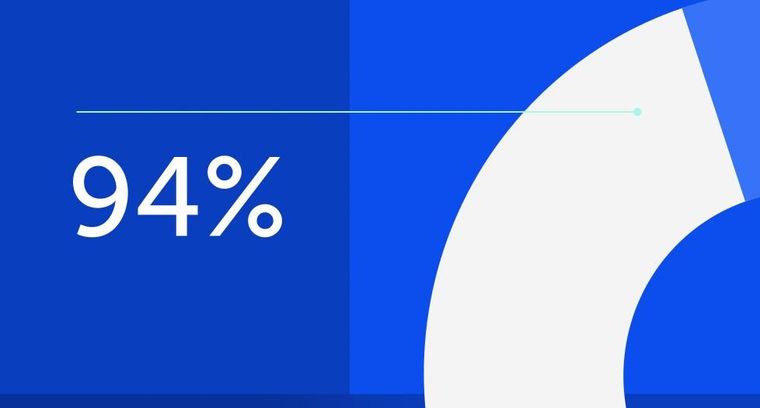
94% of researchers rate our articles as excellent or good
Learn more about the work of our research integrity team to safeguard the quality of each article we publish.
Find out more
ORIGINAL RESEARCH article
Front. Earth Sci., 12 June 2024
Sec. Geohazards and Georisks
Volume 12 - 2024 | https://doi.org/10.3389/feart.2024.1426980
Debris flow is a prevalent geological disaster in Beijing, particularly in the Mentougou District, situated in the mountainous southwestern region of Beijing City. The past irrational exploitation of minerals has led to a substantial accumulation of slag, providing abundant loose materials for the formation of debris flow in the study area. Different from the traditional method of using hazard and vulnerability to carry out debris flow risk assessment, this paper proposes a debris flow risk assessment method based on (a) the possibility of debris flow occurrence, (b) the value of the disaster-affected object, and (c) the destructiveness of the debris flow. The possibility of 41 gully debris flows occurring was determined through theoretical calculations. Using the SFLOW model, the runout of the potential debris flow was simulated. On this basis, an investigation of the value of the disaster-affected objects was carried out. At the same time, according to the simulated movement information of debris flow, its destructiveness was determined. According to the results of the occurrence possibility of the debris flow, its destructiveness, and the value of the disaster-affected object, the risk levels of 41 potential debris flow gullies in the Mentougou District were obtained. The research results can guide the warning systems for debris flows in the Mentougou District of Beijing.
Debris flow is a widely developed geological disaster (Tang et al., 2009; Wu et al., 2019), characterized by a rapid flow of water, soil and gravel mix (de Scally et al., 2010; Chang et al., 2015), resulting in extensive damages all along its way (Li Z. et al., 2021). To reduce the adverse effects of potential debris flow on societies and infrastructures, models developed by researchers regarding the possibility of occurrences and runout of debris flow and associated risk should be followed during land use planning and construction (Bahrami et al., 2020; Li et al., 2020; 2022; Zhang et al., 2023). The existence of objects affected by the disaster is crucial to classify it as a natural disaster; otherwise, it can only be considered as a natural phenomenon (Xu et al., 2018). The World Meteorological Organization defines risk as “Expected losses (of lives, persons injured, property damaged and economic activity disrupted) due to a particular hazard for a given area and reference period.” Since there is currently no generally accepted method to assess the risk of potential debris flows (Huang et al., 2010), therefore, some scholars combine vulnerability and hazard for carrying out risk assessments of debris flow (Ouyang et al., 2019; Liu et al., 2024), while others include hazard, vulnerability, and exposure during debris flow risk assessment job (Zou et al., 2019).
Currently, when conducting regional debris flow susceptibility or risk assessments, the evaluation unit can be selected as a grid unit or a watershed unit (Zhang et al., 2019; Zhang et al., 2022 Y.; Li Y. C. et al., 2021). Because the division of grid units is relatively simple, it is adopted by more scholars. However, since debris flow often occurs in a watershed, the result does not seem reasonable when calculating the susceptibility or risk of debris flow, if a gully is divided into different units, and each unit has different susceptibility or risk levels. When using watershed units for risk assessment, some scholars use the hazard of debris flows and the vulnerability of hazard-affected objects (Ouyang et al., 2019). Risk assessment is carried out by analyzing the intensity of debris flow under a certain return period and the value of the hazard-affected objects. When using the above method, it is assumed that debris flows will occur, but the occurrence of debris flows is also a complex issue, which is not only related to external environmental conditions such as rainfall, but also closely related to geological conditions in the watershed such as terrain and loose material reserves (Li et al., 2020; Li Y. C. et al., 2021). Therefore, when conducting a risk assessment of a disaster, three main factors should be considered: (a) the possibility of the occurrences of the potential disaster. If there is no possibility of it happening in the future, then there is no risk; (b) presence of any object at risk should be considered. If there is no object, regardless of the disaster occurrence there will be no risks to human beings; finally, (c) level of the destructiveness of the disaster must to be considered too. If its impact is small in terms of both scale and intensity and causes minimal harm to the affected objects, then it may pose no risk at all.
China frequently suffers from debris flow disasters (Zou et al., 2019), with a total of 899 incidents occurrences are reported alone in the year 2020 across the country. Among geological disasters, debris flow causes more casualties in Beijing (Xie et al., 2001; Zhu, 2018; Zhao et al., 2023). The research area for this study is Mentougou District, located in the southwestern part of Beijing, which is a mountainous region with abundant resources (Zhu, 2018). The early activities in this area, i.e., logging, mining, quarrying, and road construction, have adversely affected the mountain environment (Xie et al., 2001; Qi and Zhang, 2011). As a result of the mentioned past activities, a significant quantity of loose material had accumulated on hillsides, which ultimately increased the possibility of debris flow occurrence. As the Mentougou District is mostly mountainous, therefore most of the towns are situated on the gently dipping alluvial fans of debris flow (Zhuo, 2001). Numerous catastrophic debris flow incidents have been recorded in the Mentougou District (Zhuo, 2001). The current topography, geology, soil, geomorphology, and land use conditions in the district are of the extent and type that can cause destructive debris flows in the future (Corominas et al., 2014; Zhang X. et al., 2022). Therefore, it is important to identify potential debris flow gullies in Mentougou District and assess the risk of the gullies.
This article focuses on assessing debris flow risk in Mentougou District, Beijing. Through analysis of remote sensing images and field investigations in the study area, a total of 41 debris flow gullies were studied and identified. Unlike previous studies, this article evaluates the risk of potential debris flows by considering the likelihood of its occurrence, the value of the disaster-affected objects, and the destructive nature of debris flows. The outcomes of this study provide valuable guidance for resilient and sustainable land use and planning in Mentougou District and can help in future disaster management and mitigation strategies. The research objectives of this study are as follows: 1) Develop a debris flow risk assessment method based on three elements: (a) the possibility of debris flow occurrence, (b) the value of the disaster-affected object, and (c) the destructiveness of the debris flow; 2) Based on numerical simulation, theoretical calculation and analysis, evaluate the three elements of 41 debris flow gullies in Mentougou; 3) Calculate the risk of 41 debris flow gullies and conduct debris flow risk mapping in the Mentougou area.
Mentougou District is located in the mountainous area southwest of Beijing, connecting the North China Plain and the Mongolian Plateau (Wang and Luo, 2015). The Mentougou District comprise high terrain in the northwest and low terrain in the southeast (Zhuo, 2001) (Figure 1). The area of the Mentougou District is 1,455 km2, of which 98.5% is mountainous terrain and only 1.5% comes under plains. As of 2021, the permanent population of the district was around 396,000 peoples. The average annual rainfall in this region is approximately 600 mm (Wu, 2010), with the peak rainfall typically occurring from July to September, accounting for 70%–80% of the total annual rainfall (Zhao, 2013). The vegetation in the district is mainly deciduous and broad-leaved forest, and the vegetation coverage rate is 40–60% (Wu, 2010). The main river in this region is the Yongding River.
This article focuses on assessing the risk of debris flow in Mentougou District, Beijing. Through analysis of remote sensing images and field investigations in the study area, a total of 41 debris flow gullies were studied and identified. Unlike previous studies, this article evaluates the risk of potential debris flows by considering the likelihood of its occurrence, the value of the disaster-affected objects, and the destructive nature of debris flows. The outcomes of this study provide valuable guidance for resilient and sustainable land use and planning in Mentougou District and can help in future disaster management and mitigation strategies.
The Mentougou District has a diverse stratigraphy, having successions of various ages ranging from the Middle Paleozoic to the present; among them, the Mesozoic Jurassic is well-developed (Wu, 2010; Wang and Luo, 2015) (Figure 2). The dominant rock type are sandstone, dolomite, and limestone (Wang and Luo, 2015). The study area has abundant mineral resources such as coal and limestone; therefore, in the past, extensive quarrying activities have damaged the environment of the whole district (Zhu, 2018). The presence of accumulated coal gangue damps and quarrying slag damps on the slopes in the study area can provide abundant materials that may contribute to debris flow occurrences.
During the field survey, thick debris flow deposits were found in various gullies (Figure 3A). Many of these gullies has large and thick deposits of loose materials or leftover coal mining wastes (Figures 3B–D). These loose deposits in the gullies have been deeply incised by the surface runoff water (Figure 3E). In some gullies that hold substantial debris flow deposits or loose mining and other quarrying wastes, debris flow monitoring devices and warning signs are installed there (Figure 3F). The studied gullies have enough accumulation of loose material, which ultimately increases their potential to form debris flow during rainy conditions. Although monitoring systems have been installed in some gullies, there is still a need to study the risk of debris flow.
Figure 3. Photographs of debris flow gullies in the Mentougou District (A) debris flow deposits in gully channel; (B) and (C) loose material in gullies; (D) residual coal gangue; (E) deposits eroded by the flow; (F) debris flow monitoring equipment.
This article comprehensively evaluates the risk based on three broad aspects: the possibility of occurrence of debris flow, the value of the disaster-affected body, and the destructiveness of debris flows. For the occurrence probability, debris flow susceptibility is used as a proxy in this article. By predicting the movement path of the debris flows, one can easily figure out the stretch of the area that could be affected from the expected debris flow. Combining remote sensing data and on-site surveys, population and property information in the affected areas can be determined to obtain the value of the disaster-affected body. The destructiveness can be assessed from the maximum velocity and depth of the potential debris flow. Figure 4 shows the general flow chart of the method for assessing the risk of debris flows.
The possibility of a debris flow occurrence can be determined by its susceptibility level. A higher level of susceptibility suggests a greater probability of a debris flow event (Corominas et al., 2014; Xiong et al., 2020). This paper utilized the Analytic Hierarchy Process (AHP) method to obtain the susceptibility of debris flow in the investigated gullies. The AHP method, first introduced by Saaty (1980), is now a widely and commonly used multi-index analysis and evaluation technique, combining qualitative and quantitative analysis. Many scholars have conducted susceptibility assessments of geological disasters using the AHP technique (Zhang et al., 2016; Bahrami et al., 2020; Zhan et al., 2021; Li et al., 2023). This AHP method can decompose the target or criterion, and the contribution of each evaluation factor can be obtained. The analysis process is mainly divided into four steps.
1) The establishment of the hierarchical model; This article sets the model at two levels. The first level is the target layer for susceptibility evaluation, and the second level is the evaluation factors affecting the occurrence of debris flow.
2) Construction of judgment matrix; During this step, the importance of evaluation factors is compared in pairs, using the 1–9 scale method (Table 1) to indicate the importance level (Saaty and Vargas, 1991). A judgment matrix is constructed based on the comparison results. The importance of evaluation factors is obtained by consulting with experts, and the judgment matrix A can be obtained based on Eq. 1.
where
3) Weight calculation; Solve the judgment matrix A, and then obtain the weights of each factor through normalization of the eigenvectors.
4) Consistency check; The consistency test is performed on the maximum eigenvalues obtained in the previous step, requiring that the value of consistency ratio (CR) does not exceed 0.1. The calculation method is shown in Eqs 2, 3. Consistency index (CI) and random consistency index (RI) are determined by the order of the matrix A, and RI can be obtained by checking Table 2.
Debris flows are commonly triggered by both external environmental factors as well as internal geological factors (Yang et al., 2011). The occurrence of debris flow further strongly depends on the local topographic conditions, provenance conditions, and rainfall conditions within a specific area (Takahashi, 1981; Wang et al., 2024). The evaluation factors can be selected based on previous studies on debris flow susceptibility assessment.
After determining the weight of the evaluation factors, the debris flow probability index can be calculated according to Eq. 4.
where
The value assessment of the affected objects is completed by calculating the value of life and property within the debris flow threat area (Miao and Liu, 2020). SFLOW software, developed by Jilin University China, has been utilized for simulation of debris flow runout. The SFLOW mode is established based on the free-surface shallow equation (Han et al., 2017). This software is effective for analyzing irregular as well as complex terrain and successfully has been applied for simulation of debris flow motion in Beijing, Tianjin, Sichuan, and some other places in China (Han et al., 2017; Bao et al., 2019; Li et al., 2020).
In the simulation using SFLOW, topographic data, hydrographs, and some fluid parameters need to be prepared in advance (Li et al., 2020). Topographic data can be obtained by measuring gullies onsite using an unmanned aerial vehicle, or through a Digital Elevation Model (DEM) (Yu et al., 2024; Zhan et al., 2024). Additionally, it is necessary to identify a specific point in the channel as the inflow point for the debris flow. The area upstream of the inflow point is referred to as the catchment area, while the area downstream is called the drainage area. The areas upstream and downstream of the selected point are called catchment and drainage areas, respectively. The hydrographs for the different debris flow gullies at specific recurrence intervals can be obtained from the standard method outlined in the Hydrological calculation manual. The rheological parameters mainly refer to viscosity and yield stress, which are determined by the volumetric sediment concentration of fluid (Chen et al., 2013). Based on the above input data parameters, the simulation of the debris flow runout path can be carried out through SFLOW software.
The value of human lives is assessed through field surveys to estimate the population in the disaster-affected area. The value of property is calculated by summing up the economic value of various buildings, cultivated land, and roads within the disaster-affected area. Then, the overall property value of the disaster-affected object can be computed using Eqs 5, 6.
where
Debris flow destructiveness is closely related to its velocity, accumulation depth, and volume (Cui et al., 2013; Ouyang et al., 2019). By utilizing the earlier mentioned SFLOW software, the velocity and depth of the debris flow can be determined based on the simulation results. This study utilized the maximum velocity during simulated debris flow movement and the maximum depth after the movement to evaluate its destructiveness.
These two elements (maximum velocity and depth) were divided into 5 levels according to the NB method. For each gully, the level of maximum velocity and maximum accumulation depth were compared, and the larger of them was selected as the level of its destructiveness.
Assessing the risk of debris flow is a complex process that involves considering not only the possibility of its occurrence but also its severity and the value of the disaster-affected objects. Based on previous studies, we developed a mathematical model for assessing its risk, as illustrated in Eq. 7.
where
The terrain data was obtained from DEM, which comes from the ALOS PALSAR DEM products with a resolution of 12.5 m. The lithology information was determined through a combination of field surveys and 1:100,000 engineering geological maps in the study area. Remote sensing data mainly uses Google Earth images and Landsat 8 satellite data (with a resolution of 30 m) downloaded from the Geospatial Data Cloud. Normalized difference vegetation indices (NDVI) layers were extracted from the Landsat 8 satellite data with a resolution of 30 m. The rainfall data mainly comes from the historical 24-h maximum rainfall of each hydrology/rainfall monitoring station recorded in the Hydrological calculation manual of Beijing.
Through analysis of remote sensing images, consultation with local residents, and on-site surveys, a comprehensive assessment of identification of gullies contains debris flow deposits or quarrying and mining wastes, was conducted in Mentougou District. Finally, 41 potential debris flow gullies were identified (Figure 1). These gullies are distributed across the study area, posing potential risk to the nearby environment, i.e., villages, highways, and scenic spots. Through DEM, the area of each potential debris flow gully was calculated. The calculations revealed a maximum area of 10.09 km2, a minimum area of 0.04 km2, an average area of 2.09 km2, and a total area of 85.61 km2.
By reviewing the literature on debris flow susceptibility assessment studies in and around Beijing (Xie et al., 2001; Wu, 2010; Li et al., 2020) and considering the findings of the field and social survey, we selected eight indicators for carrying out debris flow susceptibility assessment. These selected eight indicators are: Gully Area (S1), Channel Curvature (S2), Channel Average Gradient (S3), NDVI (S4), Rainfall (S5), Average Slope Angle (S6), Loose Material Supply Length Ratio (S7), and Loose Material Volume per Square Kilometer (S8).
S1 reflects the gully’s ability to receive rainfall and produce loose material. S2 is the ratio of the curve length to the straight length of the gully channel, and reflects the drainage situation of the gully; the straighter the channel, the easier it is for loose materials to be discharged (Zhang et al., 2013). S3 is equal to the height difference of the gully divided by the curve length of the channel, and reflects the ability of water to carry material as it rushes out of the gully. S4 can indicate the vegetation cover. Areas with no vegetation cover are more susceptible to weathering and erosion due to external factors such as sunlight and rainfall as well as freeze and thaw action, thus providing more loose materials to the gullies as compared to those areas having vegetation cover (Li et al., 2020). S5 is an important factor in inducing disasters (Qiu et al., 2019). Heavy and prolonged rainfall, saturate and weakens the shear resistance of the loose materials in gullies thus ultimately forming a debris flow. In this study, we selected the 24-h maximum rainfall as an index of rainfall intensity. S6 is the average angle of the hillside in the gully and reflects the gentleness or steepness of the gullies; if the gullies are steep, the velocity of the flowing water down from the hillside will be high (Li Z. et al., 2021). Higher velocities increase the erosion potential, contributing the creation of loose material (Kritikos and Davies, 2015). S7 is the ratio of the length of loose material in the channel to the length of the entire channel, and represents the extent of loose material availability in the valley (Zhang et al., 2013). S8 reflects the current reserves of loose material in the valley, which is a fundamental condition for debris flow.
By comparing the importance of evaluation factors pairwise, a judgment matrix was constructed, as presented in Table 3. The maximum eigenvalue of the matrix was calculated to be 8.71, and the corresponding eigenvectors were determined as (0.286, 0.225, 0.342, 0.234, 0.495, 0.252, 0.340, 0.522). Subsequently, the weights of the factors were obtained by normalizing the eigenvectors, resulting in weights of (0.106, 0.083, 0.127, 0.087, 0.184, 0.093, 0.126, 0.194). The consistency index (CI) was calculated to be 0.102, and the consistency ratio (CR) was determined to be 0.072, indicating that the judgment matrix passed the consistency test. Furthermore, according to the value of each evaluation factor, it was divided into 5 levels (Table 4). The lower the grade, the safer the gully is, that is, it is less prone to debris flow.
According to the weights and level values of each factor, the possibility index of debris flow occurrence of 41 gullies can be calculated using Eq. 4, as shown in Table 5. The greater the possibility index, the greater the likelihood of a debris flow. According to the probability index of each gully, the NB method can be used to obtain the occurrence probability level of each gully (Table 6). Levels 1 to 5 represent extremely low, low, medium, high and extremely high occurrence probability of debris flow.
Based on the assessment results of the occurrence possibility, it can be observed that there are 7 gullies with an extremely high possibility of debris flow, 8 with high possibility, 8 with medium possibility, 13 with low possibility, and 5 with extremely low possibility.
Table 5. Index of occurrence possibility, value, destructiveness, and risk for some representative gullies.
Table 6. Level of occurrence possibility, value, destructiveness, and risk for some representative gullies.
The debris flow gullies with extremely high and high occurrence possibility are located in the south or southeastern parts of Mentougou District. Conversely, the gullies with extremely low and low occurrence possibilities are located in the northwestern or southwestern regions of Mentougou District, as shown in Figure 5.
By referring to the manual, the hydrographs of each debris flow potential gully under a 100-year return period were calculated. Then the debris flow runout of each gully was simulated by using SFLOW software. Figure 6 shows the simulation results. Based on the runout, the disaster-affected range of 41 debris flows in Mentougou District is determined.
Figure 6. The simulated accumulation depth of each debris flows gully in Mentougou District at the 100-year return period (A) All 41 debris flow gullies; (B–J) Enlarged view of part of the debris flow gully.
The number of population in the affected area was obtained through field surveys, as shown in Table 5. The area or volume of various buildings, farmland, and roads within the disaster-affected areas was measured using Google Earth software combined with on-site surveys, and the unit price was obtained through some public documents and also by interviewing village committees. Then through Eqs 5, 6, the economic value corresponding to each gully can be obtained, as shown in Table 5. Then using the NB method, the life value and property value were classified into 5 levels, respectively. Comparison of life value and property value levels were performed for each gully, and the larger value level was chosen as the level of disaster-affected object value (Table 6). Levels 1 to 5 correspond to extremely low, low, medium, high, and extremely high value, respectively. It can be seen that there are 4 extremely high value, 9 high value, 16 medium value, 6 low value, and 6 extremely low value, as shown in Figure 7.
According to the debris flow runout simulation, the maximum flow velocity and maximum accumulation depth of each gully were obtained (Table 5). The velocity and depth were classified into five levels through the NB method, respectively (Table 6). For each gully, the level of maximum velocity and maximum accumulation depth were compared, and the larger one was selected as the level of the destructiveness (Table 6). Levels 1 to 5 represent extremely low, low, medium, high, and extremely high destructiveness of the debris flow, respectively.
Based on the destructiveness of the debris flow, it can be seen that 10 gullies are associated with extremely high destructiveness, 8 with high destructiveness, 10 with medium destructiveness, 8 with low destructiveness, and 5 with extremely low destructiveness (Figure 8).
According to the occurrence possibility, the value of the disaster-affected object, and the destructiveness of the debris flow, the risk index of each gully can be obtained using Eq. 7 (Table 5). Using the NB method, the risk level of the gullies was classified into five levels according to the risk index, and the results are shown in Table 6. Levels 1 to 5 represent extremely low, low, medium, high, and extremely high risk of debris flow, respectively. The risk levels of each debris flow are shown in Figure 9, which shows that 3 gullies are extremely high risk, 4 are high risk, 9 are medium risk, 18 are low risk, and 7 are extremely low risk.
Wu (2010) combined the hazard and vulnerability assessment of geological disasters to obtain the geological disasters risk map for Mentougou District, and divided the risk in the study area into four levels, as shown in Figure 10. The larger the value of the risk level, the greater the risk. To test the accuracy of the debris flow risk assessment results of this study, the results of this evaluation were compared with the research results of Wu (2010). It was found that among the 7 debris flow gullies evaluated as extremely high and high risk in this study, 6 debris flow gullies are located in the high-risk areas in Figure 10, that is, areas with a risk level of 3 or 4. The comparison results show that the risk assessment results of this study are reliable.
Figure 10. Comparisons of debris flow risk assessment maps from this study and Wu (2010).
When assessing the risk associated with debris flow, it is essential to consider various factors. The first factor to consider is the possibility of disaster occurrence. Additionally, the presence of any objects that could be affected by the disaster in the area needs to be evaluated. Lastly, the level of destructiveness caused by the debris flow must be analyzed. Based on the above ideas, this paper conducted a comprehensive risk assessment of 41 debris flow gullies, and obtained a detailed risk level for each gully.
The evaluation of the possibility of debris flow occurrence involves the consideration of eight indicators. These are the gully area, channel curvature, channel average gradient, etc. These indicators have been chosen as evaluation factors to determine the level of occurrence possibility of debris flow in each gully. To evaluate these factors, an evaluation model based on the AHP method was constructed. Although the AHP method is widely used in the study of disasters, its accuracy may be influenced by the subjective ideas of assessment experts. Experts may have varying perspectives, which could result in inconsistent evaluation results.
When evaluating the value of disaster-affected objects, the focus is mainly on the value of life and property. However, this approach has some limitations as it does not consider environmental damage. Regarding the value of life, the statistics typically only count the number of inhabitants located in the disaster-affected area. However, more detailed statistics on the demographic characteristics of the inhabitants, such as their level of education, age, gender, etc., could provide more insights and enhance the credibility of the assessment results. Regarding property value, the statistics usually cover non-moving objects such as buildings, roads, and arable land, but do not include moving objects such as vehicles. The mobility of property, as well as life, poses a significant challenge in risk assessment. Future research should explore ways to address this issue and improve the accuracy of the assessment.
Based on the numerical simulation results of debris flow runout under a 100-year return period, the disaster-affected range, velocity and depth of the debris flow were obtained. The destructiveness is determined based on the maximum velocity and depth of the debris flow, but it should be recognized that the destructiveness is different in different areas within the disaster-affected range. In addition, it was necessary to select a debris flow inflow point during numerical simulation, which involves some subjectivity due to the lack of monitoring data. In addition, the current model does not consider the erosion of gullies by debris flows, which will undoubtedly affect the rationality of the simulation results. These challenges are not unique to this study but are common in current debris flow risk assessment. Addressing these issues will require a systematic and comprehensive approach that considers the complexities of debris flow movements and their impact on the environment and disaster-affected objects.
This article starts from the connotation of disaster (whether it is dangerous to humans) and considers three aspects: whether the disaster occurs, whether there are disaster-affected objects, and whether the disaster will cause damage to the disaster-affected objects, and proposes a new method for debris flow risk assessment based on the possibility of debris flow occurrence, the value of disaster-affected objects, and the destructiveness of debris flow. According to the new method, the risk levels of 41 debris flow gullies were obtained. The assessment result showed that three gullies were classified as having extremely high risk, while four gullies were classified as high risk. These extremely high and high-risk gullies are mainly located in the southern part of Mentougou District. Based on these findings, it is essential to increase monitoring efforts for debris flow risks and develop disaster shelter plans to minimize the adverse effects of debris flows in the Mentougou District.
The raw data supporting the conclusion of this article will be made available by the authors, without undue reservation.
YL: Conceptualization, Formal Analysis, Funding acquisition, Investigation, Methodology, Software, Validation, Visualization, Writing–original draft, Writing–review and editing. BZ: Data curation, Methodology, Writing–original draft, Writing–review and editing. SG: Formal Analysis, Methodology, Writing–original draft, Writing–review and editing. YuZ: Methodology, Writing–original draft, Writing–review and editing. YiZ: Conceptualization, Investigation, Methodology, Validation, Writing–original draft, Writing–review and editing. XH: Formal Analysis, Software, Visualization, Writing–original draft, Writing–review and editing. KZ: Writing–review and editing. MW: Writing–review and editing. SQ: Funding acquisition, Methodology, Resources, Supervision, Writing–original draft, Writing–review and editing. JC: Data curation, Investigation, Methodology, Supervision, Validation, Writing–original draft, Writing–review and editing.
The author(s) declare that financial support was received for the research, authorship, and/or publication of this article. The achievements described in this paper have been supported by the the Second Tibetan Plateau Scientific Expedition and Research Program (STEP) (grant no. 2019QZKK0904) and the National Natural Science Foundation of China (grant no. 42307247).
Thanks to Feifan Gu from Jilin University for his help during the writing of this article.
The authors declare that the research was conducted in the absence of any commercial or financial relationships that could be construed as a potential conflict of interest.
All claims expressed in this article are solely those of the authors and do not necessarily represent those of their affiliated organizations, or those of the publisher, the editors and the reviewers. Any product that may be evaluated in this article, or claim that may be made by its manufacturer, is not guaranteed or endorsed by the publisher.
The Supplementary Material for this article can be found online at: https://www.frontiersin.org/articles/10.3389/feart.2024.1426980/full#supplementary-material
Bahrami, S., Rahimzadeh, B., and Khaleghi, S. (2020). Analyzing the effects of tectonic and lithology on the occurrence of landslide along Zagros ophiolitic suture: a case study of Sarv-Abad, Kurdistan, Iran. Bull. Eng. Geol. Environ. 79, 1619–1637. doi:10.1007/s10064-019-01639-3
Bao, Y., Chen, J., Sun, X., Han, X., Li, Y., Zhang, Y., et al. (2019). Debris flow prediction and prevention in reservoir area based on finite volume type shallow-water model: a case study of pumped-storage hydroelectric power station site in Yi County, Hebei, China. Environ. Earth Sci. 78, 577. doi:10.1007/s12665-019-8586-4
Chang, M., Tang, C., Ni, H., and Qu, Y. (2015). Evolution process of sediment supply for debris flow occurrence in the Longchi area of Dujiangyan City after the Wenchuan earthquake. Landslides 12 (3), 611–623. doi:10.1007/s10346-015-0571-8
Chen, H. X., Zhang, L. M., Zhang, S., Xiang, B., and Wang, X. (2013). Hybrid simulation of the initiation and runout characteristics of a catastrophic debris flow. J. Mt. Sci. 10, 219–232. doi:10.1007/s11629-013-2505-z
Corominas, J., Westen, C., Frattini, P., Cascini, L., Malet, J. P., Fotopoulou, S., et al. (2014). Recommendations for the quantitative analysis of landslide risk. Bull. Eng. Geol. Environ. 73 (2), 209–263. doi:10.1007/s10064-013-0538-8
Cui, P., Zou, Q., Xiang, L., and Zeng, C. (2013). Risk assessment of simultaneous debris flows in mountain townships. Prog. Phys. Geog. 37 (4), 516–542. doi:10.1177/0309133313491445
de Scally, F. A., Owens, I. F., and Louis, J. (2010). Controls on fan depositional processes in the schist ranges of the Southern Alps, New Zealand, and implications for debris-flow hazard assessment. Geomorphology 122, 99–116. doi:10.1016/j.geomorph.2010.06.002
Han, X., Chen, J., Xu, P., and Zhan, J. (2017). A well-balanced numerical scheme for debris flow run-out prediction in Xiaojia Gully considering different hydrological designs. Landslides 14, 2105–2114. doi:10.1007/s10346-017-0850-7
Huang, C. F., Liu, A. L., and Wang, Y. (2010). A discussion on basic definition of disaster risk. J. Nat. Disast. 19 (06), 8–16. doi:10.13577/j.jnd.2010.0602
Kritikos, T., and Davies, T. (2015). Assessment of rainfall-generated shallow landslide/debris-flow susceptibility and runout using a GIS-based approach: application to western Southern Alps of New Zealand. Landslides 12 (6), 1051–1075. doi:10.1007/s10346-014-0533-6
Li, B., Tang, H., Gong, W., Cheng, Z., Li, T., and Wang, L. (2022). Numerical study of the runout behavior of the Kamenziwan landslide in the Three Gorges Reservoir region, China. Landslides 19, 963–976. doi:10.1007/s10346-021-01804-4
Li, Y., Chen, J., Zhang, Y., Song, S., Han, X. D., and Ammar, M. (2020). Debris flow susceptibility assessment and runout prediction: a case study in Shiyang gully, Beijing, China. Int. J. Environ. Res. 14, 365–383. doi:10.1007/s41742-020-00263-4
Li, Y., Qi, S., Zheng, B., Yao, X., Guo, S., Zou, Y., et al. (2023). Multi-scale engineering geological zonation for linear projects in mountainous regions: a case study of national highway 318 Chengdu-Shigatse section. Remote Sens. 15, 4619. doi:10.3390/rs15184619
Li, Y. C., Chen, J. P., Tan, C., Li, Y., Gu, F. F., Zhang, Y. W., et al. (2021a). Application of the borderline-SMOTE method in susceptibility assessments of debris flows in Pinggu District, Beijing, China. Nat. Hazards 105 (3), 2499–2522. doi:10.1007/s11069-020-04409-7
Li, Z., Chen, J., Tan, C., Zhou, X., Li, Y. C., and Han, M. X. (2021b). Debris flow susceptibility assessment based on topo-hydrological factors at different unit scales: a case study of Mentougou District, Beijing. Environ. Earth Sci. 80, 365. doi:10.1007/s12665-021-09665-9
Liu, C., Si, W., Yao, X., L, H., and Qi, S. (2024). Comprehensive risk assessment of geohazards in bangor county, northern tibet plateau. Chin. J. Geol. 59 (1), 249–258. (in Chinese with English abstract). doi:10.12017/dzkx.2024.017
Miao, C., and Liu, X. (2020). Characterization of acceptable risk for debris flows in China: comparison in debris-flow prone areas and nonprone areas. Int. J. Disast. Risk Re. 42, 101405. doi:10.1016/j.ijdrr.2019.101405
Ouyang, C., Wang, Z., An, H., Liu, X., and Wang, D. (2019). An example of a hazard and risk assessment for debris flows—a case study of Niwan Gully, Wudu, China. Eng. Geol. 263, 105351. doi:10.1016/j.enggeo.2019.105351
Qi, G., and Zhang, C. M. (2011). Analysis on material conditions causing to debris blow and its control countermeasures in Boddhidharma Trench. Hydrogeol. Eng. Geol. 38 (05), 102–109. doi:10.16030/j.cnki.issn.1000-3665.2011.05.015
Qiu, H., Cui, Y., Pei, Y., Yang, D., Hu, S., Wang, X., et al. (2019). Temporal patterns of nonseismically triggered landslides in Shaanxi Province, China. Catena 187, 104356. doi:10.1016/j.catena.2019.104356
Saaty, T. L., and Vargas, L. G. (1991). Prediction, projection, and forecasting: applications of the analytical hierarchy process in economics, finance, politics, games, and sports. Boston: Kluwer Academic Publishers.
Takahashi, T. (1981). Estimation of potential debris flows and their hazardous zones. Nat. Disast. Sci. 3, 57–89.
Tang, C., Zhu, J., Li, W. L., and Liang, J. T. (2009). Rainfall-triggered debris flows following the Wenchuan earthquake. Bull. Eng. Geol. Environ. 68 (2), 187–194. doi:10.1007/s10064-009-0201-6
Wang, S. S., and Luo, S. J. (2015). Discussion on the relationship between debris flow and formation lithology. Urban Geol. 10 (S1), 156–160. (in Chinese with English abstract).
Wang, X., Clague, J. J., Frattini, P., Qi, S., Lan, H., Zhang, W., et al. (2024). Effect of short-term, climate-driven sediment deposition on tectonically controlled alluvial channel incision. Geology 52 (1), 17–21. doi:10.1130/G51671.1
Wu, H. (2010). The research on geologic hazard risk regionalization and system of rescuing and testing in Mentougou district, Beijing. PhD Dissertation. Beijing: China University of Geosciences.
Wu, S., Chen, J., Zhou, W., Iqbal, J., and Yao, L. (2019). A modified logit model for assessment and validation of debris-flow susceptibility. Bull. Eng. Geol. Environ. 78, 4421–4438. doi:10.1007/s10064-018-1412-5
Xie, H., Zhong, D., and Jin, H. (2001). Debris flow and landslide disasters control in Mountain area of Beijing city. Bull. Soil Water Conserv. 6, 37–39. (in Chinese with English abstract). doi:10.13961/j.cnki.stbctb.2001.06.009
Xiong, K., Adhikari, B. R., Stamatopoulos, C. A., Zhan, Y., Wu, S., Dong, Z., et al. (2020). Comparison of different machine learning methods for debris flow susceptibility mapping: a case study in the Sichuan Province, China. Remote Sens. 12, 295. doi:10.3390/rs12020295
Xu, L., Liu, Z., and Guo, X. (2018). Review Study on the risk assessment of debris flow. IOP Conf. Ser. Mater. Sci. Eng. 423, 012021. doi:10.1088/1757-899X/423/1/012021
Yang, Q., Gao, J., Wang, Y., and Qian, B. (2011). Debris flow characteristics and risk degree assessment in changyuan gully, huairou district, beijing. Proc. Earth Planet. Sc. 2, 262–271. doi:10.1016/j.proeps.2011.09.042
Yu, Z., Zhan, J., Yao, Z., and Peng, J. (2024). Characteristics and mechanism of a catastrophic landslide-debris flow disaster chain triggered by extreme rainfall in Shaanxi, China. Nat. Hazards. doi:10.1007/s11069-024-06518-z
Zhan, J., Sun, Y., Yu, Z., Meng, H., Zhu, W., and Peng, J. (2024). Characterization of pre- and post-failure deformation and evolution of the Shanyang landslide using multi-temporal remote sensing data. Landslides. doi:10.1007/s10346-024-02257-1
Zhan, J., Yu, Z., Lv, Y., Peng, J., Song, S., and Yao, Z. (2021). Rockfall hazard assessment in the Taihang grand canyon scenic area integrating regional-scale identification of potential rockfall sources. Remote Sens. 14, 3021. doi:10.3390/rs14133021
Zhang, G., Cai, Y., Zheng, Z., Zhen, J., Liu, Y., and Huang, K. (2016). Integration of the statistical index method and the analytic hierarchy process technique for the assessment of landslide susceptibility in Huizhou, China. Catena 142, 233–244. doi:10.1016/j.catena.2016.03.028
Zhang, W., Chen, J., Wang, Q., An, Y., Qian, X., Xiang, L., et al. (2013). Susceptibility analysis of large-scale debris flows based on combination; weighting and extension methods. Nat. Hazards 66, 1073–1100. doi:10.1007/s11069-012-0539-0
Zhang, X., Du, D., Wu, Y., Ye, P., and Xu, Y. (2023). Theoretical and analytical solution on vacuum preloading consolidation of landfill sludge treated by freeze–thaw and chemical preconditioning. Acta Geotech. 19, 221–238. doi:10.1007/s11440-023-01892-7
Zhang, X., Ye, P., Wu, Y., and Zhai, E. C. (2022b). Experimental study on simultaneous heat-water-salt migration of bare soil subjected to evaporation. J. Hydrol. 609, 127710. doi:10.1016/j.jhydrol.2022.127710
Zhang, Y., Chen, J., Wang, Q., Tan, C., Li, Y., Sun, X., et al. (2022a). Geographic information system models with fuzzy logic for susceptibility maps of debris flow using multiple types of parameters: a case study in Pinggu District of Beijing, China. Nat. Hazards Earth Syst. Sci. 22, 2239–2255. doi:10.5194/nhess-22-2239-2022
Zhang, Y., Ge, T., Tian, W., and Liou, Y. A. (2019). Debris flow susceptibility mapping using machine-learning techniques in Shigatse area, China. Remote Sens. 11 (23), 2801. doi:10.3390/rs11232801
Zhao, M., Chen, J., Song, S., Li, Y., Wang, F., Wang, S., et al. (2023). Proposition of UAV multi-angle nap-of-the-object image acquisition framework based on a quality evaluation system for a 3D real scene model of a high-steep rock slope. Int. J. Appl. Earth Obs. Geoinformation 125, 103558. doi:10.1016/j.jag.2023.103558
Zhao, Y. (2013). Study on the characteristics and preventive measures of the debris flow in Tahe village of Mentougou Distract. MS thesis. Beijing: China University of Geosciences.
Zhu, D. (2018). The charactics and the studies of monitoring early warningon debris flow in Mentougou District in Beijing area. MS thesis. Beijing: China University of Geosciences.
Zhuo, B. X. (2001). Investigating and assessing flood and geological hazard by remote sensing technology in the Mentougou District of Beijing. Chin. J. Geol. Hazard Control, 87–91. (in Chinese with English abstract). doi:10.16031/j.cnki.issn.1003-8035.2001.04.017
Keywords: debris flow, risk assessment, runout, SFLOW model, Beijing
Citation: Li Y, Zheng B, Guo S, Zou Y, Zhang Y, Han X, Zada K, Waqar MF, Qi S and Chen J (2024) Assessment of debris flow risk in Mentougou District, Beijing, based on runout of potential debris flow. Front. Earth Sci. 12:1426980. doi: 10.3389/feart.2024.1426980
Received: 02 May 2024; Accepted: 24 May 2024;
Published: 12 June 2024.
Edited by:
Chong Xu, Ministry of Emergency Management, ChinaReviewed by:
Jianqi Zhuang, Chang’an University, ChinaCopyright © 2024 Li, Zheng, Guo, Zou, Zhang, Han, Zada, Waqar, Qi and Chen. This is an open-access article distributed under the terms of the Creative Commons Attribution License (CC BY). The use, distribution or reproduction in other forums is permitted, provided the original author(s) and the copyright owner(s) are credited and that the original publication in this journal is cited, in accordance with accepted academic practice. No use, distribution or reproduction is permitted which does not comply with these terms.
*Correspondence: Shengwen Qi, cWlzaGVuZ3dlbkBtYWlsLmlnZ2Nhcy5hYy5jbg==; Jianping Chen, Y2hlbmpwQGpsdS5lZHUuY24=
Disclaimer: All claims expressed in this article are solely those of the authors and do not necessarily represent those of their affiliated organizations, or those of the publisher, the editors and the reviewers. Any product that may be evaluated in this article or claim that may be made by its manufacturer is not guaranteed or endorsed by the publisher.
Research integrity at Frontiers
Learn more about the work of our research integrity team to safeguard the quality of each article we publish.