- 1School of Atmospheric Sciences, Sun Yat-sen University, Guangdong Province Key Laboratory for Climate Change and Natural Disaster Studies, Key Laboratory of Tropical Atmosphere-Ocean System, Ministry of Education, Zhuhai, China
- 2CMA Cloud-Precipitation Physics and Weather Modification Key Laboratory, Beijing, China
- 3National Observation and Research Station of Coastal Ecological Environments in Macao, Macao Environmental Research Institute, Macau University of Science and Technology, Macao, China
The “23.7” event, an extreme rainstorm that affected North China from July 29 to 2 August 2023, was simulated using the Weather Research and Forecasting (WRF) model, version 4.2. We focus on dynamically diagnosing and analyzing the mass and latent heat budgets of rainwater during the extreme precipitation event on July 31 in the Beijing area, where the hourly rainfall reached an extraordinary 111.8 mm. Generally, the model effectively simulated the rainstorm, enabling further assessment of the extreme precipitation. Results indicated that under the combined influence of three major weather systems—the residual circulation of Typhoon Doksuri (a low-pressure system after typhoon landfall), the embryonic stage of Typhoon Khanun, and the North China high-pressure dam—a continuous influx of moisture and energy was transported to the North China region, promoting heavy precipitation. Application of vorticity equation diagnostics indicates that the horizontal transport term is the primary source term. Mass balance analysis reveals that the primary source of rainwater is the accretion of cloud droplets by rain, and the condensation of water vapor into cloud droplets is the main contributor to the latent heat.
1 Introduction
Frequent occurrences of extreme heavy rainfall events have had a major impact on the socio-economic structure and ecological systems, causing widespread concern from all walks of life. (Min et al., 2011; Zahiri et al., 2016; Tellman et al., 2021). Although research into heavy rainfall mechanisms has advanced, accurately predicting these events remains a significant challenge due to their complexity, locality, and rapid variability (Christopher and Zhang, 2012; Gao et al., 2018; Yu et al., 2019). Northern China, particularly Beijing, has experienced a notable increase in extreme precipitation events, presenting critical challenges to urban infrastructure, ecological health, and public safety. Thus, a detailed investigation into the mechanisms of these events is essential not only for enhancing our understanding but also for improving forecast accuracy and developing effective disaster prevention and mitigation measures.
Previous studies have shown that cloud microphysical processes play a key role in precipitation formation and have a significant impact on the thermodynamic and cloud dynamical processes of various convective systems from mesoscale, and synoptic to large scales (Li et al., 2016; Shu et al., 2023; Gao et al., 2015). This effect is crucial for a comprehensive understanding of precipitation dynamics. Within clouds, the transformation of different types of hydrometeors (cloud water, rain, cloud ice, snow, graupel and hail) is controlled by complex cloud microphysical processes. These processes include the collision and coalescence of cloud water/rainwater, the melting and aggregation of cloud ice, and the accretion/boiling between supercooled water and ice particles, resulting in the phase change of water material (Takahashi and Kawano, 1998; Yang et al., 2015; Mao et al., 2018). Gao et al. (2021) observed that the budget of rainwater in South China rainstorms indicates that the warm-rain process plays a pivotal role during the initiation stage of convection. And previous studies have clarified that the main mechanism driving atmospheric precipitation is the large-scale movement and convergence of water vapor. These processes result in the condensation or freezing required for cloud formation and then the development and release of precipitation from these clouds (Cui, 2009; Yin et al., 2023). In addition, phase changes in hydrometeors and the resulting release of latent heat can significantly alter dynamic processes within clouds. Marinescu et al. (2016) noted that deposition and condensation were the primary reasons for the observed potential warming in simulated Mesoscale Convective Systems (MCS) during mid-latitude continental convective cloud experiments. Yin et al. (2023) demonstrated that microphysical processes typically cool the lower atmosphere while warming the upper troposphere. Clark et al. (2014) suggested that, during the mid-life of a system, cold pools are broadly related to system propagation speed, enabling the convective band to “sweep over” any convective cells triggered prior to the system. For the mechanism of precipitation, Gao et al. (2005) combined the water vapor budget with the cloud hydrometeor budget to derive a diagnostic equation-a surface rainfall budget equation in a two-dimensional cloud-resolving model framework. And then Huang et al. (2016) extended the 2D surface rainfall equation to the 3D WRF-based precipitation equation to examine the rainfall budget. Huang et al. (2019) calculated moisture, momentum, and heat budgets to study the mechanisms of record-breaking rainfall in South China.
Regarding heavy rains in Beijing, previous studies have mostly focused on the two extremely heavy rains of “7.21” (July 21–22, 2012, Beijing) and “7.20” (July 19–21, 2016, Beijing). The complex physical process mechanism of heavy rain formation is studied in many aspects such as large-scale circulation conditions, topographic effects, underlying surface effects, urban heat islands and urbanization, and mesoscale convective systems (Zhu and Xue, 2016; Dou and Miao, 2017; Chang et al., 2021; Li et al., 2020). Zhang and Zhai (2011) conducted a study on the spatiotemporal distribution characteristics of extreme precipitation in my country and pointed out that the probability of extreme precipitation events in North China is higher during the hour than around the evening. Li et al. (2017) found that the precipitation of hourly heavy rainfall events can reach more than 30% of the total precipitation in the warm season. Yang et al. (2021) found that when low-level warm and humid air encounters mountainous terrain, the local convergence formed under the influence of terrain uplift is very conducive to the triggering of convection and the occurrence and development of precipitation systems. Previous studies have mostly focused on the effects of microphysical processes on the formation and development stages of precipitation. Chen et al. (2021) found that compared to the bulk scheme, the bin scheme led to lower convective heights, which in turn resulted in stronger precipitation by the WRF model to diagnose mass and latent heat budgets in a heavy precipitation event in Beijing. The formation of cold pools during phase transitions of water substances also affects cloud dynamics. Mao et al. (2018) compared warm-sector precipitation and cold-frontal precipitation and found that the accretion of cloud droplets by rain was the biggest source of rainwater, the main cooling effect was due to the evaporation of rainwater and cloud water. However, the cloud microphysical transformation and dynamic and thermodynamic mechanisms during extremely heavy precipitation are not yet fully understood. So what are the mass source and sink terms at extremely heavy rainfall moments during heavy rain? What is the latent heat source and sink term? And what is the specific dynamic and thermodynamic mechanism? We conduct research on these issues.
An extreme precipitation event, known as the “23.7” Event, struck northern China from July 29 to 2 August 2023. Our focus is on a significant rainfall event in Beijing, where the hourly precipitation reached 111.8 mm. This study aims to explain this event’s development mechanism through a comprehensive analysis of microphysical processes, dynamic characteristics and their interactions. Utilizing the Gridpoint Statistical Interpolation (GSI) assimilation system, the Weather Research and Forecasting (WRF) mesoscale model, and observational data, this study investigates the critical characteristics of this rainstorm, which encompass thermodynamic processes, the mass balance of hydrometeors, and the energy budget. Furthermore, the research aims to develop a conceptual model that enhances understanding and prediction of such extreme precipitation events, thereby providing a scientific foundation for future weather forecasting and disaster management strategies.
2 Case overview, meteorological background, model description, and vertification
2.1 Case overview
The “23.7” event resulted in substantial economic losses, surpassing several billion yuan. In Beijing, this catastrophic event led to the tragic loss of 33 lives, with an additional 18 individuals reported missing. Remarkably, the total precipitation during this period represented 60% of Beijing’s annual average rainfall, totaling 331 mm. The districts of Mentougou and Fangshan recorded average rainfall figures of 538.1 mm and 598.7 mm, respectively. However, the most significant rainfall was observed at Changping’s Wangjiayuan Reservoir, which registered an unprecedented 744.8 mm of rain, marking the highest rainfall recorded in the city. Instrumental data confirm that this event represents the most substantial amount of precipitation recorded in the Beijing region in the past 140 years. Furthermore, Qianlingshan in Fengtai district experienced the highest hourly precipitation intensity on July 31 between 0200 and 0300 UTC, with an astonishing 111.8 mm falling per hour. This extreme weather event underscores the critical need for enhanced understanding and forecasting of meteorological phenomena to mitigate the impacts of such severe natural disasters.
2.2 Meteorological background
The meteorological background of the “23.7” event was analyzed using the European Centre for Medium-Range Weather Forecasts (ECMWF) ERA5 reanalysis data, featuring a horizontal resolution of 0.25° × 0.25°. Figure 1 shows the large-scale atmospheric circulations at 12 UTC on July 30 and 00 UTC on 31 July 2023, respectively.
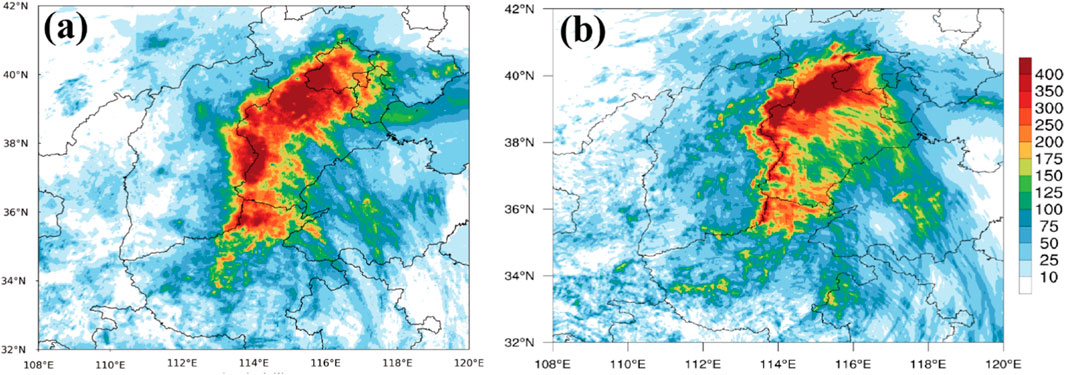
Figure 1. Distribution of 96-h (0000 UTC 29 July to 0000UTC 2 August) accumulated rainfall (mm; shaded) from the intensive surface rain gauge observations (A) and simulation (B).
On 30 July 2023, major atmospheric circulation patterns featured continental high pressure in the northwest, subtropical high pressure in the east, the residual circulation of Typhoon Doksuri in the southwest (a low-pressure system post-typhoon landfall), and the embryonic stage of Typhoon Khanun in the southeast at 0000 UTC. The intense rainfall was primarily driven by the northward and far inland movement of Doksuri’s residual circulation after landfall. This trajectory’s persistence necessitated a planetary-scale weather system larger than the typhoon itself, namely, the western Pacific subtropical high. This stable and relatively static subtropical high began its northward movement in mid-July and had strengthened by month’s end, moving slightly further north. The powerful Western Pacific subtropical high forced the typhoon’s remnants to move along its southwestern periphery, steering the airflow northwestward towards the Beijing area. By 0000 UTC on July 31, the continental and subtropical high pressures had interconnected, forming a robust and extensive “high-pressure dam” in the north. This dam blocked the northward movement of the typhoon’s remnant vortex, thereby triggering and sustaining heavy rainfall in the region. As the low vortex advanced northward, it encountered hindrance from the high-pressure dam, causing the North China region to remain under the influence of either the low vortex or its inverted trough. By 0000 UTC on July 31, the diminished inverted trough and the emerging warm shear line in central North China had created ideal conditions for heavy rainfall.
Abundant water vapor conditions were critical to the generation of sustained heavy rainfall. In this process, Doksuri and the embryonic Typhoon Khanun synergistically formed a potent water vapor transport channel. The southeastern jet stream between 700 hPa and 850 hPa in East China was abnormally strong, with jet core wind speeds of 20–24 m/s, and the specific humidity at 925 hPa reached 18 g/kg; the entire layer of precipitable water exceeded 75 mm (Figure 1).
To summarize, the genesis of this meteorological event was linked to the northward movement of Typhoon Doksuri’s remnants, which encountered substantial impediments due to the formidable blocking effect of the high-pressure system over North China. This phenomenon led to the stagnation of the typhoon’s progression. Concurrently, the persistent transport of moisture and energy from both Typhoon Doksuri and the developing Typhoon Khanun established a conducive environment. This setting, characterized by a stable, overarching large-scale atmospheric circulation, proved instrumental in fostering the formation and recurrent manifestation of mesoscale convective systems. These atmospheric configurations played a crucial role in facilitating the occurrence of significant heavy rainfall events, highlighting the complex and synergistic interactions between regional atmospheric disturbances and broader climatic systems in the genesis of extreme weather phenomena.
2.3 Model description and data
The observed precipitation data is sourced from the China Meteorological Administration Multisource Precipitation Analysis System (CMPAS) dataset, specifically the CMPAS_1 km_NRT. These datasets are provided by the National Meteorological Information Center (NMIC) of the China Meteorological Administration (CMA). The CMPAS_1 km_NRT is a radar–satellite–gauge merged precipitation product with a resolution of 0.01 × 0.01 (native resolution: 1 km), and the temporal resolution is hourly. The composite reflectivity and three-dimensional reflectivity factor data employed in this study is derived from a weather radar networking product, developed by the NMIC, CMA. This data is updated at intervals of 10 min. The single-station radar data referenced in this study is sourced from the S-band Doppler weather radar situated at the Haituoshan station in Beijing. Characterized by a wavelength of around 10 cm, this radar boasts a maximum detection range of 460 km and conducts comprehensive volumetric scans at 6-min intervals.
The three-dimensional and non-hydrostatic Advanced Research WRF (WRF-ARW) model, version 4.2, was used to simulate this “23.7” event. The numerical experiment consists of three one-way nested domains with 9-, 3-, and 1-km horizontal resolutions. We use 54 vertical levels from the surface to model top of 50 hpa. The horizontal grid points are 784 × 620, 1807 × 1,327, and 1,057 × 1,162, respectively.
The Kain-Fritsch cumulus parameterization (Kain, 1993; Kain, 2004) was applied in the 9 km domain (D01; Figure 2), but not in the 3 km domain (D02) and 1 km domain (D03; Figure 2). The other model physical parameterization schemes include the Morrison (2-moments) microphysics scheme, the Mellor-Yamada-Janjic (Eta) TKE planetary boundary layer scheme (Mesinger, 1992; Janjić, 1994) and the surface layer parameterization, the RRTMG long- and shortwave radiative scheme, and the unified Noah land surface model (Tewari et al., 2004).
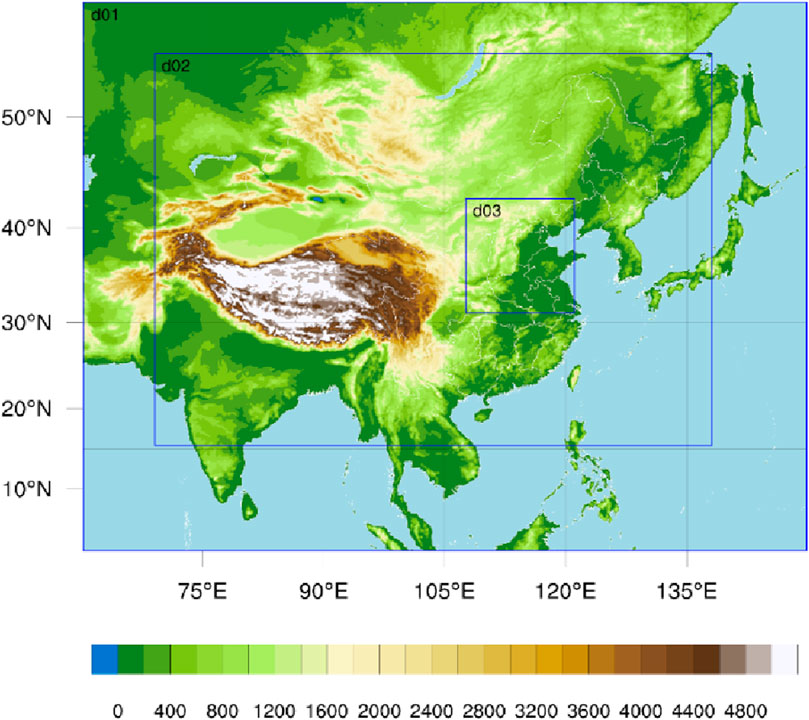
Figure 2. Nested model domains used for the WRF simulation with grid resolutions of 9 km (D01), 3 km (D02), and 1 km (D03). Shadings denote topography.
The WRF simulations over all three nested domains are integrated for 96 h, starting from 0000 UTC 29 July 2023, with outputs at 10-min intervals in D03. The European Centre for Medium-Range Weather Forecasts ERA5 reanalysis data, with a horizontal resolution of 0.25 × 0.25 and a temporal resolution of 3 h is used for the initial field and lateral boundary conditions.
In order to make the initial field of the model more accurate, we used the GSI assimilation system to assimilate the reflectance and radial wind of 49 SA-band weather radar data (including Beijing, Tianjin and Zhangjiakou in Hebei Province station) in the D01 and D02 regions nationwide (The quality control of radar-based data has been achieved through the automated radar pre-processing program 88d2arps developed by the Center for Storm Analysis and Forecasting (CAPS) at the University of Oklahoma); ground observation data from over 2000 stations of the National Station of the China Meteorological Administration (except for precipitation), including ground pressure, temperature, specific humidity, meridional wind, and zonal wind. The data undergo automated quality control during collection, including checking for extreme value ranges, and temporal and spatial consistency; the brightness temperature data from Himawari Satellite; and rainfall station data comes from the Meteorological Department, Information Center of the Ministry of Water Resources of People’s Republic of China. Rainfall stations are distributed in seven major river basins across China, with about 45,000 stations.
2.4 Model verification
From the 96-h cumulative precipitation data spanning July 29 to 2 August 2023, it is evident that the rain belt generally extends in a north-south direction, featuring two significant precipitation centers: one in the north stretching from western Beijing to central Hebei, and another in the south situated at the border between western Hebei and Shanxi Province (Figure 3A). The simulation results effectively capture the overall orientation of the rain belt and the locations of the intense precipitation centers, particularly in western Beijing (Figure 3B). However, the simulation slightly underestimates the southern precipitation center. This study primarily focuses on the intense precipitation in western Beijing from 0100 UTC to 0400 UTC on July 31, with an astonishing 111.8 mm falling per hour between 0200 and 0300 UTC. The 6-h cumulative precipitation data show that the model accurately simulates the intense precipitation center in the Beijing area, yet it underestimates precipitation in central Beijing and overestimates it in central and western Hebei (Figures 4A,B). Owing to the high precision of the simulation, some minor precipitation centers are detectable in the simulated data, which might go undetected in actual observations due to the lower resolution of observation stations. Regarding the hourly evolution of precipitation (Figure 5), the simulation shows a delay of approximately 2 hours compared to the observed data. However, the simulation accurately captures both the location of the intense precipitation center and the overall direction of the rain belt. Although the simulated area of intense precipitation is slightly smaller than observed, overall, the simulation of precipitation in western Beijing is relatively accurate.
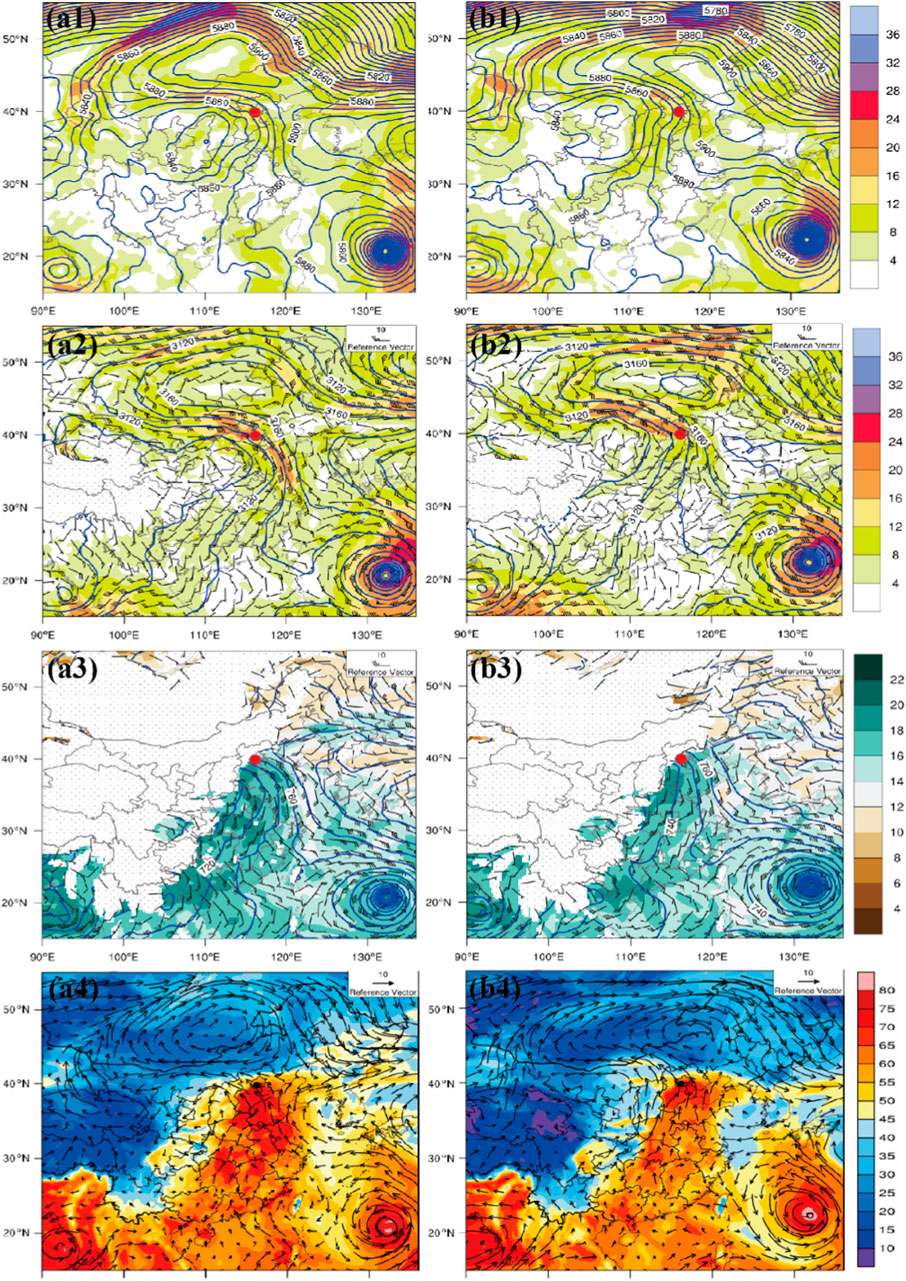
Figure 3. Synoptic-scale atmospheric circulation fields at (A1–A4) 1200UTC on 30 July and (B1–B4) 0000UTC on 31 July 2023. (A1, B1) Geopotential height (blue contours, units: gpm), wind speed (shadding, units: m/s) at 500 hpa; (A2, B2) Geopotential height (blue contours, units: gpm), wind field (barbs), wind speed (shadding, units: m/s) at 700 hpa; (A3, B3) Geopotential height (blue contours, units: gpm), wind field (barbs), specific humidity (shadding, units: kg/kg) at 925 hpa; (A4, B4) Atmospheric precipitable water (shading, units: mm) and wind vector at 500 hpa. The red dot indicates the location of Beijing.
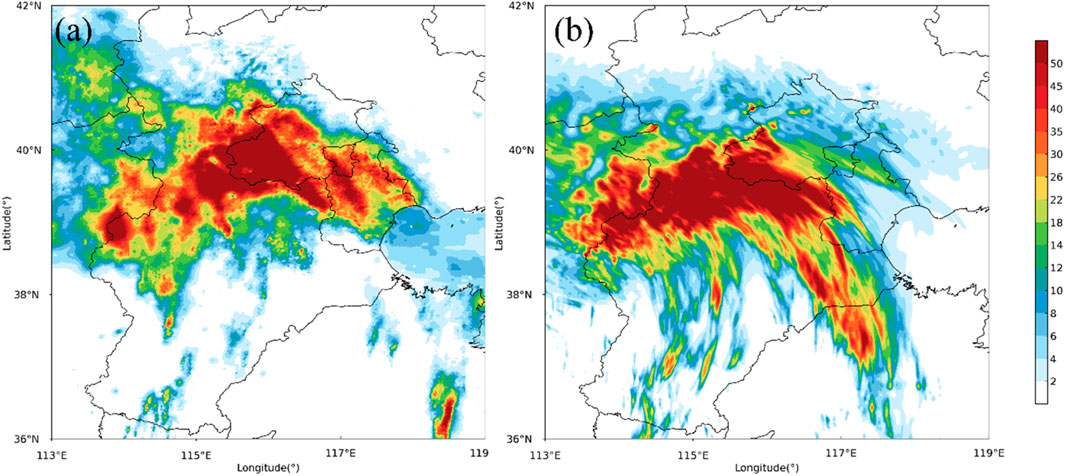
Figure 4. Distribution of observed (A), from 2300 UTC on 30 July to 0500 UTC on 31 July 2023) and simulated (B), from 2300 UTC on 30 July to 0500 UTC on 31 July 2023) 6-h accumulated rainfall (units: mm).
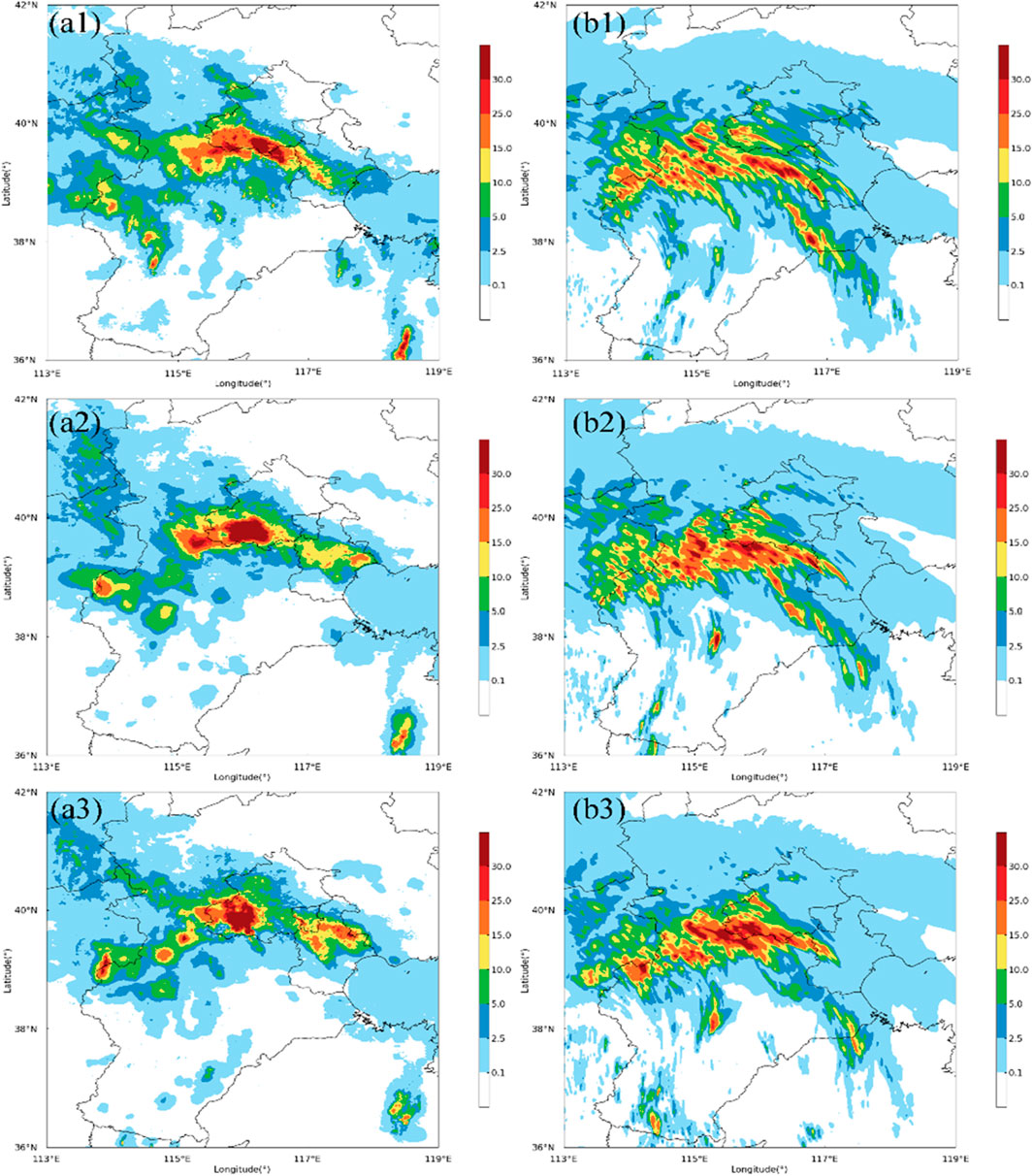
Figure 5. Distribution of observed (A1–A3) and simulated (B1-B3) 1-h accumulated rainfall (units: mm). The times are (A1) 0000UTC, (A2) 0100UTC, (A3) 0200UTC, (B1) 0200UTC (B2) 0300UTC (B3) 0400UTC 31 July 2023.
From the observation and simulation of radar reflectivity of convective cells, it is apparent that the mesoscale convective system causing heavy precipitation in Beijing originates from the Hebei convective system and moves northwest, culminating in heavy rain in Beijing. The high precision of the simulation facilitates the identification of numerous small convective systems in the radar plan view. At 2330 UTC on July 30, the convective system was located southeast of Beijing, with radar reflectivity at the convective center ranging between 45 and 50 dBZ (Figures 6A1, B1). At approximately 0030 UTC on July 31, after moving near the Fengtai district—a center of intense precipitation—the system not only maintained but also intensified, resulting in localized heavy rainfall at 0200 UTC with the convective center’s radar reflectivity exceeding 55 dBZ (Figures 6A2, B2, A6, B6). Overall, during the initial stages and throughout the movement of the convective system, the simulated convective center was slightly south of the actual location and covered a broader area, yet the simulation of the center’s intensity remained accurate. Additionally, the position of the mesoscale convective center during intense precipitation showed good correspondence between the simulation and observation.
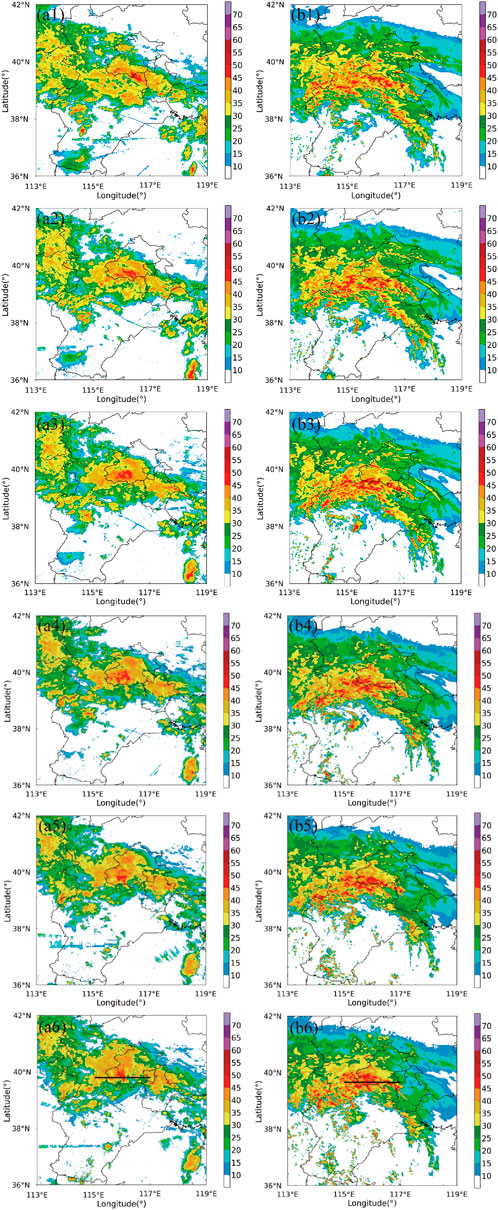
Figure 6. Time series of the observes radar reflectivity and simulated results (color shading; units: dBZ). The black straight lines in (A6, b6) denote locations of cross sections. The times are (A1) 2330UTC 30th July, (A2) 0000UTC, (A3) 0030UTC, (A4) 0100UTC (A5) 0130UTC (A6) 0200UTC 31 July 2023, (B1) 0150UTC, (B2) 0220UTC, (B3) 0250UTC, (B4) 0320UTC (B5) 0350UTC (B6) 0420UTC 31 July 2023.
As evident from the vertical cross sections of radar reflectivity (Figure 7), the observed radar reflectivity at 0030 UTC on July 31 (Figures 7A1, B1) shows the convective system just moving near 116°E. At this time, the convective system was relatively weak, with an overall radar reflectivity below 45 dBZ. Subsequently, the convective system began to intensify. The simulated cross-section at this moment indicates the onset of convection, with slightly weaker lower-level radar reflectivity, yet the location of convection initiation aligns well with observations. By 0130 UTC (Figures 7A2, B2), the convection had intensified, with 50 dBZ radar reflectivity extending up to 3 km and the 40–45 dBZ reflectivity heights surpassing the zero-degree layer. At the peak of convection at 0200 UTC (Figures 7A3, B3), the height of the 50 dBZ radar reflectivity surpassed the zero-degree layer, with 55 dBZ reflectivity developing above 3 km. At this point, a strong updraft was evident within the convective center, extending above 6 km. Overall, the location and intensity distribution of the radar echoes closely match the actual observations, making them suitable for further analysis.
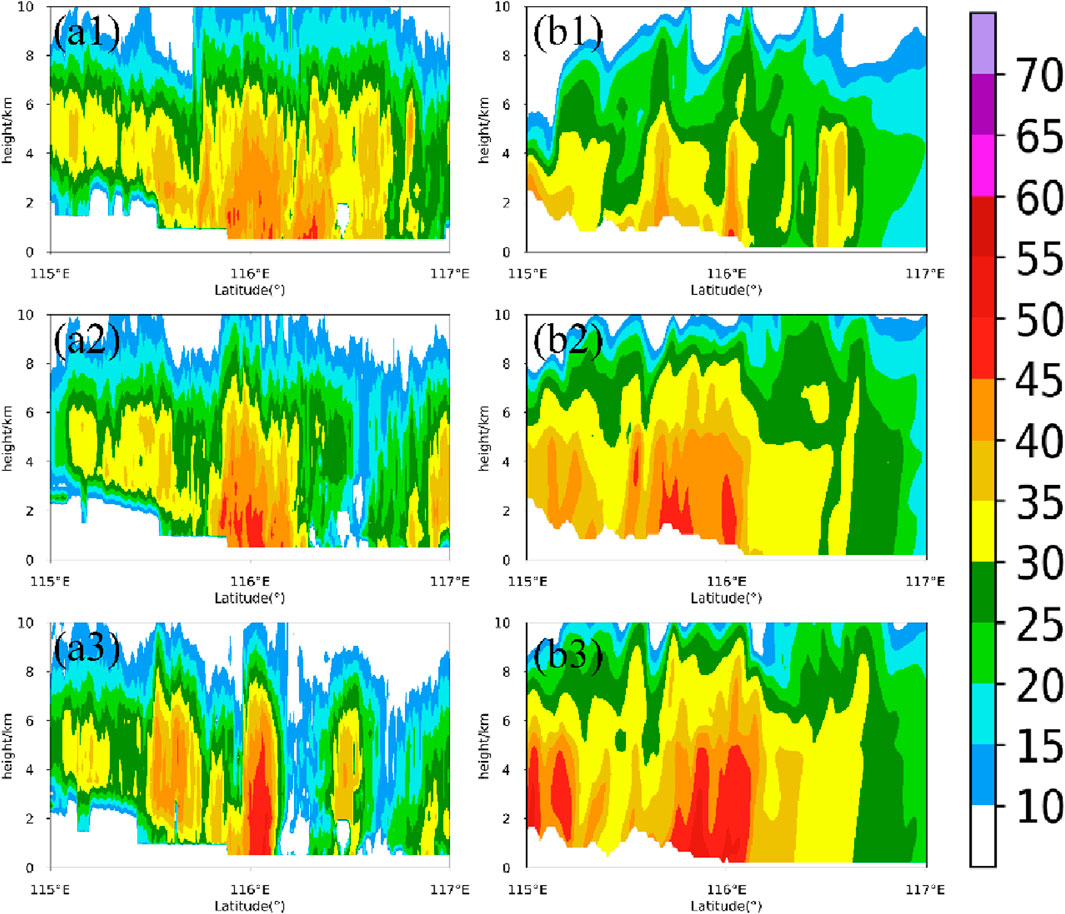
Figure 7. Vertical cross sections of radar reflectivity (color shading; units: dBZ) during the initiation, development and mature stages of observation (A1–A3) and simulation (B1–B3). The times are (A1) 0030UTC, (A2) 0130UTC, (A3) 0200UTC, (B1) 0250UTC x) 0340UTC (B3) 0400UTC 31 July 2023 (the same in subsequent similar figures.).
3 Dynamic, thermal, and microphysical characteristics of this torrential rain event
3.1 Dynamic and thermal characteristics
At the initial time (Figure 8A), vorticity is weak across all altitudes, with upward motion near the surface reaching approximately 1 m/s. A slight divergence field is evident at altitudes between 10 and 14 km. During the development stage (Figure 8B), two vorticity centers around 116°E at altitudes of 2 km and 6 km emerge and intensify rapidly. The upward motion strengthens significantly between 2 and 6 km, while the divergence field shows more modest development. In the mature stage (Figure 8C), from the surface up to 10 km, the convective center exhibits strong upper-level divergence and pronounced lower-level convergence, accompanied by clear upward motion. This pattern greatly facilitates convective development and the creation of strong instability. The overall structure, comprising upward motion, converging airflow, and diverging airflow branches, is well-aligned. The evolution of this rainstorm event shows a strong correlation with changes in the vorticity field, prompting further analysis using the vorticity equation (Feng et al., 2019; Huang et al., 2019; Fu et al., 2022). The vertical vorticity equation in Cartesian coordinates is expressed as follows:
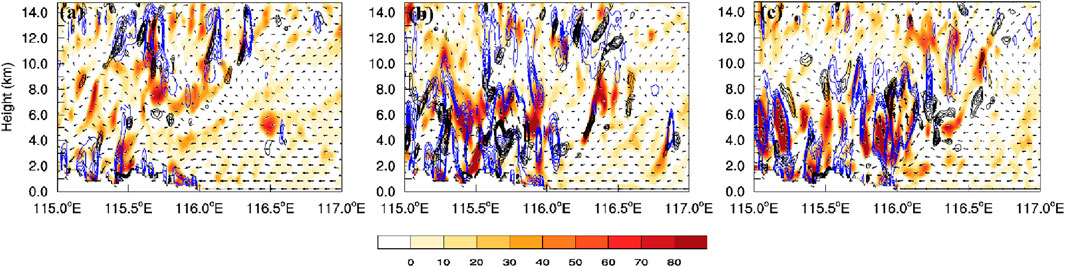
Figure 8. Vertical cross sections of the vorticity (color shading; unit: 10–5·s−1), divergence (black contours, the range is 40 to 120, 10 intervals; unit: 10–5·s−1), vertical velocity (blue contours, the range is 0.7 to 1.9, 0.3 intervals; unit: 10–5·s−1) during the initiation (A), development (B) and mature stages (C) of simulation.
In Equation 1,
Given the importance of vertical vorticity evolution in this “23.7” event, a budget analysis of vertical vorticity was conducted. Figure 9 displays vertical cross-sections showing trends in vertical vorticity due to horizontal advection, vertical advection, tilting, and stretching from the convective development to the peak phase. The solenoid and Coriolis advection terms are two orders of magnitude smaller than the aforementioned four terms and are thus ignored in this analysis. Furthermore, the residual term (RES) is sufficiently small to be neglected in this analysis.
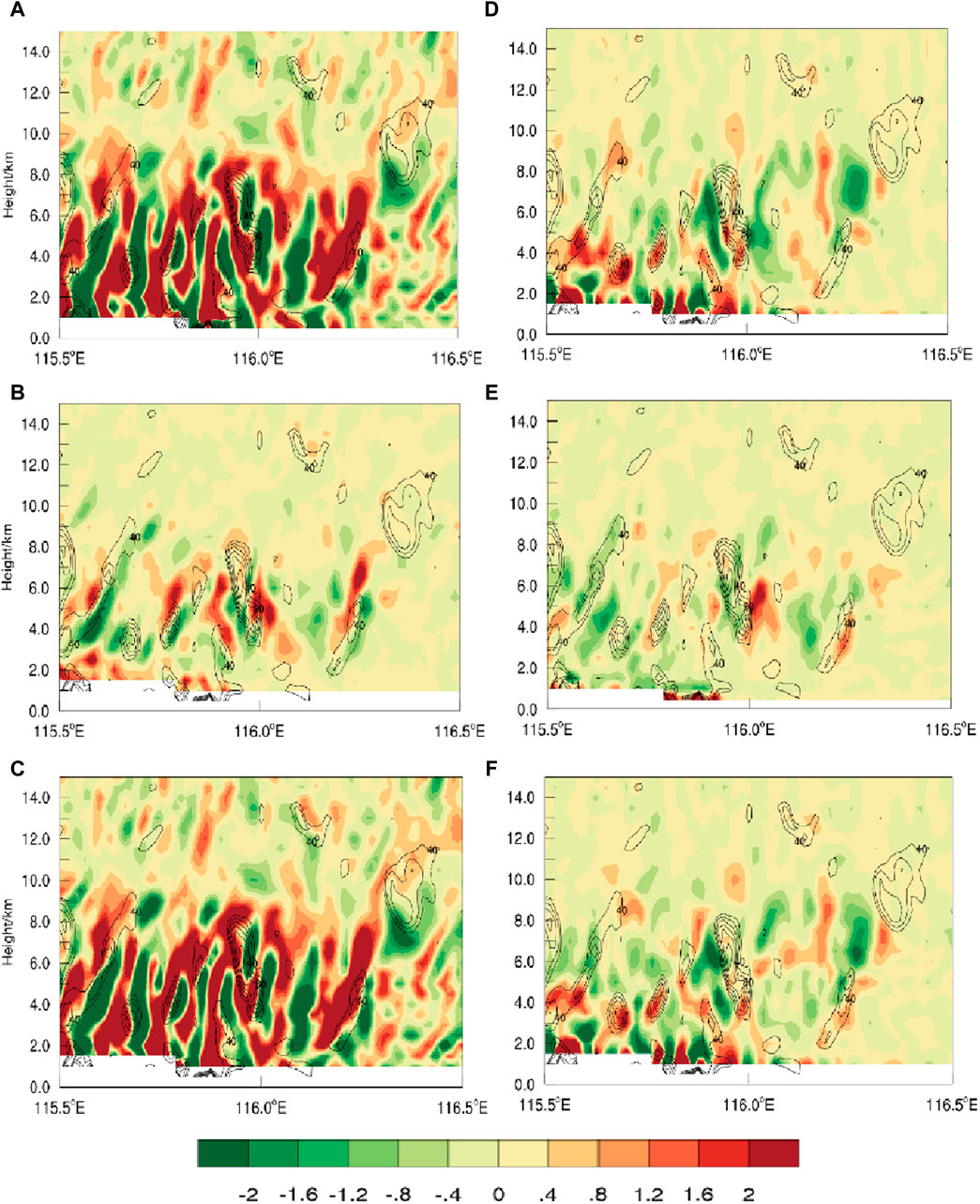
Figure 9. Vertical cross sections along the black thick solid lines shown in Figure 7 of the mature stage vertical vorticity tendencies (shaded, units: 10−6 s–2) due to (A) horizontal advection, (B) vertical advection, (C) total advection, (D) tilting, (E) stretching, and (F) sum of tilting and stretching. The contours in each panel indicate vertical vorticity (40, 60, 80, 100, 120, 140 × 10−5 s–1).
Horizontal transport is the primary source term, predominantly moving positive vertical vorticity to the left side of the maximum vertical vorticity center. Although weaker than horizontal transport, the vertical advection term mainly transports vertical vorticity from the lower to the upper levels, particularly to the right side of the maximum vertical vorticity center. Tilting was a significant source for the development of each vertical vorticity core, exerting a positive effect across the entire vertical vorticity center, primarily accumulating below the core of the positive vertical vorticity center. The stretching term additionally contributed to vertical vorticity development near 5 km (Figure 9E). Consequently, due to the horizontal and vertical transport of vorticity, local vorticity begins to develop. Strong updrafts enhanced the tilting and stretching of vertical vorticity (Figure 9F), generating robust vertical vorticity and subsequently inducing locally low dynamic perturbation pressure.
The cross-section of the disturbance in potential temperature and wind field (Figure 10) clearly illustrates these changes. In the initial stage, a thermal anomaly of approximately 0.5K is present at an altitude of 2–4 km, accompanied by a weak updraft due to early convection. As convection intensifies, the anomaly increases to approximately 2K at altitudes of 4 km and 8 km. At the peak of the event, the thermal anomaly extends to ground level, indicating strong upward motion and robust convective development.
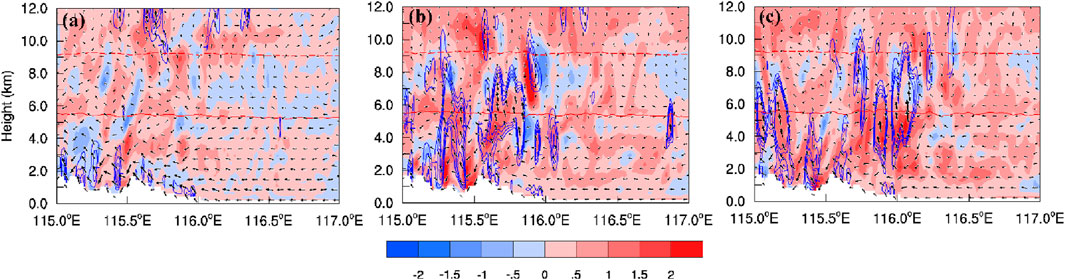
Figure 10. As in Figure 8 but for the disturbed potential temperature (color shading; units: K), vertical velocity (blue contours, the range is 0.7 to 1.9, 0.3 intervals; unit: 10−5·s−1) and wind field (vector arrows).
Simultaneously, the evolution of the convective system, through precipitation evaporation and heat absorption, cools the surrounding area, forming a cold pool within the lower 4 km layer, beneath the zero Celsius isotherm. The increase in upper-level potential temperature is attributed to water vapor condensation. The descent of hydrometeors facilitates the release of latent heat, thereby intensifying this effect. The formation of the cold pool augments thermodynamic compensation, thereby amplifying dynamical impacts. These combined thermal and dynamic processes crucially drive the development and escalation of the convective system.
Figure 11 displays vertical cross-sections of various hydrometeor mixing ratios and the vertical wind field, providing a comprehensive analysis of the interaction between the cloud water content and the dynamic field. Initially (not shown), the liquid water content was very low, accumulating mainly in the lower layers at below 1 g/kg, while solid water content was relatively higher, generally exceeding 1.8 g/kg. Rainwater particles were the primary contributors to the liquid water content, with a cloud water mixing ratio lower than that of rainwater and concentrated near the surface. Snow particles were the main source of solid water content, with a concentration of around 1.8 g/kg. The graupel mixing ratio was exceedingly low, below 0.1 g/kg, and ice particles, found mainly above 10 km, had a lower content. During the convective development phase, both liquid and solid water contents increased; however, rainwater and snow particles continued as the primary contributors to their respective categories. At this stage, the rainwater mixing ratio’s concentration center rose to 3 km, with a content of about 1.4 g/kg. The cloud water and ice mixing ratios remained low, while the graupel mixing ratio began to increase, reaching 0.6 g/kg at 6 km. The snow mixing ratio increased further to above 2.2 g/kg. In the mature stage of convection, a strong updraft emerged in the convective center, rapidly increasing the mixing ratios of all hydrometeors except for ice. At this stage, rainwater was the main contributor to liquid water, increasing sharply below the 0°C layer to above 2.4 g/kg. Meanwhile, the cloud water mixing ratio also increased near 5–8 km to 1 g/kg. Graupel and snow particles, due to increased concentrations, were the primary solid water contributors. Graupel particles increased significantly above the 0°C layer, reaching concentrations above 2 g/kg, while snow particle concentrations further increased to above 2.4 g/kg.
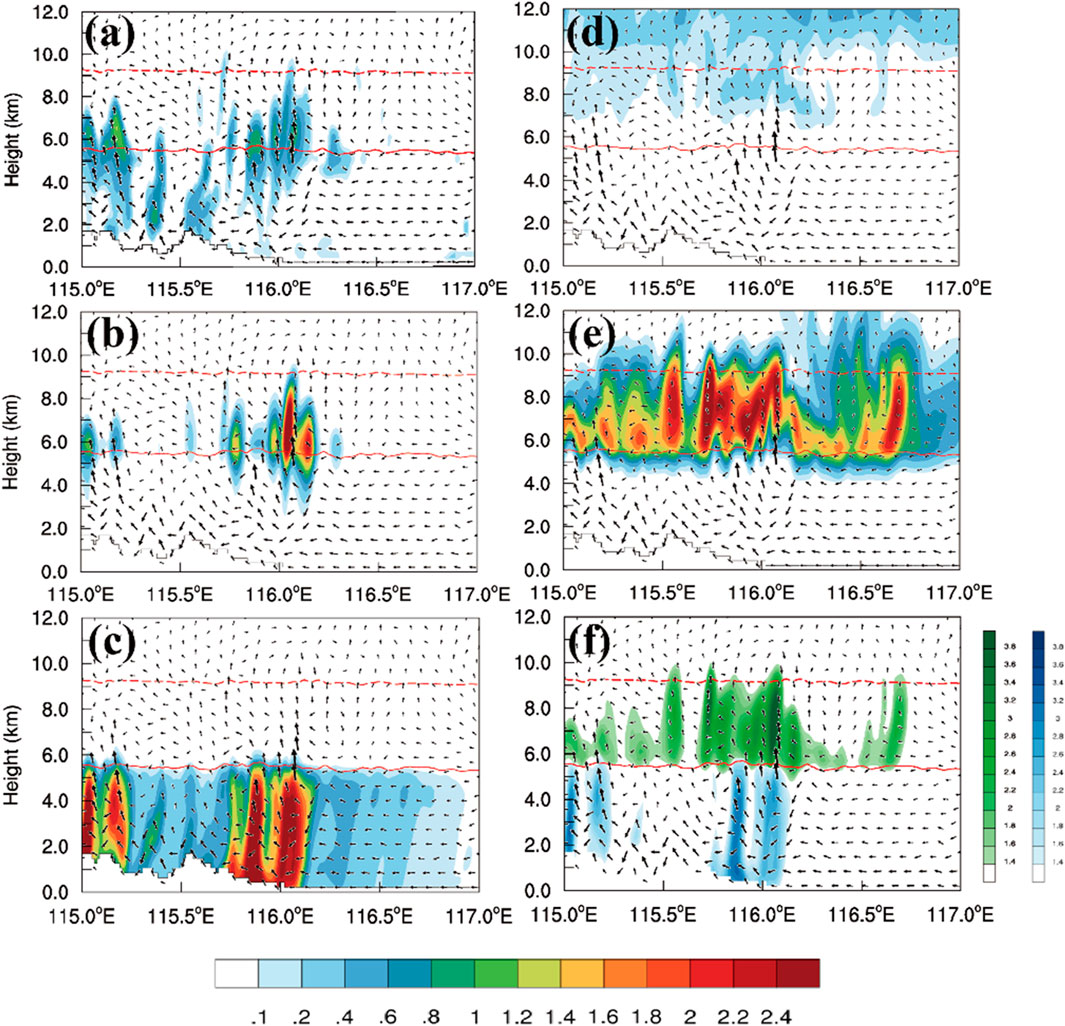
Figure 11. Vertical cross sections of various hydrometeors (color shading; units: g·kg−1) in mature stage. (A) cloud water; (B) cloud ice; (C) graupel; (D) snow; (E) rainwater; (F) the mixing ratio of liquid water (blue shading) and ice phrase (green shading).
In general, the formation of a cold surface generated a terrain-induced convergence line, resulting in strong updrafts. The convective center developed within an environment of latent heat release, with hydrometeor concentrations increasing as this environment intensified and expanded. During evaporation, rainwater absorbs heat, leading to the formation of a cold water pool in the lower atmosphere. In the upper atmosphere, water vapor condensed into ice particles, releasing heat during their descent; this heat was subsequently absorbed by the upper layers. This process resulted in a transformation from ice-phase to water-phase particles. This repetitive cycle established a dynamic interaction and feedback loop between the environmental and microphysical processes.
3.2 The microphysical characteristics
To accurately assess the impact of microphysical processes on the intense stage of convection during this torrential rain event, this section analyzes the hydrometeors within the convective system, focusing on the mass of rainwater and the latent heat budget. Utilizing previously discussed simulation data, this analysis diagnoses the microphysical processes during the mature stage of convective cells and their feedback on the mesoscale environment. A conceptual model has been developed to elucidate the potential mechanisms and effects contributing to the formation of convective cells.
From main source and sink items (Figure 12), during the mature phase of this precipitation event, the accretion of cloud droplets by rain is identified as the largest source term in the system, predominantly occurring between 2–6 km within the convective system, with an overall content exceeding 0.6 10−5 kg kg−1 s−1, accompanied by strong updrafts. This indicates that intense updrafts likely facilitate this process. The melting of graupel into rainwater, the second-largest contributor to rainwater, occurs primarily from 3 km up to the 0°C layer, with content about 0.6 10−5 kg kg−1 s−1, mainly distributed on both sides of the convective center and less so at the center itself, modestly present on the right side. Snow melting into rainwater, the third-largest source, primarily occurs from 4 km up to the 0°C height, with relatively lower content, roughly 0.25 10−5 kg kg−1 s−1, distributed on both sides of the convective center. In terms of sinks for rainwater mass, rain-graupel collection is the primary sink, distributed from the 0°C layer up to 7 km, with content approximately 0.3 10−5 kg kg−1 s−1; rain-snow collection, the second-largest sink, is more prominent on the left side of the convective center, with similar content, and less so on the right.
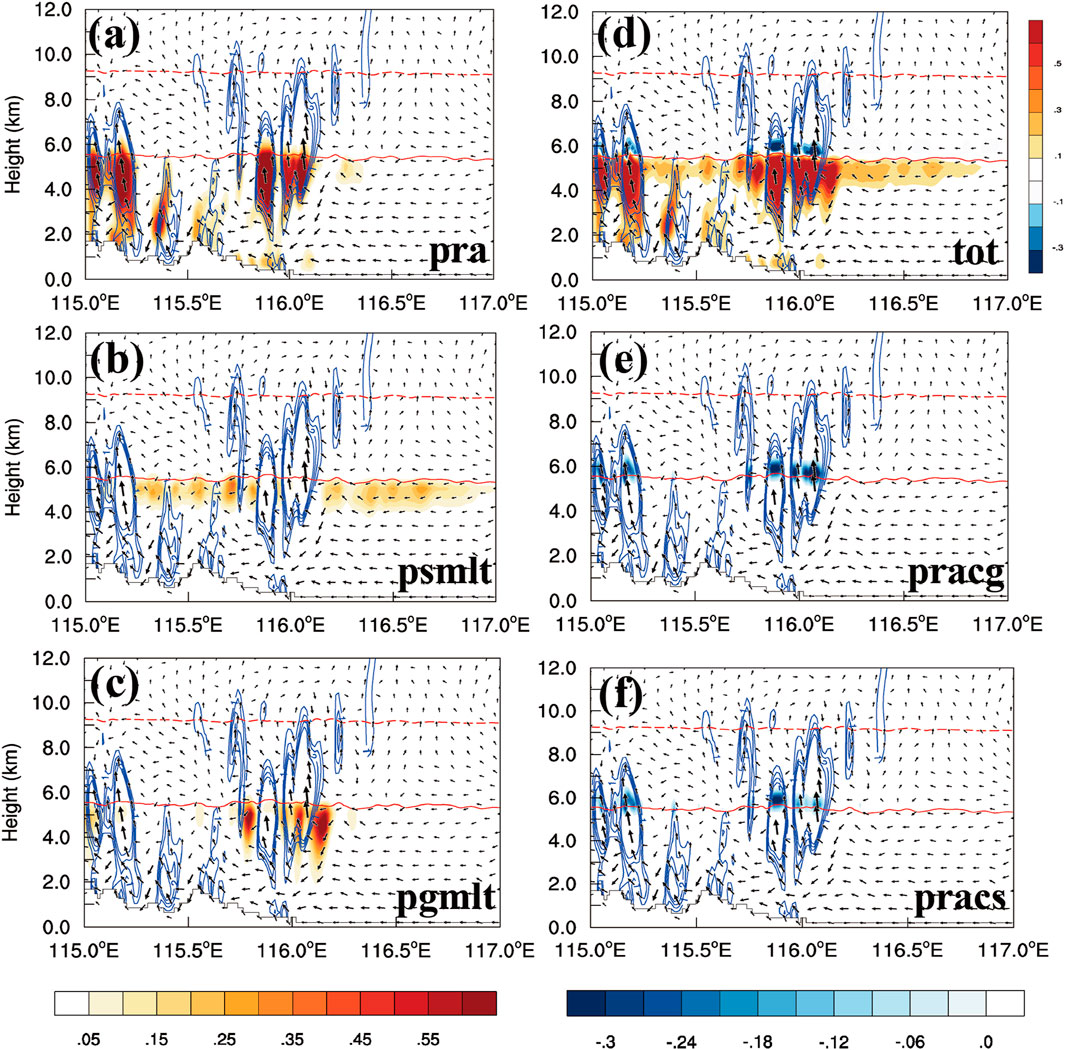
Figure 12. Vertical cross sections of main source (pra (A): accretion droplets by rain, psmlt (B): melting of snow, pgmlt (C): melting of graupel, color shading; units: 10–5 kg kg–1 s–1) and sink items (pracg (E): rain-graupel collection, pracs (F): conversion to graupel due to collection rain by snow, color shading; units: 10–5 kg kg–1 s–1) and net items (D), color shading; units: 10–5 kg kg–1 s–1) at the mature stage.
This aligns with the results from single-station dual-polarization radar measurements at Haituo Mountain (Figure 13), where the Correlation Coefficient near the convective center is close to 1, and the Differential Reflectivity values range from 1.5 to 2 dB. These values suggest that the convective center predominantly consists of medium-sized raindrops, corroborating the earlier finding that the accretion of cloud droplets by rain is the main source term.
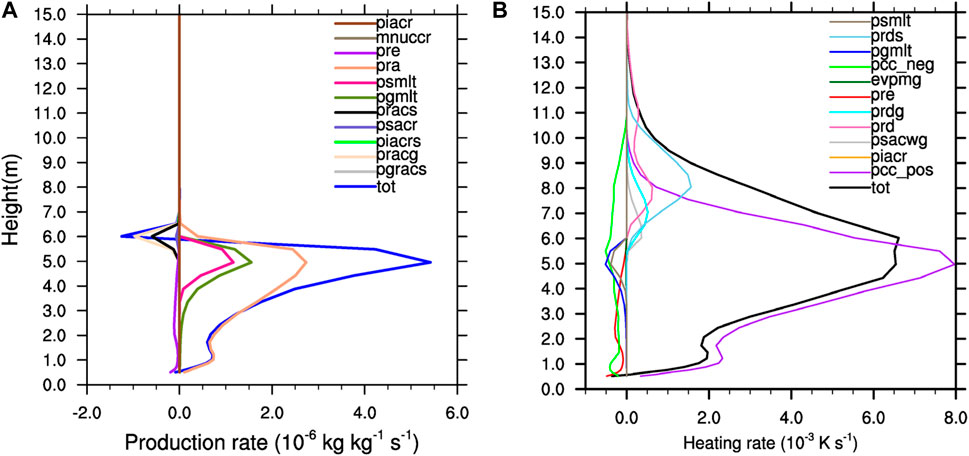
Figure 13. The mean production rates [(A), units: 10−6 kg kg−1 s−1] and mean heating rates of microphysical processes [(B), units: 10–3 K s−1] in heavy rainfall centers at the mature stage (0400UTC 31 July 2023). Here, piacr denotes ice-rain collection, mnuccr denotes contact freezing of rain, pre denotes evaporation of rain, pra denotes accretion droplets by rain, psmlt denotes melting of snow, pgmlt denotes melting of graupel, pracs denotes rain-snow collection, psacr denotes conversion due to collection of snow by rain, piacrs denotes ice-rain collection and added to snow, pracg denotes rain-graupel collection, pgracs denotes conversion to graupel due to collection rain by snow, prds denotes deposition of snow, pcc_neg denotes evaportaion of cloud droplets, evpmg denotes melting and evaporation of graupel, prdg denotes deposition of graupel, prd denotes deposition of cloud ice, psacwg denotes collection droplets by graupel, pcc_pos denotes condensation of cloud droplets.
The roles of latent heat release and cooling in cloud microphysical processes are crucial to the dynamic structure of intense convective systems. Therefore, we apply the latent heat formula to analyze the latent heat budget in hydrometeors, aiming to precisely understand the feedback of various microphysical processes on the mesoscale environment within the convective system. In cloud microphysics, condensation, freezing, and deposition contribute to heating, whereas evaporation, melting, and sublimation are cooling processes. Employing methodologies established by Hjelmfelt et al. (1989) and Guo et al. (1999), we can calculate the latent heating and cooling rates by
In Equation 2,
Figure 14B presents the profile of latent heat release terms for rainwater during the intense convective period of this precipitation event. It shows that the most significant latent heat release during this period is from the condensation of water vapor into cloud droplets, predominantly occurring below 8 km, including beneath the 0°C level. Above the 0°C layer, between 6 and 10 km, four processes contribute to latent heat release: the deposition of water vapor into snow, into ice crystals, into graupel, and the collection of droplets by graupel. The primary cooling processes include the evaporation of cloud droplets into water vapor, the evaporation of rainwater, the melting of graupel into rainwater, and the melting of snow into rainwater.
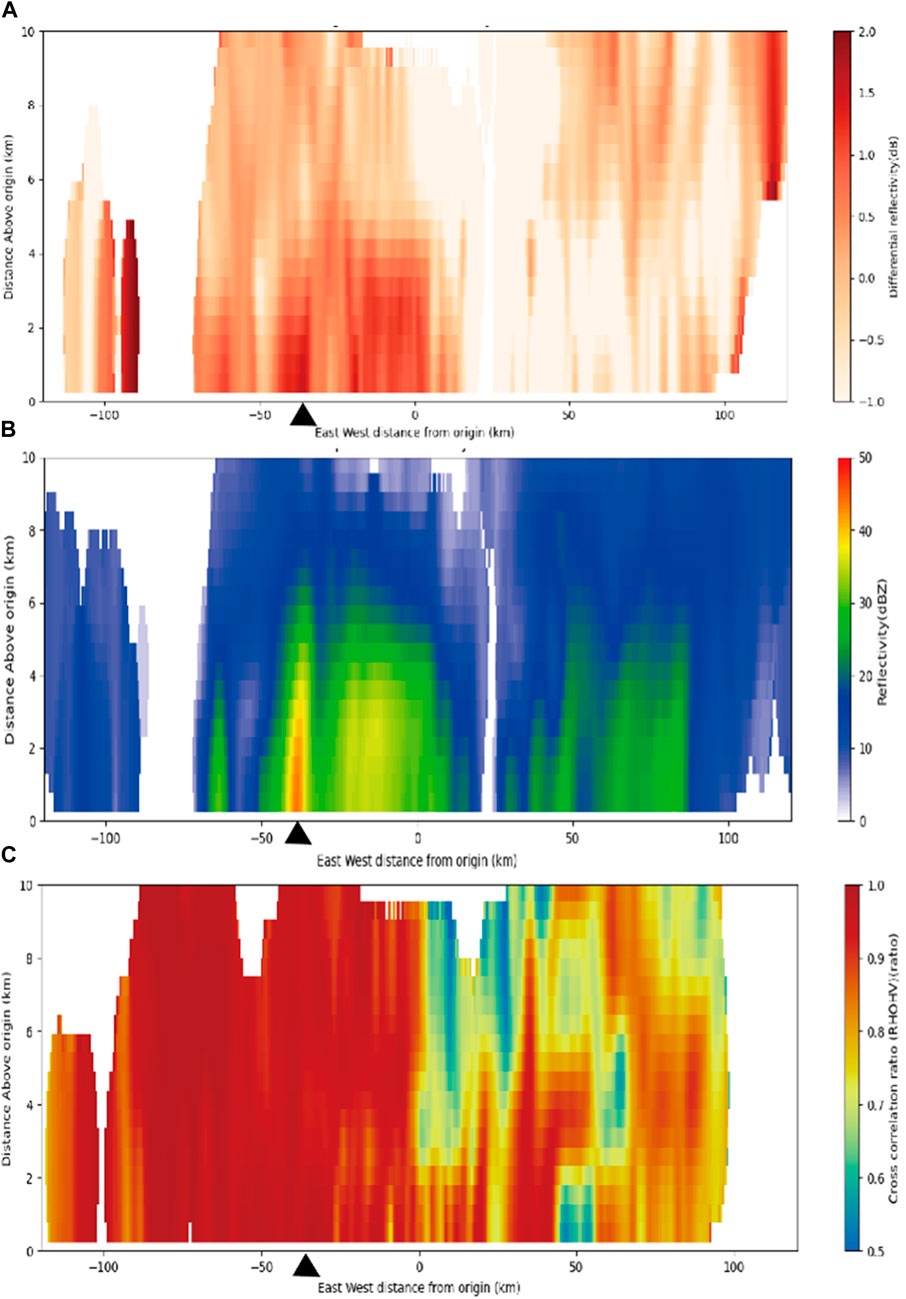
Figure 14. The polarization diagram of the single-station dual-polarization radar at Haituoshan in Beijing, including Differential Reflectivity (A), units: dB), Equivalent reflectivity factor (B), units: dBZ) and Correlation Coefficient (C).
Figure 15 further illustrates the profile distribution of the primary latent heat heating and cooling terms previously mentioned. As the largest latent heat release term, the condensation of water vapor into cloud droplets occurs predominantly throughout the interior of the convective center, accompanied by strong updrafts and an overall intensity exceeding 0.015 K s−1. As the second-largest latent heat release term, the deposition of water vapor into snow occurs primarily above 7 km, extending to the −20°C layer in the upper part of the convective center. Water vapor deposition into graupel and water vapor deposition into ice crystals, as the other latent heat release terms, have a relatively smaller impact on around 0.001 K s−1 and are primarily distributed at the upper part of the right side of the convective center. As the most significant latent heat cooling term, the evaporation of cloud droplets into water vapor primarily occurs around the 0°C layer of the convective center, absorbing heat at a rate of 0.003 K s−1. Another significant latent heat cooling term, the evaporation of rainwater into water vapor, is predominantly found in the lower layers, 2–4 km beneath the convective center. The remaining latent heat cooling terms, the melting of graupel and snow into rainwater, occur mainly at 4–6 km. The melting of graupel into rainwater is focused on both sides and particularly on the right side of the convective center, while the melting of snow into rainwater, which has a weaker cooling effect compared to graupel, is primarily on both sides of the convective center.
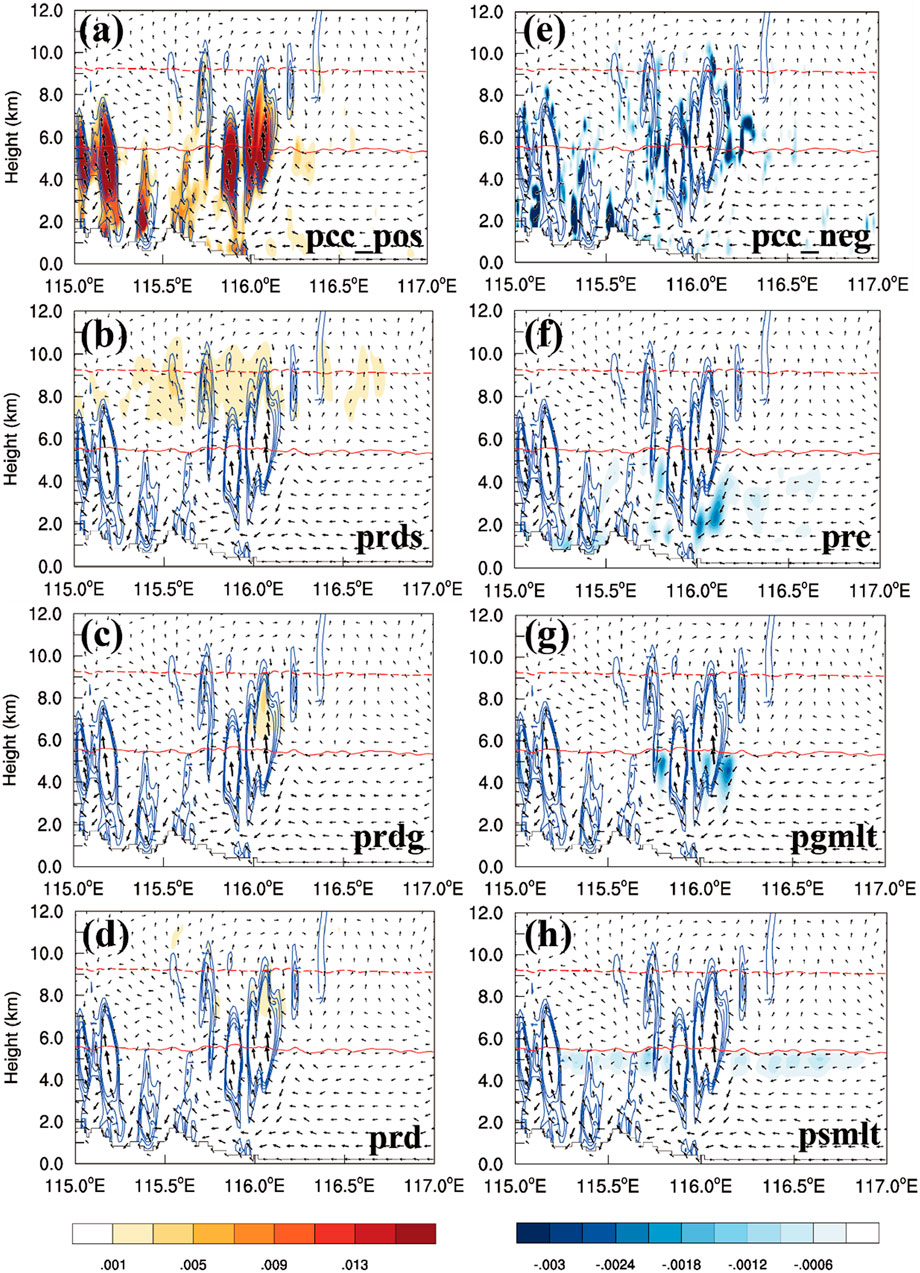
Figure 15. Vertical cross sections of main heating (A–D) and cooling items ((E–H), color shading; units: 10−5 kg kg−1 s−1) and net items (color shading; units: 10−5 kg kg−1 s−1) at the mature stage.
This suggests that latent heat absorption at the convective center enhances the thermal environment through the heat released by water particles. Concurrently, cooling processes beneath the 0°C layer amplify the cold pool and further intensify circulation, in conjunction with upper-level latent heat. This enhanced circulation facilitates the transformation among water particles, ultimately boosting rainwater production.
Based on the analysis above, we propose a conceptual model for the “23.7” event in Beijing (Figure 16), which thoroughly examines the physical mechanisms through which convection impacts precipitation. From a mass budget perspective, the primary source of rainwater is the accretion of cloud droplets by rain. This process is predominantly distributed from the ground up to the 0°C melting layer, pervading the entire convective core and creating a warm, moist environment beneath the melting layer. The second major source is the melting of graupel into rainwater, which occurs from the 0°C melting layer up to 3 km altitude, mainly located on either side of the convective center. The third source, the melting of snow into rainwater, occurs near the 0°C melting layer and is situated at the sides of the convective center.
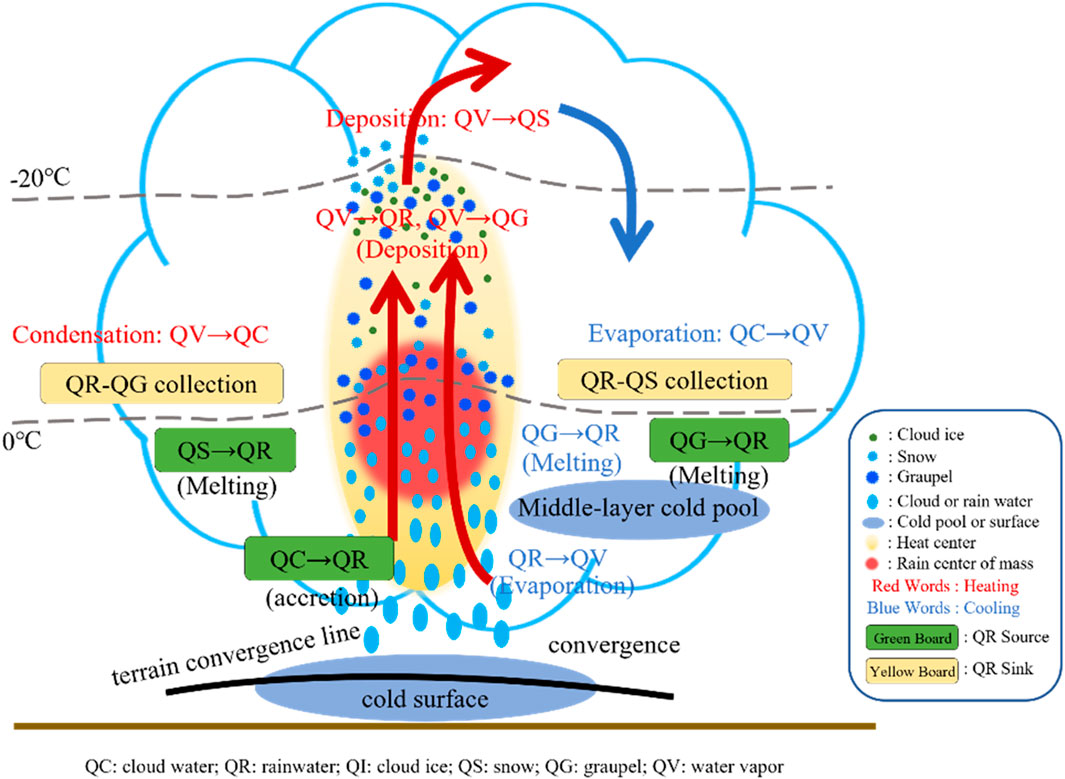
Figure 16. A conceptual model of the possible mechanism impact of cloud microphysical processes on hourly extreme precipitation in Beijing during the “23.7” event.
In terms of the heat budget, latent heat release from 2 km to near the −20°C layer is primarily caused by the condensation of water vapor into cloud droplets. The deposition of water vapor into graupel and ice crystals forms a heating center above the 0°C layer, while the deposition of water vapor into snow creates a heating center at the −20°C layer. Regarding the interplay between microphysical and dynamical processes, orographic lifting and convergence at the lower part of the convective center introduce a bottom-level inflow, resulting in strong upward motion. This strong upward motion enhances the accretion of cloud droplets by rain and forms a supercooled water region near the 0°C layer. Simultaneously, evaporation of cloud droplets into water vapor, melting of graupel, and snow into rainwater near the 0°C melting layer lead to the formation of a cold pool below this layer. The strong upward airflow within the convective center ascends and then descends in front of the center after flowing out from the top, forming a secondary circulation. The ascending branch of this circulation, influenced by the cold pool, further intensifies convection and can lead to the genesis of new convective cells.
4 Conclusion and discussion
This article presents a meteorological analysis, numerical simulation, and synoptic diagnosis of the “23.7” event that occurred in North China from July 29 to 2 August 2023. Subsequently, this study performs a dynamic diagnosis and analyzes the sources and sinks of rainwater mass and latent heat for the extreme precipitation event in Beijing on July 31, where the hourly rainfall reached 111.8 mm. This analysis identifies potential cloud microphysical processes and their feedback mechanisms during periods of extremely heavy rainfall. The main conclusions of this study are as follows:
1) The “23.7” event occurred as the remnants of Typhoon Doksuri moved northward, impeded and stalled by the blocking effect of the North China high-pressure dam. Against the backdrop of sustained moisture and energy transport from both Typhoon Doksuri and the embryonic form of Typhoon Khanun, the stable large-scale circulation created a favorable environment for the development and recurrent emergence of mesoscale convective systems, facilitating heavy rainfall.
2) Vorticity equation diagnostics indicate that the horizontal transport term is the primary source term, mainly moving positive vertical vorticity to the left side of the maximum vertical vorticity center. The vertical transport, tilting, and stretching terms have relatively smaller effects; however, their roles are significant and cannot be overlooked. The solenoid and Coriolis force terms are negligible due to their small magnitudes.
3) The mass balance analysis reveals that the primary source of rainwater is the accretion of cloud droplets by rain, predominantly distributed from the ground to the 0°C melting layer. This distribution engulfs the entire convective center, creating a warm, moist environment below the melting layer. The second and third major sources, the melting of graupel and snow into rainwater, are located on either side of the convective center, respectively.
4) The latent heat balance analysis indicates that the primary contributor to latent heat heating is the condensation of water vapor into cloud droplets. The deposition of water vapor into graupel and ice crystals forms a heating center above the 0°C layer, while the deposition into snow creates a heating center at the −20°C layer.
5) The latent heat release and cooling effects of microphysical processes enhance vertical updrafts in the secondary circulation, thereby increasing the buoyancy of the air mass. This increased buoyancy facilitates the initiation and development of convective cells, potentially representing a key mechanism through which microphysical processes influence weather patterns. The evaporation of cloud droplets into water vapor, along with the melting of graupel and snow into rainwater near the 0°C melting layer, contributes to the formation of a cold pool beneath this layer. The formation of this cold pool strengthens lifting actions, which in turn promote the development and intensification of new convection.
This research marks the first occasion that rainfall station data from the seven principal river basins under the Ministry of Water Resources have been assimilated, culminating in the successful simulation of the “23.7” event. Our findings reveal that at the peak of extreme precipitation, the predominant mass source term was the accretion of cloud droplets by rain, consistent with the findings of Mao et al. (2018). However, the primary heating sources were identified as the condensation of water vapor into cloud droplets, while the main cooling processes involved the evaporation of cloud droplets and rainwater into water vapor. Accurately representing such extreme precipitation events in cloud-resolving models remains a challenge; the effectiveness of these simulations largely depends on the choice of cloud microphysics scheme. Determining the most appropriate cloud microphysical scheme and reducing its associated uncertainties pose significant challenges for simulating heavy rainfall events. Therefore, future research should focus on comparative studies of cloud microphysical schemes under various heavy rainfall scenarios to enhance the accuracy and reliability of these models. Additionally, in future applications of artificial weather modification, referencing the transformation of water substances during heavy rains may catalyze the generation and consumption of precipitation.
Data availability statement
The data analyzed in this study is subject to the following licenses/restrictions: The datasets used or analysed during the current study are available from the corresponding author on reasonable request. Requests to access these datasets should be directed to XL, lixiang98@mail2.sysu.edu.cn; SZ, zhaosw@cma.gov.cn.
Author contributions
XL: Writing–review and editing, Writing–original draft, Visualization, Validation, Software, Resources, Project administration, Methodology, Investigation, Funding acquisition, Formal Analysis, Data curation, Conceptualization. SZ: Writing–review and editing, Visualization, Supervision, Software, Resources, Funding acquisition. DW: Writing–review and editing, Validation, Supervision, Software, Resources, Funding acquisition.
Funding
The author(s) declare that financial support was received for the research, authorship, and/or publication of this article. This work was supported and funded by Guangdong Major Project of Basic and Applied Basic Research Guangdong Major Project of Basic and Applied Basic Research (grant number: 2020B0301030004), China Meteorological Administration Innovation and Development Initiative (grant number: CXFZ2024J029), and National Key R&D Program of China (grant number: 2019YFC1510400).
Conflict of interest
The authors declare that the research was conducted in the absence of any commercial or financial relationships that could be construed as a potential conflict of interest.
Publisher’s note
All claims expressed in this article are solely those of the authors and do not necessarily represent those of their affiliated organizations, or those of the publisher, the editors and the reviewers. Any product that may be evaluated in this article, or claim that may be made by its manufacturer, is not guaranteed or endorsed by the publisher.
References
Chang, C., Li, Y., Chen, Y., Huang, J. J., and Zhang, Y. (2021). Advanced statistical analyses of urbanization impacts on heavy rainfall in the Beijing metropolitan area. Urban Clim. 40, 100987. doi:10.1016/j.uclim.2021.100987
Chen, Y., Ping, F., Zhou, S., Shen, X., and Sun, Y. (2021). Influence of microphysical processes on the initiation of the mesoscale convective system of a rainstorm over Beijing. Atmos. Res. 254, 105518. doi:10.1016/j.atmosres.2021.105518
Christopher, M., and Zhang, F. Q. (2012). Practical and intrinsic predictability of severe and convective weather at the mesoscales. J. Atmos. Sci. 69, 3350–3371. doi:10.1175/jas-d-11-0315.1
Clark, P. A., Browning, K. A., Forbes, R. M., Morcrette, C. J., Blyth, A. M., and Lean, H. W. (2014). The evolution of an MCS over southern England. Part 2: model simulations and sensitivity to microphysics. Q. J. R. Meteorological Soc. 140 (679), 458–479. doi:10.1002/qj.2142
Cui, X. P. (2009). Quantitative diagnostic analysis of surface rainfall processes by surface rainfall equation. Chin. J. Atmos. Sci. 33 (2), 375–387. doi:10.3878/j.issn.1006-9895.2009.02.15
Dou, J. J., and Miao, S. G. (2017). Impact of mass human migration during Chinese New Year on Beijing urban heat island. Int. J. Climatol. 37 (11), 4199–4210. doi:10.1002/joc.5061
Feng, S. L., Jin, S. L., Fu, S. M., Sun, J.-H., and Zhang, Y. C. (2019). Formation of a Kind of heavy-rain-producing mesoscale vortex around the Sichuan basin: an along-Track vorticity budget analysis. Atmos. Sci. Lett. 2019, e949. doi:10.1002/asl2.949
Fu, S. M., Zhang, Y. C., Wang, H. J., Tang, H., Li, W. L., and Sun, J. H. (2022). On the evolution of a long-lived mesoscale convective vortex that acted as a crucial condition for the extremely strong hourly precipitation in Zhengzhou. JGR. Atmos. 127, e2021JD036233. doi:10.1029/2021jd036233
Gao, R., Wei, Z. G., Dong, W. J., and Zhong, H. L. (2005). Impact of the anomalous thawing in the Tibetan Plateau on summer precipitation in China and its mechanism. Adv. Atmos. Sci. 22 (2), 238–245. doi:10.1007/bf02918513
Gao, S. T., Tan, Z. M., Zhao, S. X., Luo, Z., Lu, H., Wang, D., et al. (2015). Mesoscale dynamics and its application in torrential rainfall systems in China. Adv. Atmos. Sci. 32 (2), 192–205. doi:10.1007/s00376-014-0005-x
Gao, W., Liu, L., Li, J., and Lu, C. (2018). The microphysical properties of convective precipitation over the Tibetan Plateau by a subkilometer resolution cloud-resolving simulation. J. Geophys. Res. Atmos. 123, 3212–3227. doi:10.1002/2017JD027812
Gao, W. H., Xue, L. L., Liu, L. P., Lu, C. S., Yun, Y. X., and Zhou, W. H. (2021). A study of the fraction of warm rain in a pre-summer rainfall event over South China. Atmos. Res. 262, 105792. doi:10.1016/j.atmosres.2021.105792
Guo, X. L., Niino, H., and Kimura, R. (1999). Numerical modeling on a hazardous microburst-producing hailstorm. In Towards digital Earth proceedings of the international symposium on digital Earth. Vol. 1. Science Press: Beijing, 383–398.
Hjelmfelt, M. R., Roberts, R. D., Orville, H. D., Chen, J. P., and Kopp, F. J. (1989). Observational and numerical study of a microburst line-producing Storm. J. Atmos. Sci. 46, 2731–2744. doi:10.1175/1520-0469(1989)046<2731:oansoa>2.0.co;2
Huang, Y., Liu, Y., Liu, Y., and Knievel, J. C. (2019). Budget analyses of a record-breaking rainfall event in the coastal metropolitan city of Guangzhou, China. J. Geophys. Res. Atmos. 124 (16), 9391–9406. doi:10.1029/2018JD030229
Huang, Y. J., Cui, X. P., and Li, X. F. (2016). A three-dimensional WRF-based precipitation equation and its application in the analysis of roles of surface evaporation in a torrential rainfall event. Atmos. Res. 169, 54–64. doi:10.1016/j.atmosres.2015.09.026
Janjić, Z. I. (1994). The step-mountain eta coordinate model: Further developments of the convection, viscous sublayer, and turbulence closure schemes. Mon. Wea. Rev. 122, 927–945. doi:10.1175/1520-0493(1994)1220927:TSMECM2.0.CO;2
Kain, J. S., and Fritsch, J. M. (1993). Convective parameterization for mesoscale models: The Kain–Fritsch scheme. The Representation of Cumulus Convection in Numerical Models. Meteor. Monogr. 24, 165–170. doi:10.1175/0065-9401-24.46.1
Kain, J. S. (2004). The Kain–Fritsch convective parameterization: An update. J. Appl. Meteor. 43, 170–181. doi:10.1175/15200450(2004)043
Li, H. Q., Cui, X. P., and Zhang, D. L. (2017). A statistical analysis of hourly heavy rainfall events over the Beijing metropolitan region during the warm seasons of 2007-2014. Int. J. Climatol. 37 (11), 4027–4042. doi:10.1002/joc.4983
Li, J. N., Ding, C. H., Li, F. Z., and Chen, Y. L. (2020). Effects of single- and double-moment microphysics schemes on the intensity of super typhoon Sarika (2016). Atmos. Res. 238, 104894. doi:10.1016/j.atmosres.2020.104894
Li, J. N., Wu, K. L., Li, F. Z., Chen, Y. L., and Huang, Y. B. (2016). Cloud-scale simulation study on the evolution of latent heat processes of mesoscale convective system accompanying heavy rainfall: the Hainan case. Atmos. Res. 169, 331–339. doi:10.1016/j.atmosres.2015.10.014
Mao, J., Ping, F., Yin, L., and Qiu, X. (2018). A study of cloud microphysical processes associated with torrential rainfall event over Beijing. J. Geophys. Res. Atmos. 123, 8768–8791. doi:10.1029/2018JD028490
Marinescu, P. J., van den Heever, S. C., Saleeby, S. M., and Kreidenweis, S. M. (2016). The microphysical contributions to and evolution of latent heating profiles in two MC3E MCSs. J. Geophys. Res. Atmos. 121, 7913–7935. doi:10.1002/2016jd024762
Mesinger, F., and Black, T. L. (1992). On the impact on forecast accuracy of the step-mountain (eta) vs. sigma coordinate. Meteorol. Atmos. Phys. 50, 47–60. doi:10.1007/BF01025504
Min, S. K., Zhang, X., Zwiers, F., and Hegerl, G. C. (2011). Human contribution to more-intense precipitation extremes. Nature 470, 378–381. doi:10.1038/nature09763
Shu, W. X., Fu, D. H., Xiao, H., Yang, H., Sun, Y., Guo, X., et al. (2023). Cloud microphysical processes and atmospheric water budget during the 20 July 2021 extreme precipitation event in Zhengzhou, China. J. Meteor. Res. 37 (5), 722–742. doi:10.1007/s13351-023-2166-y
Takahashi, T., and Kawano, T. (1998). Numerical sensitivity study of rainband precipitation and evolution. J. Atmos. Sci. 55 (1), 57–87. doi:10.1175/1520-0469(1998)055<0057:nssorp>2.0.co;2
Tellman, B., Sullivan, J. A., Kuhn, C., Kettner, A. J., Doyle, C. S., Brakenridge, G. R., et al. (2021). Satellite imaging reveals increased proportion of population exposed to floods. Nature 596, 80–86. doi:10.1038/s41586-021-03695-w
Tewari, M., Chen, F., Wang, W., Dudhia, J., Lemone, M. A., and Mitchell, K. E. (2004). “Implementation and verification of the unified NOAH l and surface model in the WRF model,”in Proc. 20th Conference on Weather Analysis and Forecasting/16th Conference on Numerical Weather Prediction, American Meteorological Society, Seattle, United States, January 14, 2004.
Yang, H. L., Xiao, H., and Guo, C. W. (2015). Structure and evolution of a squall line in northern China: a case study. Atmos. Res. 158-159, 139–157. doi:10.1016/j.atmosres.2015.02.012
Yang, L., Chen, M., Wang, X., Song, L., Yang, M., Qin, R., et al. (2021). Classification of precipitation type in north China using model-based explicit fields of hydrometeors with modified thermodynamic conditions. Weather Forecast. 36 (1), 91–107. doi:10.1175/waf-d-20-0005.1
Yin, L., Ping, F., Mao, J. H., and Jin, S. G. (2023). Analysis on precipitation efficiency of the “21.7 ” Henan extremely heavy rainfall event. Adv. Atmos. Sci. 40 (3), 374–392. doi:10.1007/s00376-022-2054-x
Yu, R. C., Zhang, Y., Wang, J. J., Li, J., Chen, H. M., Gong, J. D., et al. (2019). Recent progress in numerical atmospheric modeling in China. Adv. Atmos. Sci. 36 (9), 938–960. doi:10.1007/s00376-019-8203-1
Zahiri, E. P. I., Bamba, A. M., Famien, A. K., Koffi, A., and Ochou, D. (2016). Mesoscale extreme rainfall events in west Africa: the cases of Niamey (Niger) and the upper Ouémé valley (Benin). Weather Clim. Extrem. 13, 15–34. doi:10.1016/j.wace.2016.05.001
Zhang, H., and Zhai, P. M. (2011). Temporal and spatial characteristics of extreme hourly precipitation over eastern China in the warm season. Adv. Atmos. Sci. 28, 1177–1183. doi:10.1007/s00376-011-0020-0
Keywords: microphysical processes, extremely heavy rainfall, Beijing, “23.7”, budget analysis
Citation: Li X, Zhao S and Wang D (2024) Roles of synoptic characteristics and microphysics processes on the heavy rain event over Beijing region during 29 July to 2 August 2023. Front. Earth Sci. 12:1394342. doi: 10.3389/feart.2024.1394342
Received: 01 March 2024; Accepted: 03 June 2024;
Published: 19 July 2024.
Edited by:
Xuejin Zhang, Atlantic Oceanographic and Meteorological Laboratory (NOAA), United StatesReviewed by:
Bin Chen, Chinese Academy of Meteorological Sciences, ChinaChunsong Lu, Nanjing University of Information Science and Technology, China
Copyright © 2024 Li, Zhao and Wang. This is an open-access article distributed under the terms of the Creative Commons Attribution License (CC BY). The use, distribution or reproduction in other forums is permitted, provided the original author(s) and the copyright owner(s) are credited and that the original publication in this journal is cited, in accordance with accepted academic practice. No use, distribution or reproduction is permitted which does not comply with these terms.
*Correspondence: Donghai Wang, wangdh7@mail.sysu.edu.cn
†These authors have contributed equally to this work