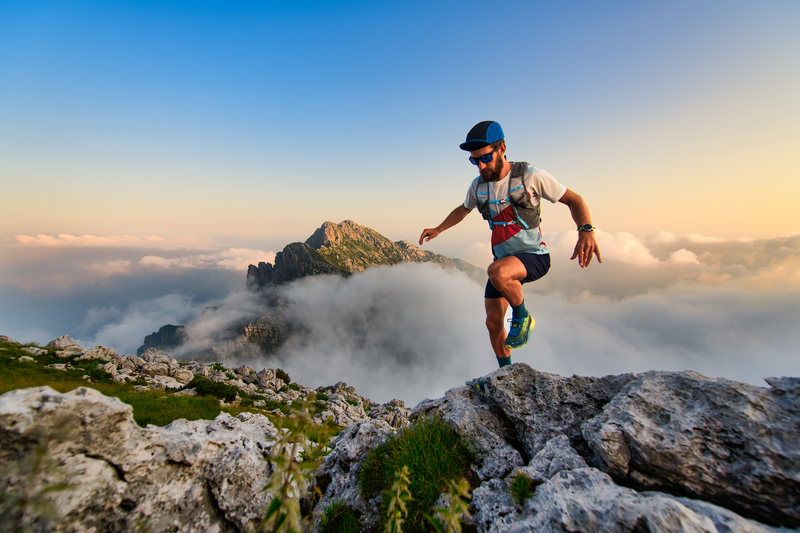
95% of researchers rate our articles as excellent or good
Learn more about the work of our research integrity team to safeguard the quality of each article we publish.
Find out more
ORIGINAL RESEARCH article
Front. Earth Sci. , 07 November 2024
Sec. Solid Earth Geophysics
Volume 12 - 2024 | https://doi.org/10.3389/feart.2024.1452324
In recent years, ambient seismic noise has gained considerable attention in seismology due to its potential to advance understanding of Earth’s subsurface dynamics and monitor anthropogenic activities. This study presents a comprehensive review of ambient seismic noise research (ASNR), by exploring its current status, key research hotspots, and emerging trends through a bibliometric and visual analysis of 3,028 articles indexed in the Web of Science database (1984–2023). Using CiteSpace, the study systematically examines key authors, institutions, and countries, as well as thematic keywords and foundational references. Techniques such as cluster analysis, co-citation network analysis, and burst detection are employed to map the evolution of research fields and identify significant collaboration patterns. The analysis reveals a dramatic increase in research output, particularly since 2004, underscoring the expanding role of ASNR in geophysics, geochemistry, and engineering applications. Additionally, the rising interest in detecting human activities through seismic noise, especially in response to events like the COVID-19 pandemic, highlights the broadening scope of ASNR. Notably, the findings emphasize the pivotal importance of ambient noise tomography, a method that has transformed subsurface imaging techniques. This review not only synthesizes the current research landscape but also highlights critical gaps and emerging opportunities, providing a roadmap for future studies. In particular, it emphasizes advancements in seismic risk mitigation, geotechnical investigations, and the monitoring of human activities, offering a timely review and valuable insights that aligns with the interests of researchers in these fields.
Seismic stations continuously record waveforms that capture not only seismic events but also ambient noise, a type of background signal caused by natural and human activities. According to Byrne (1961), ambient seismic noise arises from various sources, including natural vibrations of the Earth’s crust, oceanic movements, and anthropogenic activities. Ambient seismic noise has long been a valuable resource in seismology for monitoring Earth’s subsurface dynamics. It has become increasingly important due to advancements in seismic observation technologies and large-scale computing, which have facilitated real-time data processing. While earlier studies focused primarily on earthquake signals, the continuous waveform data from seismic stations provide rich insights beyond earthquake events, capturing a variety of environmental and anthropogenic activities. These signals have been employed to enhance understanding of Earth’s structure and offer promising tools for monitoring subsurface conditions without the need for active seismic surveys.
These ambient noise sources span diverse frequency ranges, each associated with specific activities, encompassing natural disturbances such as non-seismic motion in the deep crust, water movements, including ocean surface waves, ocean currents, and tides, meteorological changes, and cultural sources emanating from human activities. According to McNamara and Buland (2004), the primary noise sources across different frequency bands include: (1) Long-period seismic noise (10–50 s), often induced by meteorological factors like wind and atmospheric pressure changes; (2) Short-period noise (0.2–10 s), attributed to oceanographic factors, such as ocean surface waves and tides, which show seasonal variations; (3) High-frequency noise (0.025–0.2 s), often caused by human activities such as traffic and industrial machinery. Collectively, these various noise sources create a rich dataset that seismologists can leverage for numerous applications, including environmental and anthropogenic monitoring (Peterson, 1993).
ASNR has garnered significant attention since the 1950s (Aki, 1957). Early studies analyzed continuous waveform data from seismic stations, and over time, these methods have evolved into powerful tools for investigating Earth’s subsurface (Haubrich, 1965). Quantitative analysis of ambient noise characteristics servers as a widely employed technique for imaging the Earth’s structure, exploring the subsurface conditions and monitoring human activities. For instance, Ambient noise tomography, reliant on continuous waveform data recorded by broadband seismic stations, has emerged as an effective and broadly applicable method for investigating crustal velocity structure (Fang et al., 2010; Li et al., 2009; Yao et al., 2006; Zheng et al., 2011). This technique has found applications beyond seismology, playing important roles in urban planning, geology, engineering, materials science, disaster management, public safety management, and computer science. Notably, Zhang et al. (2014) demonstrated its effectiveness in monitoring building vibrations in urban environments, while Smith et al. (2021) applied ASNR methods for non-invasive material characterization in materials science.
The increasing interest in ASNR is also reflected in its role in disaster management and human-induced activity. For example, real-time monitoring systems that incorporate ASNR have been developed to provide early warnings for seismic events (Chen et al., 2023; Chen et al., 2022; Zhang et al., 2014). Moreover, ASNR has recently demonstrated its versatility by being applied to track shifts in human activity during global events such as the COVID-19 lockdowns, as highlighted by studies like Lecocq et al. (2020) and Yabe et al. (2020). Additionally, Dando et al. (2023) leveraged seismic array data to detect and analyze attacks in the Russia–Ukraine conflict, showcasing ASNR’s growing significance in geopolitical monitoring.
Despite the growing volume of research on ambient seismic noise, there is a lack of systematic reviews that assess the trends and developments in this field. This study addresses this gap by employing bibliometric and visual analysis techniques using the CiteSpace tool, to map the intellectual structure of ASNR. By analyzing 3,028 articles indexed in the Web of Science Core Collection (1984–2023), this review identifies key authors, institutions, research hotspots, and emerging trends in the field. The use of co-citation network analysis, cluster analysis, and burst detection will offer valuable insights into the collaboration patterns and thematic shifts that have shaped ASNR over time. Moreover, this review synthesizes critical research progress and proposes a roadmap for future studies, emphasizing the importance of ASNR in the fields such as seismic hazard assessment, geotechnical investigations, and human-induced activity monitoring.
The rationale behind this work is grounded in the growing multidisciplinary relevance of ASNR, not only in traditional seismological applications but also in addressing contemporary challenges such as environmental monitoring and disaster management. As ASNR continues to evolve and expand its applications, understanding its research landscape is vital for guiding future research efforts and fostering collaborations across different scientific domains. This study, therefore, contributes to the literature by providing a comprehensive review that elucidates the current status of ASNR and highlights emerging opportunities for advancing this important field.
WoSCC, provided by Clarivate Analytics, stands as a renowned multidisciplinary citation database, granting access to impactful journals across diverse scientific fields. With features like citation indexing, advanced search capabilities, and detailed bibliographic information, it is a valuable resource for researchers tracing scholarly influence. With an impressive repository of 18,000 journals and 1.3 billion citations since 1900, the Core Collection is chosen for visualization analysis through CiteSpace, justifying its exclusive use over other databases like Google Scholar and Scopus due to consistent results (Zang et al., 2022). In this study, a search was conducted using the “topic” search terms “ambient seismic noise” within the WoSCC database, spanning the period from 1984 to 2023 (retrieved on 1 November 2023). Ultimately, a total of 3,030 available results were obtained, limited to English papers.
This study employs CiteSpace (version 6.2.R4), a powerful network and visualization analysis tool developed by Chen (2004), to conduct a comprehensive visual analysis of the ASNR field. CiteSpace stands out for its ability to generate knowledge maps for authors, institutions, countries, keywords, terms and co-citation networks, offering insights into the research landscape (Chen, 2006). In comparison to other visual analysis tools such as VOSviewer, SCI2, and HistCite, CiteSpace provides superior efficiency and functionality, particularly in its data processing capabilities. Additionally, its advanced metrics like Modularity Q and Mean Silhouette score enable a more nuanced understanding of research clusters and the structure of the academic network, further enhancing its analytical power.
In this study, CiteSpace is employed to conduct a comprehensive analysis of the ASNR field through literature co-citation analysis, author co-citation analysis, burst analysis, and term cluster analysis. These methods are critical for uncovering the intellectual structure and research dynamics of the field: (1) Literature co-citation analysis identifies frequently co-cited articles, helping to uncover foundational papers and their interconnections. By mapping these citation networks, influential studies that have shaped the ASNR domain can be pinpointed, providing a structured view of the field’s development. (2) Author co-citation analysis maps influential researchers and their networks of scholarly influence. By analyzing co-citations between authors, key academic figures and collaborative networks that drive research in the field can be identified. This analysis provides insights into which researchers or groups are central to the advancement of ASNR-related topics. (3) Burst analysis detects sudden increases in the frequency of citations or keywords, indicating that these subjects have gained significant attention over a short period. A higher burst value suggests more impactful and rapid developments within the field, highlighting emerging trends and shifts in research focus. This tool is particularly useful for recognizing areas that are gaining momentum and could represent future directions in the field. (4) Term cluster analysis organizes related keywords into clusters, providing a visual representation of major research themes and their evolution over time. By identifying these clusters, we can observe how research topics have grown or shifted, offering a timeline of the thematic evolution within ASNR research.
CiteSpace also generates visualization maps consisting of nodes and links, where nodes represent various analytical elements such as countries, authors, institutions, terms, and references, while links symbolize the interactions or relationships between them. Each node is depicted as a circle, with the color corresponding to the year associated with the node, providing an intuitive representation of the temporal distribution of research elements. This visual approach offers a clearer understanding of the relationships between different research themes and how they have evolved over time.
To assess the strength of clustering within the network, CiteSpace employs Modularity Q, a critical metric that measures whether nodes within the same cluster are more densely connected internally than with the rest of the network. A higher Modularity Q value, closer to 1, indicates a clearer and more distinct structure in the network, with well-defined research themes or topics. In this study, Modularity Q is used to evaluate the cohesiveness of research themes within the ASNR field, with higher values signifying that these themes are well-structured and clearly differentiated.
Another important metric, the Mean Silhouette score, assesses the validity of clustering within the network by evaluating how similar an object is to its own cluster compared to other clusters. A higher Mean Silhouette score, closer to 1, indicates more distinct and well-separated clusters, whereas a lower score suggests overlapping or poorly defined clusters. In this study, the Mean Silhouette score is used to validate the identified research clusters within ASNR, ensuring that the themes are not only distinct but also internally consistent.
By leveraging the functionalities of literature co-citation analysis, author co-citation analysis, burst analysis, and term cluster analysis, along with the critical metrics of Modularity Q and Mean Silhouette score, this study provides a comprehensive and robust bibliometric analysis of the ASNR field. These tools contribute to a deeper understanding of research trends, intellectual structures, and the evolution of knowledge within the domain. Compared to traditional review methods, which may rely on subjective interpretation, CiteSpace enables a more objective and systematic approach, ensuring that emerging trends and foundational research are identified with precision.
The initial stage of the process entailed retrieving the literature through a “topic” search using the term “ambient seismic noise” in WoS database. The obtained records were then saved and stored in a file named “download.txt” in a format compatible and recognizable by CiteSpace. Subsequently, data deduplication was conducted using CiteSpace to eliminate any duplicate records. After the deduplication process, a total of 3,028 unique records remained in the dataset, with 2 records being discarded due to identification as duplicates during this process. Figure 1 shows the flowchart of the research methodology.
In this study, the parameters of CiteSpace were configured as follows: initially, in the time-slicing module, the “time” parameter, indicating the year range of publication, was set from January 1984 to November 2023, and “years per slice” was set as “1”, signifying that the dataset is divided into the unit by 1 year. In “term source” part, all options were selected. “Node type”, “Selection criteria”, and “Pruning” were set as “at a time”, “g-index, 25”, “pathfinder” respectively. In “visualization” section, “cluster view-static” and “show merged network” were selected. These parameter settings were applied to CiteSpace to conduct a comprehensive analysis and visualization of the ASNR literature.
Figure 2 depicts the annual distribution of ASNR. Based on the graph, the studied period can be categorized into two stages: pre-2004 and post-2004. Notably, the annual count of ASNR has consistently and significantly increased since 2004, except for minor fluctuations in 2007, 2009, and 2019. It is noteworthy that the dataset for 2023 only covers January to October. In summary, these observations underscore a continual growth in attention to ASNR over time.
Table 1 displays the top 10 subject categories for documents referencing ASNR, covering a total of 35 subject categories in WoSCC. The leading categories include geochemistry geophysics (1805 documents, 59.57% of the total), geosciences multidisciplinary (842, 27.79%), engineering geological (171, 5.64%), engineering civil (75, 2.48%), and mining mineral processing (75, 2.48%). Following closely are multidisciplinary sciences, environmental sciences, water resources, meteorology atmospheric sciences, and acoustics. The distribution highlights the prominence of geochemistry, geophysics, geosciences, and geological issues in the field.
Table 2 outlines the top 10 countries in terms of literature contributions to ambient seismic noise research. The United States (United States) leads with 1,005 documents, closely followed by Mainland China with 638 documents, and France in third place with 428 documents. Other noteworthy contributors include Italy (355 documents), Germany (236 documents), Australia (181 documents), and Switzerland (168 documents). These top 10 countries have played a substantial role in advancing literature on ambient seismic noise.
Table 3 presents the leading 10 institutions actively contributing to ambient seismic noise research. The Centre National de la Recherche Scientifique (CNRS) secures the top position with 337 articles, followed by the China Academy of Sciences with 266. Sharing the third spot are the Communaute Universite Grenoble Alpes and Universite Grenoble Alpes (UGA), each with 232 articles. Following is the Institut de Recherche pour le Developpement (IRD) with 191 documents. These institutions have made substantial contributions to ambient seismic noise research, playing a significant role in advancing the field.
Regarding the most prolific journals in ASNR (Table 4), Geophysical Journal International stands out as the leading specialist journal in ambient seismic noise research, having published 534 articles from 1984 to October 2023. Following is the Journal of Geophysical Research: Solid Earth with 338 articles, underscoring its significance in the field. Geophysical Research Letters, Bulletin of the Seismological Society of America, and Seismological Research Letters also make notable contributions with 175, 162, and 119 articles, respectively. These journals have played a crucial role in disseminating research findings and fostering advancements in the study of ambient seismic noise.
Table 5 details the top 10 cited references and their representative authors in the field of ASNR. With a primary focus on the application of broadband ambient seismic noise for surface wave tomography, as well as geological and seismic studies of specific regions, these references present pioneering methods and insights. Notably, the most cited document is “High-resolution surface-wave tomography from ambient seismic noise” by Shapiro (2005), accumulating an impressive 1,598 citations. Shapiro’s study introduces an innovative approach using short-period surface-wave group-speed measurements to construct tomographic images, uncovering deterministic information about the Earth’s crust within ambient seismic noise. The referenced studies similarly utilize ambient seismic noise to investigate crustal structures in specific countries, urban areas, or regions, significantly contributing to our understanding of geographical variations in crustal properties through the valuable data source of ambient seismic noise.
The second reference by Bensen et al. (2007) with 1,590 citations, discusses advanced processing techniques to analyze ambient seismic noise, aiming to measure surface wave dispersion over a wide range of frequencies. Such measurements are crucial for understanding the Earth’s subsurface structure. The third one by utilize data from seismic studies to examine the geological and tectonic history of the Tibetan Plateau.
The third by Royden et al. (2008) highlights the significance of ambient seismic noise in understanding these geological processes. Next, Shapiro and Campillo (2004) reveal that seismic imaging can be enhanced by extracting coherent Rayleigh wave information from ambient seismic noise, using cross-correlation techniques across various station pairs. Yao et al. (2006) introduce a technique using Empirical Green’s Functions derived from ambient seismic noise to better resolve Earth’s crust and upper mantle structures, particularly beneath Southeast Tibet, where it indicates slow shear wave propagation. Hildebrand (2009) discusses how ocean ambient noise varies across frequency bands due to a blend of human and natural activities, with low frequencies dominated by shipping and seismic exploration, mid frequencies by sea-surface actions, and high frequencies by thermal noise. McNamara and Buland (2004) present a new method for characterizing background seismic noise in the continental United States by analyzing power spectral density at various frequencies, aiding in the evaluation and optimization of seismic station performance and network placement. Lin et al. (2008) map Rayleigh and Love wave phase velocities in the western United States using ambient seismic noise from over 250 stations, demonstrating that empirical Green’s functions can be accurately derived from noise cross-correlation, leading to detailed phase speed maps that align with geological structures and validate previous research findings. Li et al. (2008) combine seismic data to image mantle structures under the Tibetan Plateau, revealing that the Indian lithosphere’s northward subduction varies spatially. Utilizing correlations of ambient seismic noise, Brenguier et al. (2008) highlights that large earthquakes in Parkfield, California, notably impact the San Andreas fault zone by inducing long-lasting seismic velocity reductions and heightened nonvolcanic tremor activity, mirroring after slip patterns seen in GPS measurements.
Overall, the top 10 cited references in ASNR collectively showcase the versatile application of ambient seismic noise in both geophysical studies and seismology. These works encompass groundbreaking methods for crustal imaging, employing surface-wave group-speed measurements, and innovative processing techniques for delving into subsurface structures. They cover diverse subjects such as seismic velocity changes due to earthquakes, varying mantle structures, and the analysis of ocean ambient noise. Fundamentally, these studies, using ambient seismic noise, have significantly advanced our understanding of Earth’s crust, mantle structures, and tectonic processes, underscoring the profound value of ambient seismic noise in advancing seismic and geological research.
Figure 3 illustrates the collaboration network in the field of ambient seismic noise research from 1984 to 2023, encompassing 112 nodes and 133 links. Countries such as the United States, Germany, France, and China are positioned at the center of the network, indicating their pivotal roles in global research collaborations. Other countries, including Japan, Switzerland, and Italy, also demonstrate strong collaborative ties, though their influence is slightly less prominent than the central nations. Peripheral countries, such as Egypt, Chile, and Norway, are involved in fewer collaborations. This network visualization highlights the global nature of ambient seismic noise research and underscores the importance of international collaboration in advancing the field. Through the facilitation of partnerships and knowledge exchange, these collaborations contribute to the collective understanding of ambient seismic noise phenomena and its applications across diverse regions.
The collaboration map of research institutes in the field of ASNR, as depicted in Figure 4, consists of 208 nodes, each representing a distinct research institute, connected by 200 links denoting collaborations established between these institutes. This visualization provides insights into the interconnected and collaborative nature of the research landscape within the ASNR field. This visualization underscores the interconnected and collaborative nature of the ASNR research landscape, showcasing the extensive knowledge-sharing and cooperative efforts among institutions worldwide. Prominent institutions such as the Chinese Academy of Sciences, CALTECH, Ist Nazl Geofis & Vulcanol, University of Science and Technology of China, Massachusetts Institute of Technology MIT and China Earthquake Administration are at the forefront of these efforts. Notable contributions from other leading research institutions include University of Grenoble 1, Stanford University, University of California, San Diego, Southern University of Science and Technology and Université Grenoble Alpes. These institutions are crucial in driving innovations in understanding ambient seismic noise phenomena, methodologies, and applications.
Figure 5 depicts the author collaboration network in the domain of ambient seismic noise research, encompassing a total of 913 nodes connected by 1,286 collaboration links. The extensive network signifies the broad range of collaborations among researchers in this field. The collaborative efforts among these authors have significantly contributed to advancing the technique of cross-correlating noise recordings between various stations to extract Green’s function, a method pivotal for accurate imaging of subsurface structures and for real-time monitoring of crustal velocity changes (Brenguier et al., 2008; Campillo and Paul, 2003; Fäh and Havenith, 2010; Parolai, 2009; Ritzwoller, 2009; Roux, 2005; Shapiro, 2005; Yang et al., 2007; Yao et al., 2008; Yao et al., 2006).
In the collaborative network of ambient seismic noise research, Michel Campillo, affiliated with CNRS and the University Grenoble Alpes in France, stands out as the leading contributor with a frequency of 68 collaborations, underscoring his extensive experience of over 3 decades in seismology and ambient noise seismic techniques. His prolific work and collaborations with researchers like Shapiro, Roux, and Brenguier have significantly advanced the field. Yao Huajian from the University of Science and Technology of China follows closely, ranking second. His highly cited articles, such as ‘Surface-wave array tomography in SE Tibet from ambient seismic noise and two-station analysis - I. Phase velocity maps’ and ‘Surface wave array tomography in SE Tibet from ambient seismic noise and two-station analysis - II. Crustal and upper-mantle structure,’ have become seminal references, laying the groundwork for further research in this field. Yang Yingjie from Southern University of Science and Technology, China, ranks third, noted for his research in seismic ambient noise and surface-wave tomography. Other key contributors include Michael H. Ritzwoller from the University of Colorado at Boulder and Chen Xiaofei from Southern University of Science and Technology, both recognized for their advancements in understanding crustal and mantle structures.
The application of co-citation analysis proves to be a valuable method for understanding the academic structure and tracing the evolution of a research field (Che et al., 2022). In this study, a cited reference co-citation analysis was conducted based on the collected documents, resulting in a co-citation map featuring 1,210 nodes and 2,882 links, as illustrated in Figure 6. The nodes in the co-citation map represent the documents of the co-cited references. The size of each node indicates the frequency of citation, with larger nodes representing higher citation frequency. The lines connecting the nodes represent co-citation relationships, meaning that the papers are frequently cited together. The analysis reveals that key papers such as Shapiro and Campillo (2004), Sabra et al. (2005), Bensen et al. (2007), Yao et al. (2006), Yang et al. (2007) and Lin et al. (2008) are highly influential in the field, forming the foundational basis of the research. These papers form clusters that reflect different subfields or themes within the research, illustrating the evolution of studies from the early 2000s to the present. The larger nodes and connections bridging multiple clusters suggest that these papers have played a crucial role in advancing ambient seismic noise research. Figure 6 offers valuable insights into the frequently cited references in the field of ambient seismic noise research.
The co-citation network diagram among authors in ASNR is illustrated in Figure 7. In the network, each node represents an author who has made contributions to the field. The size of each node indicates the frequency of citations received by the author, with larger nodes representing authors who are cited more frequently. The co-citation graph, comprising 1,323 nodes, offers a visual representation of the scholarly connections within the ASNR field. The 4,829 links connecting the nodes indicate co-citation relationships, highlighting the connections and collaborations between authors within the research community. Through the analysis of the co-citation network, it becomes possible to identify influential authors who have been widely cited by their peers. These authors play a significant role in shaping the research landscape and advancing knowledge in the field of ambient seismic noise.
Some of the most frequently cited authors, such as Shapiro NM, Bensen GD, Lin FC, Yao HJ, Yang YJ, Snieder R, Sabra KG, Nakamura Y, and Aki K, published their influential work in the mid to late 2000s and have had a profound impact on the ASNR field. Figure 7 not only reveals the structure and dynamics of academic collaborations within the ASNR domain but also assists researchers in identifying key contributors, influential research directions, and potential opportunities for interdisciplinary collaboration.
CiteSpace provides burst analysis to explore significant changes in citations during a certain period. Figure 8 presents the top 10 references with the most significant citation bursts, as identified by CiteSpace. These references have experienced significant changes in citation frequency during a specific timeframe, signifying their influential and impactful contributions to the field of ambient seismic noise research.
The initial reference is “Long-range correlations in the diffuse seismic coda” published in Science by Campillo and Paul (2003), with a citation burst from 2004 to 2008 and a burst value of 22.91. In this article, Campillo and Paul employed seismic coda analysis of 101 distant earthquakes to unveil coherent information on Earth’s elastic response, demonstrating the potential for capturing direct waves between well-positioned observation points and offering broad applications beyond seismology. The burst period for this reference spanned from 2004 to 2008.
The second reference is the paper “High-resolution surface-wave tomography from ambient seismic noise” published in Science by (Shapiro, 2005), with a burst value of 62.93. In this study, Shapiro introduced the application of cross-correlation of extended sequences of ambient seismic noise to generate tomographic images of the Earth’s interior. The research presented compelling evidence regarding the reliability and cost-effectiveness of utilizing ambient seismic noise for detecting the Earth’s crust. This reference showed a citation burst from 2005 to 2010.
The third reference, with a burst value of 31.39, is “Emergence of broadband Rayleigh waves from correlations of the ambient seismic noise” by Sabra et al. (2005). The authors extracted coherent information about Earth’s structure from ambient seismic noise, revealing clear and consistent dispersive wave trains. Through cross-correlating vertical component records across varying station pairs and distances, the method demonstrates the feasibility of extracting coherent Rayleigh waves and measuring their dispersion characteristics, introducing a novel and expansive source for surface-wave measurements. This reference experienced a burst from 2006 to 2010.
The fourth reference, titled “Processing seismic ambient noise data to obtain reliable broad-band surface wave dispersion measurements” by Bensen et al. (2007), with a citation burst from 2008 to 2012 and a burst value of 54.76, explored Earth’s structure imaging using ambient noise. The authors extracted surface wave arrival times from seismic data collected at 148 broadband stations in Southern California. Employing a straightforward tomographic procedure, the authors successfully estimated surface wave velocity structure for the region, validating that the coherent noise field between station pairs can be utilized for seismic imaging purposes.
The fifth reference, “Surface-Wave Tomography from Ambient Seismic Noise in Southern California” by Yao et al. (2006), presented a significant burst value of 23.08 with a citation burst from 2009 to 2013. This study was primarily centered on the integration of Rayleigh-wave dispersion estimates derived from empirical Green’s functions and two-station analysis, paving the way for high-resolution surface-wave array tomography of the continental lithosphere.
Another significant contribution is from Lin and Ritzwoller (2011), who presented methods for surface wave tomography using ambient seismic noise. The research, cited prominently from 2013 to 2016 with a burst value of 11.63, highlighted the advancements in processing techniques for extracting reliable surface wave data. Moving to more recent influential works, the study by Fichtner et al. (2017), titled “Seismic Noise Correlation on Heterogeneous Supercomputers” has shown significant impact with a burst value of 13.4 and a burst period from 2017 to 2023, indicating its ongoing relevance in the field. The study focused on improving imaging techniques using ambient noise data.
Similarly, Lecocq et al. (2017) presented advancements in ambient noise processing, emphasizing the enhancement of seismic imaging techniques. This reference experienced a burst period from 2019 to 2023 with a burst value of 11.54, reflecting its importance in modern seismic studies. Wang et al. (2017) also made significant contributions with a focus on utilizing ambient seismic noise for subsurface imaging, which was widely cited from 2019 to 2023. The citation burst value for this reference is 14.85, showcasing its role in advancing seismic noise research. Lastly, the study by Wathelet et al. (2020) represents the most recent influential work, with a burst value of 28.84 and a burst period extending from 2021 to 2023, emphasizes the application of ambient noise in seismic imaging, underlining its importance in the continuous evolution of geophysical methods.
These references have made substantial impacts in the realm of ambient seismic noise research, as evidenced by their widespread citation by other scholars. This underscores their pivotal role in advancing both the understanding and application of ambient seismic noise in a variety of geophysical studies.
Co-citation analysis uncovers the knowledge base and development background in a certain research field providing insights into the knowledge structure, academic research trends and future directions. Clustering as a key part of document co-citation analysis helps in understanding research evolution trends. The clustering capabilities was utilized to analyze the cited publications, aiming to discern the primary keyword clusters for each respective year. This methodical approach unveiled nine predominant clusters, each encapsulating a distinct facet of foundational knowledge in ambient seismic noise research (refer to Figure 9). The modularity Q of the network is 0.8444, which indicates that the various research fields of ASNR can be clearly defined (Chen, 2017). The Mean Silhouette score of 0.9331 further suggests that the clusters within this field are uniformly and cohesively defined, demonstrating the clear categorization of research topics in ASNR.
ASNR has experienced significant development in recent years, with various research themes emerging and evolving over time. Figure 9 illustrates the distribution of co-cited articles clusters along a timeline. Although relevant articles date back to 1984, it was not until around the year of 2000 sufficient volumes of ASNR research emerged to form meaningful clusters. These clusters, represented by different colors and numbers, each signify a group of studies that are frequently cited together, reflecting interconnected research directions within the field.
Upon further analysis, these clusters can be categorized into three main research directions. The first direction, wave propagation and imaging techniques, includes research clusters such as Seismic Velocity (Cluster #2), Greens Function (Cluster #4), Linear Receiver Array (Cluster #3), Ambient Vibration (Cluster #5), and To-Vertical Spectral Ratio (Cluster #6). Seismic Velocity (Cluster #2) spans from 2005 to 2022 and deals with the analysis and interpretation of seismic wave velocities. Keywords such as seismic velocity models, velocity inversion, and shear wave velocity are prevalent. The research in this group is largely focused on building accurate models of the Earth’s subsurface, critical for understanding seismic hazards and for oil and gas exploration. Greens Function (Cluster #4), emphasizes the mathematical formulation used to describe seismic wave propagation. It is associated with terms like Greens function, seismic response modeling, and earthquake simulation. The research focuses on applying Greens functions to simulate how seismic waves travel through different geological structures. Linear Receiver Array (Cluster #3), appearing around 2012 and continuing until 2022, this cluster revolves around the deployment and use of linear arrays of seismic receivers to capture detailed seismic waveforms. Key terms include “linear array configuration”, “seismic data acquisition” and “waveform analysis.” This approach is often used to study seismic wave propagation and to improve the precision of earthquake localization and subsurface imaging. Ambient Vibration (Cluster #5) focuses on the study of ambient vibrations and their use in characterizing subsurface structures. The research is often tied to keywords such as “ambient noise analysis”, “microtremor studies”, and “vibration monitoring”. This field of research uses background seismic noise, often from human activity or natural phenomena, to map out subsurface features without the need for active seismic sources. To-Vertical Spectral Ratio (Cluster #6), spanning from around 2002 to 2022, deals with the calculation and analysis of horizontal-to-vertical spectral ratios (HVSR) from seismic data. Keywords like “HVSR method”, “spectral analysis” and “site response” dominate this group. This technique is used to assess the resonance frequencies of different ground layers, crucial for earthquake engineering and understanding how different regions might respond to seismic shaking.
The second direction, risk assessment and monitoring, involves clusters related to the monitoring of seismic activities and the analysis of risks such as landslides. This includes clusters like Seismic Damage Distribution (Cluster #9), Using Correlation (Cluster #8), and Tanlu Fault Zone (Cluster #7). This direction emphasizes assessing and monitoring seismic risks, particularly in relation to fault zones and the distribution of seismic damage. For example, Seismic Damage Distribution (Cluster #9), spanning from 2000, focuses on the study of seismic damage patterns and their implications for infrastructure resilience. The research highlights the importance of understanding damage patterns to improve building codes and design safer structures in earthquake-prone areas. Using Correlation (Cluster #8), starting around 2002, emphasizes the use of seismic correlation techniques, particularly in passive seismic data analysis. The cluster reflects the growing use of cross-correlation methods to enhance seismic data interpretation, particularly for understanding wavefield propagation and subsurface structure. Tanlu Fault Zone (Cluster #7), emerging in the 2010s, concentrates on research related to the Tanlu Fault Zone, one of China’s most significant fault systems. The cluster includes studies on fault dynamics, seismic risk assessment, and fault-related seismic activity. The Tanlu Fault is a major subject of interest due to its potential for generating significant earthquakes in the region.
The final direction, geophysical studies and applications, encompasses clusters such as Northeast China (Cluster #0) and seismic noise correlation (Cluster #1). The clusters are focused on the application of seismic noise correlation in geophysical studies to explore Earth’s structures and processes. For instance, Northeast China (Cluster #0), appearing around 2009, is centered on studies related to the tectonic activities and seismic properties of the Northeast China region. The primary focus is on understanding fault dynamics, seismic hazard assessment, and earthquake occurrences in the region. The cluster reflects growing interest in seismic risk and geophysical analysis in this particular region. And Seismic Noise Correlation (Cluster #1) focuses on utilizing ambient seismic noise for understanding subsurface structures and detecting changes in the Earth’s crust. The studies emphasize the non-intrusive analysis of seismic data to reveal insights into the Earth’s interior, often for purposes like monitoring fault zones and volcanoes.
Additionally, the clustering of co-cited articles not only provides a clear categorization but also highlights influential authors and their works from specific years within each cluster. This visualization allows us to see the evolution of ideas and the impact of key contributors in shaping the research landscape within these clusters.
Clusters of ambient seismic noise research based on the terms shown Figure 10 reflect an evolving landscape from the 1980s to November 2023, categorized into distinct yet interconnected research fields.
The clusters of ASNR, as summarized in 10 clusters provided in Figure 10, outline a comprehensive picture of the evolving research landscape from the 1980s to November 2023. This research can be broadly categorized into three key areas, each representing distinct yet interconnected research fields.
The field of geophysical techniques has seen significant advancements, particularly in seismic tomography and wave propagation studies. This is highlighted by clusters such as Ambient Noise Tomography (Cluster #1), Seismic Velocity (Cluster #6), and Ambient Seismic Noise (Cluster #8), which emphasize the progress in imaging the Earth’s subsurface. These technological advancements allow for deeper and more precise studies, contributing to a better understanding of geological structures through improved seismic methodologies.
In addition to these technological innovations, there has been a shift towards environmental and site-specific studies, as seen in clusters focused on Shale Play Site (Cluster #2), Site Effect (Cluster #4), Crustal Structure (Cluster #5), and Uppermost Mantle Structure (Cluster #7). These studies are particularly relevant for understanding the localized geological factors that influence seismic activity. The findings from these clusters are crucial for applications in engineering, hazard mitigation, and seismic risk assessment, providing valuable insights for designing structures that can withstand seismic forces.
Moreover, the importance of seismic monitoring and impact analysis is underscored by research in clusters such as Ambient Noise (Cluster #0), Receiver Function (Cluster #3), and Ambient Noise Measurement (Cluster #9). These studies focus on differentiating between natural and anthropogenic seismic sources and on enhancing real-time monitoring systems. The development of these monitoring systems is vital for disaster preparedness and for studying environmental seismicity, reflecting the growing need for accurate and timely data to respond to seismic events effectively.
The thematic clusters identified in ASNR based on the terms illustrate a trajectory that prioritizes technological innovation, environmental applications, and the practical needs of real-time monitoring. The interconnection between refining imaging techniques, understanding site-specific seismic responses, and improving monitoring capabilities demonstrates the geophysical research community’s ongoing commitment to addressing the complexities of Earth’s seismic activities.
The field of ASNR has demonstrated considerable growth, as reflected in the increasing volume of articles and the formation of thematic clusters that highlight a deeper engagement with advanced geophysical techniques. The non-invasive and cost-effective nature of ASNR has made it particularly advantageous, allowing for the exploration of the Earth’s subsurface without the need for active seismic sources such as controlled explosions or vibrating plates. This aspect has expanded ASNR’s applicability across various geophysical and environmental contexts, making it a versatile tool for contemporary research. The non-invasive approach is especially beneficial in settings where traditional seismic methods pose logistical challenges or environmental risks, such as in urban areas or protected natural regions, where deploying active seismic sources is often impractical. Moreover, the cost-effectiveness of ASNR enables continuous monitoring over extended periods, providing high temporal resolution that is essential for detecting subtle changes in subsurface properties.
Despite its numerous advantages, ASNR is not without limitations. One of the significant challenges is its reliance on the quality and distribution of ambient noise sources. In regions where ambient seismic noise is weak or irregular, the effectiveness of ASNR can be severely compromised, resulting in lower resolution or less reliable results. Furthermore, the method is sensitive to both environmental and anthropogenic noise, which can introduce biases or obscure the seismic signals of interest. This limitation is particularly pronounced in highly urbanized or industrial areas, where noise pollution can significantly interfere with data collection, necessitating advanced filtering and noise-correction techniques.
The future research trends in ASNR can be discussed across four main fields, imaging techniques, disaster detection, anthropogenic noise studies, and the integration of artificial intelligence. These areas have seen significant development in the recent years and provide new perspectives for future research.
Imaging techniques using ambient noise data have been widely applied in ASNR, particularly in revealing subsurface structures and seismic wave propagation characteristics. Noise imaging leverages continuous background noise to reconstruct subsurface structures, offering higher resolution and stability compared to traditional seismic imaging methods (Kumar et al., 2021; Mulumulu et al., 2023). Future research is expected to further refine noise imaging algorithms to improve imaging accuracy in challenging geological settings, thereby providing more detailed data to support earthquake prediction and disaster mitigation. These advancements in noise imaging technologies hold significant value for researchers interested in improving geophysical exploration and enhancing seismic hazard monitoring.
ASNR plays a critical role in real-time monitoring of seismic and volcanic activities (Hou et al., 2023; Massa et al., 2022). Petrosino and De Siena (2021) highlighted the role of ambient seismic noise in monitoring fluid migrations and detecting seismic activity within stressed volcanic systems, offering a near real-time method for tracking changes with minimal data processing. With advancements in data processing technologies, ASNR is expected to play an even more significant role in disaster early warning and emergency response, especially in real-time monitoring and prediction of geological hazards. The function provides crucial insights for those seeking to enhance their understanding of geological processes and their potential risks.
ASNR has also shown great potential in studying the impact of human activities on seismic noise (Lecocq et al., 2020; Wu et al., 2020). Lecocq et al. (2020) studied the significant reduction in global seismic noise during the COVID-19 pandemic, demonstrating ASNR’s ability to monitor the relationship between human activities and environmental noise. Future research in this area will focus on systematically understanding the impact of factors such as urbanization and industrial activities on seismic noise, providing data support for urban planning and environmental protection (Diaz et al., 2020). Yabe et al. (2020) showed the potential of using ASNR to monitor urban noise sources in densely populated areas, paving the way for future applications of ASNR in urban environmental monitoring, while Dando et al. (2023) showcased the use of seismic array data to detect attacks in the Russia-Ukraine conflict, highlighting ASNR’s precision in tracking military actions. This underscores the value of ASNR to earth scientists, geophysicists and even urban researchers, providing them with a powerful tool for understanding the environmental impact of human activities. By integrating ASNR with other technologies, researchers can gain critical insights into how human activities influence seismic noise patterns, aiding in the development of more accurate environmental and geophysical models.
The integration of artificial intelligence (AI) with ASNR has already begun to transform research in this field. The introduction of machine learning algorithms has significantly improved data processing efficiency, making it possible to extract valuable information from large and complex datasets (Qadri and Malik, 2021; Rincon-Yanez et al., 2022; Song et al., 2023). Mousavi et al. (2020) successfully used deep learning models to classify and predict seismic events, demonstrating the critical role AI technology can play in seismic data analysis. In the future, as big data and cloud computing technologies develop, ASNR is expected to leverage global collaboration platforms for data sharing and interdisciplinary research, further expanding its applications across diverse fields.
The progression of Ambient Seismic Noise Research from 1984 to 2023, as documented in this study, reveals a field maturing through collaboration and technological innovation. The data, methodically analyzed using CiteSpace software, exhibits a marked increase in ASNR publications, indicating a heightened global interest and a collective effort towards understanding seismic phenomena.
The results revealed the progress and advancements made in ambient seismic noise research over the years. By examining various aspects such as publication trends, subject categories, collaboration networks, top authors, and highly cited references, this research gained a holistic understanding of the field’s development history.
Furthermore, the increasing importance of ambient seismic noise in various applications was highlighted, including velocity model inversion, earthquake detection, and ground motion prediction. This indicates the growing recognition of ambient seismic noise as a valuable tool in assisting seismic studies and improving our understanding of Earth’s subsurface.
The discerned clusters convey advancements across three main themes: geophysical techniques, environmental and site-specific studies, and monitoring and impact analysis. Geophysical Techniques, with clusters like Ambient Noise Tomography and Seismic Velocity, show an emphasis on refining imaging methods. This focus aligns with technological strides in analyzing seismic wave behavior for deeper insights into Earth’s subsurface. Environmental and site-specific studies, represented by clusters such as Site Effect and Crustal Structure, reflect a nuanced approach to how local geology influences seismic responses, critical for seismic hazard mitigation. Monitoring and impact analysis, with clusters like Ambient Noise and Receiver Function underscore the vital role of real-time data in distinguishing seismic sources, demonstrating the importance of advancements in surveillance technology for risk management.
In conclusion, ASNR is characterized by a robust, interdisciplinary research landscape that has steadily grown, evidenced by the comprehensive collaboration networks among countries and institutions. The study’s findings, particularly the citation bursts and keyword clusters, not only highlight the field’s key contributors and significant studies but also suggest a research trajectory prioritizing detailed imaging, localized geological understanding, and the practicality of monitoring systems. This trajectory underscores ASNR’s potential to contribute significantly to earthquake preparedness, geophysical exploration, and our broader understanding of Earth’s dynamic processes.
The original contributions presented in the study are included in the article/supplementary material, further inquiries can be directed to the corresponding author.
LW: Conceptualization, Investigation, Methodology, Software, Visualization, Writing–original draft, Writing–review and editing. JL: Conceptualization, Data curation, Methodology, Supervision, Validation, Writing–original draft. SB: Validation, Visualization, Writing–review and editing. QG: Software, Writing–review and editing.
The author(s) declare that financial support was received for the research, authorship, and/or publication of this article. This work was supported by the Scientific Research Foundation of the Education Department of Hubei Province, China under grant number Q20203004 and the Philosophy and Social Science Research Project of the Education Department of Hubei Province, China under grant number 22Q212.
The authors declare that the research was conducted in the absence of any commercial or financial relationships that could be construed as a potential conflict of interest.
All claims expressed in this article are solely those of the authors and do not necessarily represent those of their affiliated organizations, or those of the publisher, the editors and the reviewers. Any product that may be evaluated in this article, or claim that may be made by its manufacturer, is not guaranteed or endorsed by the publisher.
Aki, K. (1957). Space and time spectra of stationary stochastic waves, with special reference to microtremors. Bull. Earthq. Res. Inst. 35, 415–456.
Bensen, G. D., Ritzwoller, M. H., Barmin, M. P., Levshin, A. L., Lin, F., Moschetti, M. P., et al. (2007). Processing seismic ambient noise data to obtain reliable broad-band surface wave dispersion measurements. Geophys. J. Int. 169 (3), 1239–1260. doi:10.1111/j.1365-246X.2007.03374.x
Brenguier, F., Campillo, M., Hadziioannou, C., Shapiro, N. M., Nadeau, R. M., and Larose, E. (2008). Postseismic relaxation along the San Andreas fault at Parkfield from continuous seismological observations. Science 321 (5895), 1478–1481. doi:10.1126/science.1160943
Brenguier, F., Shapiro, N. M., Campillo, M., Ferrazzini, V., Duputel, Z., Coutant, O., et al. (2008). Towards forecasting volcanic eruptions using seismic noise. Nat. Geosci. 1 (2), 126–130. doi:10.1038/ngeo104
Byrne (1961). Instrument noise in seismometers. Bull. Seismol. Soc. Am. 51 (1), 69–84. doi:10.1785/BSSA0510010069
Campillo, M., and Paul, A. (2003). Long-range correlations in the diffuse seismic coda. Science 547-549 (5606), 547–549. doi:10.1126/science.1078551
Che, S. P. K., Zhang, S., Zhao, X., and Kim, J. H. (2022). A visualization analysis of crisis and risk communication research using CiteSpace. Int. J. Environ. Res. Public Health 19 (5), 2923. doi:10.3390/ijerph19052923
Chen, C. (2004). Searching for intellectual turning points: progressive knowledge domain visualization. Proc. Natl. Acad. Sci. 101 (Suppl. 1), 5303–5310. doi:10.1073/pnas.0307513100
Chen, C. (2006). CiteSpace II: detecting and visualizing emerging trends and transient patterns in scientific literature. J. Am. Soc. Inf. Sci. Technol. 57 (3), 359–377. doi:10.1002/asi.20317
Chen, C. (2017). Science mapping: a systematic review of the literature, J. data Inf. Sci., 2(2), 1. 40. doi:10.1515/jdis-2017-0006
Chen, W., Rao, G., Kang, D., Wan, Z., and Wang, D. (2023). Early report of the source characteristics, ground motions, and casualty estimates of the 2023 M w 7.8 and 7.5 Turkey earthquakes. J. Earth Sci. 34 (2), 297–303. doi:10.1007/s12583-023-1316-6
Chen, W., Wang, D., Zhang, C., Yao, Q., and Si, H. (2022). Estimating seismic intensity maps of the 2021 M w 7.3 Madoi, Qinghai and M w 6.1 Yangbi, Yunnan, China earthquakes. J. Earth Sci. 33 (4), 839–846. doi:10.1007/s12583-021-1586-9
Dando, B. D., Goertz-Allmann, B. P., Brissaud, Q., Köhler, A., Schweitzer, J., Kværna, T., et al. (2023). Identifying attacks in the Russia–Ukraine conflict using seismic array data. Nature 621 (7980), 767–772. doi:10.1038/s41586-023-06416-7
Diaz, J., Schimmel, M., Ruiz, M., and Carbonell, R. (2020). Seismometers within cities: a tool to connect earth sciences and society. Front. Earth Sci. 8, 9. doi:10.3389/feart.2020.00009
Fäh, D. S., and Havenith, H. B. (2010). Analysis of three-component ambient vibration array measurements, Geophys. J. R. Astronomical Soc., 172(1), 199–213. doi:10.1111/j.1365-246X.2007.03625.x
Fang, L., Wu, J., Ding, Z., and Panza, G. F. (2010). High resolution Rayleigh wave group velocity tomography in North China from ambient seismic noise, Geophys. J. Int., (2), 1171–1182. doi:10.1111/j.1365-246X.2010.04571.x
Fichtner, A., Ermert, L., and Gokhberg, A. (2017). Seismic noise correlation on heterogeneous supercomputers. Seismol. Res. Lett. 88 (4), 1141–1145. doi:10.1785/0220170043
Haubrich, R. A. (1965). Earth noise, 5 to 500 millicycles per second:1. Spectral stationarity, normality, and nonlinearity. J. Geophys. Res. Atmos. 70 (6), 1415–1427. doi:10.1029/JZ070i006p01415
Hildebrand, J. A. (2009). Anthropogenic and natural sources of ambient noise in the ocean, Mar. Ecol. Prog. Ser., 395, 5–20. doi:10.3354/meps08353
Hou, J., Wu, Q., Yu, D., Ye, Q., and Zhang, R. (2023). Study on surface-wave tomography in Abaga volcanic area, Inner Mongolia. Front. Earth Sci. 11, 1131393. doi:10.3389/feart.2023.1131393
Kumar, A., Kumar, N., Mukhopadhyay, S., and Klemperer, S. L. (2021). Tomographic image of shear wave structure of NE India based on analysis of Rayleigh wave data. Front. Earth Sci. 9, 680361. doi:10.3389/feart.2021.680361
Lecocq, T., Hicks, S. P., Van Noten, K., Van Wijk, K., Koelemeijer, P., De Plaen, R. S., et al. (2020). Global quieting of high-frequency seismic noise due to COVID-19 pandemic lockdown measures. Science 369 (6509), 1338–1343. doi:10.1126/science.abd2438
Lecocq, T., Longuevergne, L., Pedersen, H. A., Brenguier, F., and Stammler, K. (2017). Monitoring ground water storage at mesoscale using seismic noise: 30 years of continuous observation and thermo-elastic and hydrological modeling. Sci. Rep. 7 (1), 14241. doi:10.1038/s41598-017-14468-9
Li, C., Van der Hilst, R. D., Meltzer, A. S., and Engdahl, E. R. (2008). Subduction of the Indian lithosphere beneath the Tibetan plateau and Burma, Earth Planet. Sci. Lett., 274(1-2), 157–168. doi:10.1016/j.epsl.2008.07.016
Li, H., Su, W., Wang, C., and Huang, Z. (2009). Ambient noise Rayleigh wave tomography in western Sichuan and eastern Tibet, Earth Planet. Sci. Lett., 282(1/4), 201–211. doi:10.1016/j.epsl.2009.03.021
Lin, F.-C., Moschetti, M. P., and Ritzwoller, M. H. (2008). Surface wave tomography of the western United States from ambient seismic noise: Rayleigh and Love wave phase velocity maps, Geophys. J. Int., 173(1), 281–298.doi:10.1111/j.1365-246X.2008.03720.x
Lin, F.-C., and Ritzwoller, M. H. J. G. J. I. (2011). Helmholtz surface wave tomography for isotropic and azimuthally anisotropic structure. Geophys. J. Int. 186 (3), 1104–1120. doi:10.1111/j.1365-246x.2011.05070.x
Massa, M., Lovati, S., Puglia, R., Brunelli, G., Lorenzetti, A., Mascandola, C., et al. (2022). Seismo-stratigraphic model for the urban area of milan (Italy) by ambient-vibration monitoring and implications for seismic site effects assessment. Front. Earth Sci. 10, 915083. doi:10.3389/feart.2022.915083
McNamara, D. E., and Buland, R. P. (2004). Ambient noise levels in the continental United States, Bull. Seismol. Soc. Am., 94, 1517, 1527. doi:10.1785/012003001
Mousavi, S. M., Ellsworth, W. L., Zhu, W., Chuang, L. Y., and Beroza, G. C. (2020). Earthquake transformer—an attentive deep-learning model for simultaneous earthquake detection and phase picking. Nat. Commun. 11 (1), 3952. doi:10.1038/s41467-020-17591-w
Mulumulu, E., Polat, O., and Chávez-García, F. J. (2023). Ambient noise tomography of the Aegean region of Türkiye from Rayleigh wave group velocity. Front. Earth Sci. 11, 1265986. doi:10.3389/feart.2023.1265986
Parolai, S. (2009). Determination of dispersive phase velocities by complex seismic trace analysis of surface waves (CASW), Soil Dyn. Earthq. Eng., 29(3), 517–524. doi:10.1016/j.soildyn.2008.05.008
Peterson, J. (1993). Observations and modeling of seismic background noise, 93-322. Albuquerque, New Mexico: U.S.geological survey.
Petrosino, S., and De Siena, L. (2021). Fluid migrations and volcanic earthquakes from depolarized ambient noise. Nat. Commun. 12 (1), 6656. doi:10.1038/s41467-021-26954-w
Qadri, S. T., and Malik, O. A. (2021). Establishing site response-based micro-zonation by applying machine learning techniques on ambient noise data: a case study from Northern Potwar Region, Pakistan. Environ. Earth Sci. 80, 53–15. doi:10.1007/s12665-020-09322-7
Rincon-Yanez, D., De Lauro, E., Petrosino, S., Senatore, S., and Falanga, M. (2022). Identifying the fingerprint of a volcano in the background seismic noise from machine learning-based approach. Appl. Sci. 12 (14), 6835. doi:10.3390/app12146835
Ritzwoller, M. H. (2009). Ambient noise seismic imaging, Recent Dev. World Seismol., 9(31), 315–328. doi:10.1036/1097-8542.YB090130
Roux, P., Sabra, K. G., Gerstoft, P., Kuperman, W. A., and Fehler, M. C. (2005). P-waves from cross-correlation of seismic noise, Geophys. Res. Lett., 32(19), 312–321. doi:10.1029/2005GL023803
Royden, L. H., Burchfiel, B. C., and van der Hilst, R. D. (2008). The geological evolution of the Tibetan Plateau, Science, 321(5892), 1054–1058. doi:10.1126/science.1155371
Sabra, K. G., Gerstoft, P., Roux, P., Kuperman, W. A., and Fehler, M. C. (2005). Extracting time-domain Green's function estimates from ambient seismic noise, Geophys. Res. Lett., 32(3). doi:10.1029/2004GL021862
Sabra, K. G., Gerstoft, P., Roux, P., Kuperman, W. A., and Fehler, M. C. (2005). Surface wave tomography from microseisms in Southern California, Geophys. Res. Lett., 32(14). doi:10.1029/2005GL023155
Shapiro, N. M., and Campillo, M. (2004). Emergence of broadband Rayleigh waves from correlations of the ambient seismic noise, Geophys. Res. Lett., 31(7). doi:10.1029/2004GL019491
Shapiro, N. M., Campillo, M., Stehly, L., and Ritzwoller, M. H. (2005). High-resolution surface-wave tomography from ambient seismic noise, Science, 307(5715), 1615–1618. doi:10.1126/science.1108339
Smith, R. J., Pérez-Cota, F., Marques, L., and Clark, M. (2021). 3D phonon microscopy with sub-micron axial-resolution. Sci. Rep. 11 (1), 3301. doi:10.1038/s41598-021-82639-w
Song, Y., Lee, J., Yeeh, Z., Kim, M., and Byun, J. (2023). Improved lithospheric seismic velocity and density model of the Korean Peninsula from ambient seismic noise data using machine learning. J. Asian Earth Sci. 254, 105728. doi:10.1016/j.jseaes.2023.105728
Wang, Q. Y., Brenguier, F., Campillo, M., Lecointre, A., Takeda, T., and Aoki, Y. (2017). Seasonal crustal seismic velocity changes throughout Japan. J. Geophys. Res. Solid Earth 122 (10), 7987–8002. doi:10.1002/2017jb014307
Wathelet, M., Chatelain, J. L., Cornou, C., Giulio, G. D., Guillier, B., Ohrnberger, M., et al. (2020). Geopsy: a user-friendly open-source tool set for ambient vibration processing. Seismol. Res. Lett. 91 (3), 1878–1889. doi:10.1785/0220190360
Wu, L., Wang, D., Lei, Z., Fu, J., Min, S., Xu, X., et al. (2020). Campus vibration in nanwangshan campus, China university of geosciences at wuhan monitored by short-period seismometers. J. Earth Sci. 31, 950–956. doi:10.1007/s12583-020-1332-8
Yabe, S., Imanishi, K., and andNishida, K. (2020). Two-step seismic noise reduction caused by COVID-19 induced reduction in social activity in metropolitan Tokyo, Japan. Earth, Planetssp. 72, 167–211. doi:10.1186/s40623-020-01298-9
Yang, Y., Ritzwoller, M. H., Levshin, A. L., and Shapiro, N. M. (2007). Ambient noise Rayleigh wave tomography across Europe, Geophys. J. R. Astronomical Soc., 168(1), 259–274. doi:10.1111/j.1365-246X.2006.03203.x
Yao, H., Beghein, C., and Hilst, R. (2008). Surface wave array tomography in SE Tibet from ambient seismic noise and two-station analysis - II. Crustal upper-mantle Struct., 173(1), 205–219. doi:10.1111/j.1365-246X.2007.03696.x
Yao, H., Hilst, R., and Hoop, M. (2006). Surface wave array tomography in SE Tibet from ambient seismic noise and two:tation analysis – I. Phase velocity maps, Geophys. J. Int., 166. 732, 744. doi:10.1111/j.1365-246X.2006.03028.x
Zang, X., Zhu, Y., Zhong, Y., and Chu, T. (2022). CiteSpace-Based bibliometric review of pickup and delivery problem from 1995 to 2021, Appl. Sci., 12(9), 4607. doi:10.3390/app12094607
Zhang, Y., Wang, R., Zschau, J., Chen, Y. t., Parolai, S., and Dahm, T. (2014). Automatic imaging of earthquake rupture processes by iterative deconvolution and stacking of high-rate GPS and strong motion seismograms. J. Geophys. Res. Solid Earth 119 (7), 5633–5650. doi:10.1002/2013jb010469
Keywords: ambient seismic noise, bibliometric analysis, earth science, hotspots, research trend
Citation: Wu L, Li J, Bao S and Gong Q (2024) Visualization analysis of ambient seismic noise research. Front. Earth Sci. 12:1452324. doi: 10.3389/feart.2024.1452324
Received: 20 June 2024; Accepted: 28 October 2024;
Published: 07 November 2024.
Edited by:
Mourad Bezzeghoud, Universidade de Évora, PortugalReviewed by:
Wei Zhang, Nanjing University, ChinaCopyright © 2024 Wu, Li, Bao and Gong. This is an open-access article distributed under the terms of the Creative Commons Attribution License (CC BY). The use, distribution or reproduction in other forums is permitted, provided the original author(s) and the copyright owner(s) are credited and that the original publication in this journal is cited, in accordance with accepted academic practice. No use, distribution or reproduction is permitted which does not comply with these terms.
*Correspondence: Jinling Li, ZWJfMjAwNGFuZDIwMDVAMTI2LmNvbQ==
Disclaimer: All claims expressed in this article are solely those of the authors and do not necessarily represent those of their affiliated organizations, or those of the publisher, the editors and the reviewers. Any product that may be evaluated in this article or claim that may be made by its manufacturer is not guaranteed or endorsed by the publisher.
Research integrity at Frontiers
Learn more about the work of our research integrity team to safeguard the quality of each article we publish.