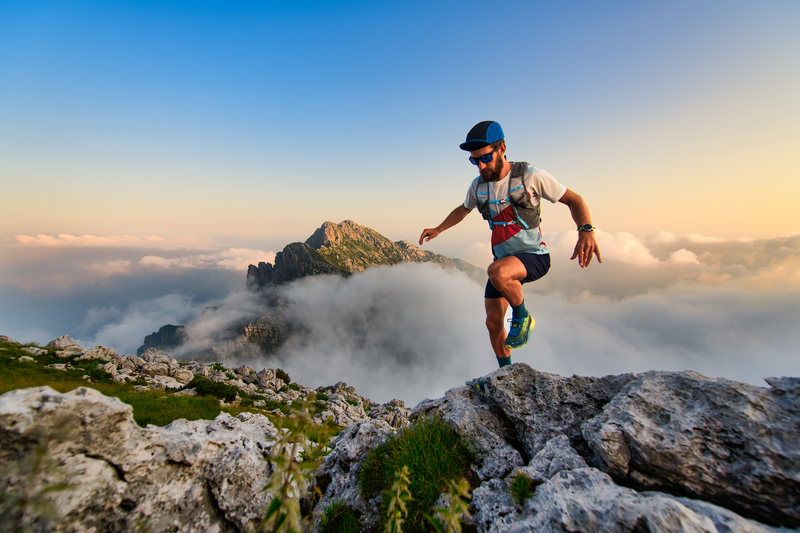
94% of researchers rate our articles as excellent or good
Learn more about the work of our research integrity team to safeguard the quality of each article we publish.
Find out more
ORIGINAL RESEARCH article
Front. Earth Sci. , 10 September 2024
Sec. Interdisciplinary Climate Studies
Volume 12 - 2024 | https://doi.org/10.3389/feart.2024.1397857
Introduction: The global rise in climatic calamities necessitates effective strategies for understanding and addressing Earth’s climate complexities. Although recent research underscores simulation tools’ effectiveness in elucidating complex concepts related to Earth’s climate, partner influence on climatic decisions within simulation studies has been overlooked.
Methods: Employing the Partner Interactive Climate Change Simulator (P-ICCS), we examined 180 participants across varied conditions. The study manipulated partner nature (optimal, irrational, or none) and climate change probability (high or low) to observe effects on participants’ responses (mitigation or adaptation) within P-ICCS.
Results: Results revealed a significant impact of partner presence, with the optimal partner notably enhancing monetary investments against climate change compared to the irrational partner. Intriguingly, climate change probability did not sway participants’ investment behavior.
Discussion: The findings emphasize the pivotal role of partner influence in climate decision-making, offering insights for effective mitigation and adaptation strategies.
The increase in greenhouse gases (GHGs) on Earth has led to a rise in average global temperature and subsequent climate disasters (Harkins, 2019; IPCC, 2021). These calamities have widespread effects on individuals, societies, and countries worldwide (Heshmati, 2021). As a result, urgent measures are required to mitigate and adapt to climate change by reducing GHG emissions (NASA, 2020). Climate mitigation aims to decrease GHG levels in the environment to slow global warming, while climate adaptation involves taking preventive measures to reduce the impact of climate disasters (NASA, 2020). Despite continuous efforts, mitigation initiatives have achieved limited success since the global increase in GHG levels (Hasson et al., 2010). Therefore, adaptative techniques, such as insurance schemes to mitigate climate damages, provide an alternative approach to counter the adverse effects caused due to changing climatic conditions (Hasson et al., 2010). Including adaptation and mitigation strategies in climate decision-making is crucial (Bhamidipati et al., 2014).
Previous research suggests that the failure to limit GHG increases may be attributed to individuals’ “wait-and-see” biases towards climate change (Dutt and Gonzalez, 2012a; 2012b). This bias implies that individuals believe climate mitigation and adaptation measures can be postponed until clear indications of a global climate crisis emerge (Botzen et al., 2021). Studies indicate that simulation tools, such as climate microworlds, can help alleviate people’s wait-and-see tendencies and promote pro-environmental behavior. For example, the Interactive Landslide Simulator (ILS) proposed by Chaturvedi et al. (2018) effectively increased people’s mitigation efforts against landslides through induced feedback. Similarly, the Interactive Climate Change Simulator (ICCS) developed by Choudhary and Dutt (2021) utilized feedback and varied probability models to raise awareness of climate change and encourage pro-environmental behavior by investing more resources in mitigation and adaptation efforts.
Prior literature has argued on empirical grounds that prevailing social norms can effectively impact a person’s climate change behavior (Griskevicius et al., 2008). Additionally, probability models of climate change can shape people’s sensitivity toward’s climatic disasters and their pro-environmental actions (Choudhary and Dutt, 2021; Dutt and Gonzalez, 2012a). For instance, owing to the contemporary social norms around eco-consciousness and consumerism, research suggests that individuals with communitarian-egalitarian values tend to perceive greater climate risks and support mitigation efforts compared to those with individualist-hierarchical values (Rooney-Varga et al., 2021). Environmental values and identities at personal and group levels motivate climate action (Bouman et al., 2021). Additionally, consumer activism beliefs and global warming concerns predict green purchasing goals and behaviors (Roser-Renouf et al., 2016). Therefore, neuroscientific evidence suggests that awakening ecological consciousness can lead to eco-friendly consumption (Yin et al., 2022).
Social psychology theories suggest that prevalent norms within a social group/setting can significantly influence individuals’ behavior, leading them to comply with these norms and imitate the behavior of others (Bernheim, 1994). Social norms can also influence pro-environmental actions concerning climate change (Griskevicius et al., 2008). For instance, a study on towel reuse in hotels found that a card emphasizing guests’ pro-environmental behavior was most effective in increasing towel reuse rates (Goldstein et al., 2008). However, social norms can also have negative effects on decision-making. For example, signs warning against vandalism of petrified wood in a national park inadvertently increased theft among individuals with no prior history of vandalism (Cialdini et al., 2006). The influence of social norms on individuals’ monetary behavior regarding uncertain problems like climate change has received less attention but studying them can provide insights into how these norms affect people’s awareness of climate investment, particularly when the outcomes are probabilistic.
The likelihood of experiencing climatic calamity and the prevailing social norms can significantly affect individuals’ decision-making towards climate change (Choudhary and Dutt, 2021; Vanderheiden, 2016). Uncertainties associated with future climate change consequences can affect individuals’ perceptions and monetary behavior towards climate change (Nordhaus, 1994). Research indicates that higher-probability events evoke more concern than lower probability events (Weber, 2006). Therefore, a high probability of a climatic catastrophe may lead to more pro-environmental actions than a low-probability event. Furthermore, individuals tend to overestimate low-probability outcomes and underestimate high-probability outcomes when presented in text form (Hertwig, 2012). However, when individuals experience the consequences, the weighting of different probabilities becomes reversed, with high-probability experiences being given more importance. Experiencing a climatic catastrophe with a high likelihood can help individuals overcome their wait-and-see preferences (Dutt and Gonzalez, 2012a), and this overcoming may be even more pronounced in the presence of reinforcing social norms (Vanderheiden, 2016). The combined influence of prevalent social norms and uncertainties in climate change consequences on individuals’ decisions against climate change has been insufficiently studied.
This study aims to address the gaps in the literature regarding the influence of prevalent social norms and climate change probabilities on individuals’ decisions against climate change. To achieve this, we made an interactive climate microworld named the “Partner Interactive Climate Change Simulator” (P-ICCS). The P-ICCS involves an unreal partner who plays alongside the participant, making mitigative and adaptative choices to counter climate change and experiencing the consequences of those choices. The model behind the P-ICCS introduces two types of partners: an optimal partner, who invests optimally toward climate change, and an irrational partner, who invests non-optimally. Additionally, the P-ICCS tool varies the likelihood of facing climate change consequences, allowing participants to experience different probabilities within the simulation.
The following experiment investigates the effects of prevalent social norms and climate change probabilities on individuals’ decisions against climate change. The proposed P-ICCS simulation tool is a novel approach that incorporates these factors and can be cost-effectively used in climate change education and policymaking to enhance people’s awareness of climate change. The following sections summarize the relevant literature, describe the design and operation of the P-ICCS tool, and present the results of our experiment.
Literature indicates that an individual’s behavior against climate change could be affected by the prevalent social norms (Vesely et al., 2020; Nolan et al., 2008). For example, Nolan et al. (2008) placed four different messages on the doorknobs of a community. The first three messages urged people to conserve energy on the grounds of helping the environment, benefiting society, and saving money; whereas, the fourth message used normative appeal to promote energy conservation. The results indicated that the normative appeal message was most effective in promoting pro-environmental actions within the community.
Additionally, the nature of social norms can also affect an individual’s environmental actions (Cialdini et al., 2006; Vesely et al., 2020). For example, in Arizona’s Petrified National Park, visitors seeing the sign that other visitors are involved in the act of vandalism attempted to steal some wood for themselves (Cialdini et al., 2006). Conversely, in their social dilemma setting for climate change, (Vesely et al., 2020) found that the presence of a pro-environmental partner in a group motivated other group members to invest in group goals than personal goals. Overall, one can expect that different rational (optimal) and irrational social norms might uniquely affect an individual’s pro-environmental behaviors. Therefore, we hypothesize that.
H1. The presence of a partner will affect mitigation and adaptation decisions against climate change, and a partner that invests optimally against climate change will elicit greater human pro-environmental behaviors than a partner that invests irrationally.
Next, studies indicate that people pay more attention to climate change when the likelihood of its occurrence is elevated (Weber, 2006). Public goods game experiments indicate that a greater likelihood of climatic calamity increases people’s cooperation towards climatic goals (Pfeiffer and Nowak, 2006). Furthermore, the prevalent social norm to invest towards climate change, induced due to the partner investing pro-environmentally, may further increase human pro-environmental behaviors (Bernheim, 1994). Inversely, based on the literature on the free-riding tendencies of people towards climate change (Vanderheiden, 2016), one might expect people to invest less against climate change when playing with a pro-environment partner, especially in low-probability conditions. Therefore, we hypothesize that.
H2. The proportion of investments against climate change would be affected by both the probability of climate consequences and the prevalent social norms.
The P-ICCS model of climate microworld was adapted from the ICCS model of Choudhary and Dutt (2021). In the P-ICCS model, participants could invest in mitigation and adaptation. The P-ICCS simulated the catastrophic effects of climate change on the basis of investments made against climate change. In the P-ICCS model, the futrure climate change probablity was the same for both the unreal partners and the human players. These players’ mitigation investments determined this climate change probability.
While investment towards mitigation reduced the likelihood of climate change events, adaptation attenuated the losses simulated due to climatic catastrophes. In the P-ICCS model, the climate change catastrophes were simulated in the form of cyclones, floods, or droughts. The three natural calamities further simulated the monetary losses through injury, fatality, and property damages. Cyclones and floods simulated damages in all three forms, while droughts only caused damages in the form of injury and fatality. Three corresponding insurance plans (adaptation schemes) existed: health insurance, life insurance, and property insurance to attenuate the losses simulated due to climatic calamities. The player and their partner had to buy insurance separately to attenuate losses. Only the individual (human player or unreal partner) who had purchased insurance scheme(s) in any given trial availed the advantages of the insurance scheme. Thus, while the participant’s and their unreal partner’s investment in mitigation determined the future climate change probability, the attenuated loss due to adaptation was only applicable when the player or their unreal partner had bought the insurance scheme.
As mentioned previously, in the P-ICCS model, an unreal partner’s presence was used to study the effects of prevalent social norms on an individual’s monetary behavior against climate change. Like the real world, where collective efforts are required to reduce the GHG concentration in the environment (CLC, 2021), in the P-ICCS model, the future climate change probability was determined based on the participant and their unreal partner’s investment towards climate mitigation. However, the advantages of enrolling in an insurance scheme were not explicitly shared amongst the participants and their unreal partners. In P-ICCS, the participants learned from their experience, while the unreal partner’s behavior was pre-determined, and the partner either invested optimally or irrationally against climate change. While the optimal partner invested most efficiently for increasing its overall assets, the irrational partner invested least efficiently. The exact investment behavior of both partners is detailed ahead in the manuscript.
As mentioned, the climate change probability depended on the investment made in climate mitigation. The likelihood of climate change occurrence was high if people invested less and low if they invested more in climate mitigation. The following function was used to determine the climate change probability in the P-ICCS model:
Where
In the P-ICCS model, there was a possibility of attenuating the losses incurred due to climatic catastrophes by enrolling in a corresponding insurance scheme. Property insurance attenuated the losses incurred due to property damages, while health and life insurance attenuated the losses incurred due to injury and fatality, respectively. In the P-ICCS model, the advantages of investing in adaption were not shared between the participant and their partner, and only the person who purchased insurance experienced an attenuated loss. Insurance once purchased was only applicable for that particular trial and had to be repurchased for the subsequent trial. Additionally, the P-ICCS model incorporated a smart insurance scheme similar to the ICCS model proposed Choudhary and Dutt (2021), within which the loss incurred was derived on the basis of how many times an insurance scheme was bought. The more times insurance was purchased, the greater the loss. The following function was used to determine the attenuated percentage of loss in both models:
Where
The P-ICCS is a climate microworld designed to investigate players’ investment behaviors towards climate change. PHP and MySQL, open-source programming languages, were used to program the tool. The P-ICCS tool enabled participants to make monetary investments towards climate change and then provided them with the outcomes of their decisions. Furthermore, in the P-ICCS tool, an unreal partner played alongside the participant, and the P-ICCS tool’s interface enabled participants to see the monetary decisions of their partner and the corresponding outcomes. The P-ICCS tool ran for 36 trials, where every trial corresponded to a year. Participants were provided with a fixed initial annual income and property wealth. Participants could make mitigation and adaptation decisions using their annual income. Additionally, losses were suffered to property wealth and annual income through damages simulated by climatic catastrophes. Adaptive insurance schemes attenuated the losses suffered from climatic catastrophes. The individuals who participated in the P-ICCS tools were tasked with maximizing their remaining total wealth, which was the sum of their remaining property assets and uninvested income.
The P-ICCS tool’s interface comprised mainly of the investment and the feedback screen. Figure 1. Depicts the participants’ monetary investment screens to make monetary decisions against changing climatic conditions. In the P-ICCS tool, the partner’s investment behavior was pre-decided, and depending upon the partner’s nature (optimal or irrational), the investment decisions for the partner were executed in the P-ICCS tool. The interface that participants utilize to invest money in climate adaptation or mitigation is shown in Panel a1. Participants could simultaneously invest in climate mitigation and adaptation as long as they had the necessary capital. Panel a2 presents the different game parameters and their corresponding values. In the P-ICCS tool, the participants (blue color) were also shown their partner’s (black color) game parameters in a color-coded manner. The P-ICCS tool presented parameters such as the probability of climate change, remnant annual income, property assets, and total income.
Figure 1. (A) The investment screen of the P-ICCS tool. (A1) Participants choose how much money to put toward addressing climate change. (A2) The parameters within P-ICCS for the participant (blue color) and their partner (black color). (A3) The line graphs used in P-ICCS tool to depict crucial information in a color coded manner for the participant and their partner.
Moreover, in the P-ICCS tool, parameters common for both the player and the participant, like the anthropogenic probability of climate change, were presented first, and uncommon parameters were presented later in a color-coded manner. The participants could infer the investment strategies employed by their partner (optimal or irrational) by studying their investment made available on the investment screen. Lastly, in the P-ICCS tool the total probability of climate change, total income not invested in climate change, and property wealth were graphically displayed as shown in panel a3. Again, in the P-ICCS tool (panel a3), unreal partners’ game parameters were available in a color-coded manner. The game parameters and graphical depictions provided were to help participants understand the effect of their and their partner’s monetary behavior on the future climate change probability and to make educated and informed monetary decisions against climate change. Once the participants had made their investment decision, they clicked the “Invest” button (panel a1).
The feedback page was presented to the participants upon clicking the “Invest” button. (see Figure 2). As shown in Figure 2, the feedback screen presented participants with outcomes of the monetary decisions made against climate change. The feedback screen in the P-ICCS tool was of two types: negative feedback (Figure 2A) and positive feedback (Figure 2B). The negative feedback screen was simulated in case of a climatic catastrophe. In the P-ICCS tool, the feedback screen was split into two segments. The left segment presented information about the outcomes faced by the participants, and the right segment presented information about the outcomes faced by their partner. The left and right sides of the feedback screen presented information in a similar pattern. The screen first depicted which climatic calamity had occurred (for example, a flood occurred). Next, it presented the outcomes corresponding to the investments made and the damages inflicted due to the occurrence of a climatic catastrophe. Finally, it showed images corresponding to the damage incurred due to a climatic calamity. The positive feedback screen (Figure 2B) was generated when no climatic catastrophe occurred in a particular trial. It informed participants about the investments made towards climate change and their remaining income. A mix of positive and negative feedback screens was possible in the P-ICCS tool, i.e., climate calamity occurred for you but not your partner, and vice versa. Lastly, to get back to the investment screen for the next round, the “Return to Game” button was pressed.
Figure 2. Split feedback displays of the P-ICCS tool. (A) Participants are informed of the losses arising from climate catastrophes through the negative feedback screen. (B) Participants are informed that there was no climate disaster by the positive feedback screen.
Experiment. Influence of prevalent social norms and climate change probability.
The experiment tests the effectiveness of prevalent social norms and climate change probability on people’s mitigation and adaptation investments in P-ICCS.
In the experiment, we used the P-ICCS tool to study the effects of prevalent social norms (via an unreal optimal or irrational partner, or no partner) and climate change probability (high (cubic) probability or low (linear) probability) on participants (N = 180) monetary behavior against climate change. The unreal partner conditions (optimal or irrational partner) were the experimental groups of the study and the condition with no partner acted as the control group in the study. The P-ICCS tool allowed participants to invest against climate change while playing alongside a partner that also made monetary investments towards climate mitigation and adaptation. The P-ICCS tool simulated climate change based on the investment made towards climate change by the participants and their partner. The two probability models, i.e., linear and cubic involved Equation 1 with h = 1 and h = 3, respectively. In contrast to the cubic probability, which produced an abrupt (non-linear) change in probability, the linear probability caused a gradual (linear) shift in probability of climate change. In the optimal condition, the unreal partner invested in the most optimal way against climate change, while in the irrational condition, the unreal partner invested in the least optimal way against climate change (more details are presented below). In the no-partner condition, no partner was present in P-ICCS and there was only 1 single human participant playing in P-ICCS. The six between-subject conditions based on the probability of climate change and the nature of the partner were: linear optimal (N = 30), linear irrational (N = 30), linear no-partner (N = 30), cubic optimal (N = 30), cubic irrational (N = 30), and cubic no-partner (N = 30).
The parameters for the P-ICCS tool were motivated from similar climate microworlds (Choudhary and Dutt, 2021). In the P-ICCS tool each between-subject condition was 36 years long. Participants used their income in each trial to invest and counter climate change. Participants starting income was set at 8760 EC/year (EC being an imaginary currency), and their initial property wealth was 50, 000, 00 EC. Participants could decide how much of their annual income to invest in climate mitigation (from 0 EC to present income available); however, each adaptive insurance scheme had a fixed cost. Property insurance cost 1884 EC/year, health insurance costed 1173 EC/year, and life insurance cost 488 EC/year. These amounts were inspired by how the insurance premiums are charged in the real world. The objective of all participants in all conditions was to maximize their remaining total wealth by investing in climate change.
In P-ICCS, climatic catastrophe was simulated in the form of three climatic calamities, i.e., cyclones, droughts, and floods, and they occurred with equal 33%, 33%, and 34% chance, respectively, in any given trial. In the case of a climatic calamity, the likelihood of incurring losses due to injury, fatality, and property damages was set at 30%, 9%, and 50%, respectively. As mentioned previously drought did not simulate property damage, and thus, it simulated only cataclysmic effects of climate change in the form of injury (30%) and fatality (9%).
Additionally, the P-ICCS tool introduced an unreal partner (optimal or irrational) to invest against climate change along with the participant. The investment course for the optimum and irrational partners was computed through Monte-Carlo simulations. In the optimal conditions of P-ICCS, the partner invested in the most optimum manner with respect to maximizing its total wealth. The optimal partner always purchased property insurance with a 100% chance and invested the rest of its remaining capital into mitigating climate change. If the partner did not have the capital to buy the property insurance, it invested its remaining capital into mitigation.
In contrast, the irrational partner invested least optimally, i.e., it functioned in a manner that minimized its total wealth. The irrational partner only made an investment in any given trial with a 10% chance, and when it did make an investment, it was always into buying property insurance only. The irrational partner never invested in mitigation or purchased health or life insurance. The computational analysis for determining the monetary behaviors for the unreal optimal and irrational partners, respectively, are made available in the Supplementary File. Finally, no unreal partner was present to play alongside the participants in the no-partner condition.
Furthermore, participants were subjected to two climate change probability models based on Equation 1; the cubic model (Equation 1 with k = 3) and the linear model (Equation 1 with k = 1). Participants subjected to the cubic model faced a higher climate change probability than participants subjected to the linear model, even if the proportion of investment in climate mitigation was similar in both probability models. In the case of a climatic calamity, property damages reduced property wealth by 50%. Likewise, injury and fatality reduced the latest annual income by 12.5% and 25%. However, if an investment was made in corresponding insurance schemes, the participants suffered an attenuated percentage of loss, determined using Equation 2. To prevent (r) from never being more than the actual loss incurred when no insurance was bought, an upper limit of 10%, 20%, and 50% for injury (health insurance), fatality (life insurance), and property damages (property insurance), respectively, were placed on (r).
Participants’ monetary investments in three insurance schemes and climate mitigation were converted into six investment ratios for statistical analysis. The six investment ratios were namely total investment, mitigation, total insurance, property insurance, health insurance, and life insurance ratios. The investment ratios were obtained by computing the money spent towards a certain action till a given (
A signed written consent form was taken from the participants before the experiment. Amazon Mechanical-Turk, a crowdsourcing site, randomly recruited subjects from India (Mason and Suri, 2012). Men made up 72.22% of the participants, while women made up 27.78%. Participants age was between 18 and 63 years (Mage = 32.50, S.D. = 6.82). 64.44% of participants had completed their undergraduate degree, 1.67% had completed their High-school education, and 33.89% had a postgraduate degree. 41.67% of the participants were from non-STEM backgrounds, while 58.33% were from a STEM background. Each participant received an INR 30 (USD 0.4) participation stipend. A fortunate draw contest was held for the top three performers across each circumstance. A random participant was chosen to receive a gift voucher worth INR 500 (USD 6.73).
Specific instructions were given to the participants before the start of their experiment. The participant’s aim was to maximize their remaining total wealth by making investments in climate mitigation and adaptation. Participants were required to play all 36 trials and were recognized and compensated for their time and effort once the experiment was completed. Participants were not told that they were playing against an unreal partner. They were told that there may be a partner who is playing with them located somewhere else in the world.
The conditions for using analysis of variance (ANOVA) for the six dependent variables were met, as the variables were found to be normally distributed (Q-Q plots) and homogenous (Levene’s test) (Field, 2013). Since the sample sizes were equal across all conditions, a Tuckey post hoc test was employed. The dependent variable’s significance was tested through Greenhouse-Geisser’s corrected degrees of freedom, because Significant result of Mauchley’s test of sphericity indicated that no dependent variable met with the condition of sphericity. Therefore, we performed 3 (partner conditions) × 2 (probability conditions) × 36 (trials) mixed-factorial ANOVA, with an alpha level of 0.05, to study the effects of partner presence and probability on the six dependent variables, i.e., the investment ratios.
Table 1 shows the results for the impact of the prevalent social norms, probability of climate change taking place, and the interaction between the two on each of the six dependent variables. As shown in Figure 3, the total investment (Figure 3A), mitigation (Figure 3B), and health insurance ratio(s) (Figure 3C) were significantly higher in the presence of a partner than in the no-partner condition. These findings support our expectations. In contrast to our expectation, the partner’s presence did not significantly influence the total insurance, property insurance, and life insurance ratios. Furthermore, none of the six dependent variables showed any discernible effects from the model’s climate change probability. As shown in Figure 4, previous research conducted by Choudhary and Dutt (2021), where they used the ICCS tool (no partner was deployed to play alongside the participant), the cubic model showcased a significantly higher likelihood of facing climatic calamity than the linear model.
Table 1. Results for the effect of the prevalent social norms and climate change probability of the six dependent variables.
Figure 3. Results of influence of prevalent social norms and probability models of climate change. (A) Total investment ratio in optimal partner, irrational partner, and no-partner conditions. (B) Mitigation ratio in optimal partner, irrational partner, and no-partner conditions. (C) Health insurance ratio in optimal partner, irrational partner, and no-partner conditions. The text in top-right corner show outcomes of the Tuckey post hoc test. The error bars show 95% CI around the point estimate.
Figure 4. The average probability of climate change was obtained by simulating the two probability models one thousand times. The error bars show 95% CI around the point estimate. Figure adapted from Choudhary and Dutt (2021).
Furthermore, there was no significant connection between the presence of a partner and the likelihood of climate change for five out of the six dependent variables. But as Figure 5 shows, there was a substantial interaction for the property insurance ratio between the influence of the partner and the probability of climate change.
Figure 5. The average property insurance ratio under each of the optimal, irrational, and no-partner conditions of the partner effect, as well as the cubic and linear probability of climatic change. The error bars show 95% CI around the point estimate.
Table 2 shows the results for the six dependent variables over 36 trials. As shown in Figure 6, the investment in all six dependent variables increased significantly over 36 trials. No significant interaction existed between prevalent social norms and trials for any of the six dependent variables. Moreover, for any of the six dependent variables, there was no significant interaction found between trials and the probability of climatic change. For any of the six dependent variables, there was also no significant three-way interaction between trials, the probability of climate change, and prevailing societal norms.
Table 2. Results for the effect of the prevalent social norms and climate change probability of the six dependent variables.
Figure 6. Results of investment over 36 trials. (A) Total investment ratio over trials. (B) Mitigation ratio over trial. (C) Total insurance ratio over trials. (D) Property insurance ratio over trials. (E) Health insurance ratio over trials. (F) Life insurance ratio over trials. The error bars show 95% CI around the point estimate.
The main objective of this paper was to develop simulation tools based on prevalent social norms and probability of climate change that can be used to increase people’s cognizance of climate change and promote pro-environmental actions. We used the P-ICCS tool to study the effects of the prevalent social norms, climate change probability, and the interaction between the two on people’s monetary behaviors against climate change. Per our hypothesis, a partner’s presence significantly affected the participant’s investment in total investment, mitigation, and health insurance ratios. We speculate that prevalent social norms induced through partners significantly affect participants’ environmental decisions in P-ICCS (Griskevicius et al., 2008). Furthermore, the investment in total investment ratio and mitigation ratio were significantly higher when the participants played alongside an optimal partner than an irrational partner, further emphasizing the importance of positive influence in increasing pro-environmental actions (Goldstein et al., 2008). The optimal partner may have motivated participants to invest against climate change and reduce climatic damage. The optimal partner irrespective of the players investment behavior always invested towards climate mitigation and property insurance. The human player might have replicated the same investment strategy due to the prevalent social norms and this would have resulted in a significant increase in mitigation and overall investment against climate change. The reduced climatic damages due to lower probability of climate change may have further reinforced the action of investing against climate change.
The participants’ desire to create a positive public image may have also contributed to increasing pro-environmental actions (Fenigstein et al., 1975). Participants in the study were unaware that they were playing alongside an unreal partner and may have thought that, just as they can see their partner’s monetary decisions, their partner may be able to see their decisions, too. Thus, to create a positive public image, they may have invested significantly higher in the presence of a partner than in its absence (Kumar and Dutt, 2019).
In contradiction to our hypothesis, results indicated that three out of six investment ratios (i.e., ratios for total insurance, property insurance, and life insurance) were not significantly affected by the presence of a partner. One likely reason for this finding could be that the partner’s presence or absence influenced people’s mitigation decisions, but not their adaptation decisions. This reasoning is supported by the fact that the optimal partners invested in mitigation and may have motivated the participants to invest in mitigation too. For irrational and no partner conditions, the high climate change probability could have caused participants to deplete their monetary assets, making them unable to buy insurances.
The climate change probability did not significantly influence investments in P-ICCS. We speculate that within the P-ICCS tool parameters, the two probability models may have resulted in not very different climate change probabilities, which were driven by participants’ and partners’ mitigation actions. For example, if in the cubic model (high) of climate change a participant was playing with an optimal partner, then to reduce the likelihood of climate change, the participant and the optimal partner may have invested more in mitigation. Thus reducing the future probability of climate change. Inversely, in the linear model (low) of climate change the partner playing along with an optimal partner may have invested less in climate mitigation and would have decided to safeguard their assets as the probability of climate change was already low.
Finally, the interaction between partner presence and the probability of climate change significantly affected the average property insurance ratio. The participants invested less in property insurance when playing alongside a partner in the cubic probability model than in the linear probability model. Inversely, in the no-partner condition, the investment towards property insurance was higher in the cubic model than in the linear probability model. We speculate that the participants, in the face of a higher probability of climate change and in the absence of a partner to reduce the future likelihood of climate change, participants may have prioritized saving their assets and primarily property assets (which contributes most towards the total wealth remaining) while mitigating climate change periodically, as it was an intelligent way to attenuate the losses due to climatic calamities. It is also supported by the observation that when playing alongside an optimal partner, participants prioritized mitigation to reduce the overall probability of climate change to face no cataclysmic effects.
In contradiction to our hypothesis, the interaction between the probability and social norms was insignificant for five out of six investment ratios. Thus, the investment behaviors of participants against optimal, irrational, and no partners were similar for the linear and cubic probability models. The likely reason for this finding could be because both linear and cubic probability models produced similar probability values, which were driven by the participants’ and their partners’ mitigation actions.
One limitation of the present work is that the P-ICCS tool compresses space and time. Thus, the effects simulated over the years in the tool were experienced by participants over a few minutes. Overall, the implication of these results may not be identical to that of the real world. Second, our immediate goal was to study the effects of the prevalent social norms and climate change probability on people’s climatic decisions. For this purpose, the P-ICCS tool used approximate values of climate change losses and consequences. Overall, the actual real-life values may differ from those used in the simulation tool.
Furthermore, it is noted that graphs were used in the P-ICCS tool on the investment screen to help participants better understand the relationship between climate mitigation and the future probability of climate change. Such dynamic graphs depicting the exact probability of climate change are not readily available in real life. Moreover, participants were not presented with all the knowable parameters, like the cost of climatic damage if insurance is bought. Though, complementary information like the total income not invested in climate change (which had to be maximized) was provided. We acknowledge that specific tool parameters did not wholly adhere to real-world settings. In future, we plan to develop different versions of P-ICCS tool with closer to real-life information to study the effect of information availability on participants’ monetary behaviors.
Despite the limitation mentioned above, the P-ICCS tool is excellent for studying the impact of various policy and environmental variables on the likelihood of climate change occurrence. Policymakers can incorporate different partner types within the P-ICCS tool to study the influence of different partner investment strategies on people’s monetary behavior towards climate change. Moreover, the flexible nature of the P-ICCS tool allows policymakers to incorporate additional mitigation and adaptation strategies within the P-ICCS tool following their need and the demography they are studying and enabling them to understand the preferences of people when making monetary investments against climate change. Similarly, environmental variables such as the likelihood of facing climate change (probability), types of climatic calamities, and damages inflicted by climatic calamities, among other environmental variables, can be altered within the P-ICCS tool. Enabling the P-ICCS tool to study the effects of such environmental variables on the monetary behavior of the participants. The mentioned variables can be altered depending on the demographic being studied and the subjective needs of the researcher.
Thus, the P-ICCS tool shows excellent potential for real-world climate change education, communication, policymaking, and learning climate investment behavior. Utilizing the P-ICCS tool to introduce empirical evidence indicating the positive effect of collective efforts against climate change, climate communicators and policymakers can create engaging, informative and contextualized narratives to promote pro-environmental actions on community levels (Corner et al., 2018). Climate educationists can similarly leverage the P-ICCS tool and prevalent societal norms to raise awareness about the need to collectively counter the problem of climate change.
Prior research indicates that people continue to exhibit wait-and-see preferences against climate change (Sterman, 2008; Botzen et al., 2021). However, our results indicate that simulation tools like P-ICCS can be a cost-effective medium to promote pro-environmental actions. Furthermore, the P-ICCS tool can have pedagogical applications to help increase people’s cognizance towards climate change and educate them about the adverse effects of climatic catastrophes. Our future research plans to incorporate stochastic climate events (e.g., wildfires) within our simulation tools. Additionally, we plan to use virtual reality (VR) techniques to deliver more immersive and realistic gameplay and test its effectiveness in promoting pro-environmental actions (Markowitz et al., 2018).
Moreover, we plan to study the effects of other system variables like the severity of damages, information availability, and different forms of feedback (like verbal and visual) on participants’ environmental decisions. Finally, the current results generated from the Indian demography can help determine the climate actions in other emerging economies but for better generalizability of results we plan to cover a larger cross-country sample.
This study aimed to develop simulation tools based on prevalent social norms and climate change probabilities to enhance awareness and promote pro-environmental actions. Utilizing the P-ICCS tool, we found that the presence of an optimal partner significantly increased participants’ investments in mitigation and overall climate-related actions, underscoring the strong impact of positive social norms. Conversely, irrational partners did not significantly alter investment behaviors, highlighting the critical role of constructive influence. Despite aforementioned limitations, the P-ICCS tool demonstrates considerable potential for climate change education, policymaking, and the study of investment behaviors in response to climate-related risks, providing a foundation for future research and practical applications in climate change mitigation and adaptation strategies.
The raw data supporting the conclusions of this article will be made available by the authors, without undue reservation.
The studies involving humans were approved by the Ethics Committee at the Indian Institute of Technology Mandi, India. The studies were conducted in accordance with the local legislation and institutional requirements. The participants provided their written informed consent to participate in this study.
GC: Data curation, Formal Analysis, Investigation, Methodology, Validation, Writing–original draft, Writing–review and editing. DS: Methodology, Investigation, Writing–original draft. VD: Methodology, Supervision, Validation, Writing–review and editing.
The author(s) declare that financial support was received for the research, authorship, and/or publication of this article. The study was funded and supported by the Indian Institute of Technology Mandi, Himachal Pradesh, India.
The authors declare that the research was conducted in the absence of any commercial or financial relationships that could be construed as a potential conflict of interest.
The author(s) declared that they were an editorial board member of Frontiers, at the time of submission. This had no impact on the peer review process and the final decision.
All claims expressed in this article are solely those of the authors and do not necessarily represent those of their affiliated organizations, or those of the publisher, the editors and the reviewers. Any product that may be evaluated in this article, or claim that may be made by its manufacturer, is not guaranteed or endorsed by the publisher.
The Supplementary Material for this article can be found online at: https://www.frontiersin.org/articles/10.3389/feart.2024.1397857/full#supplementary-material
Bernheim, B. D. (1994). A theory of conformity. J. political Econ. 102 (5), 841–877. doi:10.1086/261957
Bhamidipati, S., van der Lei, T., and Herder, P. (2014). “From mitigation to adaptation in asset management for climate change: a discussion,” in Proceedings of the 7th world congress on engineering asset management (WCEAM 2012) (Cham: Springer International Publishing), 103–115.
Botzen, W., Duijndam, S., and van Beukering, P. (2021). Lessons for climate policy from behavioral biases towards COVID-19 and climate change risks. World Dev. 137, 105214. doi:10.1016/j.worlddev.2020.105214
Bouman, T., Van der Werff, E., Perlaviciute, G., and Steg, L. (2021). Environmental values and identities at the personal and group level. Curr. Opin. Behav. Sci. 42, 47–53. doi:10.1016/j.cobeha.2021.02.022
Chaturvedi, P., Arora, A., and Dutt, V. (2018). Learning in an interactive simulation tool against landslide risks: the role of strength and availability of experiential feedback. Nat. Hazards Earth Syst. Sci. 18 (6), 1599–1616. doi:10.5194/nhess-18-1599-2018
Choudhary, G., and Dutt, V. (2021). Experience in a climate simulator: influence of probability function and feedback on decisions against climate change. Frontiers in Psychology 12, 674892. doi:10.3389/fpsyg.2021.674892
Cialdini, R. B., Demaine, L. J., Sagarin, B. J., Barrett, D. W., Rhoads, K., and Winter, P. L. (2006). Managing social norms for persuasive impact. Soc. Influ. 1 (1), 3–15. doi:10.1080/15534510500181459
CLC (2021). Why climate progress is deadlocked. Available at: https://clcouncil.org/why-climate-progress-is-deadlocked/.
Corner, A., Shaw, C., and Clarke, J. (2018). Principles for effective communication and public engagement on climate change: a handbook for IPCC authors. Clim. Outreach.
Dutt, V., and Gonzalez, C. (2012a). Decisions from experience reduce misconceptions about climate change. J. Environ. Psychol. 32 (1), 19–29. doi:10.1016/j.jenvp.2011.10.003
Dutt, V., and Gonzalez, C. (2012b). Human control of climate change. Clim. Change 111 (3-4), 497–518. doi:10.1007/s10584-011-0202-x
Fenigstein, A., Scheier, M. F., and Buss, A. H. (1975). Public and private self-consciousness: assessment and theory. J. Consult. Clin. Psychol. 43 (4), 522–527. doi:10.1037/h0076760
Goldstein, N. J., Cialdini, R. B., and Griskevicius, V. (2008). A room with a viewpoint: using social norms to motivate environmental conservation in hotels. J. Consumer Res. 35, 472–482. doi:10.1086/586910
Griskevicius, V., Cialdini, R. B., and Goldstein, N. J. (2008). Social norms: an underestimated and underemployed lever for managing climate change. In In.
Hasson, R., Löfgren, Å., and Visser, M. (2010). Climate change in a public goods game: investment decision in mitigation versus adaptation. Ecol. Econ. 70 (2), 331–338. doi:10.1016/j.ecolecon.2010.09.004
Hertwig, R. (2012). The psychology and rationality of decisions from experience. Synthese 187 (1), 269–292. doi:10.1007/s11229-011-0024-4
Heshmati, E. M. (2021). Impact of climate change on life. Environmental issues and sustainable development, 1–20.
IPCC (2021). Summary for policymakers. Climate change 2021: the physical science basis. UK: Cambridge University Press.
Kumar, M., and Dutt, V. (2019). Collective Risk Social Dilemma: role of information availability in achieving cooperation against climate change. J. Dyn. Decis. Mak., 2–1. doi:10.11588/jddm.2019.1.57360
Markowitz, D. M., Laha, R., Perone, B. P., Pea, R. D., and Bailenson, J. N. (2018). Immersive virtual reality field trips facilitate learning about climate change. Front. Psychol. 9, 2364. doi:10.3389/fpsyg.2018.02364
Mason, W., and Suri, S. (2012). Conducting behavioral research on Amazon's Mechanical Turk. Behav. Res. Methods 44, 1–23. doi:10.3758/s13428-011-0124-6
NASA. (2020). Climate change adaptation and mitigation. Available at: https://climate.nasa.gov/solutions/adaptation-mitigation/ (Accessed February 24, 2021,
Nolan, J. M., Schultz, P. W., Cialdini, R. B., Goldstein, N. J., and Griskevicius, V. (2008). Normative social influence is underdetected. Personality Soc. Psychol. Bull. 34 (7), 913–923. doi:10.1177/0146167208316691
Pfeiffer, T., and Nowak, M. A. (2006). All in the game. Nature 441 (7093), 583–584. doi:10.1038/441583a
Rooney-Varga, J. N., Hensel, M., McCarthy, C., McNeal, K., Norfles, N., Rath, K., et al. (2021). Building consensus for ambitious climate action through the world climate simulation. Earth's Future 9 (12), e2021EF002283. doi:10.1029/2021ef002283
Roser-Renouf, C., Atkinson, L., Maibach, E., and Leiserowitz, A. (2016). Climate and Sustainability| the consumer as climate activist. Int. J. Commun. 10, 24.
Sterman, J. D. (2008). Risk communication on climate: mental models and mass balance. Science 322 (5901), 532–533. doi:10.1126/science.1162574
Vanderheiden, S. (2016). Climate change and free riding. J. Moral Philosophy 13 (1), 1–27. doi:10.1163/17455243-4681046
Vesely, S., Klöckner, C. A., and Brick, C. (2020). Pro-environmental behavior as a signal of cooperativeness: evidence from a social dilemma experiment. J. Environ. Psychol. 67, 101362. doi:10.1016/j.jenvp.2019.101362
Weber, E. U. (2006). Experience-based and description-based perceptions of long-term risk: why global warming does not scare us (yet). Clim. change 77 (1), 103–120. doi:10.1007/s10584-006-9060-3
Keywords: climate simulation tool, partner influence, social norms, climate change, climate mitigation and adaptation
Citation: Choudhary G, Sihag D and Dutt V (2024) Climate decision making: influence of prevailing social norms and probability of climate change. Front. Earth Sci. 12:1397857. doi: 10.3389/feart.2024.1397857
Received: 08 March 2024; Accepted: 22 August 2024;
Published: 10 September 2024.
Edited by:
Adam Schlosser, Massachusetts Institute of Technology, United StatesReviewed by:
Josef Křeček, Czech Technical University in Prague, CzechiaCopyright © 2024 Choudhary, Sihag and Dutt. This is an open-access article distributed under the terms of the Creative Commons Attribution License (CC BY). The use, distribution or reproduction in other forums is permitted, provided the original author(s) and the copyright owner(s) are credited and that the original publication in this journal is cited, in accordance with accepted academic practice. No use, distribution or reproduction is permitted which does not comply with these terms.
*Correspondence: Gitanshu Choudhary, bmJnaXRhbnNodUBnbWFpbC5jb20=
Disclaimer: All claims expressed in this article are solely those of the authors and do not necessarily represent those of their affiliated organizations, or those of the publisher, the editors and the reviewers. Any product that may be evaluated in this article or claim that may be made by its manufacturer is not guaranteed or endorsed by the publisher.
Research integrity at Frontiers
Learn more about the work of our research integrity team to safeguard the quality of each article we publish.