, and Indonesia) (Figure 3A). This proportion rises to six in the midstream segment (Malaysia, China, Thailand, India, Indonesia, and Turkey) (Figure 3C), but falls to two in the downstream segment (Thailand and China) (Figure 3E). This highlights the crucial role those Asian countries in the primary processing stage of niobium products.
Figure 3. The impact of supply restrictions on primary products in China and Malaysia on the industrial chain. (A) and (B) represent import losses for countries in the upstream primary product layer, in the same way that (C) and (D) represent the intermediate product layer and (E) and (F) represent the downstream final product layer.
In contrast, while three of the top 10 countries experiencing losses in the primary product layer are located in Europe (Netherlands, Finland, France), this number increases to five in the final product layer (Germany, France, United Kingdom, Belgium and Spain). This highlights the dominance of developed European countries in niobium deep processing technology. However, even advanced technological capabilities are ultimately vulnerable to constraints in the upstream supply of primary products. Across all stages of the industry chain, China’s impact on United States import losses is undeniable. Across all stages of the industry chain, China’s impact on United States import losses is undeniable. Notably, supply constraints originating in China hinder the supply of niobium products throughout its domestic industry chain, leading to a gap between production and demand within the country. China, the world’s largest consumer of niobium, plays a dominant role in the global niobium industry chain. Its heavy reliance on imports, coupled with its ambitious global infrastructure projects under the Belt and Road Initiative, makes securing a stable niobium supply a strategic priority. This mirrors China’s approach to other critical minerals, such as rare earth elements, where it has exerted significant market influence, even resorting to export restrictions in the past, as seen during the 2010–2011 rare earth crisis. Furthermore, China’s increasing investments in resource-rich African countries, some of which have significant niobium reserves, have geopolitical implications. Therefore, any disruption to China’s niobium supply could have significant repercussions for the global economy, similar to the disruptions experienced during the rare earth crisis, highlighting the importance of diversification and resilient supply chains for this critical mineral.
Within the international niobium industry chain, Malaysia plays a crucial role in processing and smelting niobium ore. Leveraging its advantageous geographical location and well-developed port facilities, Malaysia exports processed niobium products to major consumer countries like China, the United States, Japan, and those in Europe. While Malaysia holds a significant position in the primary product layer, it heavily relies on imports of intermediate goods. This figure rises to 100% in both the midstream and downstream layers (Figures 3D, F). Consequently, the production and development of related industries in numerous countries heavily reliant on Malaysian raw materials would experience severe disruptions. Malaysia’s role as a critical processing and export hub for niobium is deeply intertwined with its broader economic strategy of leveraging its geographical advantage and well-developed port infrastructure. This reliance on trade, similar to that observed in other Southeast Asian nations like Singapore, while contributing to economic growth, also increases its vulnerability to supply chain disruptions. Furthermore, Malaysia’s position in the niobium industry chain mirrors that of other critical mineral processing centers, such as Vietnam’s role in rare earth processing. However, unlike Vietnam, which primarily focuses on attracting foreign investment for processing, Malaysia emphasizes developing domestic processing capacity, and actively seeks to establish long-term strategic partnerships with major consuming countries to secure stable market access. Therefore, understanding Malaysia’s specific vulnerabilities is crucial for assessing the overall resilience of the niobium supply chain.
Restrictions on primary product exports from Malaysia would impact over 97% of trading participants in this layer (Figure 3B), higher than China’s around 95%. This figure rises to 100% in both the midstream and downstream layers (Figures 3D, F). Consequently, the production and development of related industries in various countries heavily reliant on Malaysian raw materials would experience severe disruptions. Despite their distinct roles in the global economy, China and Malaysia exhibit similar avalanche outcomes due to their comparable upstream positioning within the niobium industry chain. This suggests that countries with similar roles in international trade of primary niobium products also share similar structural characteristics in their trading networks. This further underscores Malaysia’s irreplaceable role in the industry. Export restrictions imposed by Malaysia in the upstream layer trigger a chain reaction, leading to widespread avalanches in the midstream layer. This is not a direct upstream effect but rather a consequence of the midstream product layer’s dependence on the primary product layer. An exception to this pattern is observed in countries like Greenland, which are not involved in international trade of primary commodities. These countries remain unaffected in the upstream layer when either China or Malaysia acts as the source of risk. However, this does not imply immunity from shocks originating from key players. The impact of such shocks becomes evident in the midstream and downstream layers of international trade.
The United States plays an essential role in the global niobium industry chain, actively participating across upstream, midstream, and downstream stages. Despite possessing limited niobium resources and facing production constraints that fall short of domestic demand, the United States remains a significant player in upstream trade. Figure 4A illustrates the United States’s efforts to secure niobium resources and diversify its supply channels. Furthermore, the United States boasts strong processing capabilities and holds a leading technological advantage in the midstream segment of the niobium industry chain. As a major global consumer of niobium, the United States excels in the research, development, production, and application of niobium materials, enabling it to manufacture high-value-added products. This establishes the United States as a key player in the downstream end-product market (Figures 4C, E). Specifically, in sectors like aerospace, defense, and energy, niobium alloys are crucial for manufacturing key components. Disruptions to niobium supply could lead to production delays, cost increases, and even production halts in these sectors. To address potential supply disruptions, the United States actively pursues diversification of its supply sources, such as establishing long-term partnerships with Brazil and Canada. Furthermore, the United States is exploring research into substitute materials and recycling technologies for niobium (e.g., potential substitutes for niobium in high-strength steel alloys) to reduce reliance on external supplies. However, if the United States were to restrict the supply of niobium primary products, various parts of its domestic industrial chain would experience disruptions, with the midstream layer bearing the brunt of the impact. Notably, the most significant import losses for the United States would occur in the final product layer. Although both China and the United States are major consumers of niobium, their roles within the niobium industry chain differ significantly. China possesses greater advantages in the upstream and midstream segments, while the United States, with its advanced processing technology and focus on high-value-added products, plays a more significant role in the downstream layer. Nevertheless, the results for both countries underscore the need to not only mitigate the risks associated with supply chain disruptions originating from other countries but also to strengthen domestic stability. Ultimately, a failure in any single link within their respective domestic industry chains could potentially lead to severe disruptions.
Figure 4. The impact of primary product supply restrictions in the United States and Brazil on the industrial chain. (A) and (B) represent import losses for countries in the upstream primary product layer, in the same way that (C) and (D) represent the intermediate product layer and (E) and (F) represent the downstream final product layer.
Brazil boasts the world’s largest niobium reserves and production capacity. Companhia Brasileira de Metalurgia e Mineração (CBMM) headquartered in Brazil, is the world’s leading producer of niobium, controlling a significant portion of the global supply and wielding considerable influence over the entire niobium industry chain. CBMM’s dominant market share grants it significant influence over global niobium prices and supply. This concentrated market power raises concerns about potential price volatility and the vulnerability of downstream industries reliant on niobium. Brazil’s niobium industry primarily focuses on upstream mining and primary processing. The majority of its niobium products are exported as raw materials, such as ferro-niobium and niobium oxides. This has led to an overreliance on resource exports in the upstream segment. Furthermore, Brazil’s domestic applications for niobium products remain relatively limited, resulting in a dependence on imported processed niobium goods. This limited domestic consumption can be attributed to a relatively underdeveloped downstream industrial base. While Brazil possesses a growing manufacturing sector, industries that are major consumers of niobium, such as high-performance steel production for automotive and aerospace applications, and advanced manufacturing utilizing niobium alloys, are not as robust as in other advanced economies. While restricting Brazil’s upstream primary exports would undoubtedly have a significant impact on the global niobium market, the repercussions on upstream trading partners would be less pronounced compared to the effects of similar restrictions imposed by Malaysia or China (Figure 4B). This suggests that a country’s export volume does not necessarily correlate directly with the magnitude of its impact on the industry chain. In reality, the most vulnerable countries across all stages of the chain are those that function as import-processing-export hubs (Figures 4D, F). Nonetheless, similar to the scenarios involving Malaysia and China, export restrictions imposed by Brazil in the upstream segment can still lead to import losses for countries in the downstream segment.
Extreme contingencies such as war, a total blockade, severe natural disasters, political instability, or drastic economic policies are most likely catalysts for a country to impose restrictions on the export of all products throughout its entire industrial chain. While the frequency of such events is relatively low, the challenges posed to global supply chains by the COVID-19 pandemic necessitate considering this scenario as a realistic possibility. Therefore, we simulate a scenario where a country within the GNICN restricts the export of all primary, intermediate, and final products. This implies that the country becomes a source of risk at the outset of each layer, simultaneously propagating supply risk shocks throughout the network. The interdependence between adjacent layers across countries further accelerates the speed of avalanche propagation. Although the overall size of the avalanche in this scenario does not significantly alter the ranking of the top ten most affected countries listed in Table 3, these countries do experience a slight increase in avalanche size at the primary product level. Notably, however, the magnitude of commodity avalanches induced by China decreases to 83%. This indicates that when China acts as a source of risk, comprehensive export channel restrictions can effectively disrupt transmission pathways within the commodity layer, thereby mitigating the overall impact of China as a risk source. In summary, China, the United States, Brazil, and Malaysia play key roles in the vulnerability of the niobium industry chain. While the magnitude of avalanches triggered by key risk sources in the midstream and downstream segments appears larger than in the upstream segment, a closer examination reveals that import losses are most substantial for upstream trading partners, followed by downstream partners, and finally, midstream partners. This pattern reflects the dual nature of risk propagation within the niobium industry chain: horizontal diffusion across trade networks and vertical transmission along the industry value chain. The geographical clustering of affected countries highlights the existing division of labor within the international niobium industry. Upstream Asian countries are particularly vulnerable to disruptions, while the European region experiences greater losses in downstream exports. To mitigate their vulnerability, Asian countries like China, Malaysia, and India should prioritize the development of high-value-added advanced technologies and reduce their reliance on the initial processing of raw materials. Furthermore, ensuring the stability of exports from resource-exporting countries, key hub countries, and major consuming countries is paramount for the sustainable development of the GNICN.
To pinpoint the detailed transmission pathways within the niobium industry chain, uncover the avalanche dynamics across different stages, and proactively identify potential risk points and vulnerabilities, we aim to optimize the industry structure and ensure the sustainable operation of the niobium industry chain. This involves a comprehensive analysis of risk propagation, enabling us to better predict and mitigate potential disruptions. The risk propagation path stemming from primary products supply constraints follow a crisscrossing impact propagation pattern that impacts the primary product layer, intermediate product layer, and final product layer. Specifically, export restrictions on primary niobium products imposed by risk-source countries initially trigger a contagion effect within the upstream primary network layer. Subsequently, due to the domestic production linkages between the primary and intermediate stages, shocks propagate to the midstream intermediate layer. Finally, avalanche nodes within the intermediate product layer impact the downstream end-product layer, further spreading the impact among downstream trade participants. To illustrate a more detailed risk propagation pathway, we focus on the severe consequences of China’s supply constraints and the reality of China’s deep participation in international trade. Setting a risk threshold parameter of 0.1 and using China as the risk source, we analyze the impact propagation pathway of China’s primary product supply restriction on the entire industry chain, along with the specific characteristics of risk propagation within each layer. The results demonstrate that supply constraints imposed by China on primary products lead to widespread disruptions within the primary product layer, triggering a rapid diffusion of risk throughout the niobium industry chain. This, in turn, causes devastating consequences for international trade in both intermediate and final products. Notably, the rate of risk diffusion from the intermediate product layer to the final product layer is significantly faster than the diffusion rate from the primary product layer to the intermediate product layer, as illustrated in Figure 5A. This accelerated diffusion can be attributed to the larger number of participants involved in international trade of intermediate and final products, as well as the stronger structural correlations within these layers. While downstream avalanche rates are higher than those observed in the midstream and upstream segments, it is important to note that upstream countries have suffered higher import losses. This result implies that upstream countries with substantial niobium imports play an essential role in spreading risk throughout the industry chain, which contradicts the emphasis on supply constraints. More significantly, if we focus only on the transmission of risks in a single layer of international trade networks at a specific stage of the industrial chain, without accounting for the complex relationships between different stages arising from domestic production, it will inevitably overlook a large number of hidden sources of risk and underestimate the destructive nature of supply constraints.
Figure 5. Risk transmission paths of China’s supply restrictions on primary product resources. (A) risk propagation in the GNICN. China as a source of risk is shown in red, while the upstream primary product layer, midstream intermediate product layer, and downstream final product layer are shown in green, orange and purple, respectively. (B), (C) and (D) show the propagation paths of the primary, intermediate, and final product layers, respectively. The size of the nodes is proportional to the occurrence, and red, blue, and green represent the order of the avalanche of nodes.
During the transmission process, upstream supply constraints rapidly spread through core countries such as the United States and Germany, directly triggering avalanches 32% of the countries within the primary product layer. The resulting chain reaction leads to disruptions in 56% of the countries in this layer. The increase in avalanche size observed in the midstream and downstream layers is also a direct consequence of the initial wave of avalanches affecting these core countries. Specifically, 24% of midstream avalanches are directly caused by upstream node failures, while the remaining 74% are primarily driven by the cascading effects of these initial disruptions. Similarly, over 66% of downstream country avalanches are directly attributed to midstream node failures, highlighting the significant impact of interlayer interactions. While these cross-layer effects might appear to contradict the patterns observed in the midstream layer, they underscore a crucial characteristic of supply constraint impacts: a cascading effect that combines both ascending and radiating diffusion patterns throughout the industry chain. Furthermore, certain core countries, despite playing a limited role in the upstream network avalanche process, exert a substantial influence on the final product network (Figures 5B–D). Asian countries like Japan and Korea demonstrate a greater impact on the primary and intermediate product layers compared to the final product layer. The influence of European countries is primarily concentrated in the intermediate product layer (e.g., United Kingdom, Germany) and the final product layer (e.g., Finland, Poland). Notably, Brazil’s impact is weakest in the end-product trade network compared to the other two layers, further emphasizing its reliance on primary product trade and processing. This underscores the urgent need for Brazil to develop advanced deep processing technologies to expand its competitive advantage within the niobium industry chain. Interestingly, the avalanches observed in China’s midstream and downstream layers are not directly caused by upstream disruptions, demonstrating the resilience of China’s domestic niobium industry chain. However, it is important to note that only a limited number of countries can maintain the stability of a segment of their domestic industry chain in the face of supply constraints imposed by key risk sources. Therefore, fostering synergy and mutual assistance among countries participating in various trade segments is crucial to mitigate the impact of domestic supply shocks and enhance the overall resilience of the niobium industry chain.
The preceding analysis demonstrates that a country need not be a direct trading partner of the risk source, nor participate in the same segment of international trade as the risk source, to experience the cascading effects of an avalanche. Figure 6 presents the number of countries directly, secondarily (second-round), and indirectly affected under a scenario of total export restrictions for all products originating from China, Malaysia, Brazil, and the United States. China exhibits the largest number of directly affected countries across all layers, reflecting its crucial role in every stage of the niobium industry chain, particularly in the intermediate product layer. Furthermore, China exerts the most significant direct influence on countries within all three segments, underscoring its undisputed dominance in the niobium intermediate product market. While Malaysia’s direct impact capacity is relatively limited at the primary product level, its combined impact (direct and indirect) is the strongest. This suggests that Malaysia plays a more substitutable role in upstream processing, refining, and export activities. Although most of Malaysia’s trading partners have alternative import sources, the potential impact of supply constraints originating from Malaysia should not be disregarded. Notably, the final product layer experiences the most significant impact when Brazil imposes comprehensive export restrictions. This reveals that despite Brazil’s advantage in upstream resource endowments, its global role extends throughout the entire industry chain, including the downstream segment, where it plays a vital role. In contrast, the impact of United States export restrictions on the primary product layer is primarily confined to its direct trading partners and their immediate partners, with minimal indirect effects leading to widespread disruptions. Furthermore, the indirect effects of export restrictions imposed by all four countries are predominantly observed in the upstream segment, suggesting a less tightly interconnected trade network in this layer. This collectively indicates that the primary product layer exhibits greater robustness compared to the intermediate and final product layers.
Figure 6. Countries are directly or indirectly affected by supply constraints on all product resources (1, 2, and 3 represent the upstream primary product layer, the intermediate product layer in the middle, and the downstream final product layer of GNICN, respectively).
This study offers valuable insights for countries involved in the niobium industry chain to maintain the stable and sustainable development of each stage and provides a framework for other industries to build multi-layer network models of their respective supply chains and quantify the impact propagation of supply constraints. However, several crucial points warrant further attention. First, the increasing interdependence of the global economy has put the stability of supply chains in the spotlight. Recent years have witnessed a surge in geopolitical risks, natural disasters, and other disruptive events, leading to repeated shocks to global supply chains. In this context, identifying key risk sources and developing effective coping strategies is paramount. The study reveals that China, Malaysia, Brazil, and the United States are key risk sources, each with distinct industry and regional characteristics, leading to differentiated impacts on global supply chains. The industrial structure and positioning of different countries in global value chains determine the extent and manner of their risk exposure. Asian countries are more vulnerable to upstream and midstream risks owing to their widespread participation in the lower and middle segments of global value chains and the high dependence of their related industries on external supplies of raw materials and components for their development; there was a massive avalanche. Asian countries, for instance, are more vulnerable to upstream and midstream risks due to their extensive participation in the lower and middle segments of global value chains. This reliance on external sources for raw materials and components makes them highly susceptible to disruptions, as evidenced by the large-scale avalanche effects observed in our simulations. Specifically, countries like Japan and South Korea, heavily reliant on imported niobium for electronics and steel production, face significant risks if supply from major producers like Brazil or China is disrupted. In contrast, European countries are more concentrated at the high end of the global value chain, focusing on R&D and the production of high-value-added products. Consequently, their exposure to risk is more pronounced in the downstream segments. For example, Germany, a leading manufacturer of high-performance alloys used in aerospace and automotive applications, is highly sensitive to disruptions in the supply of processed niobium products.
This differentiated risk transmission mechanism underscores the need for countries to tailor their coping strategies to their specific circumstances. Asian countries, such as Japan and South Korea, heavily reliant on niobium imports for electronics and steel production, are particularly vulnerable to supply disruptions from major producers like Brazil or China. These countries should prioritize bolstering their monitoring and early warning systems for key risk sources. Enhancing supply chain resilience can be achieved through diversifying sourcing channels and establishing strategic reserves of intermediate products like ferroniobium and niobium oxides. Furthermore, fostering technological innovation to ascend the global value chain and reduce reliance on imported raw materials is crucial. Specific policy measures could include government subsidies to incentivize businesses to build reserves, forging long-term supply agreements with resource-rich nations, and investing in research and development of niobium substitutes. European countries, exemplified by Germany, are more focused on research and development and the production of high-value-added products, leading to greater downstream risk exposure. They should closely monitor shifts in downstream market demand, address the rise of competitors, explore emerging markets to optimize market structure, and continue investing in R&D to maintain technological leadership and product competitiveness. Building strategic reserves of finished products like niobium-containing steel and alloys should also be considered. Concrete steps could involve establishing government-backed reserve repositories, providing subsidies for businesses to maintain reserves, encouraging expansion into emerging markets like Africa and Southeast Asia, and increasing investment in the development of advanced niobium alloys. Second, there are significant differences in the significance and approaches to improving the risk-resistance among the countries participating in different segments of the niobium industry chain. Notably, increasing domestic production and reserves in countries involved in the final product trade is highly effective in preventing and mitigating large-scale avalanches caused by supply constraints. By boosting domestic production capacity, countries can reduce their reliance on imported products and exert greater control over their supply chains. Countries should carefully consider the role of each stage in the industrial chain and adopt tailored solutions to address systemic risks while maximizing their national strengths. For example, resource-rich countries like Brazil can prioritize developing upstream industries to ensure a stable supply of raw materials. Countries with advanced manufacturing industries, such as Germany or Japan, can focus on upgrading their technology and strengthening the resilience of their industrial chains. Countries with large consumer markets, like China or the United States, can actively develop domestic production and reserves to reduce external dependence. However, extending the industrial chain and building a robust industrial system are strategies applicable to all countries, regardless of their specific position in the global value chain.
Third, we must consider the hidden sources of systemic risk that emerge as disruptions propagate along the industrial chain. These hidden risks often lurk in seemingly stable links within the chain, arising from the interdependence between upstream and downstream stages. This interdependence exacerbates the destructive potential of disruptions. Our research shows that Japan and South Korea play essential roles in the upstream and midstream links of some critical industrial chains. Their stable operation is crucial for the production and trade of downstream countries. For example, the 2011 earthquake in Japan caused severe disruption to the global automotive industry chain, forcing manufacturers in many countries to halt production due to a shortage of parts from Japan (Carvalho et al., 2020; Uchida and Bürgmann, 2021). The destructive power of hidden risk sources underscores the importance of recognizing and proactively addressing these risks.
Finally, it is imperative to establish an international cooperation and coordination mechanism encompassing all stages of the niobium industry. Given the close interconnectedness of countries involved in the niobium industry, heightened attention must be paid to the hidden risk sources identified in this study. This includes establishing information-sharing mechanisms, strengthening risk communication and coordination, and jointly developing contingency plans. For instance, regional industrial chain cooperation mechanisms can be improved to facilitate joint responses to emergencies such as natural disasters and pandemics. Cooperation with international organizations can also be strengthened to formulate strategies and measures for addressing global risks collectively.
While the policy recommendations outlined above offer crucial steps towards enhancing the resilience of the niobium supply chain, it is essential to consider how emerging challenges might necessitate further adaptation. The potential impacts of climate change, geopolitical instability, and the development of substitute materials require proactive strategies to mitigate future risks. This includes investing in climate-resilient infrastructure, developing early warning systems for supply chain disruptions, fostering international collaboration on resource security, and supporting research into alternative materials and technologies.
This study examines the resilience of the global niobium supply chain using a multi-layer network approach, which is a relatively unexplored area in the existing literature. While previous studies have analyzed supply chain risks in other industries or using different methodologies (Shi et al., 2022; van den Brink et al., 2022), few have specifically focused on the niobium industry and its unique characteristics. Furthermore, the application of a multi-layer network model allows us to capture the interconnectedness of different stages within the niobium supply chain and analyze the cross-layer impacts of disruptions, which provides a more comprehensive understanding of the system’s vulnerability compared to traditional single-layer network approaches. This study contributes to the growing body of literature on supply chain resilience by providing a detailed analysis of the global niobium industry and its vulnerability to disruptions. Our findings offer valuable insights for policymakers and businesses seeking to develop strategies to mitigate supply chain risks and enhance the sustainability of the niobium industry.
With the global competition of high-tech industries heating up, the sustainable development of the niobium industrial chain has become a pursuit of countries worldwide. The niobium industry chain consists of an upstream primary product, a middle intermediate product, and a downstream final product; each link is interdependent. Disruptions in one part of this chain can have ripple effects throughout the entire system. Therefore, based on all the international trade data in 2022 of the United Nations trade database, this study constructs a multi-layer trade network model of the niobium industry chain and then constructs a multi-layer dynamic risk propagation model to understand how disruptions can spread and affect different parts of the industry. The main conclusions are as follows:
Different stages of the niobium supply chain exhibit varying levels of vulnerability to disruptions. The downstream final product stage is the most susceptible, implying that disruptions in the supply of upstream primary products or middle intermediate products can significantly hinder the production of final products. An analysis of critical sources of risk found that certain countries, such as China and Malaysia, play a pivotal role in the niobium supply chain. Disruptions originating in these countries can have far-reaching consequences, underscoring the importance of diversifying supply sources and mitigating reliance on any single nation. China, a major consumer and importer of niobium, has the potential to trigger substantial disruptions if its supply is constrained. This underscores the interconnectedness of the global niobium industry. Malaysia, a prominent processor and exporter of niobium, serves as a crucial link between upstream primary product suppliers and downstream final product manufacturers. Disruptions in Malaysia can severely impact the entire supply chain. The United States, a major consumer and technology leader, is also susceptible to disruptions, especially in the middle intermediate product segment. This demonstrates that even technologically advanced nations are not immune to supply chain risks. Brazil, the largest supplier of upstream primary product, exerts a considerable influence on the global market, but its impact is less pronounced compared to China and Malaysia. This suggests that a country’s position and role within the trade network, not solely its resource reserves, determine its overall influence. Asian countries like Japan and South Korea, along with European nations such as the United Kingdom and Germany, also play significant roles in the niobium supply chain and can contribute to disruptions. This emphasizes the need for international collaboration to effectively manage risks.
Enhancing a country’s capacity to withstand disruptions is essential for bolstering the resilience of the entire niobium supply chain. This can be achieved through various strategies, including augmenting domestic production and reserves, particularly for countries engaged in the downstream final product stage. For instance, China could encourage domestic niobium exploration and development, support R&D in processing and recycling technologies, and diversify import sources through international collaborations. Brazil could focus on developing downstream processing capabilities, expanding niobium applications, and establishing stable partnerships with downstream manufacturers. Malaysia could enhance its processing technology, diversify export markets, and strengthen collaborations with upstream and downstream partners. Furthermore, international trade agreements should promote information sharing, fair trade practices, and customs cooperation to prevent smuggling and ensure a stable trade environment. Establishing a strategic niobium reserve mechanism and fostering multilateral cooperation to address risks associated with climate change and geopolitical instability are also crucial for building a more resilient global niobium supply chain.
This study provides valuable insights into the risks and vulnerabilities of the niobium supply chain. However, the future of this supply chain also faces emerging challenges, such as the impacts of climate change (e.g., extreme weather events and stricter environmental regulations) on mining and processing, shifting geopolitical dynamics (including resource nationalism and trade disputes), and the development of substitute materials—all of which could impact demand and reshape the industry. These emerging challenges underscore the need for future research focused on quantifying these risks and developing proactive mitigation strategies. Further research directions include exploring tailored risk thresholds based on individual country circumstances, analyzing the impact of demand-side shocks, and investigating specific niobium-dependent industries and products. This information can help policymakers and businesses develop strategies to enhance the resilience and sustainability of the global niobium industry.
Publicly available datasets were analyzed in this study. This data can be found here: https://comtradeplus.un.org/?trk=public_post-text.
FL: Conceptualization, Data curation, Methodology, Resources, Software, Visualization, Writing–original draft. WL: Investigation, Methodology, Software, Supervision, Writing–review and editing. AQ: Conceptualization, Formal Analysis, Supervision, Validation, Writing–review and editing. LQ: Conceptualization, Formal Analysis, Investigation, Supervision, Writing–review and editing. QL: Formal Analysis, Funding acquisition, Investigation, Resources, Writing–review and editing.
The author(s) declare that financial support was received for the research, authorship, and/or publication of this article. This study was funded by the National Natural Science Foundation of China (72074199, 71991483), the China Geological Survey (DD20221795).
Author AQ was employed by China Aerospace Planning and Design Group Co., Ltd. Author LQ was employed by Sichuan Development Environmental Science and Technology Research Institute Co., Ltd.
The remaining authors declare that the research was conducted in the absence of any commercial or financial relationships that could be construed as a potential conflict of interest.
All claims expressed in this article are solely those of the authors and do not necessarily represent those of their affiliated organizations, or those of the publisher, the editors and the reviewers. Any product that may be evaluated in this article, or claim that may be made by its manufacturer, is not guaranteed or endorsed by the publisher.
Artime, O., Grassia, M., De Domenico, M., Gleeson, J. P., Makse, H. A., Mangioni, G., et al. (2024). Robustness and resilience of complex networks. Nat. Rev. Phys. 6 (2), 114–131. doi:10.1038/s42254-023-00676-y
Bhamra, R., Small, A., Hicks, C., and Pilch, O. (2024). Impact pathways: geopolitics, risk and ethics in critical minerals supply chains. Int. J. Operations and Prod. Manag. doi:10.1108/ijopm-03-2024-0228
Bier, T., Lange, A., and Glock, C. H. (2020). Methods for mitigating disruptions in complex supply chain structures: a systematic literature review. Int. J. Prod. Res. 58 (6), 1835–1856. doi:10.1080/00207543.2019.1687954
Bigerna, S., D’Errico, M. C., and Polinori, P. (2021). Energy security and RES penetration in a growing decarbonized economy in the era of the 4 industrial revolution. Technol. Forecast. Soc. Change 166, 120648. doi:10.1016/j.techfore.2021.120648
Bridge, G., and Faigen, E. (2022). Towards the lithium-ion battery production network: thinking beyond mineral supply chains. Energy Res. Soc. Sci. 89, 102659. doi:10.1016/j.erss.2022.102659
Burton, J., Kemp, D., Barnes, R., and Parmenter, J. (2024). A socio-spatial analysis of Australia’s critical minerals endowment and policy implications. Resour. Policy 88, 104448. doi:10.1016/j.resourpol.2023.104448
Carvalho, V. M., Nirei, M., Saito, Y. U., and Tahbaz-Salehi, A. (2020). Supply chain disruptions: evidence from the great east Japan earthquake. Q. J. Econ. 136 (2), 1255–1321. doi:10.1093/qje/qjaa044
Chen, C. Y., Zhao, Y., Gao, J., and Stanley, H. E. (2020). Nonlinear model of cascade failure in weighted complex networks considering overloaded edges. Sci. Rep. 10 (1), 13428. doi:10.1038/s41598-020-69775-5
Chen, G., Kong, R., and Wang, Y. (2020). Research on the evolution of lithium trade communities based on the complex network. Phys. A Stat. Mech. its Appl. 540, 123002. doi:10.1016/j.physa.2019.123002
Chen, Z., and Su, S.-I. I. (2020). International competition and trade conflict in a dual photovoltaic supply chain system. Renew. Energy 151, 816–828. doi:10.1016/j.renene.2019.11.085
Chowdhury, P., Paul, S. K., Kaisar, S., and Moktadir, M. A. (2021). COVID-19 pandemic related supply chain studies: a systematic review. Transp. Res. E Logist. Transp. Rev. 148, 102271. doi:10.1016/j.tre.2021.102271
David, L. O., Nwulu, N. I., Aigbavboa, C. O., and Adepoju, O. O. (2022). Integrating fourth industrial revolution (4IR) technologies into the water, energy and food nexus for sustainable security: a bibliometric analysis. J. Clean. Prod. 363, 132522. doi:10.1016/j.jclepro.2022.132522
Dong, G., Qing, T., Du, R., Wang, C., Li, R., Wang, M., et al. (2020). Complex network approach for the structural optimization of global crude oil trade system. J. Clean. Prod. 251, 119366. doi:10.1016/j.jclepro.2019.119366
El Baz, J., and Ruel, S. (2021). Can supply chain risk management practices mitigate the disruption impacts on supply chains’ resilience and robustness? Evidence from an empirical survey in a COVID-19 outbreak era. Int. J. Prod. Econ. 233, 107972. doi:10.1016/j.ijpe.2020.107972
Gao, Z., Geng, Y., Xiao, S., and Zhuang, M. (2022). Mapping the global anthropogenic chromium cycle: implications for resource efficiency and potential supply risk. Environ. Sci. and Technol. 56 (15), 10904–10915. doi:10.1021/acs.est.2c00709
Gómez, M., Li, J., and Zeng, X. (2024). Niobium: the unseen element-A comprehensive examination of its evolution, global dynamics, and outlook. Resour. Conservation Recycl. 209, 107744. doi:10.1016/j.resconrec.2024.107744
Hu, X., Wang, C., Lim, M. K., Bai, X., and Yao, C. (2021a). Evaluating waste and scrap trade risks in Belt and Road Initiative countries. Resour. Conservation Recycl. 173, 105728. doi:10.1016/j.resconrec.2021.105728
Hu, X., Wang, C., Lim, M. K., Chen, W.-Q., Teng, L., Wang, P., et al. (2023). Critical systemic risk sources in global lithium-ion battery supply networks: static and dynamic network perspectives. Renew. Sustain. Energy Rev. 173, 113083. doi:10.1016/j.rser.2022.113083
Hu, X., Wang, C., Zhu, X., Yao, C., and Ghadimi, P. (2021b). Trade structure and risk transmission in the international automotive Li-ion batteries trade. Resour. Conservation Recycl. 170, 105591. doi:10.1016/j.resconrec.2021.105591
Kalubanga, M., and Gudergan, S. (2022). The impact of dynamic capabilities in disrupted supply chains—the role of turbulence and dependence. Ind. Mark. Manag. 103, 154–169. doi:10.1016/j.indmarman.2022.03.005
Kang, X., Wang, M., Chen, L., and Li, X. (2023). Supply risk propagation of global copper industry chain based on multi-layer complex network. Resour. Policy 85, 103797. doi:10.1016/j.resourpol.2023.103797
Kang, X., Wang, M., Wang, T., Luo, F., Lin, J., and Li, X. (2022). Trade trends and competition intensity of international copper flow based on complex network: from the perspective of industry chain. Resour. Policy 79, 103060. doi:10.1016/j.resourpol.2022.103060
Li, B., Li, H., Ren, S., Liu, H., and Wang, G. (2023a). Commodity supply risk assessment of China’s copper industrial chain: the perspective of trade network. Resour. Policy 81, 103297. doi:10.1016/j.resourpol.2023.103297
Li, B., Ng, S. J., Han, J.-C., Li, M., Zeng, J., Guo, D., et al. (2023b). Network evolution and risk assessment of the global phosphorus trade. Sci. Total Environ. 860, 160433. doi:10.1016/j.scitotenv.2022.160433
Li, C., Guo, X., Lin, W., Tang, Z., Cao, J., and Zhang, Y. (2023). Multiplex network community detection algorithm based on motif awareness. Knowledge-Based Syst. 260, 110136. doi:10.1016/j.knosys.2022.110136
Li, Y., Gao, X., An, S., Zheng, H., and Wu, T. (2021). Network approach to the dynamic transformation characteristics of the joint impacts of gold and oil on copper. Resour. Policy 70, 101967. doi:10.1016/j.resourpol.2020.101967
Liang, Z., Geng, Y., Zhong, C., Xiao, S., and Wei, W. (2024). Tracking the evolution of niobium cycle in China from 2000 to 2021: a dynamic material flow analysis. J. Clean. Prod. 434, 140455. doi:10.1016/j.jclepro.2023.140455
Liu, Q., Sun, K., Ouyang, X., Sen, B., Liu, L., Dai, T., et al. (2022). Tracking three decades of global neodymium stocks and flows with a trade-linked multiregional material flow analysis. Environ. Sci. and Technol. 56 (16), 11807–11817. doi:10.1021/acs.est.2c02247
McCaffrey, D. M., Nassar, N. T., Jowitt, S. M., Padilla, A. J., and Bird, L. R. (2023). Embedded critical material flow: the case of niobium, the United States, and China. Resour. Conservation Recycl. 188, 106698. doi:10.1016/j.resconrec.2022.106698
McNulty, B. A., and Jowitt, S. M. (2021). Barriers to and uncertainties in understanding and quantifying global critical mineral and element supply. iScience 24 (7), 102809. doi:10.1016/j.isci.2021.102809
Naqvi, A., and Monasterolo, I. (2021). Assessing the cascading impacts of natural disasters in a multi-layer behavioral network framework. Sci. Rep. 11 (1), 20146. doi:10.1038/s41598-021-99343-4
Pacini, H., Shi, G., Sanches-Pereira, A., and Filho, A. C. d.S. (2021). Network analysis of international trade in plastic scrap. Sustain. Prod. Consum. 27, 203–216. doi:10.1016/j.spc.2020.10.027
Shao, L., Kou, W., and Zhang, H. (2022). The evolution of the global cobalt and lithium trade pattern and the impacts of the low-cobalt technology of lithium batteries based on multiplex network. Resour. Policy 76, 102550. doi:10.1016/j.resourpol.2022.102550
Shi, Q., Sun, X., Xu, M., and Wang, M. (2022). The multiplex network structure of global cobalt industry chain. Resour. Policy 76, 102555. doi:10.1016/j.resourpol.2022.102555
Shuai, J., Zhao, Y., Xiong, X., Shuai, C., and Wang, J. (2023). The co-opetition relationships of critical mineral resources for the solar PV industry between China and the world major powers. J. Clean. Prod. 426, 139171. doi:10.1016/j.jclepro.2023.139171
Silveira, J. W., and Resende, M. (2020). Competition in the international niobium market: a residual demand approach. Resour. Policy 65, 101564. doi:10.1016/j.resourpol.2019.101564
Sun, X., Shi, Q., and Hao, X. (2022). Supply crisis propagation in the global cobalt trade network. Resour. Conservation Recycl. 179, 106035. doi:10.1016/j.resconrec.2021.106035
Uchida, N., and Bürgmann, R. (2021). A decade of lessons learned from the 2011 Tohoku-Oki earthquake. Rev. Geophys. 59 (2), e2020RG000713. doi:10.1029/2020rg000713
van den Brink, S., Kleijn, R., Sprecher, B., Mancheri, N., and Tukker, A. (2022). Resilience in the antimony supply chain. Resour. Conservation Recycl. 186, 106586. doi:10.1016/j.resconrec.2022.106586
Wang, C., Huang, X., Hu, X., Zhao, L., Liu, C., and Ghadimi, P. (2021). Trade characteristics, competition patterns and COVID-19 related shock propagation in the global solar photovoltaic cell trade. Appl. Energy 290, 116744. doi:10.1016/j.apenergy.2021.116744
Wang, C., Sun, W., Lim, M. K., Hu, X., Gao, Y., and Ghadimi, P. (2022). Structural evolution of global plastic life cycle trade: a multi-layer network perspective. Sustain. Prod. Consum. 33, 1031–1042. doi:10.1016/j.spc.2022.08.027
Wang, C., Zhao, L., Lim, M. K., Chen, W.-Q., and Sutherland, J. W. (2020). Structure of the global plastic waste trade network and the impact of China’s import Ban. Resour. Conservation Recycl. 153, 104591. doi:10.1016/j.resconrec.2019.104591
Wang, X., Wang, A., Zhong, W., Zhu, D., and Wang, C. (2022a). Analysis of international nickel flow based on the industrial chain. Resour. Policy 77, 102729. doi:10.1016/j.resourpol.2022.102729
Wang, X., Wang, A., and Zhu, D. (2022b). Simulation analysis of supply crisis propagation based on global nickel industry chain. Front. Energy Res. 10. doi:10.3389/fenrg.2022.919510
Wickramaratne, D., and Mazin, I. I. (2022). Effect of alloying in monolayer niobium dichalcogenide superconductors. Nat. Commun. 13 (1), 2376. doi:10.1038/s41467-022-29213-8
Williams-Jones, A. E., and Vasyukova, O. V. (2023). Niobium, critical metal, and progeny of the mantle. Econ. Geol. 118 (4), 837–855. doi:10.5382/econgeo.4994
Yin, D., Huang, W., Shuai, B., Liu, H., and Zhang, Y. (2022). Structural characteristics analysis and cascading failure impact analysis of urban rail transit network: from the perspective of multi-layer network. Reliab. Eng. and Syst. Saf. 218, 108161. doi:10.1016/j.ress.2021.108161
Zeng, X., Gómez, M., Bakry, M., Geng, Y., and Li, J. (2024). Anthropogenic mineral generation and its potential resource supply: the case of niobium. Sci. China Earth Sci. 67 (8), 2583–2591. doi:10.1007/s11430-023-1349-2
Zhou, L., Geng, Y., Chen, Z., Xiao, S., and Wei, W. (2024). Tracking the evolution of niobium cycle in China from 2000 to 2021: a dynamic material flow analysis. J. Clean. Prod. 434, 140455. doi:10.1016/j.jclepro.2023.140455
Keywords: niobium, supply risk, multi-layer network, cascading failure, trade policy
Citation: Luo F, Liu W, Qin A, Qu L and Liu Q (2024) The supply risk of the global niobium industry chain: propagation path and impact analysis based on multi-layer network. Front. Earth Sci. 12:1490811. doi: 10.3389/feart.2024.1490811
Received: 03 September 2024; Accepted: 22 November 2024;
Published: 12 December 2024.
Edited by:
Hongjian Zhu, Yanshan University, ChinaReviewed by:
Jianda Wang, Hong Kong Polytechnic University, Hong Kong SAR, ChinaCopyright © 2024 Luo, Liu, Qin, Qu and Liu. This is an open-access article distributed under the terms of the Creative Commons Attribution License (CC BY). The use, distribution or reproduction in other forums is permitted, provided the original author(s) and the copyright owner(s) are credited and that the original publication in this journal is cited, in accordance with accepted academic practice. No use, distribution or reproduction is permitted which does not comply with these terms.
*Correspondence: Qunyi Liu, MTQ0NzgxNDlAcXEuY29t
Disclaimer: All claims expressed in this article are solely those of the authors and do not necessarily represent those of their affiliated organizations, or those of the publisher, the editors and the reviewers. Any product that may be evaluated in this article or claim that may be made by its manufacturer is not guaranteed or endorsed by the publisher.
Research integrity at Frontiers
Learn more about the work of our research integrity team to safeguard the quality of each article we publish.