- 1Sichuan Earthquake Administration, Chengdu, Sichuan, China
- 2Institute of Geology, China Earthquake Administration, Beijing, China
- 3Institute for Disaster Management and Reconstruction, Sichuan University, Chengdu, Sichuan, China
- 4Remote Sensing Center of Yunnan Province, Yunnan, China
Seismic landslide susceptibility evaluation models are usually built on the basis of historical sample data; however, the evaluation results are often unsatisfactory when the environmental settings differ between the historical sample data region and application region. Therefore, similarity between the environmental settings is important for the application of such models. In this paper, a seismic landslide susceptibility evaluation model was first built using data from the 2008 Ms 8.0 Wenchuan earthquake-induced landslide, and the model was then used to evaluate the 2022 Ms 6.8 Luding earthquake area. In addition, the grade of susceptibility is typically represented by the landslide density, which is insufficient for capturing the details of landslides, such as their sizes, frequencies, and spatial distribution patterns. The authors therefore use a large and concentrated landslide as the susceptibility grade for the Luding earthquake area. The test results demonstrate that these two areas have similar background environments. The area under the curve (AUC) value of the receiver operating characteristics (ROC) curve of the evaluation accuracy for the model applied to the Luding earthquake area is 0.889, which indicates relatively high accuracy. Besides, the results also demonstrate that the evaluations are consistent with the disaster situation of the Moxi Platform, Wandong Village, as well as the Dagangshan Hydropower Station area. Therefore, it is reliable to apply the susceptibility evaluation model based on the Wenchuan earthquake data to the Luding earthquake area. These results show that better evaluations can be obtained based on environmental similarity tests between the areas used for historical data modeling and areas to which the models are applied.
1 Introduction
Earthquake-induced landslides are the result of multiple factors, such as ground motions, geology, and geomorphology (Xu et al., 2009, 2013; Havenith, 2022). Seismic landslide susceptibility evaluations are helpful for reducing the losses caused by landslides and are also the focus of the present research on prevention and mitigation of earthquake-induced disasters (Micu et al., 2023; Yuan et al., 2013; Li et al., 2013; Dai et al., 2023). Current susceptibility evaluation models are usually based on historical sample data from around the world, but background similarity tests between the historical sample regions and current application regions are not performed often before implementing these models (Ullah et al., 2022; Ozdemir et al., 2013; Chen et al., 2020; Quesada et al., 2019, Quesada RA, 2021a; Quesada RA, 2021b; Quesada RA, et al., 2021c; Arroyo Solórzano et al., 2022; Porras et al., 2021). Therefore, we attempted to conduct background environment similarity tests before model application to ensure that the susceptibility evaluation model used is reasonable; this study involved assessment of the evaluation model developed for the Wenchuan earthquake region for application to the Luding earthquake region that has a similar background environment. In addition, susceptibility evaluation models currently use landslide density as the index representing susceptibility grade (Nowicki et al., 2014; Wang et al., 2017; Fan et al., 2018a; Sun et al., 2018; Sun et al., 2020; Liu et al., 2021a). Landslide characteristics such as scale, frequency, and spatial distribution pattern are often quite different in different regions; thus, the landslide density index cannot comprehensively reflect the landslide characteristics (Liu et al., 2021b). The spatial distribution pattern of a landslide is hence used as the evaluation index in this work to reflect more detailed characteristics that could improve the evaluation results. Furthermore, the evaluation model implemented in this work uses only the significant influencing factors and not all factors that improve the accuracy of the evaluation results.
2 Study area
The southeastern edge of the Tibetan Plateau is a region with many active tectonics, large terrain differences, and frequent earthquakes. Since the 2008 Wenchuan Ms 8.0 earthquake, several earthquakes have occurred in the region, such as the Ms 7.0 Lushan earthquake in 2013, Ms 6.5 Ludian earthquake in 2014, Ms 7.0 Jiuzhaigou earthquake in 2017, and Ms 6.8 Luding earthquake in 2022 (Huang et al., 2008; Zhang et al., 2008; Fan et al., 2018b; Liu et al., 2023). As the earthquake with the highest magnitude in southwest China in recent years, the 2008 Wenchuan Ms 8.0 earthquake induced the largest number of landslides, causing serious disasters. In the early period following this earthquake, researchers counted nearly 8,000 landslides in the region; five months later, more than 10,000 landslides had occurred (Huang et al., 2009). In the 6-year period following the earthquake, a total of 197,481 landslides were reported, whose data included both the locations and size of the landslides (Xu et al., 2014c) that provide details for studying the landslide distribution pattern.
The 2008 Wenchuan earthquake originated in the Longmen Mountain thrust tectonic belt on the eastern edge of the Tibetan Plateau and induced two reverse faults (Xu et al., 2008), namely, the Beichuan-Yingxiu and Guanxian-Jiangyou faults. This earthquake area has high terrain in the northwest and low terrain in the southeast, including alluvial plains (elevation of approximately 500 m), alpine landforms (elevation of approximately 2,000–5,000 m), and plateau landforms (elevation of approximately 4,000–5,000 m). The relative altitude difference in this area is more than 4,000 m, and the slope ranges between 20° and 50° (Qiao et al., 2009). The earthquake-induced landslides were distributed in an elliptical zone of area approximately 110,000 km2 along the Beichuan-Yingxiu surface rupture, and the high-density landslides were concentrated in an area southwest of this surface rupture. The number, scale, and degree of concentration of these landslides in the hanging wall of the fault were significantly larger than those in the footwall, indicating the hanging wall effect.
3 Landslide clustering analysis
The characteristics of landslide distribution are usually described as “concentrated in a certain zone” or “concentrated along the fault,” which do not reflect the “clustering degree,” “clustering scale,” and “clustering location” of the landslides. In this paper, the clustering characteristics of landslides were quantified via clustering analysis, and the susceptibility evaluation model of the large-scale concentrated landslide was constructed. Large-scale concentrated landslides are quantified by local spatial autocorrelation analysis, which is also called as landslide clustering analysis. This method can obtain the clustering locations of large and small landslides; additionally, it can obtain the locations where large landslides are surrounded by small landslides as well as locations where small landslides are surrounded by large landslides. This analysis method involves calculating the local Moran’s I value (Eq. 1), z-score, p-value, and clustering type of each landslide [Copyright(C) 1995–2013 Esri].
where Xiis the area value of landslide i;
The score of the statistics is calculated as Eq. 2
The ZIi score is statistically calculated as Eqs 3–5
In the study area, the results of landslide clustering analysis showed that 1) the zone extending along the Beichuan-Yingxiu surface rupture, from the epicenter to the southwest, was predominantly characterized by small concentrated landslides. 2) The zone extending from the epicenter to the north was characterized by large landslides surrounded by smaller ones. 3) The zone along the Minjiang River was predominantly characterized by large concentrated landslides. 4) The zone extending along the Beichuan-Yingxiu surface rupture, from the epicenter to the northeast, showed a transition from “small scattered pattern” to “small concentrated pattern” until the Xiaoyudong surface rupture that interrupts the Beichuan-Yingxiu surface rupture. 5) The Beichuan-Yingxiu surface rupture reemerged on the northeast side of the Xiaoyudong surface rupture and continued to extend further northeast while a parallel Guanxian-Jiangyou surface rupture became apparent simultaneously; the pattern of landslides observed on the hanging walls of these two parallel surface ruptures indicated a concentration of large landslides, with the smaller landslides being surrounded by the larger landslides. 6) The location where the Guanxian-Jiangyou surface rupture terminated was the point at which the largest single landslide of the Wenchuan earthquake, known as the Daguangbao landslide, occurred (Tan et al., 2013) (Figure 1).
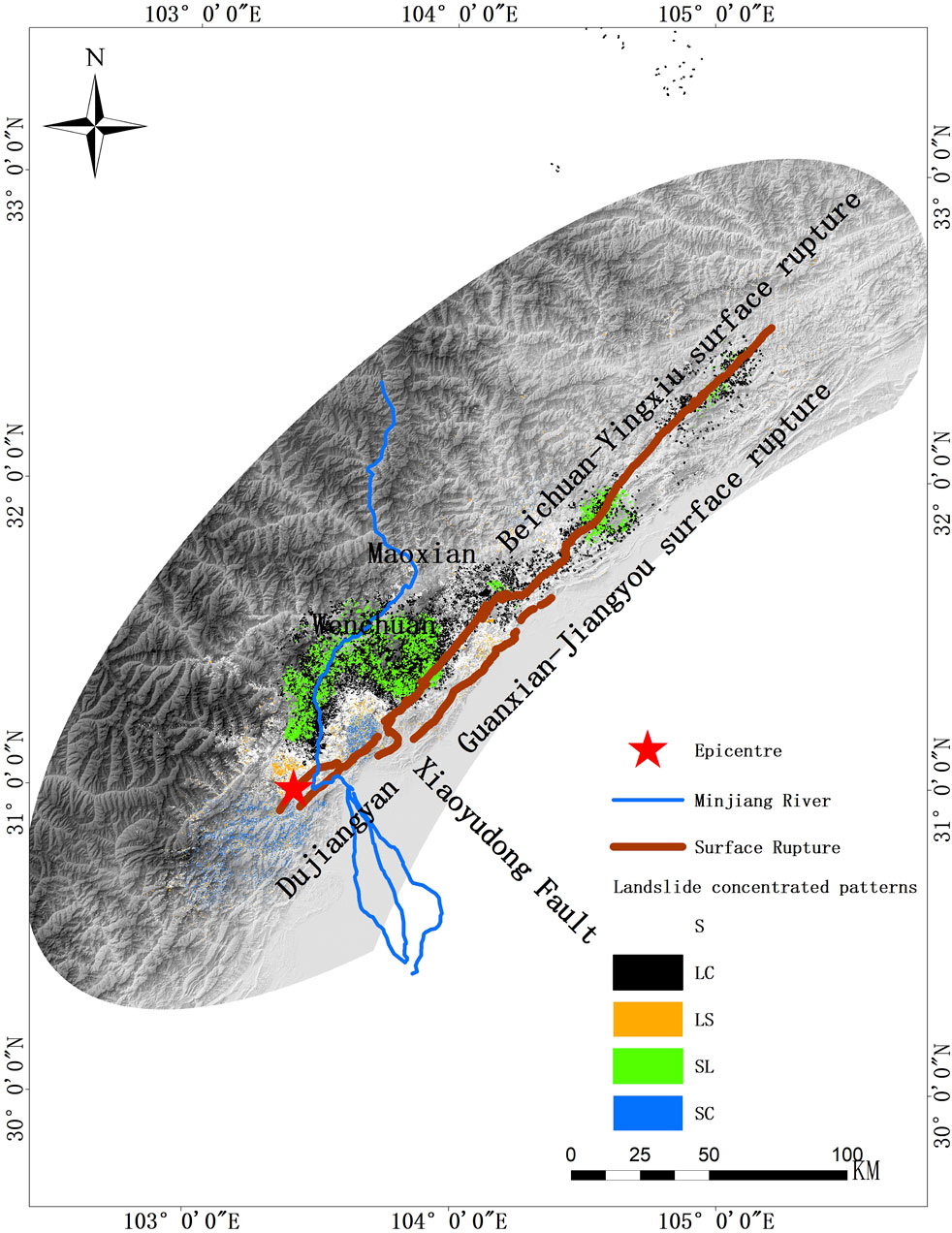
Figure 1. Spatial distribution of landslides in the Wenchuan earthquake area. Note: S for scattered landslides; LC for large concentrated landslides; LS for large landslide surrounded by small landslides; SL for small landslide surrounded by large landslides; SC for small concentrated landslides.
4 Landslide susceptibility evaluation model
Along the Beichuan-Yingxiu surface rupture, the density of landslides on the hanging wall was higher than that on the footwall; there were approximately 150,000 landslides on the hanging wall along the Beichuan-Yingxiu surface rupture that constituted about 80% of the total number of landslides recorded. The study area encompassed a 20 km range on both sides of the surface rupture. The study area accounted for approximately 100,000 landslides, constituting 52% of the total number of events reported. The study area also exhibited various landslide patterns, including large concentrated pattern, small concentrated pattern, large landslides surrounded by small landslides, and large single landslides. These diverse patterns provide valuable foundational data for the present study (Figure 2).
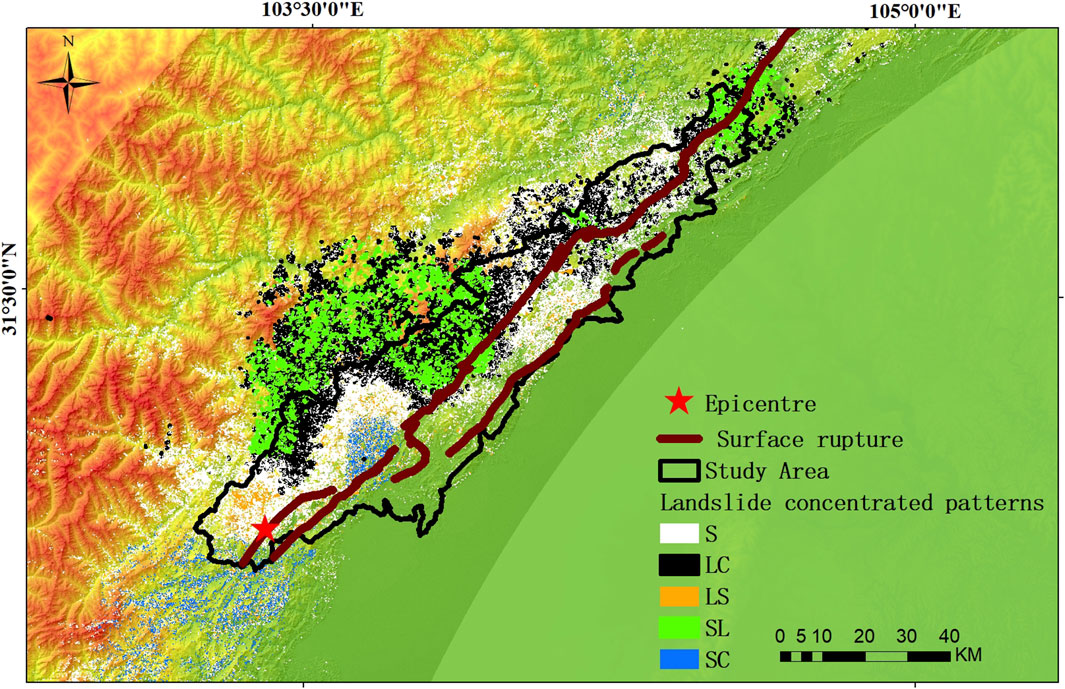
Figure 2. Different landslide patterns in the Wenchuan earthquake study area. Note: S for scattered landslides; LC for large concentrated landslides; LS for large landslide surrounded by small landslides; SL for small landslide surrounded by large landslides; SC for small concentrated landslides.
4.1 Collinearity diagnosis
Based on the landslide clustering analysis, five landslide patterns were obtained as follows: 1) large concentrated landslides; 2) large landslide surrounded by small landslides; 3) small landslide surrounded by large landslides; 4) small concentrated landslides; 5) scattered landslides. Among these five patterns, the large concentrated landslides were considered to be the most hazardous, so more attention was focused on this particular pattern. In this paper, these five patterns were divided into two evaluation indexes to construct the susceptibility evaluation model. Patterns 1) and 2) were categorized into the large concentrated landslide (LCL) evaluation index, while the remaining three patterns were classified into the non-large concentrated landslide (NLCL) evaluation index. The values of the indexes were considered to be dependent variables, where 1 represented the LCL and 0 represented the NLCL evaluation indexes.
The evaluation model incorporated 10 influencing factors, including slope aspect, slope angle, elevation, peak ground acceleration (PGA), intensity, surface rupture distance, fault-locked segment, duration of strong motions, lithology, and river distance. Before constructing the model, the collinearity of the influencing factors was tested to identify and exclude those factors that exhibited multicollinearity; collinearity diagnosis by regression analysis was employed to assess the presence of multicollinearity among the influencing factors. Factors exhibiting high levels of multicollinearity were excluded from the model to ensure the independence and reliability of the remaining factors. In the diagnosis results, if the tolerance value of a factor was less than 0.1 or the variance inflation factor (VIF) exceeded 10, it was considered to be multicollinear. In such cases, the corresponding factor was excluded from the model to mitigate the effects of multicollinearity and ensure the reliability of the remaining factors. From the results presented in Table 1, all 10 influencing factors were found to have tolerance values greater than 0.1 and VIF values less than 10, indicating the absence of multicollinearity. Thus, all 10 factors were utilized to construct the model without the need for exclusion.
4.2 Significant independent variables selection
We used watershed as the evaluation unit for this selection. There were a total of 231 watershed regions within the designated zone. Based on the landslide clustering analysis, 83 watershed regions exhibited the characteristics of a large landslide concentrated distribution, and the remaining 148 watershed areas were characterized as having a non-large landslide concentrated distribution (Figure 3). These two characteristics corresponded to the two evaluation indexes LCL and NLCL described above, which help in understanding the spatial distributions and patterns of landslides in the study area. Among the watershed areas, 70% were designated as training samples, and the remaining 30% were used as verification samples. In the evaluation model, the landslide concentrated evaluation index of each watershed area was considered to be the dependent variable, while the 10 influencing factors were treated as the independent variables. Logistic regression analysis was then used to identify the significant independent variables in the evaluation model; this statistical technique is particularly suitable when the dependent variable is binary or dichotomous, as is the case with the landslide concentrated evaluation indexes in this work. Logistic regression helps to assess the relationships between the independent variables (influencing factors) and probability of a watershed area being classified into one of the two categories. By analyzing the coefficients and significance levels of the independent variables, we determined the variables that had significant impacts on the predictions of the dependent variables. The non-significant independent variables were excluded from the model by evaluating the statistical significance of each independent variable and removing those that did not contribute significantly to the model’s predictive power. By eliminating the non-significant variables, the model is more focused and robust, including only those variables that have meaningful impacts on the dependent variables. In the model construction process, six significant independent variables were finally selected to construct the model: slope aspect, elevation, intensity, fault-locked segment, duration of strong motions, and river distance (Table 2). The regression coefficients associated with these factors were used as the weights in the construction of the model.
Here, PWC is the evaluation probability of the LCL, and its value is between 0 and 1; a higher value of PWC indicates a greater possibility of LCL occurrence in the watershed area. ZWC is the weighted sum of the influencing factors. These influencing factors were indicated by letters as follows: S for aspect, E for elevation, I for intensity, F for fault-locking section, R for river distance, and D for strong movement duration.
5 Model application
5.1 Background environment similarity test
The Luding area is situated at the boundary of the Sichuan-Yunnan rhombic block, which is considered as a potential seismic area. In this study, the Luding earthquake region was used as the application region for the susceptibility evaluation model for earthquake prevention evaluation. The landslides induced by the 2022 Ms 6.8 Luding earthquake were used as the verification samples for the model. Before applying the model, a background environment similarity test was conducted between the Wenchuan and Luding earthquake regions to assess the similarities or comparability of the environmental conditions and factors. The comparisons were made between the factors influencing the Wenchuan earthquake study area and Luding earthquake application area.
5.1.1 Seismic factor similarity test
The Wenchuan earthquake area is situated at the eastern edge of the Bayankala block, which is known for its high seismic activity and considered as one of the most active blocks within the Tibetan Plateau. The Luding earthquake area is located at the eastern edge of the Sichuan-Yunnan block, which is also recognized as one of the most active blocks within the Tibetan Plateau and characterized by significant tectonic activity.
The epicenter of the 2022 Luding earthquake was located in the Hailuogou Glacier Forest Park at a depth of 16 km. A surface rupture along the Moxi fault is observed south of the epicenter, with a length of 15–25 km, exhibiting a nearly straight dip and northwest strike, consistent with the movement and strike of the Xianshuihe fault (Xu et al., 2022; Li et al., 2023). The Xianshuihe fault is indeed located at the boundary between the Bayankala and Sichuan-Yunnan blocks, spanning a total length of approximately 400 km; this fault begins in Ganzi and extends through Luhuo County, Daofu County, Kangding County, Moxi Town, before ending south of Xinmin Town (Deng et al., 2003; Zhang et al., 2003). The Xianshuihe fault and the southwest part of the Longmenshan fault interact at Moxi Town in Luding County. The seismic faults associated with the Wenchuan earthquake are the Guanxian-Jiangyou and Beichuan-Yingxiu faults. Both these faults are characterized as thrust faults with right-lateral strike-slip components. The Beichuan-Yingxiu fault dips to the northwest at an angle greater than 70°.
The Luding earthquake fault is a left-lateral strike-slip fault. Generally, there are differences between thrust and strike-slip earthquakes in terms of the distribution and attenuation of ground motions. In strike-slip earthquakes, the ground motions on the active plate are typically stronger than those on the passive plate. Ground motions from strike-slip earthquakes are rapidly attenuated with distance, particularly beyond zones that are approximately 5 km away from the fault. As a result, landslides induced by strike-slip earthquakes tend to be concentrated within a narrow region. The occurrence of landslides decreases as the distance from the fault increases. In a thrust fault, the ground motions are generally larger at the hanging wall than at the footwall; this is because the hanging wall experiences greater compression and uplift during an earthquake. Additionally, the rate of attenuation of ground motions for a thrust fault is typically lower than that for a strike-slip fault. This means that the intensity of ground shaking from a thrust earthquake decreases more slowly with distance from the fault. As a result, landslides induced by thrust faults can occur over a wider region compared to those induced by strike-slip faults (Figures 4, 5).
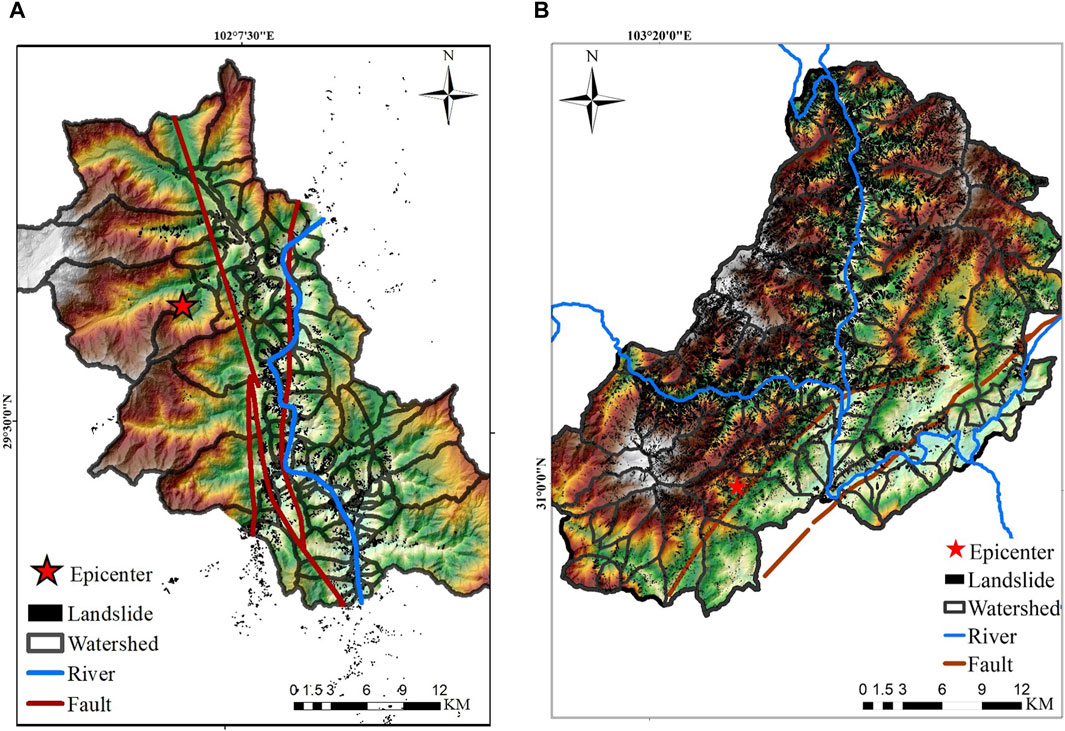
Figure 4. Surface ruptures and landslides in the (A) Luding and (B) Wenchuan earthquake typical zones.
Despite the differences in magnitude, intensity, ground motion characteristics, landslide numbers, and scale between the Wenchuan and Luding earthquakes, both zones were near surface ruptures and are considered as areas with slow ground motion attenuation. In these zones, the ground motions do not attenuate rapidly with distance from the epicenter or fault rupture, meaning that the intensity of ground shaking remains relatively high at areas near the surface rupture. These zones are characterized by relatively strong ground motions compared to other areas and are therefore considered as typical zones in this paper. The spatial distribution pattern of landslides is similar for these two earthquake typical zones. It is observed that landslides tend to align along the faults and exhibit a high density of occurrence in both cases. In addition, the dip angles of both surface ruptures are large (Table 3). The steep dip angle of the surface rupture in conjunction with the characteristics of the lithology plays a significant role in increasing the probability of landslide occurrences in both earthquake areas. The high dip angles generate significant stresses, which can lead to the development of joints and cracks in brittle rocks. The dominant lithology is metamorphic volcanic rock in the Wenchuan earthquake area, while that in the Luding earthquake area is granite. Both these lithologies are characterized as hard rocks with well-developed structural planes that serve as potential sliding surfaces. Based on an analysis of the seismic influencing factors, including the attenuation rate of ground motions and dip angle of the surface rupture, it is concluded that the typical zones in both earthquake areas have similar influences on landslides.
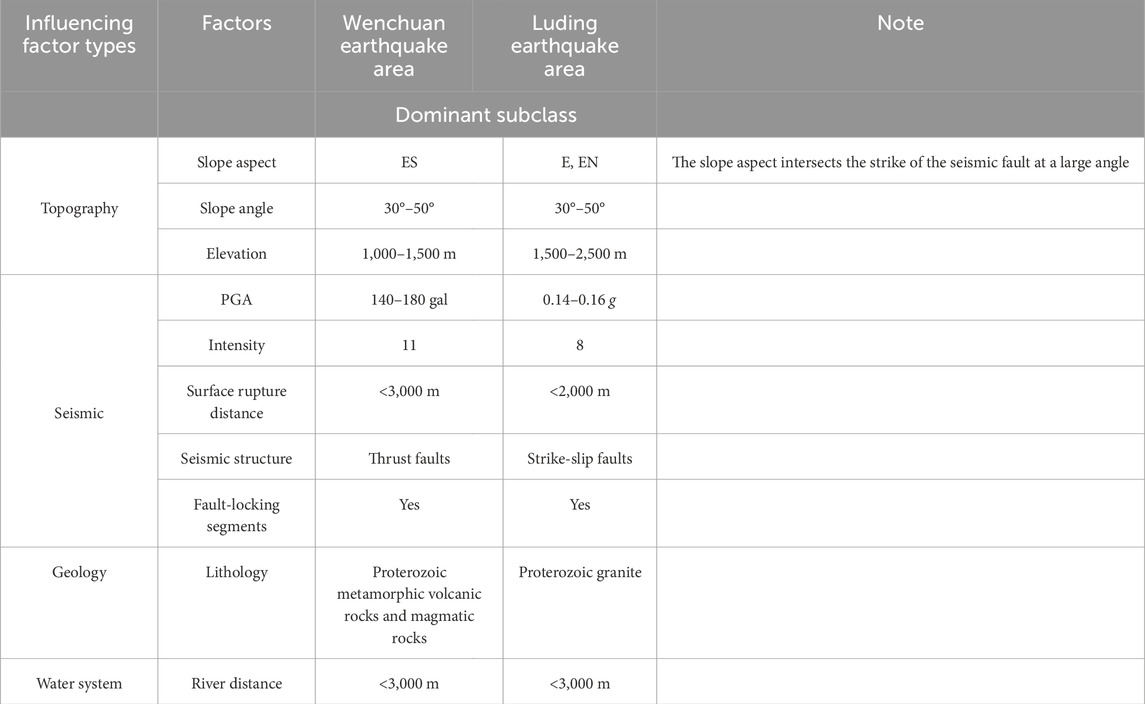
Table 3. Comparison of background factors influencing the Wenchuan and Luding earthquake typical zones.
5.1.2 Topographic and geological factor similarity test
The Luding earthquake occurred in a high mountain area with a very large altitude difference of approximately 6,570 m and the highest altitude being 7,556 m, indicating steep slopes and varied topography within the region. This area is traversed by the Dadu River, which is the largest tributary of the Minjiang River. The dominant lithology in this zone includes proterozoic granite, permian quartzite, and quaternary gravel and sand. When the dominant slope aspects intersect the strike of the seismic fault at a large angle, they result in strong destructive effects on slopes during earthquakes. Further, the combination of mountainous valley landforms, well-developed rivers, hard lithology, and cracked rocks increases the possibility of occurrence of large landslides in both the Wenchuan and Luding earthquake typical zones (Table 3).
5.2 Evaluation model application
5.2.1 Model construction
For the purpose of this paper, the zones along both sides of Dadu River in the Luding earthquake area are selected as the application zones, and these are within 10 km from the fault, covering an area of approximately 884.5562 km2. The Luding earthquake area zone includes approximately 78 watershed regions of similar sizes. The model based on the Wenchuan earthquake (Eqs. (6, 7)) was used to construct the evaluation model of the Luding application zone (Eqs. (8, 9)). The evaluation model is based on 10 influencing factors, namely, slope aspect, slope angle, elevation, PGA, intensity, surface rupture distance, fault-locked segment, duration of strong motions, lithology, and river distance (Figure 6). The landslide susceptibility of the Luding earthquake zone is classified on the basis of the susceptibility evaluation grade (Figure 7). The receiver operating characteristics (ROC) curve test was performed for the landslide samples, and the results show that the area under the curve (AUC) value was 0.889; this indicates that the model evaluation result is high (Figure 8).
where PLD is the evaluation probability of LCLs in the Luding earthquake zone having a value between 0 and 1; the larger the value of this probability, the greater is the possibility of LCL occurrence. ZLD is the weighted sum of the influencing factors, where S indicates slope aspect, E is the elevation, I is the intensity, F is the fault-locked segment, R is the river distance, and D is the duration of strong motions.
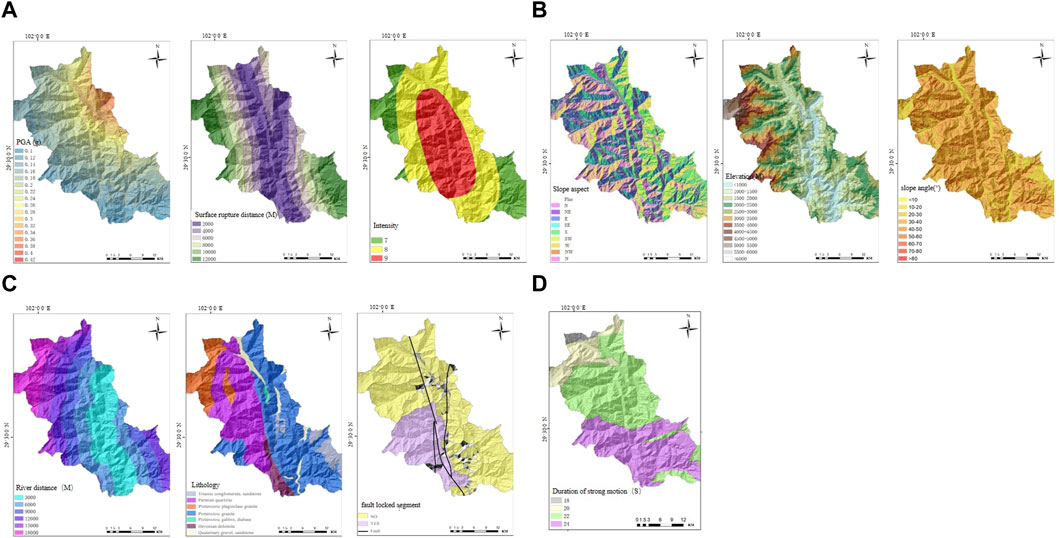
Figure 6. Ten types of factors influencing the Luding earthquake zone: (A) peak ground acceleration (PGA), surface rupture distance, and intensity; (B) slope aspect, elevation, and slope angle; (C) river distance, lithology, and fault-locked segment; (D) duration of strong motions.
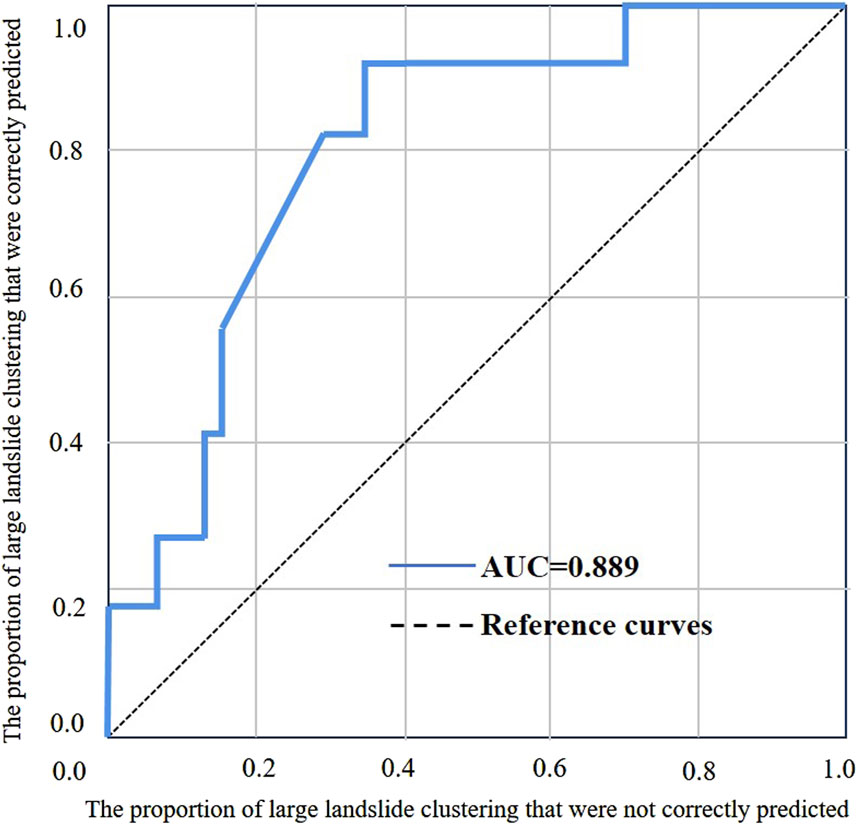
Figure 8. Receiver operating characteristics (ROC) curve of the large concentrated landslides in the Luding earthquake zone.
5.2.2 On-site investigation
Upon occurrence of the Luding earthquake, our research team promptly arrived at the affected site to carry out investigations. The specific locations investigated were Moxi Town and Detuo Town in Luding County as well as Wanggangping Town in Shimian County. Most of the large-scale landslides in the Luding earthquake area were distributed along the river and roads, resulting in road burial, collapse, and roadbed damage. In this paper, we compared the on-site investigation results and model evaluation results of LCLs in Moxi Platform, Wandong Village of Detuo Town, and Wanggangping Town (Figure 9). The details of these evaluations are as follows:
1) The landslide disaster in Wandong Village was more serious than that at the other two sites. The Wandong River channel was blocked by the large earthquake-induced avalanche, which created a dammed lake and endangered the safety of downstream areas. Many houses were buried near the Shenyun Hot Spring Villa of Wandong Village. Here, the alluvium from the upper stream accumulated to a height of nearly 10 m; the area is approximately 1 km from the dammed lake, and the road to the village was buried by the landslides and debris flows, resulting in diversion of the original river path (Figure 10). We compared the on-site investigation results with those of the landslide susceptibility evaluations in Wandong Village and found that both of these were well-matched with “very high” grade (Figures 9, 10).
2) The scale and number of landslides in Wanggangping Town was smaller than that at the other two sites. There were some small landslides at the exit of Taoba Tunnel and south side of Dagangshan hydropower station. Moreover, scattered landslides were also noted on both sides of the road from Taoba Tunnel to Aiguo Village. The on-site investigations were consistent with the landslide susceptibility evaluation results, with the evaluation grade being “high” (Figures 9, 11).
3) Although Moxi Platform was the closest site to the epicenter, the scale of landslides in this area was not as large as that in Wandong Village. The landslides were concentrated on both sides of the river. The on-site findings were consistent with the landslide susceptibility evaluation results, with the evaluation grade being “high” (Figures 9, 12).
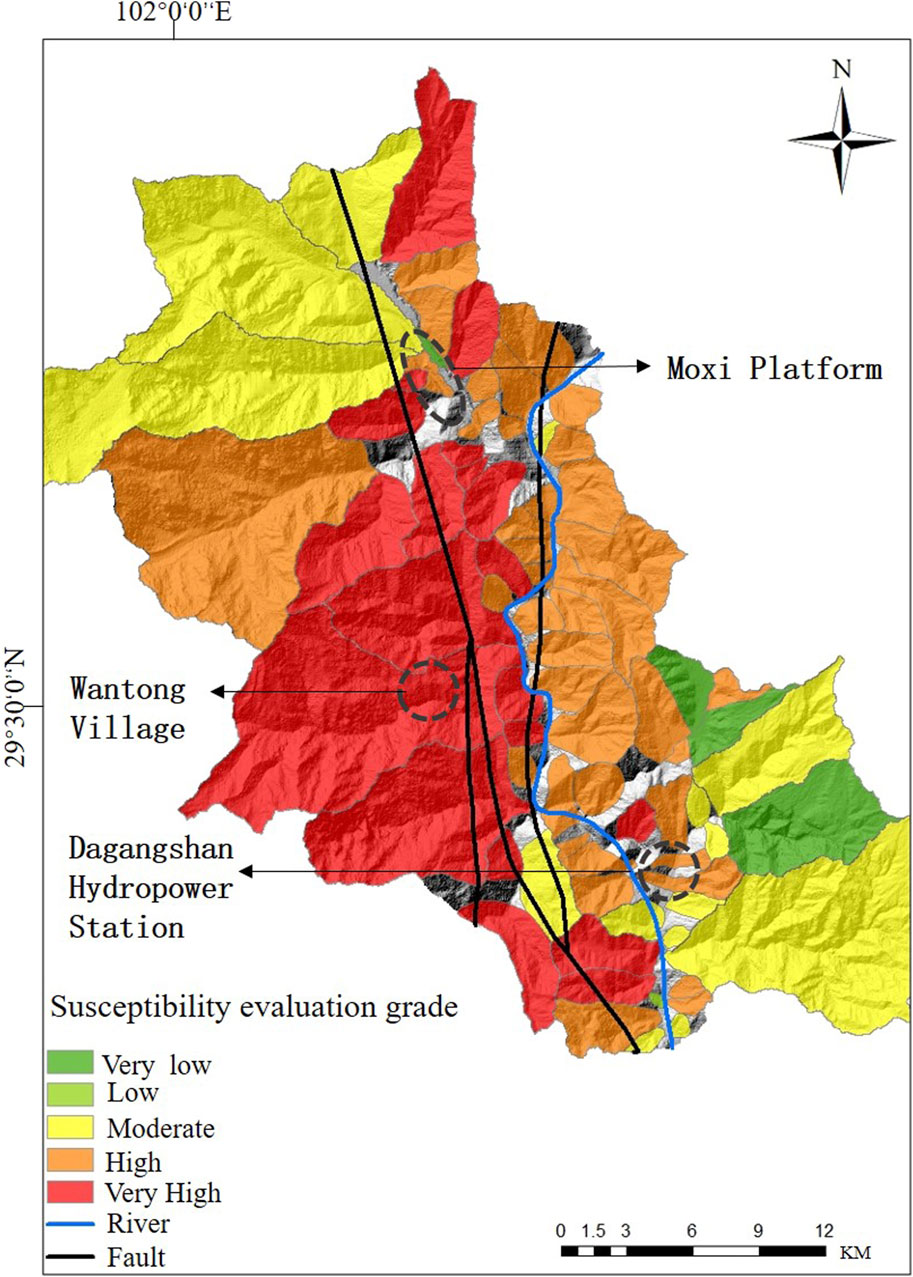
Figure 9. Locations of Moxi platform, Wantong village of Detuo town, and Dagangshan hydropower station.
By comparing the on-site and evaluation results for the three investigation sites, two sites were found to match well, and one site was almost completely matched. It is thus concluded that the model used for the Luding earthquake area is applicable. The technical procedures used to develop the susceptibility evaluation model based on the Wenchuan earthquake area can thus be used in other potential earthquake areas with similar background environments.
6 Discussion
Nowadays, most of the seismic landslide susceptibility evaluation models are not implemented based on similarities in the background environments. The extant models were built using historical data from all over the world and are being applied to different regions; however, these regions may have different background environments, meaning that the factors influencing the models may not be suitable for all regions. Only regions with similar background environments have higher probabilities of success when applying such models. Most of the available studies have not addressed this issue and have not conducted tests for similarities in the background environments. Our research focuses on this issue and such similarity tests. Conducting a background environment similarity test imposes specific requirements on both the historical sample region and current application region; both regions should have similar geologies, topographies, and landform characteristics. Additionally, it is preferable for both regions to be in close proximity.
In recent years, researchers have made great efforts to build and preserve historical samples in their original forms. Their findings have provided valuable data for this research (Martinez et al., 2021; Gnyawali et al., 2016; Xu et al., 2014a; Xu C, et al., 2014b; Xu C, et al., 2014c; Ferrario et al., 2019; Li et al., 2014). However, finding suitable samples still remains a challenge. The two regions studied herein are one of the few pairs of samples that meet the required criteria. Hence, this research was conducted with the hope that the findings could provide ideas for future research endeavors. As more landslide samples are restored, this type of research can be expanded further in the future.
7 Conclusion
In this paper, the susceptibility evaluation model based on the Wenchuan earthquake area was applied to the Luding earthquake area that has a similar background environment. The evaluation model results demonstrate good accuracy. Additionally, we utilized LCLs as the evaluation index instead of landslide density. This choice allowed us to highlight the spatial distribution characteristics of the significant landslides, which is crucial for post-earthquake relief efforts. Therefore, the evaluation results presented in this paper can provide more valuable information. The main conclusions of this work are as follows:
1) Before using historical sample data to construct susceptibility evaluation models for earthquake-induced landslides, it is important to conduct background environment similarity tests between the historical and application areas. Accurate results can then be obtained if the model is applied to an area with a similar background environment.
2) Different earthquakes can trigger different landslide distribution characteristics. Landslide density often reflects only the total number of landslides, and the distribution degree of the landslide scale reflects the spatial distribution characteristics of the landslides. It is found that the evaluation index should be based on the pattern of LCLs, rather than relying solely on the landslide density. This approach allows obtaining more useful evaluation results.
3) The susceptibility evaluation model used in this work considers only the significant influencing factors rather than all factors to enhance model efficiency. Thus, six significant independent variables, namely, slope aspect, elevation, intensity, fault-locked segment, duration of strong motions, and river distance, were used to construct the model.
Data availability statement
The original contributions presented in the study are included in the article/supplementary material; further inquiries can be directed to the corresponding authors.
Author contributions
XL: conceptualization, formal analysis, investigation, methodology, visualization, writing–original draft, and writing–review and editing. XY: writing–review and editing and project administration. RY: funding acquisition and writing–review and editing. RX: methodology and writing–review and editing. CL: writing–review and editing.
Funding
The authors declare that financial support was received for the research, authorship, and/or publication of this article. This research was financially supported by the National Non-profit Fundamental Research Grant of China, Institute of Geology, China Earthquake Administration (grant no. IGCEA2202), Youth Key Projects of Earthquake Emergency and Information (grant no. CEAEDEM202316), and Science and Technology Department of Sichuan Province (grant no. 2022JDRC0002).
Conflict of interest
The authors declare that the research was conducted in the absence of any commercial or financial relationships that could be construed as a potential conflict of interest.
Publisher’s note
All claims expressed in this article are solely those of the authors and do not necessarily represent those of their affiliated organizations, or those of the publisher, the editors, and the reviewers. Any product that may be evaluated in this article, or claim that may be made by its manufacturer, is not guaranteed or endorsed by the publisher.
References
Arroyo Solórzano, M., Quesada, R. A., and Barrantes Castillo, G. (2022). Seismic and geomorphic assessment for coseismic landslides zonation in tropical volcanic contexts. Nat. Hazards 114 (3), 2811–2837. doi:10.1007/s11069-022-05492-8
Chen, W., Chen, Y., Tsangaratos, P., Ilia, I., and Wang, X. (2020). Combining evolutionary algorithms and machine learning models in landslide susceptibility assessments. Remote Sens. 12, 3854. doi:10.3390/rs12233854
Dai, L., Fan, X., Wang, X., Fang, C., Zou, C., Tang, X., et al. (2023). Coseismic landslides triggered by the 2022 Luding Ms6.8 earthquake, China. Landslides 20, 1277–1292. doi:10.1007/s10346-023-02061-3
Deng, Q. D., Zhang, P., Ran, Y., Yang, X., Min, W., and Chu, Q. (2003). Basic characteristics of active tectonics of China. Sci. China Ser. D-Earth Sci. 46, 356–372. doi:10.1360/03yd9032
Fan, X. M., Domènech, G., Scaringi, G., Huang, R., Xu, Q., Tristram, C., et al. (2018a). Spatio-temporal evolution of mass wasting after the 2008 Mw 7.9 Wenchuan earthquake revealed by a detailed multi-temporal inventory. Landslides 15, 2325–2341. doi:10.1007/s10346-018-1054-5
Fan, X. M., Scaringi, G., Xu, Q., Zhan, W., Dai, L., Li, Y., et al. (2018b). Coseismic landslides triggered by the 8th August 2017 Ms 7.0 Jiuzhaigou earthquake (Sichuan, China): factors controlling their spatial distribution and implications for the seismogenic blind fault identification. Landslides 15, 967–983. doi:10.1007/s10346-018-0960-x
Ferrario, M. F. (2019). Landslides triggered by multiple earthquakes: insights from the 2018 Lombok (Indonesia) events. Nat. Hazards 98, 575–592. doi:10.1007/s11069-019-03718-w
Gnyawali, K. R., and Adhikari, B. R. (2016). Spatial relations of earthquake induced landslides triggered by 2015 Gorkha earthquake Mw 7.8. Eur. Geosci. Union General Assem. Conf. Abstr. 18, 18429.
Havenith, H. B. (2022). “Recent earthquake-triggered landslide events in central asia, evidence of seismic landslides in the lesser caucasus and the carpathians,” in Coseismic landslides. Springer natural Hazards. Editors I. Towhata, G. Wang, Q. Xu, and C. Massey (Singapore: Springer).
Huang, R. Q., and Li, W. L. (2009). Analysis of the geo-hazards triggered by the 12 may 2008 wenchuan earthquake, China. Bull. Eng. Geol. Environ. 68 (3), 363–371. doi:10.1007/s10064-009-0207-0
Huang, R. Q. R., and Qiang, Xu (2008). Typical catastrophic landslides in China. J. Eng. Geol. 16 (5), 1.
Li, C. Y., Sun, K., Jun, Ma, Junjie, Li, Liang, M., and Fang, L. (2023). The 2022 M6.8 LuDing Earthquake: a complicated event by faulting of the MoXi segment of the XianShuiHe fault zone. Seismol. Geol. 44 (6), 1648–1666.
Li, G., West, A. J., Densmore, A. L., Jin, Z., Parker, R. N., and Hilton, R. G. (2014). Seismic mountain building: landslides associated with the 2008 Wenchuan earthquake in the context of a generalized model for earthquake volume balance. Geochem. Geophys. Geosystems 15, 833–844. doi:10.1002/2013gc005067
Li, W. L., Huang, R., Xu, Q., and Tang, C. (2013). Rapid prediction of co-seismic landslides triggered by Lushan earthquake, Sichuan, China. Journal of Chengdu University of Technology Science and Technology Edition, 264–274. 040.003.
Liu, X. M., Su, P., Li, Y., Xia, Z., Ma, S., Xu, R., et al. (2023). Spatial distribution of landslide shape induced by Luding Ms6.8 earthquake, Sichuan, China: case study of the Moxi Town. Landslides 20, 1667–1678. doi:10.1007/s10346-023-02070-2
Liu, X. M., Su, P., Li, Y., Zhang, J., and Yang, T. (2021a). Susceptibility assessment of small, shallow and clustered landslide. Earth Sci. Inf. 14, 2347–2356. doi:10.1007/s12145-021-00687-2
Liu, X. M., Su, P., Yong, Li, Rui, Xu, Jun, Z., Yang, T., et al. (2021b). Spatial patterns and scaling distributions of earthquake-induced landslides—a case study of landslides in watersheds along dujiangyan–wenchuan highway. Front. Earth Sci. 9 (10), 3389. doi:10.3389/feart.2021.659152
Martinez, S. N., Allstadt, K. E., Slaughter, S. L., Schmitt, R., Collins, E., Schaefer, L. N., et al. (2021). Landslides triggered by the august 14, 2021, magnitude 7.2 nippes, Haiti, earthquake: U.S. Geological survey open-file Report.17, 1112.
Micu, M., Micu, D., and Havenith, H. B. (2023). Earthquake-induced landslide hazard assessment in the vrancea seismic region (eastern carpathians, Romania): constraints and perspectives. Geomorphology 427, 108635. doi:10.1016/j.geomorph.2023.108635
Nowicki, M. A., Wald, D. J., Hamburger, M. W., Hearne, M., and Thompson, E. M. (2014). Development of a globally applicable model for near real-time prediction of seismically induced landslides. Eng. Geol. 173, 54–65. doi:10.1016/j.enggeo.2014.02.002
Ozdemir, A., and Altural, T. (2013). A comparative study of frequency ratio, weights of evidence and logistic regression methods for landslide susceptibility mapping: sultan mountains, SW Turkey. J. Asian Earth Sci. 64, 180–197. doi:10.1016/j.jseaes.2012.12.014
Porras, J., Massin, F., Arroyo Solórzano, M., Arroyo, I., Linkimer, L., Böse, M., et al. (2021). Preliminary results of an earthquake early warning system in Costa Rica. Front. Earth Sci. 9, 700843. doi:10.3389/feart.2021.700843
Qiao, B. C., Li, Y., Dong, S., Yan, L., Chen, H., Ma, B. L., et al. (2009). The surface rupture of the M_s8.0 wenchuan earthquake on the northern segment of central fault. China Earthq. Eng. J. 31 (4), 333–348. doi:10.1042/BSR20080061
Quesada, R. A. (2021a). Review of the geomorphological effects of the 1991 Limón earthquake. Rev. Geol. América Cent. 65, 370–395.
Quesada, R. A. (2021b). Landslides and floods zonation using geomorphological analyses in a dynamic basin of Costa Rica. Rev. cartográfica 102, 125–138. doi:10.35424/rcarto.i102.901
Quesada, R. A., Castro-Chacón, J. P., and Boraschi, S. F. (2021c). Geomorphology, land use, and environmental impacts in a densely populated urban catchment of Costa Rica. J. S. Am. Earth Sci. 112, 103560. doi:10.1016/j.jsames.2021.103560
Quesada, R. A., Fallas López, B., Hernández, E. K., Stoffel, M., and Ballesteros-Cánovas, J. A. (2019). Relationships between earthquakes, hurricanes, and landslides in Costa Rica. Landslides 16, 1539–1550. doi:10.1007/s10346-019-01209-4
Sun, X. H., Chen, J., Bao, Y., Han, X., Zhan, J., and Peng, W. (2018). Landslide susceptibility mapping using logistic regression analysis along the jinsha river and its tributaries close to derong and deqin county, southwestern China. ISPRS Int. J. Geo-Information 7 (11), 438. doi:10.3390/ijgi7110438
Sun, X. H., Chen, J., Han, X., Bao, Y., Zhou, X., and Peng, W. (2020). Landslide susceptibility mapping along the upper Jinsha River, south-western China: a comparison of hydrological and curvature watershed methods for slope unit classification. Bull. Eng. Geol. Environ. 79, 4657–4670. doi:10.1007/s10064-020-01849-0
Tan, X. B., Yuan, R., Xu, X., Chen, guihua, and Chang, chunpai (2013). Co-seismic rupture and displacement in the XiaoYuDong area produced by the 2008 wenchuan earthquake, China, and its mechanism. Seismol. Geol. 0253-496702-0247-14. (In China).
Ullah, I., Aslam, B., Shah, S. H. I. A., Tariq, A., Qin, S., Majeed, M., et al. (2022). An integrated approach of machine learning, remote sensing, and GIS data for the landslide susceptibility mapping. Remote Sens. GIS Data Landslide Susceptibility Mapp. Land 11 (8), 1265. doi:10.3390/land11081265
Wang, F., Xu, P., Wang, C., Wang, N., and Jiang, N. (2017). Application of a GIS-based slope unit method for landslide susceptibility mapping along the longzi river, southeastern Tibetan plateau, China. ISPRS Int. J. Geo-Information. 6, 172. doi:10.3390/ijgi6060172
Xu, C., Dai, F., suning, Xu, xiwei, Xu, He, H., Wu, X., et al. (2013). Application of logistic regression model on the Wenchuan earthquake triggered landslide hazard mapping and its validation. Hydrogeology Eng. Geol. 3, 7.
Xu, C., Dai, F., Yao, X., Chen, J., Tu, X., Sun, Yu, et al. (2009). GIS-Based landslide susceptibility assessment using analytical hierarchy process in WenChuan earthquake region. Chin. J. Rock Mech. Eng. 28 (S2).
Xu, C., Xu, X., Shen, L., Dou, S., Wu, S., Tian, Y., et al. (2014b). Inventory of landslides triggered by the 2014 Ms 6.5 Ludian earthquake and its implications on several earthquake parameters. Seismol. Geol. 36 (4), 1186–1203.
Xu, C., Xu, X., Shyu, J. B. H., Zheng, W., and Min, W. (2014c). Landslides triggered by the 22 July 2013 Minxian–Zhangxian, China, Mw 5.9 earthquake: inventory compiling and spatial distribution analysis. J. Asian Earth Sci. 92, 125–142. doi:10.1016/j.jseaes.2014.06.014
Xu, C., Xu, X., Yao, X., and Dai, F. (2014a). Three (nearly) complete inventories of landslides triggered by the May 12, 2008 Wenchuan Mw 7.9 earthquake of China and their spatial distribution statistical analysis. Landslides 11, 441–461. doi:10.1007/s10346-013-0404-6
Xu, T. R., Dai, D., Yang, Z., Xi, N., Deng, W., Zhang, Y. J., et al. (2022). Preliminary study results of the 6.8 magnitude earthquake in luding, sichuan province on september 5, 2022. Earthq. Res. China 38 (3), 412–424. (In China).
Xu, X. W., Wen, X., Ye, J. Q., Ma, B. Q., Cheng, J., Zhou, R. Q., et al. (2008). Wenchuan Ms8.0 earthquake surface rupture zone and its seismic structure. Seismol. Geol. 03. (In China).
Yuan, R. M., Deng, Q. H., Cunningham, D., Xu, C., Xu, X. W., and Chang, C. P. (2013). Density distribution of landslides triggered by the 2008 wenchuan earthquake and their relationships to peak ground acceleration. Bull. Seismol. Soc. Am. 103 (4), 2344–2355. doi:10.1785/0120110233
Zhang, P. Z., Deng, Q., Zhang, G., jin, Ma, Weijun, G., Wei, M., et al. (2003). Strong seismic activity and active plate in China. Sci. China (Series D) 33, 9.
Keywords: seismic landslide, Wenchuan earthquake, Luding earthquake, susceptibility evaluation, influencing factor
Citation: Liu X, Yang X, Yuan R, Xu R and Liu C (2024) Seismic landslide susceptibility evaluation model based on historical data and its application to areas with similar environmental settings. Front. Earth Sci. 12:1419851. doi: 10.3389/feart.2024.1419851
Received: 19 April 2024; Accepted: 20 May 2024;
Published: 03 July 2024.
Edited by:
Chong Xu, Ministry of Emergency Management, ChinaReviewed by:
Langping Li, Chinese Academy of Sciences (CAS), ChinaAdolfo Quesada-Román, University of Costa Rica, Costa Rica
Copyright © 2024 Liu, Yang, Yuan, Xu and Liu. This is an open-access article distributed under the terms of the Creative Commons Attribution License (CC BY). The use, distribution or reproduction in other forums is permitted, provided the original author(s) and the copyright owner(s) are credited and that the original publication in this journal is cited, in accordance with accepted academic practice. No use, distribution or reproduction is permitted which does not comply with these terms.
*Correspondence: Xianhe Yang, yangxh36@scdzj.gov.cn; Renmao Yuan, yuanrenmao@ies.ac.cn