- 1School of Geosciences and Technology, Southwest Petroleum University, Chengdu, China
- 2Exploration and Development Research Institute, PetroChina Southwest Oil & Gasfield Company, Chengdu, Sichuan, China
Deep carbonate reservoirs are attractive targets for gas development. These reservoirs are deeply buried, and commonly possess strong heterogeneity and poor seismic data quality, making the identification of favorable production areas (“sweet spots”) challenging. Furthermore, sedimentary facies and fault systems markedly impact reservoir quality, and identifying these features in seismic data is also crucial for sweet spot identification. To solve these problems, we propose a dual-factor-controlled sweet spot identification method with two steps. First, sedimentary facies and faults are identified separately at different seismic scales using different attributes by the steerable pyramid (SP) method. The SP method decomposes the original seismic data into high-frequency and low-frequency data. The amplitude attributes from high-frequency data are used to identify sedimentary facies, and coherence attributes based on low-frequency data are used to characterize the fault systems. Second, after separately identifying the sedimentary facies and faults, the two attribute volumes are merged together to identify reservoir sweet spots. The results are verified by using well production data. The results of a field study in the Dengying Formation deep carbonate reservoir in the central Sichuan Basin, China, indicate that reservoir sweet spots are primarily developed in ideal sedimentary facies along strike-slip fault systems. Sedimentary facies generally control the type and distribution of reservoirs, whereas strike-slip fault systems control the migration and accumulation of gas. In addition, the fault systems serve as karst channels that further improve the reservoir properties. The proposed dual-factor method might help to maximize exploration potential in deep carbonate reservoirs with similar settings.
1 Introduction
Carbonate reservoirs are extremely important for oil and gas development, because they host more than 60% of the world’s oil reserves and 40% of gas reserves (Hendry et al., 2021). In recent years, deep carbonate reservoirs (burial depth >3,500 m) have become important targets for hydrocarbon production, such as the pre-salt carbonate reservoir in Brazil, Lower Cretaceous carbonates in Venezuela, and the Sichuan Basin and Tarim Basin carbonates in China (Pppelreiter et al., 2005; Carvalho et al., 2022; Shi et al., 2023). Seismic exploration is commonly applied to identify reservoir distribution and delineate reservoir structure; however, the deep burial depth of reservoirs often results in weak seismic reflections and low seismic resolution, leading to poor seismic data quality (Pan et al., 2020; Chen et al., 2021). Marine carbonate reservoirs generally have strong heterogeneity, making their geophysical response characteristics complex and variable (Azerêdo et al., 2021; Wang et al., 2022). The poor data quality and strong heterogeneity of carbonate reservoirs are important challenges for reservoir sweet spot identification. Additionally, the quality of deep carbonate reservoirs is commonly controlled by various factors, including the depositional environment, lithology, diagenesis, karstification, and tectonic deformation (Massaro et al., 2018; Tian et al., 2020; Wadas et al., 2023). A good understanding of these factors is required for successful reservoir identification (Wang et al., 2024). In general, the main tasks for identifying deep carbonate reservoirs are to improve the seismic data quality and to consider the main reservoir controlling factors.
There are multiple ways to improve seismic data quality, including denoising, deconvolution and frequency decomposition (Naghizadeh, 2012; Liu and Fomel, 2013; Li et al., 2022). Frequency decomposition is a crucial method for post-stack seismic data processing: the method decomposes the original seismic signals into their constituent frequency components (Liu and Fomel, 2013; Chopra and Marfurt, 2016). This technique can enhance the resolution and interpretation of seismic data, allowing geoscientists to better understand subsurface structures and properties. The method has been applied to identify deep carbonate reservoirs (Naseer and Asim, 2018; Xu et al., 2019), but its stability still needs to be improved. Another seismic data processing method is the steerable pyramid method, which is commonly used to detect channels and thin sand bodies in sandstone reservoirs (Mathewson and Hale, 2008; Zhao et al., 2021).
Extensive research has suggested that the sedimentary environment (ideal sedimentary facies) is one of the most important factors controlling the reservoir quality of deep carbonates (Shen et al., 2008; Hairabian et al., 2014; Luo et al., 2015; Azerêdo et al., 2021; Nabawy et al., 2023). Luo et al. (2015) proposed that the deep carbonate reservoirs in the Sichuan Basin are primarily controlled by mound–shoal facies, with effective identification of mound–shoal complexes and accurate restoration of karst paleo-geomorphology being crucial for reservoir characterization. Shen et al. (2008) suggested that carbonate reef complexes are the ideal reservoir facies for platform-margin depositional environments in both Western Australia and South China. Nabawy et al. (2023) concluded that there are three favorable microfacies of Upper Cretaceous carbonates in the Gulf of Suez, and demonstrated that diagenetic modifications, including fracturing, dissolution and dolomitization, have enhanced the reservoir facies properties and quality.
In recent studies, it was found that strike-slip fault systems also control and modify carbonate reservoirs (Jiao et al., 2021; Jia et al., 2022; He et al., 2023; Ma et al., 2023). Jiao et al. (2021) concluded that the large strike-slip fault zone in the central Sichuan Basin can effectively connect hydrocarbon source centers, enhancing hydrocarbon migration and forming favorable fault-controlled natural gas reservoirs. Jia et al. (2022) indicated that strike–slip faults control the development of carbonate reservoirs and the enrichment of oil and gas in the Tarim Basin. He et al. (2023) proposed that strike-slip fault zones develop fractures and karstic cavities, which markedly improve the petrophysical properties of carbonate reservoirs.
Previous studies have achieved considerable progress in analyzing reservoir control factors and have produced innovative reservoir identification methods (Lien Eide et al., 2002; Lucia et al., 2003; Ahr, 2011; Malki et al., 2023; Sarhan, 2024). However, most of these studies applied a single control factor for seismic reservoir identification, and only a few studies comprehensively integrated multiple factors to constrain the identification. For practical application, we found that reservoir identification based on single factors often fails to meet the production requirements for accurately locating reservoir sweet spots; therefore, it is important to consider multiple factors when identifying reservoir sweet spots. In this case, our work begins by considering two aspects: the sedimentary environment and structural background. Taking the example of the Dengying Formation deep carbonate reservoir in the Sichuan Basin, China, we analyze the controlling effects of sedimentary facies and fault systems on the Dengying Formation carbonate reservoirs, and then identify sedimentary facies and faults separately at different seismic scales. Steerable pyramid (SP) processing is deployed to decompose the seismic data into various scales and enhance data quality. Field study suggests that the SP method is more stable and effective than frequency decomposition. Under the dual constraints of sedimentary facies and fault systems, reservoir sweet spots are accurately identified. The aim of this study is to provide technical support for the efficient development of deep carbonate reservoirs, especially those primarily controlled by both sedimentary facies and fault systems.
2 Geological background
2.1 Overview of the study area and stratigraphy
The Anyue Gas Field is located in the eastern wing of the Lesan–Longnvsi Uplift in the Sichuan Basin (Xie et al., 2021), where the widely distributed Sinian Dengying Formation has experienced a long sedimentary period, with deep burial depth (Luo et al., 2015). The main lithology of the Dengying Formation is dolomite (Tian et al., 2020). On the basis of sedimentary and lithological characteristics, the entire Dengying Formation can be divided into four members (Li et al., 2023). This study focuses on the Deng 4 Member. The study area is the Gaoshi-18 well block in the Gaoshiti region of the Anyue Gas Field, for which 3D seismic data for an area of approximately 200 km2 are available. The Deng 4 Member has burial depths ranging from 5,000 to 5,500 m, and the regional stratigraphic thickness is 260–350 m (Zhang et al., 2021). This member can be further subdivided into upper and lower sub-members, and the upper sub-member contains a widely distributed, continuous siliceous interlayer (Luo et al., 2019). The main storage types of the Deng four member reservoirs are intergranular pores, fractures, and karst caves (Xiao et al., 2018; Zhang et al., 2021). High-quality reservoirs are mainly concentrated within the top 100 m of the Deng 4 Member (Tian et al., 2020).
2.2 Sedimentary environment and favorable sedimentary facies
The sedimentary environment of the Dengying Formation in the Sichuan Basin is a carbonate platform. The main facies are platform margin mound–shoal facies, intra–platform mound–shoal facies, inner–mound marine facies, and evaporite platform facies (Zou et al., 2011; Lan et al., 2019). The platform margin and intra-platform mound–shoal facies are favorable reservoir facies, and were extensively developed in the shallow-water area of the Deyang–Anyue rift margin.
Mound-shoal facies refer to the combined deposition of algal mounds and grain shoals. As algal mounds and grain shoals commonly develop in adjacent areas and both serve as good reservoirs, they are collectively referred to as “mound–shoal complexes” (Lan et al., 2019). Mound–shoal complexes have a mound-like shape. They grow on topographic highs of paleomorphology, with strong hydrodynamic environments and abundant sunlight. This environment is favorable for the development of microbialite mounds and stromatolites, and for the deposition of granular carbonate rocks (Xu et al., 2022).
2.3 Structural background and fault system
The Sichuan Basin has undergone multiple episodes of tectonic movement, such as the Tongwan, Caledonian, Yanshan, and Himalayan Movements (Jiao et al., 2021), which have led to the development of a series of strike-slip fault systems in the Neoproterozoic Dengying Formation (Ma et al., 2023). These strike-slip fault systems initially formed during the late Precambrian as part of the Tongwan II movement. During this period, the Sichuan Basin was characterized by a differential extensional background, with extension that was stronger in the north and weaker in the south. This condition made the region highly prone to the development of obliquely oriented, dextral trans-tensional strike-slip fault systems to accommodate the differential extensional displacement between the northern and southern regions (Ma et al., 2023). In this context, the central Sichuan region developed a series of nearly EW-trending main strike-slip faults, with lengths ranging from 80 to 200 km (Figure 1), which dominated the tectonic evolution of the central Sichuan Basin (He et al., 2023). Controlled by these main faults, secondary strike-slip faults developed in the Gaoshiti–Moxi area: these faults are primarily NW-trending (with a few NE-trending examples) and extend over thousands of meters. These faults exhibit large extension lengths and wide distributions, but relatively small vertical displacements (Wu et al., 2020; Ma et al., 2023). Seismic profiles show that the vertical displacements of the strike-slip faults are generally less than 50 m, and that the faults cut through multiple layers from the Precambrian basement to Permian strata. The strike-slip fault systems were inherited and reactivated at later times, some during the Mesozoic and Cenozoic. The Tongwan II movement also enhanced the reservoir physical properties by exposing the Deng 4 Member strata to extensive karstification.
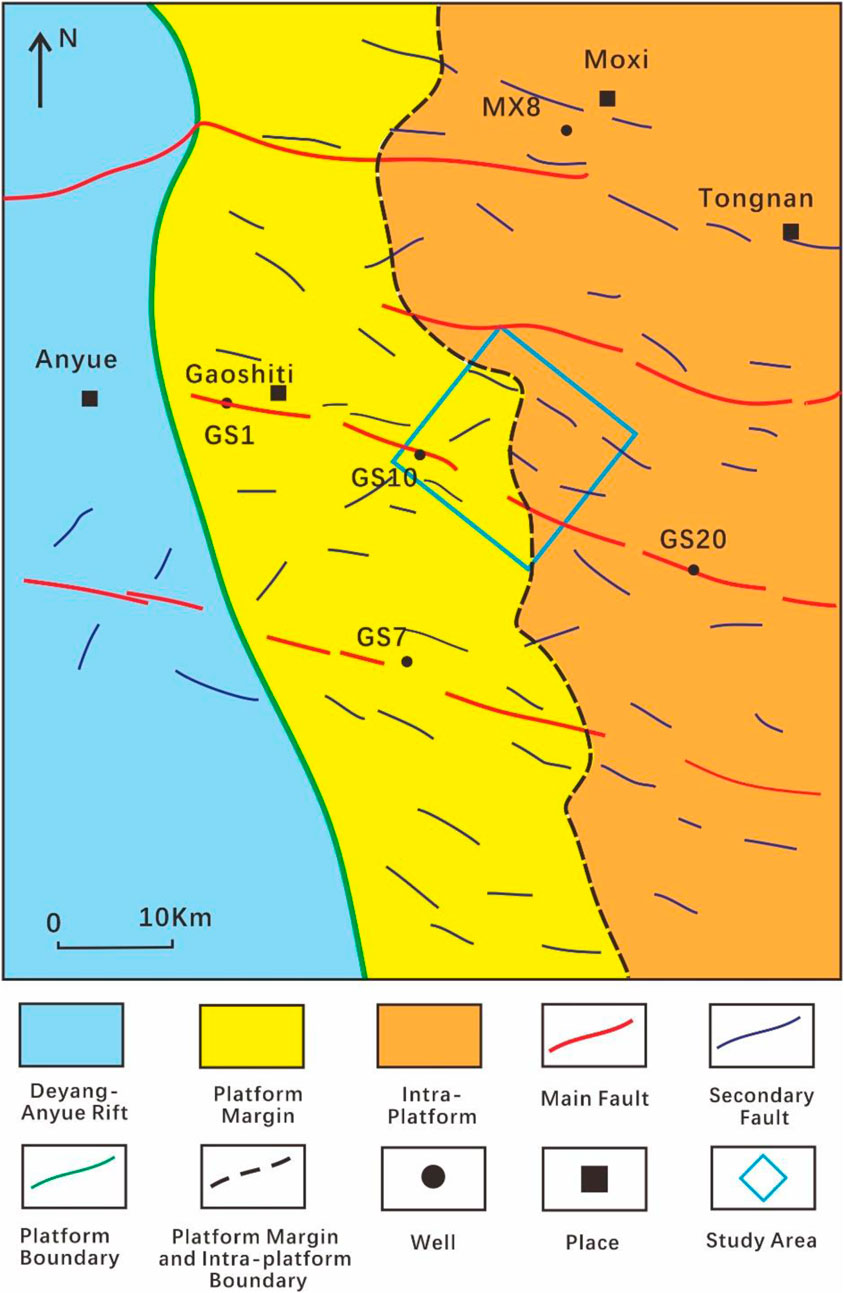
Figure 1. Schematic map of the sedimentary environments and strike-slip faults in the Gaoshiti–Moxi region (after Tian et al., 2020; Ma et al., 2023).
3 Workflow and methodology
In this study, we create a workflow for reservoir sweet spot identification under dual-factor control (Figure 2). First, considering the sedimentary environment and structural background of the study area, we analyze the reservoir patterns and features of mound–shoal facies and strike-slip faults. By deploying seismic forward modeling, we investigate the seismic response characteristics of the mound–shoal facies and strike-slip faults; Simultaneously, we apply SP processing to the original seismic data. This method effectively decomposes seismic data into sub-band data of different scales. By selecting and recombining these sub-bands, two new seismic datasets are constructed: low-frequency and high-frequency data. After analyzing these datasets, we extract the average absolute amplitude attribute from the high-frequency data to identify mound–shoal facies reservoirs. For the low-frequency data, we extract coherent attribute and apply tensor voting processing to identify the strike-slip fault system. Then, we merge the two identification volumes and achieve 3D spatial characterization of reservoirs under dual-factor control. Finally, we use an attribute fusion method to perform the reservoir sweet spot identification.
4 Results
4.1 Seismic response characteristics of faults and reservoir
4.1.1 Seismic response of mound–shoal facies reservoir
The dominant frequency of seismic data is approximately 30 Hz. Limited by seismic resolution, the morphological characteristics of mound–shoal complexes are difficult to identify directly. Based on the lithological characteristics, we investigate the seismic response of mound–shoal complexes using seismic forward modeling. First, considering the lithological conditions when mound–shoal complexes are not developed, the Deng four upper sub-member can be simplified to the model shown in Figure 3A. The Deng four member consists of tight dolomite with high acoustic impedance, underlain by a 30-m-thick layer of Qiongzhusi Formation shale. The shale has lower P-wave velocity and density than the carbonate rocks. A continuous siliceous interlayer occurs approximately 50 m below the Deng four top boundary, with lower P-wave velocity and density than the surrounding rocks. Seismic forward modeling using a 30 Hz dominant frequency wavelet yields a strong wave peak reflection interface at the Deng four top boundary, with a weaker wave peak reflection occurring at the bottom of the siliceous interlayer (Figure 3B).
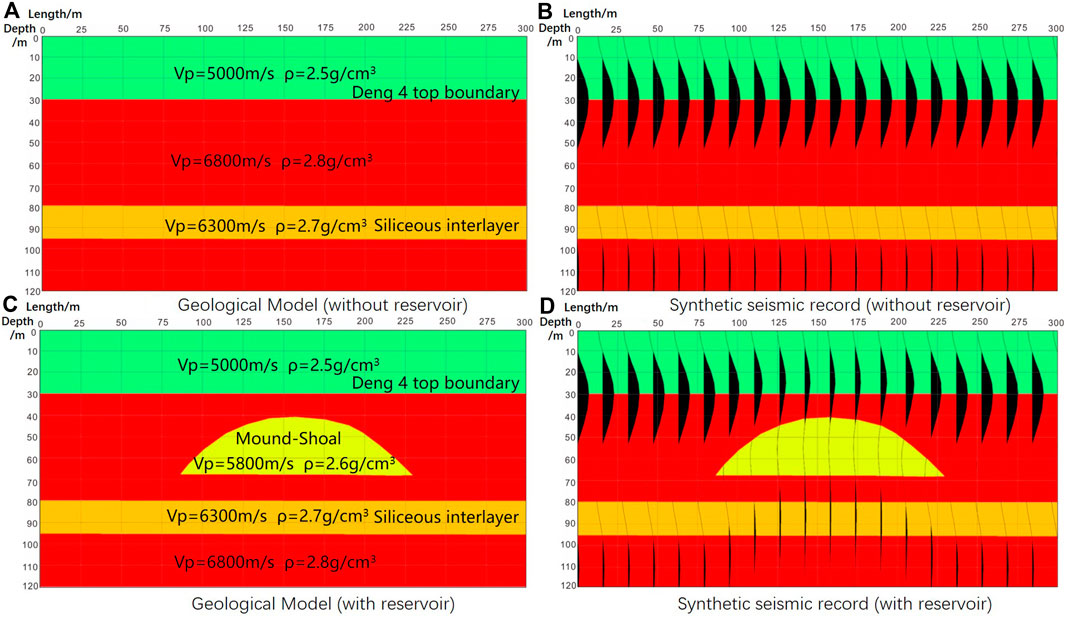
Figure 3. Seismic forward modeling of mound–shoal facies reservoirs: (A) Geological model (without reservoir) (B) Synthetic seismic record (without reservoir) (C) Geological model (with reservoir) (D) Synthetic seismic record (with reservoir).
Then, we consider the condition when a mound–shoal complex is present near the top of the Deng four member (Figure 3C). The mound–shoal complex has low P-wave velocity and density, resulting in lower acoustic impedance compared than the surrounding tight dolomite. The synthetic seismic record using a 30 Hz wavelet (Figure 3D) shows that the presence of a mound–shoal complex leads to a decrease in the acoustic impedance at the Deng four top, causing a pronounced amplitude reduction on the wave peak. A weak amplitude peak response is observed at the mound–shoal complex top, and a decrease in the amplitude of wave trough reflections is observed within the complex reservoir. Additionally, a weaker wave peak response is observed at the base of the mound–shoal complex, suppressing the seismic response of the siliceous interlayer and causing up-shifting of the reflection.
From the forward modeling results, the seismic response of the mound–shoal complex at the Deng four upper member can be summarized as follows: (1) weak amplitude response at the top of the mound–shoal complex, corresponding to a relatively low-amplitude peak at the Deng four top boundary; (2) attenuation of the trough reflection within the mound–shoal complex, possibly with a weak-amplitude peak response at the bottom.
4.1.2 Seismic response of combined strike-slip fault and fault-mound shoal
The main fault type in the Gaoshiti area is strike-slip faults; these faults are characterized by small fault throws and steep dip angles. Rock damage occurs on both sides of the strike-slip fault planes, resulting in the development of fault damage zones and associated fractures. A forward modeling model is established on the basis of the characteristics of strike-slip faults (Figure 4A). Low-impedance fault damage zones (orange areas) are present on both sides of the fault plane, with P-wave velocities ranging from 5,500 to 6,000 m/s and gradually increasing away from the fault plane in both directions. The synthetic seismic record shows time-shift along the seismic event, accompanied by a decrease in amplitude on both sides of the fault damage zone (Figure 4B).
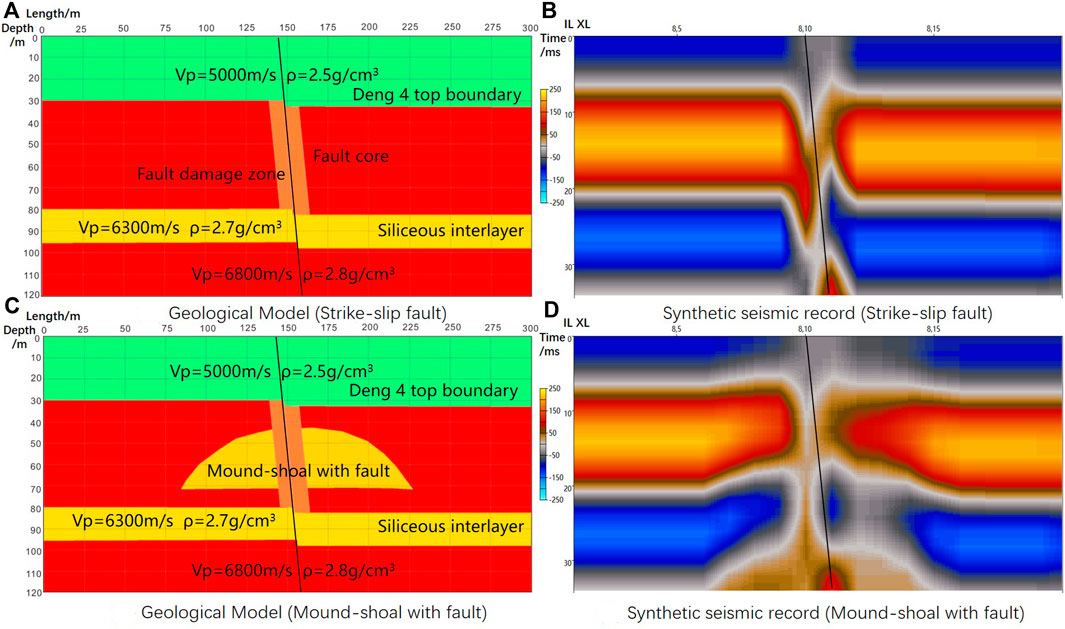
Figure 4. Seismic forward modeling of strike-slip fault and faulted-mound shoal: (A) Geological model (strike-slip fault) (B) Synthetic seismic record (strike-slip fault) (C) Geological model (mound–shoal with fault) (D) Synthetic seismic record (mound–shoal with fault).
We further consider simultaneous development of strike-slip faults and mound–shoal complexes (Figure 4C). The corresponding seismic response (Figure 4D) shows time-shift along the seismic event and pronounced amplitude reduction on both damage zones. A reflection event up-shift phenomenon is present in the lower trough, faintly indicating the mound-like morphology of the mound–shoal complex.
The seismic characteristics of the strike-slip fault system include high-angle time-shift along the seismic event and amplitude reduction on both sides of the fault damage zone. These characteristics are somewhat similar to the response characteristics of amplitude reduction in the mound–shoal complex. In reality, associated fractures are developed near the fault damage zone of the strike-slip fault, enhancing karstification and further weakening the amplitude along the seismic event. Therefore, in cases where strike-slip faults and mound–shoal complexes are both present, it is difficult to distinguish the two based only on low-amplitude features. Using weak amplitude features as the basis for mound–shoal reservoir identification, while using seismic event time-shift features for identifying strike-slip faults, can effectively reduce interference and improve accuracy.
4.2 Reservoir and fault-system identification
This study proposes an approach for multi-scale identification based on the seismic characteristics of reservoirs and fault systems. The thickness of mound–shoal facies reservoirs is generally lower than the vertical resolution of seismic data; thus, further enhancement of seismic resolution and extraction of small-scale features are required to highlight the seismic response characteristics of mound–shoal reservoirs. In contrast, strike-slip faults are characteristically large-scale and extend over long distances, allowing for identification in larger-scale data. Small-scale seismic event folding and discontinuity artifacts may adversely affect identification accuracy. By combining different seismic attributes in seismic data at different scales, we can accurately identify both mound–shoal facies reservoirs and strike-slip fault systems.
4.2.1 Steerable pyramid processing and validation
In this study, the SP method is deployed to perform multi-scale decomposition and reconstruction of seismic data, with the aim of enhancing the quality of seismic data and further highlighting the fault systems. The SP method is a multi-scale data processing method based on image pyramids and directionally steerable filtering. The specific principles of the method can be found in the literature (Freeman and Adelson, 1991; Mathewson and Hale, 2008; Zhao et al., 2021), and will not be further elaborated in this paper. The SP processing decomposes the seismic data of the study area into five different frequency sub-bands (referred to as L1–L5). The seismic profile of the original seismic data and different SP processed sub-band data are shown in Figure 5, and the corresponding spectral analysis of each seismic data is presented in Table 1.
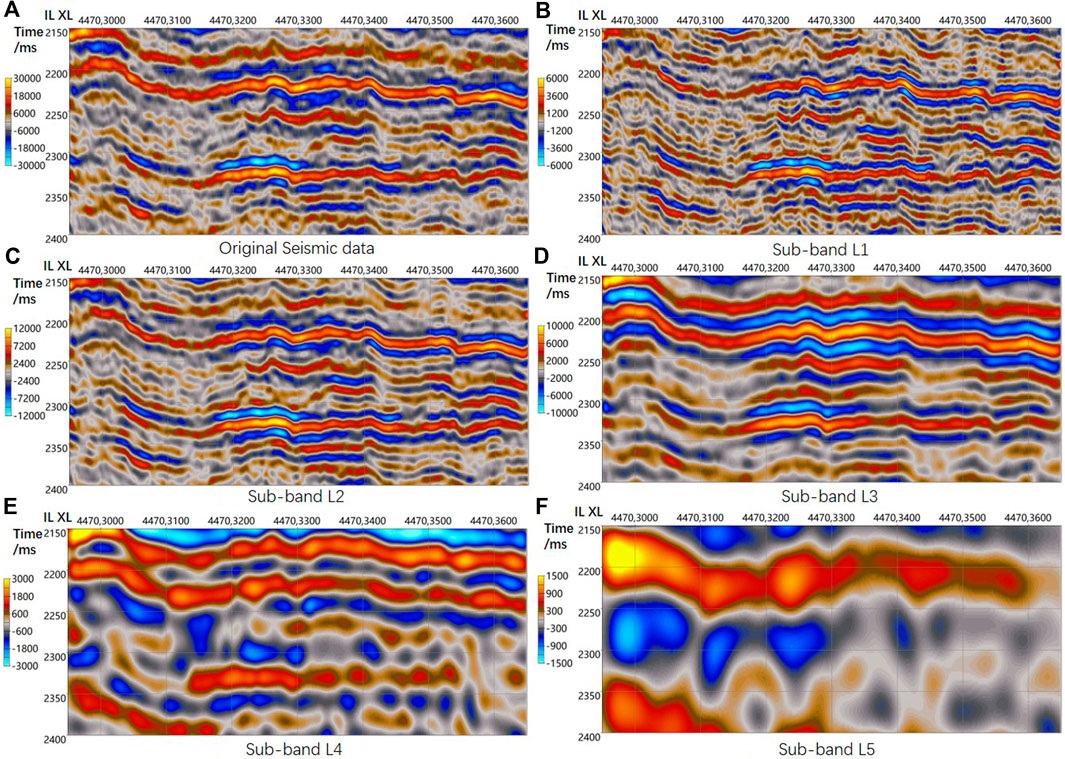
Figure 5. Seismic profiles of the original seismic data and SP processed sub-band data: (A) Original seismic data (B) Sub-band L1 (C) Sub-band L2 (D) Sub-band L3 (E) Sub-band L4 (F) Sub-band L5.
From the seismic profiles and spectral analysis, it can be observed that sub-band L5 has a large scale but very low resolution, and contains only macro-scale seismic information; therefore, its contribution to identifying the fault systems and reservoirs is minimal, and this sub-band is not considered further in this study. L1–L4 are considered to be the key sub-bands for further analysis. L1 has a higher seismic center and dominant frequency compared to the original seismic data, with an overall frequency enhancement of approximately 15 Hz. On the seismic profiles, L1 reveals more details and discontinuities in seismic events, thereby capturing more small-scale geological information and details in the original seismic data. Sub-band L2 is generally similar to the original seismic profile, with a slightly higher center frequency (38.4 Hz) than the original seismic data (32.8 Hz), and exhibits similar geological structures and features while eliminating some noise effects. L3 has a lower center frequency (26.3 Hz) compared to the original seismic data. Although its resolution is slightly lower, it is advantageous for highlighting larger-scale fault features compared to the original seismic data, enhancing the continuity of seismic events and eliminating some fault artifacts. L4 further emphasizes large-scale geological structures compared to L3 but lacks detail, making it suitable for comprehensive analysis in combination with other sub-bands.
For the purpose of reservoir and fault systems identification, the SP processed sub-bands are divided into two groups: the high-frequency group (sub-bands L1 and L2) and the low-frequency group (sub-bands L3 and L4). Then, we stack each group of sub-bands to reconstruct new seismic data, with SP L1+2 representing high-frequency data and SP L3+4 representing low-frequency data. Both new seismic datasets maintain the resolution characteristics of the sub-bands and effectively compensate for the deficiencies in individual sub-band seismic data. In this study, the high-frequency data (SP L1+2) are used for reservoir identification and the low-frequency data (SP L3+4) are used for characterization of fault systems.
To verify the effectiveness and enhancement of the SP processing, we compare the processed datasets with the original seismic data, and also apply the commonly used frequency decomposition (FD) method as a comparison. Corresponding high and low-frequency seismic data were constructed using the FD method. The spectral analysis results for the SP and FD processed data are provided in Table 1. The FD constructed seismic datasets closely match the SP data in terms of dominant frequency and center frequency, achieving maximum control over the frequency aspect. However, as a result of differences in algorithms, the frequency bandwidths of SP processed data are wider than those of frequency decomposition data.
We compare SP low-frequency data with original seismic data and FD low-frequency data (Figure 6). Typical seismic profiles passing through faults are illustrated in Figures 6A–C; the corresponding coherence attribute profiles are shown in Figures 6D–F. Two large strike-slip faults are more clearly visible in both the SP and FD low-frequency data than in the original seismic data (Figures 6A–C). However, there is an obvious amplitude distortion in the lower part of the FD profile, with noticeable changes in the wave peaks and troughs (Figure 6C). This phenomenon is caused by the lack of translation invariance in the FD method, resulting in pronounced amplitude changes on the seismic events. When further considering the attribute profiles, we observe that the coherence attributes of both the SP and FD data clearly show the shape of the strike-slip fault. The difference is that the SP data attribute eliminates some high-frequency noise and attribute artifacts (Figure 6E), whereas the FD data attribute has some noise and artifacts remaining (Figure 6F).
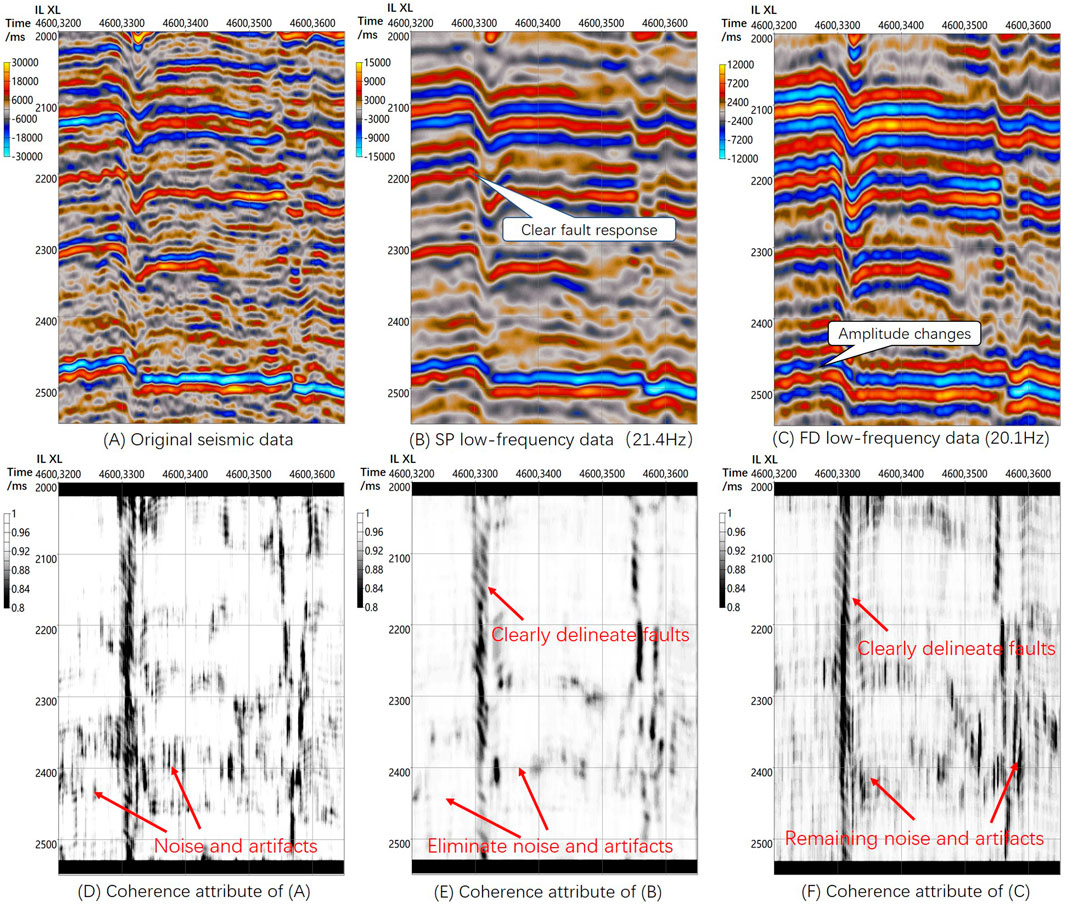
Figure 6. Comparison of original seismic data and SP and FD low-frequency data: (A) Original seismic data (B) SP low-frequency data (C) FD low-frequency data (D) Coherence attribute of (A) (E) Coherence attribute of (B) (F) Coherence attribute of (C).
The comparison between the SP high-frequency data, the original data and the FD processed data is illustrated using a cross-well profile (Figure 7). In the original seismic profile, the top boundary of the Deng 4 Formation exhibits strong wave peak reflection characteristics with high continuity. A high-quality mound–shoal reservoir (indicated by red circles in the figure) is present near the top of the Deng four zone in well GS10. However, because of the limited seismic resolution of the original seismic data, wave interference occurs; as a result, the weak amplitude response expected at the reservoir location is not visible in Figure 7A. After SP processing, the seismic resolution is improved, greatly reducing the wave interference phenomenon and revealing the weak amplitude reservoir response at the Deng four top boundary. The SP processing also enhances the seismic response of the siliceous inter-layer throughout the region, corresponding to the continuous wave peak 20 ms below the Deng four top (Figure 7B).
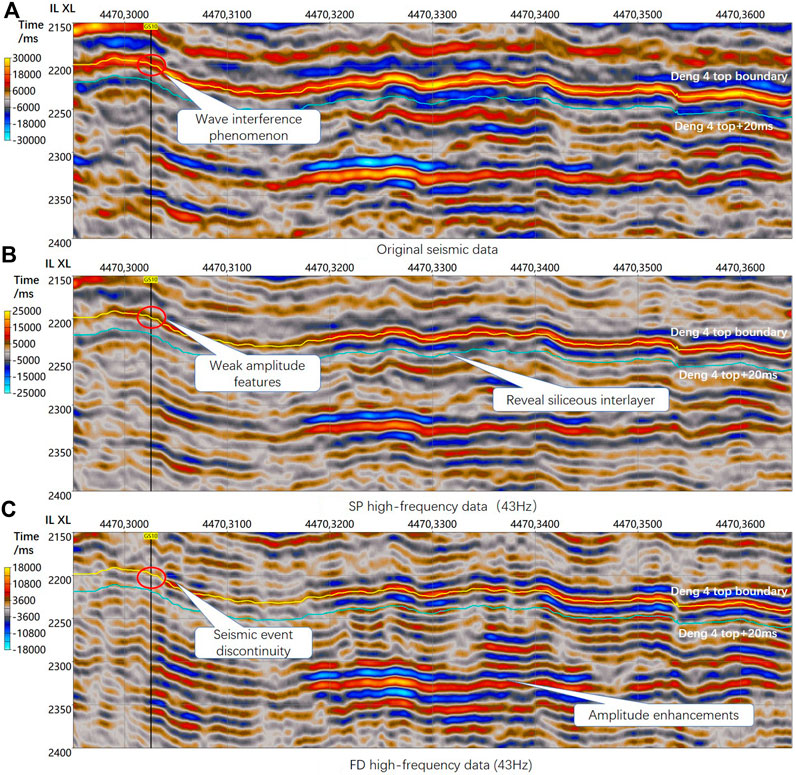
Figure 7. Comparison of original seismic data and SP and FD high-frequency data: (A) Original seismic data (B) SP high-frequency data (C) FD high-frequency data. Red circles indicate a high-quality mound–shoal reservoir.
The FD high-frequency data (Figure 7C) also display weakened amplitude at the Deng four top boundary. Although the weak amplitude feature of the reservoirs in well GS10 are highlighted, the Deng four top boundary shows poor continuity of seismic events with excessively low amplitude. Obvious amplitude distortion on the seismic events can be observed in the middle part of the profile, which differs markedly from the structure of the original seismic data. According to the spectral analysis results, although FD processing enhances the center frequency and dominant frequency of seismic data, the frequency bandwidth of the processed seismic data is narrower. This difference results in the loss of a large amount of low-frequency information, leading to obvious changes in the geological structure and seismic events in the seismic data.
From the above validation and analysis, it can be concluded that SP low-frequency data can clearly characterize the shape of faults by eliminating a large amount of high-frequency noise and artifacts, which aids in interpreting large-scale fault systems. SP high-frequency data markedly improve seismic resolution, highlighting the weak amplitude features of the reservoir at the Deng four top boundary. In the FD data, obvious changes are observed in the stratigraphic structure and seismic events in both high- and low-frequency data. These changes arise because in FD processing the frequency changes lack translational invariance, making it difficult to maintain good consistency between the decomposed frequency components and geological features. In general, the SP method is superior to the FD method in terms of stability and processing effects at different scales.
4.2.2 Mound–shoal facies reservoir identification
We identify the mound–shoal facies reservoirs of the Deng four member using SP high-frequency data. The identification is based on the seismic characteristics of the reservoir, which include: (1) weak amplitude response at the Deng four top boundary; and (2) attenuation of the trough reflection within the mound–shoal complex, with decreased amplitude of peaks 20 ms below the Deng four top boundary. These features indicate that the mound–shoal reservoir causes an overall decrease in reflection amplitudes. The average absolute amplitude can effectively be used to visualize the amplitude decrease of the reservoir, with ideal reservoir locations corresponding to low attribute values. We extract the average absolute amplitude using a window from the Deng four top boundary to 20 ms below the boundary to identify mound–shoal reservoirs at the top of the formation.
Wells with higher productivity, such as GS122, GS125, and GS132, have well trajectories passing through large segments of reservoir zones (dark red color in Figure 8), whereas wells with relatively lower productivity, such as GS119 and GS120, are located in the brown-red areas with slightly higher attribute values. When the well productivity is analyzed against the average attribute values (Figure 12A), there is a negative correlation overall, consistent with the understanding described above. However, it is notable that the data points corresponding to wells GS10 and GS118 deviate markedly from the correlation trend line. Well GS10 exhibits good reservoir properties and is located in the low-value area, indicating high-quality reservoirs in this well. The reason for the low well productivity might be because of a shorter reservoir thickness compared to other wells. The trajectory of well GS118 does not fall within the ideal low-amplitude reservoir zone, even though it has very high productivity. It is preliminarily inferred that, because the well trajectory passes through a strike-slip fault, karstification surrounding the fault has resulted in the formation of favorable fracture-porosity reservoirs around the fault zone. As these reservoirs are not mainly controlled by sedimentary facies, their presence is not obvious in the reservoir identification based on detection of mound–shoal facies.
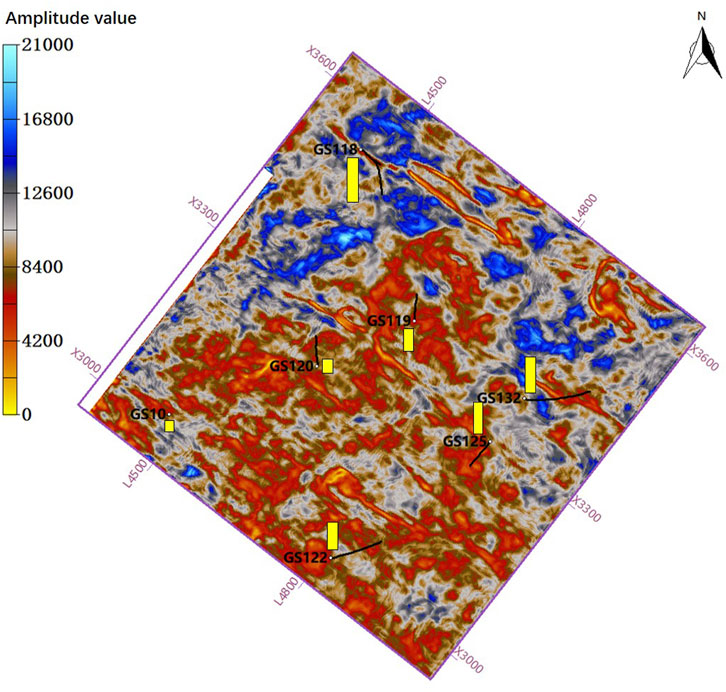
Figure 8. Identification of mound–shoal facies reservoirs based on amplitude. Black lines represent well trajectories, yellow bars represent well productivity, and light-colored low-value areas indicate reservoir locations.
4.2.3 Strike-slip fault system identification
In fault identification, coherence attributes can effectively highlight the features of large-scale fault systems. We extract 3D coherence attributes from SP low-frequency data and identify the fault systems of the Deng four member along the horizon. Strike-slip faults are primarily oriented in the NW direction, with clear shapes for most of the faults (Figure 9A). However, low-continuity areas can be observed in certain faulted locations, and some outlier values result in blurring near the faults. To address these issues, we deploy the tensor voting method to enhance the coherence attribute. This method is based on analyzing structural tensor characteristics and using eigenvalues voting to detect and enhance structural features within the seismic data. The process can be summarized in three steps: first, we input a 2D dataset (planar seismic attribute) and use the gradient vectors to construct the structure tensor for each pixel. Next, by analyzing the eigenvalues of the structure tensors, we can extract the pixels with strong anisotropy (referred as seed points, representing potential fault locations). Finally, a specific voting domain is constructed, and voting is performed on each seed point. The seed points of potential faults will receive higher votes. By setting a reasonable threshold, the tensor voting-enhanced attribute result is obtained (Cui et al., 2021).
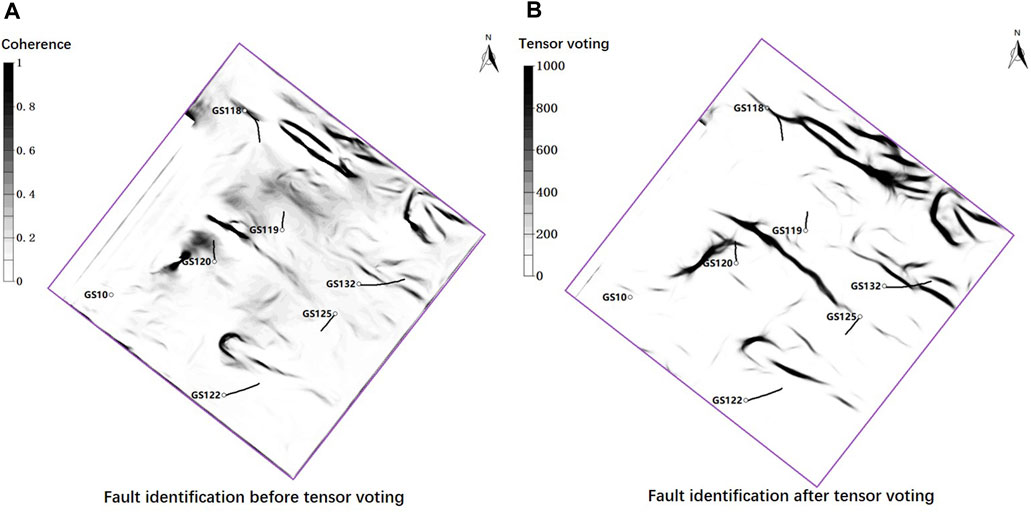
Figure 9. Results of fault systems identification: (A) Fault identification before tensor voting (B) Fault identification after tensor voting.
In this study, tensor voting is applied to coherence attributes to further highlight the morphology of strike-slip fault systems. The tensor voting processing effectively enhances the continuity of large-scale faults and eliminates blurry regions caused by low attribute values and noise interference, achieving an accurate representation of the fault systems (Figure 9B). The major strike-slip faults in the study area are primarily oriented northwest to southeast, with a few faults oriented in northeast to southwest. The faults extend for several kilometers, and some secondary minor faults and fractures are also developed near the fault planes, consistent with the analysis from previous tectonic movement.
To further assess the influence of the strike-slip fault system on reservoir quality, we correlate well productivity with distance from faults. Overall, there is a negative correlation between the two factors (Figure 12B), indicating that wells closer to faults exhibit higher production. The associated fractured zones of the strike-slip faults have a certain impact on the reservoir development within a radius of approximately 2 km, with more pronounced effects observed within a range of 500 m around the fractured zone. This finding suggests that the strike-slip fault system has a controlling effect on reservoirs.
4.3 Reservoir spatial characterization and sweet spot identification
4.3.1 Dual-factor-controlled reservoir spatial characterization
As described above, reservoir identification based solely on sedimentary facies results in a poor match with production data; therefore, considering the control and modification effects of fault systems on reservoirs is necessary. We characterize the distribution of fault systems and mound–shoal sedimentary facies in three-dimensional space, and merge these two volumes to achieve spatial characterization of reservoirs under dual-factor control. First, by setting thresholds to eliminate outlier values in the attribute volumes, 3D characterization volumes of the fault systems and mound–shoal reservoirs are obtained (Figures 10A,B). Then, the two modified attribute volumes are merged with the trajectories of development wells (Figure 10C). The red region in the figure indicates the development of strike-slip fault systems, and the yellow region represents the distribution of mound–shoal facies reservoirs. The mound–shoal facies are more widely distributed than the strike-slip fault systems, and overlap some strike-slip faults. Most of the development wells, such as GS125 and GS132, pass through or are located near mound–shoal reservoirs or strike-slip fault zones.
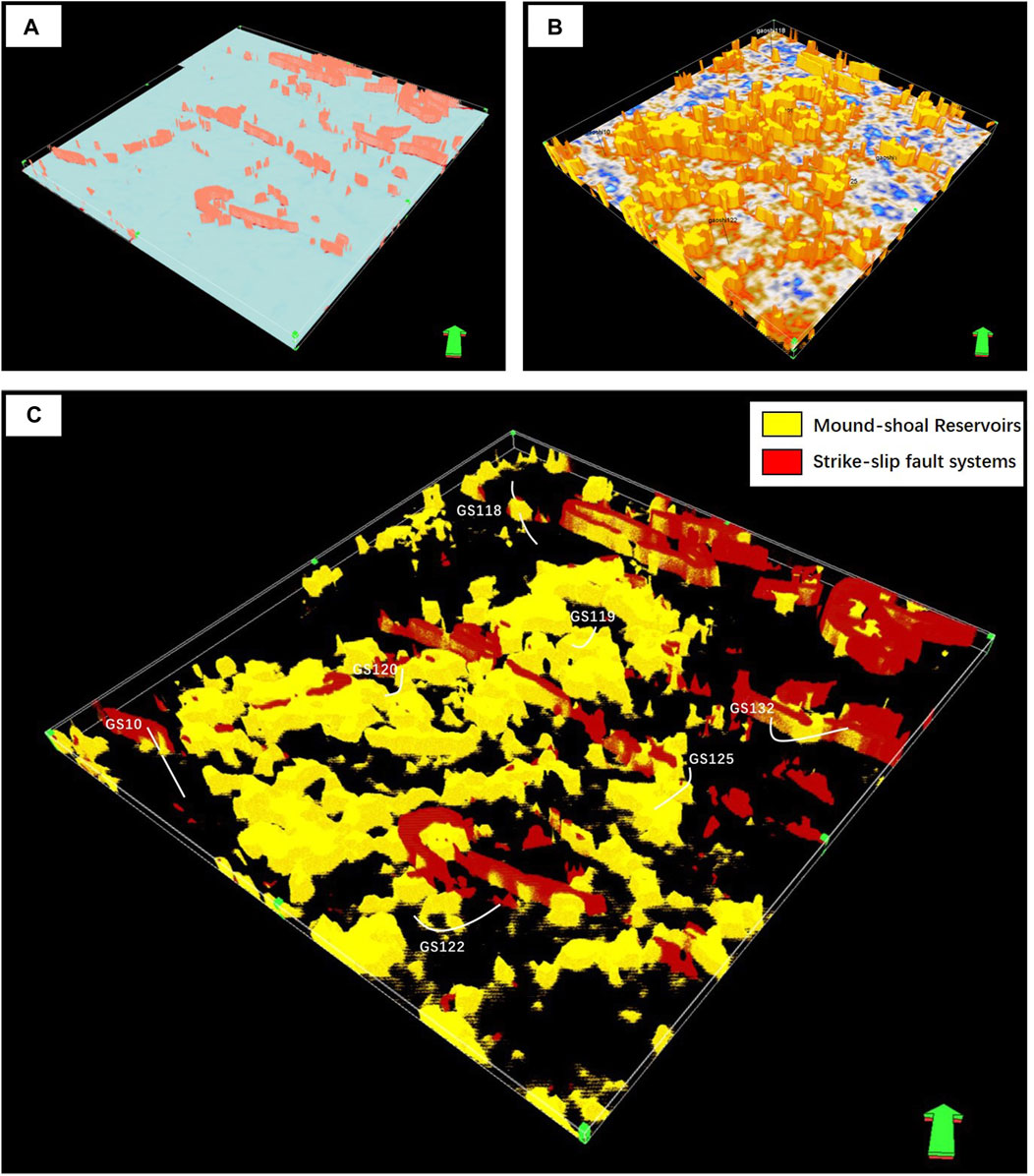
Figure 10. Spatial 3D characterization of strike-slip fault systems and mound–shoal reservoirs: (A) 3D characterization of the strike-slip fault systems (B) 3D characterization of mound–shoal reservoirs (C) Dual-factor-controlled 3D characterization.
Thicker reservoir units are developed near the strike-slip fault zones (Figure 10C), further indicating the controlling effect of strike-slip faults on reservoir quality. We conclude that the control of strike-slip fault systems on reservoirs mainly manifests in two aspects: (1) large strike-slip fault zones provide migration channels for oil and gas, making areas near the fault zones more favorable for hydrocarbon accumulation; (2) strike-slip faults and secondary fracture systems enhance karstification of carbonate reservoirs, facilitating the formation of secondary pores and cavities and thus improving reservoir properties. Under karstification, the secondary dissolution porosity of the Deng 4 Member carbonate reservoirs can increase by 5%–20%; additionally, because of the widespread development of fractures associated with faults, the permeability of carbonate matrix rocks can increase by one to two orders of magnitude (Jiao et al., 2021; He et al., 2023).
To summarize, the carbonate reservoirs of the Dengying Formation are controlled by two factors: mound–shoal sedimentary facies and strike-slip fault systems. The mound–shoal facies serve as the main material basis for reservoir development, determining the type and distribution of reservoirs, while the strike-slip fault systems further enhance hydrocarbon accumulation and reservoir properties.
4.3.2 Identification of reservoir sweet spots
To further quantify the influence of sedimentary facies and fault systems on reservoirs and to locate sweet spots, we deploy a method combining normalization attribute fusion with a smoothing filter. First, the planar predictive attributes of fault systems and mound–shoal complexes are normalized separately using the following equation:
where x represents the original planar attribute value; xmax and xmin correspond to the maximum and minimum values of the original attribute, respectively; and y represents the normalized attribute value.
After normalization, the two attributes are merged to obtain a fusion attribute map that simultaneously reflects faults and sedimentary facies (Figure 11A). In the figure, low values (bright colors) denote the development of sedimentary facies and faults; for example, bright yellow typically corresponds to reservoir areas controlled by both faults and mound–shoal facies.
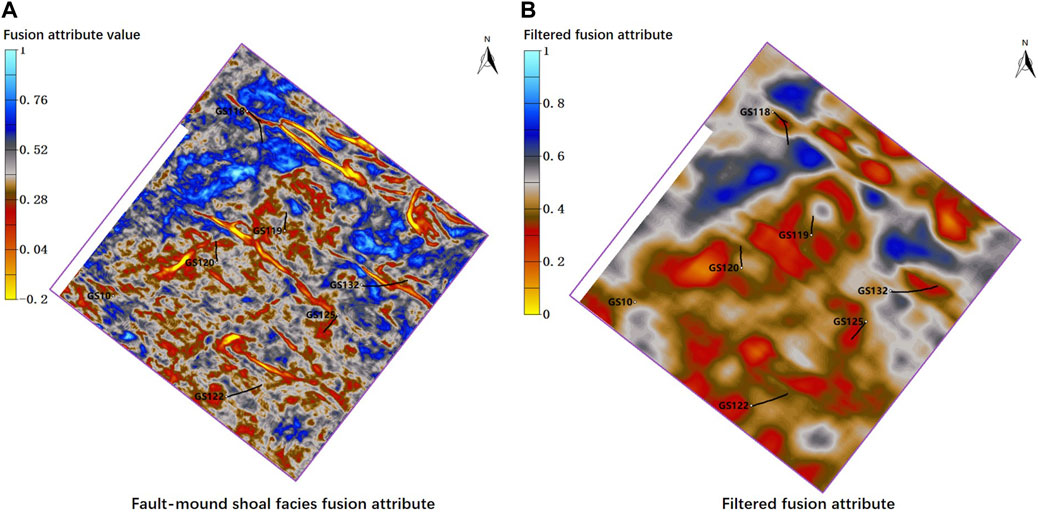
Figure 11. Identification of reservoir sweet spots: (A) Fusion attribute map of faults and mound–shoal facies (B) Fusion attribute map after smooth filtering.
Finally, we extract favorable reservoir characteristics from the fusion attribute using a 50 × 50 smoothing filter, as shown in Eq. 2:
where m and n respectively correspond to the coordinates of the attribute values; Attribute represents the fusion attribute coordinate; and i and j correspond to the size of the smoothing window.
In the filtered fusion attribute map (Figure 11B), the red-colored low-value regions indicate ideal reservoir development areas that are controlled by both strike-slip faults and mound–shoal facies, brown areas represent reservoirs that are likely influenced by single-factor effects, and blue regions indicate reservoirs with low-quality properties or non-reservoir areas. The overall red “sweet spot” areas are similar to the distribution of mound–shoal facies reservoirs, with a few variations in certain locations. This result further confirms that the sedimentary facies control the reservoir distribution at a larger scale.
There is a clear negative correlation between production data and the filtered fusion attribute values (Figure 12C), indicating a better overall fit and higher reliability compared to single-factor identification results (Figures 12A,B). In summary, wells GS118, GS125, and GS132 are located in the reservoir sweet spot area, which is controlled by both faulting and sedimentary facies, and these wells possess better reservoir properties and higher well productivity compared to other wells. Through this method, the sweet spots (red areas) in the study area can be effectively identified, providing support and assistance for further development in the study area.
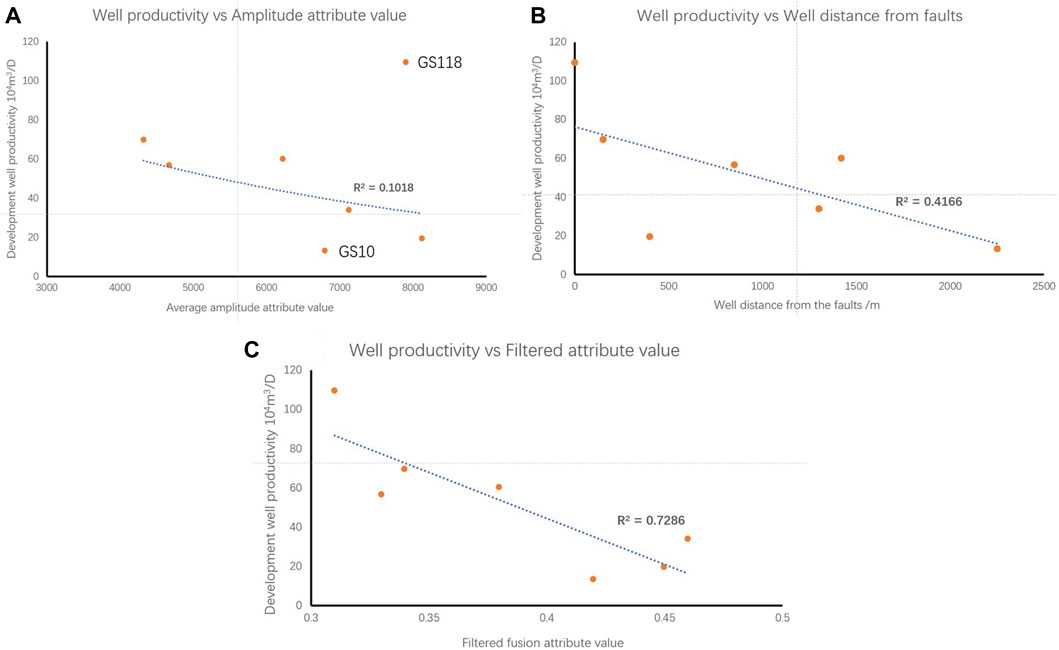
Figure 12. Analysis of reservoir controlling factors: (A) Well productivity versus amplitude attribute values (from Figure 8) (B) Well productivity versus well distance from faults (from Figure 9B) (C) Well productivity versus filtered fusion attribute values (from Figure 11B).
5 Discussion
Our study demonstrates that integrating sedimentary facies and fault systems markedly improves the identification of reservoir sweet spots in deep carbonate formations, specifically focusing on the Dengying Formation in the Sichuan Basin.
Considering the complex seismic response characteristics of sedimentary facies and fault systems, it is essential to identify these features separately at different seismic scales. The steerable pyramid (SP) method proved to be very effective at decomposing seismic data into various scales, improving data quality and resolution. This multi-scale method has been shown to be more stable and effective than the traditional frequency decomposition (FD) method, allowing us to better interpret the complex geophysical responses associated with deep carbonate reservoirs.
Selection of appropriate seismic attributes is also important for the identification of sedimentary facies and faults. In this study, we chose average absolute amplitude and coherence attributes based on their seismic response characteristics; in other areas, other types of amplitude-related or phase-related attributes may be useful for identifying sedimentary facies. For fault identification, commonly used attributes such as coherence, variance and curvature are always worth trying, and the results should be verified with well data to decide the proper attribute. Tensor voting has also been proven to be an effective method to enhance the identification results, particularly for improving fault continuity; however, appropriate parameters should be set to prevent over-enhancement.
In deep carbonate reservoirs, favorable sedimentary facies always determine reservoir quality and distribution on a large scale, and precisely locating these facies is the primary task of reservoir identification. Fault systems serve as essential pathways for hydrocarbon migration and accumulation; they also play a crucial role in karstification, which improves reservoir quality. Understanding fault systems helps to locate the ideal reservoir sweet spots. Our identification of sedimentary facies and faults is consistent with previous studies that suggested that both the sedimentary environment and structural influences play critical roles in reservoir quality. However, unlike prior studies that primarily focused on single factors, our workflow provides a more comprehensive analysis.
The implications of our findings are substantial for both theoretical understanding and practical applications of sweet spot identification. By considering multiple control factors, more accurate and reliable reservoir sweet spot identification can be achieved, which is essential for the efficient development of hydrocarbon resources. However, our study has limitations; for example, the specific carbonate reservoir characteristics of the Sichuan Basin may not be directly applicable to other regions. Additionally, in the present work, fault identification mainly focused on large-scale fault systems, and smaller-scale faults and fractures were not fully considered. Future in-depth research will involve several aspects: investigation of the applicability of our methods to deep carbonate reservoirs in other regions; identification of multi-scale faults and their associated fractures by using various seismic attributes with different scales of seismic data, and analysis of the contributions of faults and fractures at different scales to carbonate reservoirs.
6 Conclusion
(1) The primary reservoir sedimentary facies in the Dengying Formation is mound–shoal complexes. The study area was influenced by multiple episodes of tectonic movements and has developed fault systems primarily dominated by strike-slip faults. The sedimentary facies were identified at a high-frequency scale using amplitude attributes, and the strike-slip faults were identified with coherence attribute and tensor voting at a lower-frequency scale.
(2) Steerable pyramid processing is an effective way to improve seismic data quality. This method involves decomposition of the original seismic data into high-frequency and low-frequency components. The high-frequency data improve the seismic resolution and highlight the amplitude response characteristics of reservoirs. The low-frequency data effectively eliminate noise interference, facilitating attribute identification. The steerable pyramid method is more stable and effective in practical applications than the traditional frequency decomposition method.
(3) In deep carbonate reservoirs, sedimentary facies and fault systems have marked impacts on reservoir quality. Favorable sedimentary facies generally determine reservoir quality and distribution on a large scale, whereas fault systems serve as essential pathways for hydrocarbon migration and potential channels for carbonate karstification. Identifying sedimentary facies and fault systems separately at different seismic scales is an effective way to detect reservoirs. The proposed dual-factor method can effectively locate reservoir sweet spots as part of deep carbonate reservoir exploration and development.
Data availability statement
The original contributions presented in the study are included in the article/Supplementary material, further inquiries can be directed to the corresponding author.
Ethics statement
Written informed consent was obtained from the individual(s) for the publication of any potentially identifiable images or data included in this article.
Author contributions
GZ: Methodology, Validation, Writing–original draft, Writing–review and editing. XH: Funding acquisition, Project administration, Supervision, Writing–review and editing. YX: Funding acquisition, Supervision, Writing–review and editing. ST: Funding acquisition, Methodology, Writing–review and editing. KC: Data curation, Project administration, Resources, Writing–review and editing. DP: Data curation, Project administration, Writing–review and editing.
Funding
The author(s) declare that financial support was received for the research, authorship, and/or publication of this article. This research was funded by the National Natural Science Foundation of China (U20B2016) and the Youth Fund of the National Natural Science Foundation of China (42304136).
Acknowledgments
We thank the Exploration and Development Research Institute of CNPC for providing the data and supporting this research. We also thank Lucy Muir, PhD, from Liwen Bianji (Edanz) (www.liwenbianji.cn) for editing the English text of a draft of this manuscript.
Conflict of interest
Authors KC and DP were employed by PetroChina Southwest Oil & Gasfield Company.
The remaining authors declare that the research was conducted in the absence of any commercial or financial relationships that could be construed as a potential conflict of interest.
Publisher’s note
All claims expressed in this article are solely those of the authors and do not necessarily represent those of their affiliated organizations, or those of the publisher, the editors and the reviewers. Any product that may be evaluated in this article, or claim that may be made by its manufacturer, is not guaranteed or endorsed by the publisher.
References
Ahr, W. M. (2011). Geology of carbonate reservoirs: the identification, description and characterization of hydrocarbon reservoirs in carbonate rocks. Hoboken, NJ: John Wiley and Sons.
Azerêdo, A. C., Duarte, L. V., and Silva, A. P. (2021). The challenging carbonates from the Pre-Salt reservoirs offshore Brazil: facies, palaeoenvironment and diagenesis. J. S. Am. Earth Sci. 108, 103202. doi:10.1016/j.jsames.2021.103202
Carvalho, A. M. A., Hamon, Y., De Souza, O. G., Carramal, N. G., and Collard, N. (2022). Facies and diagenesis distribution in an Aptian pre-salt carbonate reservoir of the Santos Basin, offshore Brazil: a comprehensive quantitative approach. Mar. Petroleum Geol. 141, 105708. doi:10.1016/j.marpetgeo.2022.105708
Chen, Y., Zhao, L., Pan, J., Li, C., Xu, M., Li, K., et al. (2021). Deep carbonate reservoir characterisation using multi-seismic attributes via machine learning with physical constraints. J. Geophys. Eng. 18 (5), 761–775. doi:10.1093/jge/gxab049
Chopra, S., and Marfurt, K. J. (2016). Spectral decomposition and spectral balancing of seismic data. Lead. Edge 35 (2), 176–179. doi:10.1190/tle35020176.1
Cui, X., Huang, Xi, Yang, J., Zhang, D., Chen, X., and Li, K. (2021). Seismic discontinuity feature enhancement method based on tensor voting. Pet. Geophys. Explor. 56 (06), 1351–1358. doi:10.13810/j.cnki.issn.1000-7210.2021.06.018
Freeman, W. T., and Adelson, E. H. (1991). The design and use of steerable filters. IEEE Trans. Pattern analysis Mach. Intell. 13 (9), 891–906. doi:10.1109/34.93808
Hairabian, A., Fournier, F., Borgomano, J., and Nardon, S. (2014). Depositional facies, pore types and elastic properties of deep-water gravity flow carbonates. J. Petroleum Geol. 37 (3), 231–249. doi:10.1111/jpg.12581
He, X., Tang, Q. S., Wu, G. H., Li, F., Tian, W. Z., Luo, W. J., et al. (2023). Controlling and storage role of sinian strike-slip faults in Anyue gas field, Sichuan Basin. Petroleum Explor. Dev. 06, 1116–1127. doi:10.11698/PED.20220611
Hendry, J., Burgess, P., Hunt, D., Janson, X., and Zampetti, V. (2021). Seismic characterization of carbonate platforms and reservoirs: an introduction and review, Geol. Soc, London, Spec. Pub. 509, 1–28. doi:10.1144/sp509-2021-51
Jia, C., Ma, D., Yuan, J., Wei, G., Yang, M., Yan, L., et al. (2022). Structural characteristics, formation and evolution and genetic mechanisms of strizke–slip faults in the Tarim Basin. Nat. Gas. Ind. B 9 (1), 51–62. doi:10.1016/j.ngib.2021.08.017
Pan, J., Li, J., Wang, H., Li, C., Feng, C., and Zhou, J. (2020). Research progress and trend of seismic prediction technology for deep and ultra-deep carbonate reservoirs. China Pet. Explor. 25 (3), 156. doi:10.3969/j.issn.1672-7703.2020.03.014
Jiao, F. Z., Yang, Y., Ran, Q., Wu, G. H., and Liang, H. (2021). Distribution of strike-slip faults and natural gas exploration in the central Sichuan Basin. Nat. Gas. Ind. 08, 92–101. doi:10.3787/j.issn.1000-0976.2021.08.009
Lan, C., Xu, Z., Ma, X., Hu, C., Chen, H., and Zou, H. (2019). Development and distribution of mound-shoal complex in the Sinian Dengying Formation, Sichuan Basin and its control on reservoirs. Acta Pet. Sin. 40 (9), 1069. doi:10.7623/syxb201909005
Li, C. M., Peng, C., Wei, L. Y., Bie, J., Wang, Z. D., Li, W. Q., et al. (2022). Seismic prediction technology for small-scale cavity-type carbonate reservoirs: a case study of the fourth section of the Dengying Formation reservoir in well GS18, internal belt of the Sichuan Basin. Fault-Block Oil Gas Field 02, 189–193. doi:10.6056/dkyqt202202008
Li, J., Yang, C., Xie, W., Rui, Y., Wang, X., Zhang, L., et al. (2023). Differences in natural gas accumulation between the marginal and interior areas of the Sinian platform in Anyue Gas Field, Sichuan Basin, and their exploration implications. Petroleum Nat. Gas Geol. 01, 34–45. doi:10.11743/ogg20230103
Lien Eide, A., Omre, H., and Ursin, B. (2002). Prediction of reservoir variables based on seismic data and well observations. J. Am. Stat. Assoc. 97 (457), 18–28. doi:10.1198/016214502753479194
Liu, Y., and Fomel, S. (2013). Seismic data analysis using local time-frequency decomposition. Geophys. Prospect. 61 (3), 516–525. doi:10.1111/j.1365-2478.2012.01062.x
Lucia, F. J., Kerans, C., and Jennings, J. W. (2003). Carbonate reservoir characterization. J. petroleum Technol. 55 (06), 70–72. doi:10.2118/82071-ms
Luo, B., Yang, Y., Luo, W., Wen, L., Wang, W., and Chen, K. (2015). Factors controlling reservoir development and distribution in the ancient uplifts of central Sichuan: a case study of the Dengying Formation. Acta Pet. Sin. 04, 416–426. doi:10.7623/syxb201504003
Luo, W., Xu, W., Zhu, Z., Liu, X., Wang, Q., Shen, Y., et al. (2019). Origin and geological significance of siliceous rocks in the fourth member of the Sinian Dengying Formation in the Gaoshiti area of the Sichuan Basin. Nat. Gas Explor. Dev. 42 (03), 1–9. doi:10.12055/gaskk.issn.1673-3177.2019.03.001
Ma, B., Liang, H., Wu, G., and Tang, Q. (2023). Formation and evolution of multi-stage strike-slip faults in the central Sichuan Basin. Petroleum Explor. Dev. 50 (02), 333–345. doi:10.11698/PED.20220655
Malki, M. L., Saberi, M. R., Kolawole, O., Rasouli, V., Sennaoui, B., and Ozotta, O. (2023). Underlying mechanisms and controlling factors of carbonate reservoir characterization from rock physics perspective: a comprehensive review. Geoenergy Sci. Eng. 226, 211793. doi:10.1016/j.geoen.2023.211793
Massaro, L., Corradetti, A., Vinci, F., Tavani, S., Iannace, A., Parente, M., et al. (2018). Multiscale fracture analysis in a reservoir-scale carbonate platform exposure (Sorrento Peninsula, Italy): implications for fluid flow. Geofluids 2018, 1–10. doi:10.1155/2018/7526425
Mathewson, J., and Hale, D. (2008). “Detection of channels in seismic images using the steerable pyramid,” in SEG International Exposition and Annual Meeting (SEG-2008), Las Vegas, Nevada, November 2008.
Nabawy, B. S., El-Bialy, M., Hamimi, Z., Khamis, H. A., Wahed, S. A. A., Osman, R. A., et al. (2023). Implication of the diagenetic evolution, litho-and microfacies types on the storage capacity of the carbonate rocks in West Esh El Mallaha area, SW onshore Gulf of Suez, Egypt. J. Afr. Earth Sci. 204, 104971. doi:10.1016/j.jafrearsci.2023.104971
Naghizadeh, M. (2012). Seismic data interpolation and denoising in the frequency-wavenumber domain. Geophysics 77 (2), V71–V80. doi:10.1190/geo2011-0172.1
Naseer, M. T., and Asim, S. (2018). Characterization of shallow-marine reservoirs of Lower Eocene carbonates, Pakistan: continuous wavelet transforms-based spectral decomposition. J. Nat. Gas Sci. Eng. 56, 629–649. doi:10.1016/j.jngse.2018.06.010
Pppelreiter, M., Balzarini, M. A., De Sousa, P., Engel, S., Galarraga, M., Hansen, B., et al. (2005). Structural control on sweet-spot distribution in a carbonate reservoir: concepts and 3-D models (Cogollo Group, Lower Cretaceous, Venezuela). AAPG Bull. 89 (12), 1651–1676. doi:10.1306/08080504126
Sarhan, M. A. (2024). Editorial: advanced techniques and applications for characterizing the hydrocarbon potential in carbonate reservoirs. Front. Earth Sci. 12, 1385645. doi:10.3389/feart.2024.1385645
Shen, J. W., Webb, G. E., and Jell, J. S. (2008). Platform margins, reef facies, and microbial carbonates; a comparison of Devonian reef complexes in the Canning Basin, Western Australia, and the Guilin region, South China. Earth-Science Rev. 88 (1-2), 33–59. doi:10.1016/j.earscirev.2008.01.002
Shi, J., Zhao, X., Pan, R., Zeng, L., and Zhu, Z. (2023). Characteristics of natural fractures in the Sinian Dengying Formation carbonate reservoir and their impact on gas well productivity in the central Sichuan area. Petroleum Nat. Gas Geol. 02, 393–405. doi:10.11743/ogg20230211
Tian, X., Peng, H., Wang, Y., Yang, D., Sun, Y., Zhang, X., et al. (2020). Reservoir differences and controlling factors of the fourth section of the Sinian Dengying Formation between the platform margin and interior in Anyue Gas Field, central Sichuan. Nat. Gas. Geosci. 09, 1225–1238. doi:10.11764/j.issn.1672-1926.2020.04.007
Wadas, S. H., Krumbholz, J. F., Shipilin, V., Krumbholz, M., Tanner, D. C., and Buness, H. (2023). Advanced seismic characterization of a geothermal carbonate reservoir–insight into the structure and diagenesis of a reservoir in the German Molasse Basin. Solid earth. 14 (8), 871–908. doi:10.5194/se-14-871-2023
Wang, Q., Zhang, Y., Xie, Z., Zhao, Y., Zhang, C., Sun, C., et al. (2022). The advancement and challenges of seismic techniques for ultra-deep carbonate reservoir exploitation in the Tarim Basin of northwestern China. Energies 15 (20), 7653. doi:10.3390/en15207653
Wang, R., Tang, Y., Yang, F., She, J., Li, X., Chen, N., et al. (2024). A fracture modeling method for ultra-deep reservoirs based on geologic information fusion: an application to a low porosity sandstone reservoirs in X gas field of a basin in western China. Front. Earth Sci. 11, 1351264. doi:10.3389/feart.2023.1351264
Wu, G. H., Zhang, T., Zhu, Y. F., Wan, X. G., and Xiong, C. (2020). Structure, distribution, and development mechanism of carbonate rock fracture zones. Geol. Sci. 55 (01), 68–80. doi:10.12017/dzkx.2020.006
Xiao, F. S., Chen, K., Ran, Q., Zhang, X., Xie, B., Liu, X. G., et al. (2018). New understanding of seismic patterns of high-yield wells in the Sinian Dengying Formation gas reservoirs in Gaoshiti area of Sichuan Basin. Nat. Gas. Ind. 02, 8–15. doi:10.3787/j.issn.1000-0976.2018.02.002
Xie, J., Tang, Q., Peng, X., Deng, H., and Xu, W. (2021). Key technologies for the efficient development of ultra-deep ancient dolomite karst gas reservoirs: a case study of the Sinian Dengying Formation gas reservoir in the Anyue gas field of the Sichuan Basin. Nat. Gas. Ind. B 8 (6), 588–595. doi:10.1016/j.ngib.2021.11.006
Xu, M., Fu, J., Yang, X., Liu, J., and Wang, Y. (2019). Seismic detection of ultra-deep carbonate pore-fracture zones in the northwest of the Sichuan Basin. Nat. Gas. Ind. 39 (11). doi:10.3787/j.issn.1000-0976.2019.11.003
Xu, Z., Lan, C., Zhang, B., Hao, F., Lu, C., Tian, X., et al. (2022). Impact of diagenesis on the microbial reservoirs of the terminal Ediacaran Dengying Formation from the central to northern Sichuan Basin, SW China. Mar. Petroleum Geol. 146, 105924. doi:10.1016/j.marpetgeo.2022.105924
Zhang, M., Dai, X. F., Pang, C. X., Jiang, L., Geng, C., and Xu, Y. P. (2021). Recognition and application of high-quality reservoirs in the internal belt of the Dengying Formation in the central Sichuan region. Nat. Gas. Geosci. 05, 764–771. doi:10.11764/j.issn.1672-1926.2020.12.007
Zhao, Y., Huang, X., Chen, Y., Song, H., Zhang, D., Cui, X., et al. (2021). Geological body boundary identification method using the steerable pyramid and its application in conglomerate reservoirs. Geophys. Prospect. Petroleum (03), 414–420+429. doi:10.3969/j.issn.1000-1441.2021.03.007
Keywords: Sichuan Basin, Dengying formation, mound-shoal complex, strike-slip fault, reservoir sweet spot, steerable pyramid processing
Citation: Zhang G, Huang X, Xu Y, Tang S, Chen K and Peng D (2024) Deep carbonate gas reservoir sweet spot identification with seismic data based on dual-factor control of sedimentary facies and fault system. Front. Earth Sci. 12:1427426. doi: 10.3389/feart.2024.1427426
Received: 03 May 2024; Accepted: 13 June 2024;
Published: 02 July 2024.
Edited by:
Shu Tao, China University of Geosciences, ChinaReviewed by:
Jun Lu, China University of Geosciences, ChinaShihang Feng, Los Alamos National Laboratory (DOE), United States
Suzhen Shi, China University of Mining and Technology, China
Copyright © 2024 Zhang, Huang, Xu, Tang, Chen and Peng. This is an open-access article distributed under the terms of the Creative Commons Attribution License (CC BY). The use, distribution or reproduction in other forums is permitted, provided the original author(s) and the copyright owner(s) are credited and that the original publication in this journal is cited, in accordance with accepted academic practice. No use, distribution or reproduction is permitted which does not comply with these terms.
*Correspondence: Guanyu Zhang, ZGVpdHl3aW5kc29uZ0AxNjMuY29t