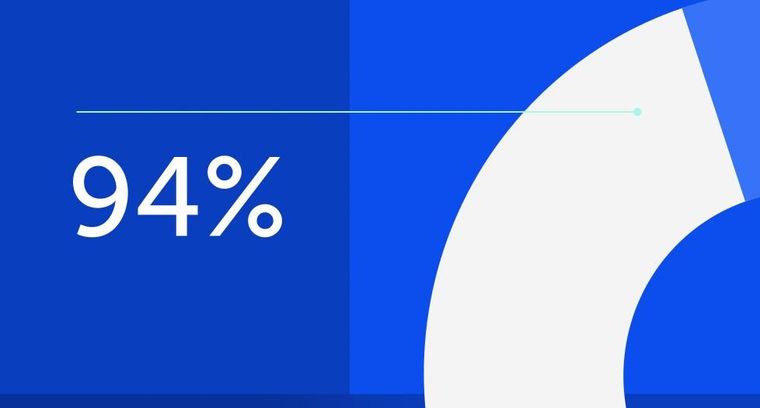
94% of researchers rate our articles as excellent or good
Learn more about the work of our research integrity team to safeguard the quality of each article we publish.
Find out more
REVIEW article
Front. Earth Sci., 10 May 2024
Sec. Cryospheric Sciences
Volume 12 - 2024 | https://doi.org/10.3389/feart.2024.1381323
This article is part of the Research TopicRemote Sensing of the CryosphereView all 10 articles
The spatial and temporal variation of the seasonal snowpack in mountain regions is recognized as a clear knowledge gap for climate, ecology and water resources applications. Here, we identify three salient topics where recent developments in snow remote sensing and data assimilation can lead to significant progress: snow water equivalent, high resolution snow-covered area and long term snow cover observations including snow albedo. These topics can be addressed in the near future with institutional support.
Every year, the seasonal snow covers up to 40% of the Earth’s land surface, including 45% of the global mountain area (i.e. 21 millions km2, Figure 1). As a result, the snow cover is a key driver of ecological, atmospheric, and hydrological processes in mountain environments. In addition, snowmelt from mountain regions represents an important contribution to river flow and groundwater recharge in many anthropized catchments, providing water resources to billions of humans (Mankin et al., 2015; Sturm et al., 2017; Qin et al., 2020). Consistent and reliable observations on the physical properties of the mountain snow cover are needed both for understanding mountain ecosystems but also in an operational context for water resources management and weather forecasting. Given the high spatial and temporal variability of seasonal snow in mountain regions, satellite observations have become an irreplaceable asset to monitor snow cover in complement to in situ observations and model simulations especially in data scarce regions (Fayad et al., 2017; Dong, 2018). In addition, long term observations of the seasonal snow cover are critically needed to evaluate the pace and the impact of climate change in mountain regions. Yet, the spatial and temporal variation of snow cover was listed as one of the clear knowledge gaps in the High Mountain Areas chapter of The Ocean and Cryosphere in a Changing Climate Special Report of the Intergovernmental Panel on Climate Change (2022). In NASA’s Decadal Survey, “Quantify rates of snow accumulation, snowmelt, ice melt, and sublimation from snow and ice worldwide at scales driven by topographic variability” was prioritized as one of the most important objectives (National Academies of Sciences, Engineering, and Medicine, 2018). Snow-related variables in the Decadal Survey are “snow depth and snow water equivalent, including high spatial resolution in mountain areas,” and “snow reflectivity” is also mentioned among the “surface biology and geology” observables.
Figure 1. Spatial distribution of the snow cover duration and the evolution of the snow-covered area at global scale as observed from space over the past 2 decades. The snow cover area time series was computed in four land classes (Greenland, Antarctica, mountains regions and flat areas) to highlight the significance of the seasonal snow in mountain regions. The snow-covered area was obtained from MOD10C1 monthly products (Hall and Riggs, 2021) and post-processed to fill missing values using a linear interpolation in the time dimension. Mask of the mountain areas sourced from Viviroli et al. (2020). The snow cover area time series indicate that the interannual variability is low in comparison with the seasonal variability.
Acknowledging the importance of monitoring the snow cover evolution across our planet, the Global Climate Observing System (GCOS) stated the snow-covered area, snow water equivalent and snow depth as essential climate variables (ECVs) for monitoring through satellite remote sensing, in alignment with Committee on Earth Observation Satellites (CEOS) agencies. Space agencies currently distribute a variety of datasets on the snow cover derived from remote sensing observations. CEOS maintains a list of operational snow products accompanied with their spatial and temporal coverage, spatial and temporal resolution and a link to validation information (CEOS, 2023). Some of them are already widely used for scientific studies and operational water resource management (Awasthi and Varade, 2021). Operational products currently belong mostly either to the category of optical remote sensing of the snow cover extent or to snow water equivalent products generated by assimilating in situ snow measurements with passive microwave satellite. Snow-covered area products can be either binary (snow cover absence or presence), or fractional (provides the fraction of a pixel which is covered by snow). Binary snow cover and fractional snow cover products are the only snow products to have reached validation stage 2 (CEOS, 2023). In particular, the collection of MODIS/VIIRS snow products distributed by the NASA since the early 2000’s (Hall et al., 2002) offers the best tradeoff in terms of coverage (global), revisit (daily), resolution (500 m) and accessibility (open data policy) for mountain snow studies.
Other available operational products include snow-covered area from low resolution optical and microwave sensors aboard geostationary and polar-orbiting meteorological satellites (GOES, MSG, MetOp), wet/dry snow derived from passive microwave sensors (AMSR-E, SSM/I), snow albedo and grain size from MODIS, and high resolution snow-covered area from Landsat 8/9 and/or Sentinel-2 over specific regions (United States, Europe). However, as detailed below, this portfolio of products remains insufficient in the specific context of the mountain regions for three main reasons: 1) lack of snow water equivalent observations, 2) lack of systematic and regular high resolution observations and 3) lack of long term observations.
1. Operational satellite remote sensing approaches to retrieve the snow water equivalent (SWE) in mountain terrain at high spatial resolution are still lacking (Dozier et al., 2016; Tsang et al., 2022). This constitutes a key shortcoming as an accurate knowledge of SWE spatial distribution is essential to predict streamflow in snow dominated mountain catchments (Freudiger et al., 2017). A possible approach to reconstruct high resolution SWE over large mountain regions relies on the assimilation of snow-covered area products in a snowpack model (Girotto et al., 2020; Liu et al., 2021). This approach enables to generate accurate snow reanalyses once the snow season is over, but is less suitable to retrieve SWE distribution in near real time. Direct methods based on active microwave remote sensing are being developed since the 2000’s but more research is still needed to reach the user needs (Guneriussen et al., 2001; Tsang et al., 2022). Acknowledging the lack of an operational concept or sensor to measure the spatial distribution of SWE from space, NASA developed the airborne snow observatory using lidar to fulfill the need to estimate water resources in the western United States (Painter et al., 2016). Yet, lidar does not measure snow water equivalent but snow depth, so it can only fulfill this need in conjunction with modeled snow density.
2. Near-daily observations of the snow-covered area are available but their spatial resolution can be too coarse for a range of studies in mountain hydrology and ecology, or even operational needs (Malnes et al., 2015). This is because the snow cover variability has a typical length scale on the order of 100 m in mountain regions (Blöschl, 1999). In particular, the widely-used MODIS snow products have a resolution of approximately 500 m which can fail to capture the spatial heterogeneity of snow cover induced by solar radiation in steep terrain (Bouamri et al., 2021). A high spatial resolution (∼10 m) is also useful for ecologists to characterize habitats of mountain plants and animals (Dedieu et al., 2016; Alba et al., 2023; Niffenegger et al., 2023). Whereas a remotely sensed SWE is the holy grail of snow hydrology, ecologists have shown that the snow cover duration or melt out date are important predictors of alpine plants productivity, distribution and diversity (Galen and Stanton, 1995; Jonas et al., 2008; Carlson et al., 2015; Choler, 2015; Revuelto et al., 2022). These indicators can be easily derived from existing remote sensing technology, but the challenge is to get the information at high spatial and temporal resolutions to capture the snow cover phenology at the relevant scales and with sufficient precision.
3. Long term snow cover observations are necessary to characterize the impact of climate change on land-atmosphere feedbacks, water resources and ecosystems especially in mountain regions where the warming trend often exceeds the global average (Pepin et al., 2022). Existing long term (>30 years) remote sensing datasets are an invaluable source to study global or regional snow cover changes in the context of climate change (Hüsler et al., 2014) but may be inadequate in some cases due to their coarse resolution (Bormann et al., 2018). The MODIS dataset is increasingly used to determine trends in snow cover extent and duration (Saavedra et al., 2018; Notarnicola, 2020; Shi et al., 2022). Yet, its relatively short duration (23 years) makes it difficult to separate a long term trend from natural climate variability (Bormann et al., 2018; Fugazza et al., 2021). This is because snow cover changes not only reflect temperature changes but also precipitation variations which may be subject to multidecadal climatic oscillations (Monteiro and Morin, 2023; Gottlieb and Mankin, 2024).
The snow remote sensing community is actively working to adapt existing algorithms to new spaceborne sensors or to develop algorithms to retrieve new variables from space. A broad review of the remote sensing techniques for estimating the snow geophysical properties in mountain regions is already available (Awasthi and Varade, 2021), updating a previous review on a similar scope (Nolin, 2010). More specifically, a review of fundamental advances towards the global monitoring of SWE using high-frequency radar remote sensing has been published recently (Tsang et al., 2022). Complementary reviews of snow cover remote sensing methods using spaceborne synthetic aperture radar (SAR) (Tsai et al., 2019) or optical sensors (Dumont and Gascoin, 2016) are also available. New sensing concepts are supported by space agencies and may lead to solve the above issues in the long term (after 2030). A mission dedicated to snow depth and snow water equivalent, including high spatial resolution in mountain areas was listed as a priority for a new program element in the 2017 Decadal Survey and the Canadian Space Agency is developing the Terrestrial Snow Mass Mission for the same objective (Derksen et al., 2019; L. Wang et al., 2022). Here, we focus on recent advances that have the potential to specifically address the above three issues in the short term. First, we argue that progress can be made by applying existing algorithms to recent Earth Observation missions. Second, we highlight recent methodological progresses which have the potential to go beyond the current status. Then, we summarize current challenges to be addressed to go beyond the current status. We conclude by outlining a series of recommendations targeted at international organizations and space agencies. These recommendations are based on the consultation of a multi-national community of experts at the Mountain Snow Workshop held by the World Meteorological Organization and EUMETSAT in Darmstadt, Germany in 2023.
The Copernicus Earth Observation programme offers the opportunity to monitor the snow cover at high resolution and global scale. Sentinel-1 and Sentinel-2 observations now span nearly a decade. The datasets are freely distributed and tools are available to process them. Following a method developed for ERS-1 data (Rott and Nagler, 1995; Nagler and Rott, 2000), wet snow can be detected from Sentinel-1 C-band backscatter (Nagler et al., 2016; Tsai et al., 2019). Similarly, early algorithms to map the snow-covered area developed for Landsat TM (Dozier, 1989) have been extended to Sentinel-2 multispectral imagery (Wayand et al., 2018; Gascoin et al., 2019). Both methods benefit from the enhanced spatial resolution and revisit times of Sentinel-1 and Sentinel-2 with respect to previous missions, allowing the development of a new generation of snow products at European scale (European Environment Agency, 2021). The combination of Sentinel-1 wet snow-covered area and Sentinel-2 snow-covered area would increase the number of observations to estimate the area and duration of the snow cover at decametric resolution (Karbou et al., 2021).
Similarly, spectral unmixing methods developed for MODIS are being adapted to Landsat 8/9 and Sentinel-2 (Aalstad et al., 2020; Bair et al., 2020; Stillinger et al., 2023). Keuris et al. (2023) proposed a method for estimation fractional snow extent exploiting the full spectral capabilities of moderns satellite sensors such as Sentinel-2, Landsat-7/8/9 and Sentinel-3 SLSTR and OLCI, applying multispectral unmixing with local adaptive endmember selection and accounting for the high variable solar illumination in mountainous areas. These approaches take advantage of all available spectral information to retrieve fractional snow cover and other properties such as snow albedo, grain size or the presence of light absorbing particles (Nolin et al., 1993; Painter et al., 2009).
In recent years, several methods were applied to retrieve snow depth from space. A mountain snow depth map obtained from satellite data only was generated by differencing digital elevation models from Pléiades stereoscopic imagery (Marti et al., 2016). The estimated random error was 0.6 m at a spatial resolution of 2 m. This method was refined and further evaluated against airborne lidar measurements showing that the accuracy of this method increases down to 0.3 m at 100 m resolution (Deschamps-Berger et al., 2020). It is limited by the swath width of Pléaides (or WorldView), which typically allows imaging a region of 400–1,000 km2 in a single pass depending on the acquisition geometry, but it is a viable alternative to airborne campaigns (Eberhard et al., 2021). A conceptual method was proposed to retrieve mountain snow depth at northern hemisphere scale from Sentinel-1 polarimetric backscatter observations with a change detection technique (Lievens et al., 2019). Using a single global calibration factor this method yielded a mean absolute error of ∼ 0.3 m at 1 km resolution, where regional calibration enabled improved performance (Lievens et al., 2022). With the 6–12 days revisit of Sentinel-1, this approach could provide useful frequent snow depth data. It is not applicable during the melt season when the radar signal is absorbed by the liquid water contained in the snowpack, and retrieval performance is reduced in regions with shallow or intermittent snow, and with dense vegetation. More recently, ICESat-2 land surface elevation retrievals were successfully used to retrieve snow depth in the Sierra Nevada (Deschamps-Berger et al., 2023). The method is not yet applicable at global scale as it requires an accurate snow-off digital terrain model. This is because ICESat-2 orbit is not repeated outside polar regions. Such reference elevation model could be obtained from VHR stereo imagery in areas where lidar surveys are not available. Last, machine/deep learning algorithms have also been successfully trained to infer snow depth in the Alps, either from AMSR-E at 10 km resolution (Santi et al., 2014) or from Sentinel-1/2 at 10 m resolution (Daudt et al., 2023). These methods rely on in situ or airborne data for model training, which prevents their application to other regions.
Machine learning algorithms are also increasingly used to detect the snow cover in high resolution satellite images, especially those devoid of shortwave infrared bands. This includes very high resolution imagery from commercial satellites operated by Maxar (Hu and Shean, 2022), Planet (Yang et al., 2023) or Venµs (Baba et al., 2020). These sensors offer the opportunity to map the snow cover at metric resolution, even in steep alpine terrain. Convolutional neural networks are efficient to perform the snow and cloud object segmentation as they account for the pixel neighborhood in addition to the pixel-wise spectral information (Lu et al., 2022). This approach is especially useful to extract the snow-covered area from historical satellite images with poor radiometric resolution and in the absence of a shortwave infrared band such as SPOT 1-4 and Landsat 1-4 (Barrou Dumont et al., 2023).
Methods to retrieve snow depth and snow-covered area can be used in combination with empirical snow density models to estimate catchment scale SWE. Error on snow density are rather low if the snow depth is known (Avanzi and De Michele, 2015). Jonas et al. (2009) reported an error of ±45 kg.m-3 (±1 standard deviation) on snow density using in situ snow depth data as predictor. In addition, land surface temperature monitoring open new research avenues on this topic. Indeed, the snow density could be estimated from Landsat with an RMSE of 82 kg m−3 (Colombo et al., 2023). A machine-learning approach enabled to estimate snow density from multiple MODIS and reanalyses datasets with an RMSE of 43 kg m−3 (H. Wang et al., 2023). However, as we argue below, a more optimal method to convert snow depth to SWE is through the assimilation in a snowpack model.
The above satellite methods provide incomplete information due to orbital constraints, sensor geometry, cloud cover and applicability domain of the retrieval algorithm. Indeed, most approaches do not work well in all snow climate and/or environmental conditions (e.g., deep vs. shallow snowpack). Microwave approaches are generally more uncertain in case of wet snow or dense forest cover. Data assimilation enables to merge different types of satellite observations accounting for the retrieval uncertainty to generate physically consistent, spatially and temporally continuous datasets of the snow cover properties, including SWE, snow depth, etc. (Girotto et al., 2020; Largeron et al., 2020). Previous studies have shown the value of data assimilation for water resources studies (Margulis et al., 2015), operational near real time applications (Cluzet et al., 2021), climate reanalysis (Hersbach et al., 2020), numerical weather prediction (de Rosnay et al., 2015, 2022), and seasonal prediction (Orsolini et al., 2019). The recent release of the Multiple Snow data Assimilation System (MuSA) (Alonso-González et al., 2022b) should facilitate the development of data assimilation experiments and the generation of snow datasets in mountain regions taking advantage of various remote sensing products. Data assimilation studies would also benefit from radiative transfer models like the Snow Microwave Radiative Transfer model (SMRT), which enables to compute backscattering and brightness temperature in active and passive mode from multilayered snowpack (Picard et al., 2018). SMRT enables to test the assimilation of low level satellite measurements (e.g., radiance) as it is typically done in numerical weather prediction.
As briefly discussed above, the availability of petabytes of satellite data enables the observation of multiple snow variables with ever-increasing temporal and spatial precision at the scale of entire mountain ranges (Lievens et al., 2019; Liu et al., 2021). However, to fully harness the wealth of satellites, there remain challenges in accessing, processing and interpreting results from large datasets. This is especially the case with the Sentinel-1, Sentinel-2 and Landsat datasets, but it will be the case with upcoming missions like NISAR. Snow scientists increasingly rely on commercial cloud geoprocessing platforms such as Google Earth Engine and Microsoft Planetary Computer to process remote sensing datasets (Crumley et al., 2020; Notarnicola, 2020; Gascoin et al., 2022; Gagliano et al., 2023). High performance and cloud computing is also becoming critical to perform intensive computations for machine and deep learning and ensemble-based data assimilation.
In addition to the computing resources issue, the scarcity of snow-specific data assimilation software has hindered the adoption of data assimilation in snow science. Data assimilation algorithms can be complex and sometimes require advanced knowledge in applied mathematics. Several well-documented open source snow models with different levels of complexity are now available, but, in contrast, there is only one open source snow model including data assimilation tools to our best knowledge (Alonso-González et al., 2022a). The open-source NASA Land Information System software also embeds land surface models including snow modules and data assimilation code (Kumar et al., 2006). Another key challenge that should be addressed to facilitate data assimilation is the quantification of remote sensing data accuracy in various conditions, because a good knowledge of the observation error is needed to balance the weight of the observations in the model analysis.
From the numerical weather prediction and climate reanalysis perspectives, the main challenges are (i) the availability and sustainability of relevant operational satellites sensitive to snow properties, (ii) the complexity of the radiative transfer processes that link snow properties to the signal that the satellite measures. Radiative transfer models are required to optimally use satellite observations to analyze snow.
Despite several decades of research in optical remote sensing of the snow cover, there remain some challenges. Cloud cover hinders our capacity to observe basic variables such as snow cover. This issue is particularly pronounced in key regions like the Eastern Himalaya and the Pacific Northwest of North America. Moreover, the confusion between clouds and snow cover in optical images classification is an issue that is often underestimated (Stillinger et al., 2019). Evaluation studies have typically focused on clear-sky images whereas cloud cover can mask over 50% of observations in temperate mountain ranges (Rittger et al., 2013; Masson et al., 2018). The issue of snow-cloud discrimination is also largely unaddressed in studies using commercial very high resolution imagery, which prevents their more systematic use on a large scale. Even a very accurate snow detection algorithm is of limited utility if the associated cloud and cloud shadow detection algorithm is too conservative and masks most of the snow pixels. Another important challenge is that optical methods are very uncertain in forested areas (Xin et al., 2012; Muhuri et al., 2021). This is also true for microwave methods. As a result, our ability to characterize the snow-covered area, snow depth or snow albedo from remote sensing is very limited in forested area.
Although this is a long standing issue in remote sensing, there often remains a scale gap between remote sensing retrievals, in situ measurements and model outputs (Blöschl, 1999). This can lead to systematic differences between these quantities. For example, the intrinsic snow albedo that can be measured in situ or simulated by snowpack models differs from the apparent (or effective) albedo retrieved from space due to snow surface microtopography (Bair et al., 2022). The scale mismatch with in situ measurements similarly impedes the evaluation of many remote sensing products like snow depth and SWE.
After the workshop, the experts agreed on a list of recommendations to go beyond the current status in mountain snow remote sensing. This document is provided in Supplementary Appendix. We highlight and develop here the key points to address the three key issues that were highlighted in the introduction (#1. lack of representative snow water equivalent observations, #2. lack of systematic and regular high resolution observations and #3. lack of long term observations).
First, we consider that a range of satellite snow products and methods are already available to go beyond the status quo in the short term. Global scale, high resolution (10–30 m) monitoring of the snow-covered area can be achieved using Sentinel-2 and Landsat-8/9 observations with a revisit lower than 5 days (cloud permitting). Although snow and cloud flags are already included in standard level 2 Sentinel-2 and Landsat products distributed by ESA and USGS, they can be improved using existing algorithms tailored to snow cover mapping. Open source software is available to generate fractional snow cover maps at continental scale from Sentinel-2 and is already operated in Europe (European Environment Agency, 2020). In addition, Sentinel-1 data are now used by the same agency to increase the effective observation frequency of the snow-covered area when the snowpack contains liquid water. Similarly, the USGS distributes Landsat fractional snow cover products over the United States. Such datasets if available globally would enable to address key issue #2, enabling many applications in mountain ecology.
Secondly, while there is currently no operational method to retrieve mountain SWE from space, we argue that the SWE question (key issue #1) can be tackled by the assimilation of multiple remote sensing observations in a distributed snowpack model. Recent advances in snow depth remote sensing with Sentinel-1, ICESat-2 are promising as the snow depth is the main driver of SWE variability. More systematic repeat-track ICESat-2 campaigns over non-polar, snow-covered mountain regions would enable to retrieve snow depth from ICESat-2 data only (Besso et al., 2024). This would be especially useful to better estimate snow depth in forested areas thanks to the unique ability of ICESat-2 to measure surface elevation below the forest canopy, where other optical and microwave sensors provide limited information. In the meantime, merging satellite snow depth from Sentinel-1 and snow-covered area from Sentinel-2 should be tested. While Sentinel-1 snow depth algorithm is not applicable during the melt season, Sentinel-2 provides frequent observations of the snow-covered area during spring and summer, an additional constraint to estimate SWE (Figure 2). Further research is required to take full advantage of those observations. In particular it is essential to characterize their uncertainty, as it is a key input for data assimilation. To go forward in this topic, we need accurate benchmarking datasets in pilot sites. An outstanding example is the Tuolumne river basin in California Sierra Nevada, where snow depth is frequently monitored by airborne lidar since 2012, including very dry and very wet years. Several instrumented sites are available in other mountain ranges. A recent compilation of research catchments is available from the International Network for Alpine Research Catchment Hydrology (INARCH) website (Pomeroy et al., 2015). However, each site includes different types of observations with varying spatio-temporal resolutions, etc. Assimilating these observations into processed-based snow models would allow assembling benchmarking datasets that are physically consistent and complete in space and time. The development of such benchmarking datasets would help maximize the usage of in situ observations for the evaluation of remote sensing products. Regarding the assimilation method itself, previous studies recommend the use of ensemble approaches (in contrast with variational approaches) because they make it possible to use off-the-shelf nonlinear snowpack models, without the need to implement an adjoint model (Helmert et al., 2018; Largeron et al., 2020; Alonso-González et al., 2022b). We note however that this status may change soon in the future with the adoption of automatic differentiation tools in the Earth science modeling community.
Figure 2. Schematic representation of how various remote sensing data can be used to estimate the snow water equivalent in mountain regions by data assimilation in a snowpack model. Remote sensing observations and model forcing come with errors (top diagram) that are reflected in the posterior model uncertainties (bottom diagram). The blue lines in the bottom diagram represent the bounds of uncertainty of the simulated SWE.
Third, data assimilation is also a way to address the lack of long term observations (key issue #3), as it allows merging multiple discontinuous satellite time series and different variables of interest. In this case, the batch smoothing approach is well suited (in contrast with a filtering algorithm) since it assimilates simultaneously all available observations over a time window so that late snow season observations are used to update the early snow season parameters distribution and reciprocally (Durand et al., 2008). Again, this requires a good knowledge of the data uncertainties especially if the objective is to study trends due to climate change. A detailed intercomparison of global to northern hemispheric daily snow extent products is being carried out in the Satellite Snow Product Intercomparison and Evaluation Exercise (SnowPEx and SnowPEx+). A national program led by China National Satellite Meteorological Center called RetrospectIve Calibration of Historical Chinese Earth Observation Satellite data (RICH-CEOS) aims to cross-calibrate historical datasets from multiple satellites operated by China. The multispectral data provided by HJ-1A and HJ-1B missions should be of particular interest for the snow community due to their high spatial resolution (30–150 m) and 4 days revisit period. To facilitate the fusion of multiple remote sensing datasets, we encourage data providers to adopt standardized data and meta-data formats and we recommend to provide uncertainty estimates on a pixel-by-pixel basis. Machine-learning products should also include their uncertainty and domain of applicability.
Finally, we expect that upcoming Earth observation missions will create new opportunities to improve our knowledge of the mountain snow. L-band SAR missions (ROSE-L, NISAR, ALOS-4) should open the door to InSAR retrieval of the SWE changes in complex terrain (Tarricone et al., 2023). Thermal infrared missions (TRISHNA, LSTM, SBG) will allow the assimilation of near daily surface temperature to improve SWE simulations (Alonso-González et al., 2023). These missions will also allow the retrieval of snow albedo at finer resolution than the current products from Sentinel-3 or VIIRS thanks to their multispectral and multiangle imaging capabilities. To foster the development of new algorithms and prepare future missions, we recommend a better coordination of on-demand satellite data acquisitions (e.g., very high resolution SAR and optical stereoscopic images) over pilot mountain sites by space agencies and commercial providers following the example of NASA SnowEx campaigns.
SG: Visualization, Writing–original draft. KL: Conceptualization, Writing–review and editing. TN: Writing–review and editing. HL: Writing–review and editing. MM: Writing–review and editing. TJ: Writing–review and editing. ZZ: Writing–review and editing. PD: Writing–review and editing.
The author(s) declare that no financial support was received for the research, authorship, and/or publication of this article.
Many experts contributed to the WMO-EUMETSAT workshop on which this paper is based. The authors would like to thank Adnan Shafiq Rana, Charles Fierz, Freddy Saavedra, Rijan Bhakta Kayastha, Shawn Marshall, Sonam Lotas, Suhaib Bin Farhan, Wolfgang Schöner, Zuhal Akyurek, Kenneth Holmlund, Lijuan Ma, Tao Che, Samuel Buisan, Marie Dumont, Zhaojun Zheng, Colleen Mortimer, Sean Helfrich, Jeff Key, Sean Helfrich. The authors greatly thank Rodica Nitu and the Global Cryosphere Watch (WMO) for coordinating this event and her help to realize this publication. The authors also would like to thank Ran Zhang for the organization of the workshop. Figure 2 was inspired by a similar graphic created by Jessica Lundquist for the SnowEx campaign.
Authors KL and HL were employed by Snowcap BV, a start-up based on scientific research at KU Leuven. Author TN was employed by ENVEO Environmental Earth Observation IT GmbH.
The remaining authors declare that the research was conducted in the absence of any commercial or financial relationships that could be construed as a potential conflict of interest.
All claims expressed in this article are solely those of the authors and do not necessarily represent those of their affiliated organizations, or those of the publisher, the editors and the reviewers. Any product that may be evaluated in this article, or claim that may be made by its manufacturer, is not guaranteed or endorsed by the publisher.
The Supplementary Material for this article can be found online at: https://www.frontiersin.org/articles/10.3389/feart.2024.1381323/full#supplementary-material
Aalstad, K., Westermann, S., and Bertino, L. (2020). Evaluating satellite retrieved fractional snow-covered area at a high-Arctic site using terrestrial photography. Remote Sens. Environ. 239, 111618. doi:10.1016/j.rse.2019.111618
Alba, R., Oddi, L., Rosselli, D., and Chamberlain, D. (2023). Avalanches create unique habitats for birds in the European Alps. J. Ornithol. 164 (2), 377–388. doi:10.1007/s10336-022-02039-3
Alonso-González, E., Aalstad, K., Baba, M. W., Revuelto, J., López-Moreno, J. I., Fiddes, J., et al. (2022a). MuSA: the multiscale snow data assimilation system (v1.0). Geosci. Model Dev. Discuss., 1–43. doi:10.5194/gmd-2022-137
Alonso-González, E., Aalstad, K., Baba, M. W., Revuelto, J., López-Moreno, J. I., Fiddes, J., et al. (2022b). The multiple snow data assimilation system (MuSA v1.0). Geosci. Model Dev. 15 (24), 9127–9155. doi:10.5194/gmd-15-9127-2022
Alonso-González, E., Gascoin, S., Arioli, S., and Picard, G. (2023). Exploring the potential of thermal infrared remote sensing to improve a snowpack model through an observing system simulation experiment. Cryosphere 17 (8), 3329–3342. doi:10.5194/tc-17-3329-2023
Avanzi, F., and De Michele, C. (2015). On the performances of empirical regressions for the estimation of bulk snow density. Geografia Fisica e Dinamica Quaternaria, 105–112. doi:10.4461/GFDQ.2015.38.10
Awasthi, S., and Varade, D. (2021). Recent advances in the remote sensing of alpine snow: a review. GIScience Remote Sens. 58, 852–888. doi:10.1080/15481603.2021.1946938
Baba, M. W., Gascoin, S., Hagolle, O., Bourgeois, E., Desjardins, C., and Dedieu, G. (2020). Evaluation of methods for mapping the snow cover area at high spatio-temporal resolution with VENμS. Remote Sens. 12 (18), 3058. doi:10.3390/rs12183058
Bair, E. H., Dozier, J., Stern, C., LeWinter, A., Rittger, K., Savagian, A., et al. (2022). Divergence of apparent and intrinsic snow albedo over a season at a sub-alpine site with implications for remote sensing. Cryosphere 16 (5), 1765–1778. doi:10.5194/tc-16-1765-2022
Bair, E. H., Stillinger, T., and Dozier, J. (2020). Snow property inversion from remote sensing (SPIReS): a generalized multispectral unmixing approach with examples from MODIS and landsat 8 oli. IEEE Trans. Geoscience Remote Sens. 59, 7270–7284. doi:10.1109/TGRS.2020.3040328
Barrou Dumont, Z. B., Gascoin, S., and Inglada, J. (2024). Snow and cloud classification in historical SPOT images: an image emulation approach for training a deep learning model without reference data. IEEE Journal of Selected Topics in Applied Earth Observations and Remote Sensing, 1–13. doi:10.1109/JSTARS.2024.3361838
Besso, H., Shean, D., and Lundquist, J. D. (2024). Mountain snow depth retrievals from customized processing of ICESat-2 satellite laser altimetry. Remote Sens. Environ. 300, 113843. doi:10.1016/j.rse.2023.113843
Blöschl, G. (1999). Scaling issues in snow hydrology. Hydrol. Process. 13 (14–15), 2149–2175. doi:10.1002/(sici)1099-1085(199910)13:14/15<2149::aid-hyp847>3.0.co;2-8
Bormann, K. J., Brown, R. D., Derksen, C., and Painter, T. H. (2018). Estimating snow-cover trends from space. Nat. Clim. Change 8 (11), 924–928. doi:10.1038/s41558-018-0318-3
Bouamri, H., Kinnard, C., Boudhar, A., Gascoin, S., Hanich, L., and Chehbouni, A. (2021). MODIS does not capture the spatial heterogeneity of snow cover induced by solar radiation. Front. Earth Sci. 9. doi:10.3389/feart.2021.640250
Carlson, B. Z., Choler, P., Renaud, J., Dedieu, J.-P., and Thuiller, W. (2015). Modelling snow cover duration improves predictions of functional and taxonomic diversity for alpine plant communities. Ann. Bot. 116 (6), 1023–1034. doi:10.1093/aob/mcv041
CEOS Land product validation subgroup. (2023). Available at: https://lpvs.gsfc.nasa.gov/producers2.php?topic=snow.
Choler, P. (2015). Growth response of temperate mountain grasslands to inter-annual variations in snow cover duration. Biogeosciences 12 (12), 3885–3897. doi:10.5194/bg-12-3885-2015
Cluzet, B., Lafaysse, M., Cosme, E., Albergel, C., Meunier, L.-F., and Dumont, M. (2021). CrocO_v1.0: a particle filter to assimilate snowpack observations in a spatialised framework. Geosci. Model Dev. 14 (3), 1595–1614. doi:10.5194/gmd-14-1595-2021
Colombo, R., Pennati, G., Pozzi, G., Garzonio, R., Di Mauro, B., Giardino, C., et al. (2023). Mapping snow density through thermal inertia observations. Remote Sensing of Environment 284, 113323. doi:10.1016/j.rse.2022.113323
Crumley, R. L., Palomaki, R. T., Nolin, A. W., Sproles, E. A., and Mar, E. J. (2020). SnowCloudMetrics: snow information for everyone. Remote Sens. 12 (20), 3341. doi:10.3390/rs12203341
Daudt, R. C., Wulf, H., Hafner, E. D., Bühler, Y., Schindler, K., and Wegner, J. D. (2023). Snow depth estimation at country-scale with high spatial and temporal resolution. ISPRS J. Photogrammetry Remote Sens. 197, 105–121. doi:10.1016/j.isprsjprs.2023.01.017
Dedieu, J.-P., Carlson, B. Z., Bigot, S., Sirguey, P., Vionnet, V., and Choler, P. (2016). On the importance of high-resolution time series of optical imagery for quantifying the effects of snow cover duration on alpine plant habitat. Remote Sens. 8 (6), 481. doi:10.3390/rs8060481
Derksen, C., Lemmetyinen, J., King, J., Belair, S., Garnaud, C., Lapointe, M., et al. (2019). A dual-frequency ku-band radar mission concept for seasonal snow. IGARSS 2019 - 2019 IEEE Int. Geoscience Remote Sens. Symposium, 5742–5744. doi:10.1109/IGARSS.2019.8898030
de Rosnay, P., Browne, P., de Boisséson, E., Fairbairn, D., Hirahara, Y., Ochi, K., et al. (2022). Coupled data assimilation at ECMWF: current status, challenges and future developments. Q. J. R. Meteorological Soc. 148 (747), 2672–2702. doi:10.1002/qj.4330
de Rosnay, P., Isaksen, L., and Dahoui, M. (2015). Snow data assimilation at ECMWF. Meteorology. doi:10.21957/LKPXQ6X5
Deschamps-Berger, C., Gascoin, S., Berthier, E., Deems, J., Gutmann, E., Dehecq, A., et al. (2020). Snow depth mapping from stereo satellite imagery in mountainous terrain: evaluation using airborne laser-scanning data. Cryosphere 14 (9), 2925–2940. doi:10.5194/tc-14-2925-2020
Deschamps-Berger, C., Gascoin, S., Shean, D., Besso, H., Guiot, A., and López-Moreno, J. I. (2023). Evaluation of snow depth retrievals from ICESat-2 using airborne laser-scanning data. Cryosphere 17 (7), 2779–2792. doi:10.5194/tc-17-2779-2023
Dong, C. (2018). Remote sensing, hydrological modeling and in situ observations in snow cover research: a review. J. Hydrology 561, 573–583. doi:10.1016/j.jhydrol.2018.04.027
Dozier, J. (1989). Spectral signature of alpine snow cover from the landsat thematic mapper. Remote Sens. Environ. 28, 9–22. doi:10.1016/0034-4257(89)90101-6
Dozier, J., Bair, E. H., and Davis, R. E. (2016). Estimating the spatial distribution of snow water equivalent in the world’s mountains. Wiley Interdiscip. Rev. Water 3, 461–474. doi:10.1002/wat2.1140
Dumont, M., and Gascoin, S. (2016). Optical remote sensing of snow cover. Land Surf. Remote Sens. Cont. Hydrology, 115–137. doi:10.1016/B978-1-78548-104-8.50004-8
Durand, M., Molotch, N. P., and Margulis, S. A. (2008). Merging complementary remote sensing datasets in the context of snow water equivalent reconstruction. Remote Sens. Environ. 112 (3), 1212–1225. doi:10.1016/j.rse.2007.08.010
Eberhard, L. A., Sirguey, P., Miller, A., Marty, M., Schindler, K., Stoffel, A., et al. (2021). Intercomparison of photogrammetric platforms for spatially continuous snow depth mapping. Cryosphere 15 (1), 69–94. doi:10.5194/tc-15-69-2021
European Environment Agency (2020). Fractional snow cover (raster 20m) 2016-present, Europe, daily, jul. 2020 (01.00) [GeoTIFF]. Eur. Environ. Agency. doi:10.2909/3E2B4B7B-A460-41DD-A373-962D032795F3
European Environment Agency (2021). High resolution snow and ice. EEA Geospatial Data Cat. Available at: https://sdi.eea.europa.eu/catalogue/srv/api/records/45e60a9f-f08b-47a9-97b6-21805cf22940.
Fayad, A., Gascoin, S., Faour, G., López-Moreno, J. I., Drapeau, L., Page, M. L., et al. (2017). Snow hydrology in Mediterranean mountain regions: a review. J. Hydrology 551, 374–396. doi:10.1016/j.jhydrol.2017.05.063
Freudiger, D., Kohn, I., Seibert, J., Stahl, K., and Weiler, M. (2017). Snow redistribution for the hydrological modeling of alpine catchments: snow redistribution for hydrological modeling. Wiley Interdiscip. Rev. Water 4 (5), e1232. doi:10.1002/wat2.1232
Fugazza, D., Manara, V., Senese, A., Diolaiuti, G., and Maugeri, M. (2021). Snow cover variability in the greater alpine region in the MODIS era (2000–2019). Remote Sens. 13 (15), 2945. doi:10.3390/rs13152945
Gagliano, E., Shean, D., Henderson, S., and Vanderwilt, S. (2023). Capturing the onset of mountain snowmelt runoff using satellite synthetic aperture radar. Geophys. Res. Lett. 50 (21), e2023GL105303. doi:10.1029/2023GL105303
Galen, C., and Stanton, M. L. (1995). Responses of snowbed plant species to changes in growing-season length. Ecology 76 (5), 1546–1557. doi:10.2307/1938156
Gascoin, S., Grizonnet, M., Bouchet, M., Salgues, G., and Hagolle, O. (2019). Theia Snow collection: high-resolution operational snow cover maps from Sentinel-2 and Landsat-8 data. Earth Syst. Sci. Data 11 (2), 493–514. doi:10.5194/essd-11-493-2019
Gascoin, S., Monteiro, D., and Morin, S. (2022). Reanalysis-based contextualization of real-time snow cover monitoring from space. Environ. Res. Lett. 17 (11), 114044. doi:10.1088/1748-9326/ac9e6a
Girotto, M., Musselman, K. N., and Essery, R. L. H. (2020). Data assimilation improves estimates of climate-sensitive seasonal snow. Curr. Clim. Change Rep. 6, 81–94. doi:10.1007/s40641-020-00159-7
Gottlieb, A. R., and Mankin, J. S. (2024). Evidence of human influence on Northern Hemisphere snow loss. Nature 625 (7994), 293–300. doi:10.1038/s41586-023-06794-y
Guneriussen, T., Hogda, K. A., Johnsen, H., and Lauknes, I. (2001). InSAR for estimation of changes in snow water equivalent of dry snow. IEEE Trans. Geoscience Remote Sens. 39 (10), 2101–2108. doi:10.1109/36.957273
Hall, D. K., and Riggs, G. A. (2021). MODIS/Terra snow cover daily L3 global 0.05Deg CMG, version 61. NASA Natl. Snow Ice Data Cent. Distributed Act. Archive Cent. doi:10.5067/MODIS/MOD10C1.061
Hall, D. K., Riggs, G. A., Salomonson, V. V., DiGirolamo, N. E., and Bayr, K. J. (2002). MODIS snow-cover products. Remote Sens. Environ. 83 (1–2), 181–194. doi:10.1016/S0034-4257(02)00095-0
Helmert, J., Şensoy Şorman, A., Alvarado Montero, R., De Michele, C., De Rosnay, P., Dumont, M., et al. (2018). Review of snow data assimilation methods for hydrological, land surface, meteorological and climate models: results from a COST HarmoSnow survey. Geosciences 8 (12), 489. doi:10.3390/geosciences8120489
Hersbach, H., Bell, B., Berrisford, P., Hirahara, S., Horányi, A., Muñoz-Sabater, J., et al. (2020). The ERA5 global reanalysis. Q. J. R. Meteorological Soc. 146 (730), 1999–2049. doi:10.1002/qj.3803
Hu, J. M., and Shean, D. (2022). Improving Mountain snow and land cover mapping using very-high-resolution (VHR) optical satellite images and random forest machine learning models. Remote Sens. 14 (17), 4227. doi:10.3390/rs14174227
Hüsler, F., Jonas, T., Riffler, M., Musial, J. P., and Wunderle, S. (2014). A satellite-based snow cover climatology (1985–2011) for the European Alps derived from AVHRR data. Cryosphere 8 (1), 73–90. doi:10.5194/tc-8-73-2014
Intergovernmental Panel on Climate Change (IPCC) (2022). “High Mountain areas,” in The Ocean and Cryosphere in a changing climate: special report of the intergovernmental Panel on climate change (Cambridge University Press), 131–202. doi:10.1017/9781009157964.004
Jonas, T., Marty, C., and Magnusson, J. (2009). Estimating the snow water equivalent from snow depth measurements in the Swiss Alps. Journal of Hydrology 378, 161–167. doi:10.1016/j.jhydrol.2009.09.021
Jonas, T., Rixen, C., Sturm, M., and Stoeckli, V. (2008). How alpine plant growth is linked to snow cover and climate variability. J. Geophys. Res. 113 (G3), G03013. doi:10.1029/2007JG000680
Karbou, F., Veyssière, G., Coleou, C., Dufour, A., Gouttevin, I., Durand, P., et al. (2021). Monitoring wet snow over an alpine region using sentinel-1 observations. Remote Sens. 13 (3), 381. doi:10.3390/rs13030381
Keuris, L., Hetzenecker, M., Nagler, T., Mölg, N., and Schwaizer, G. (2023). An adaptive method for the estimation of snow-covered fraction with error propagation for applications from local to global scales. Remote Sensing 15, 1231. doi:10.3390/rs15051231
Kumar, S., Peterslidard, C., Tian, Y., Houser, P., Geiger, J., Olden, S., et al. (2006). Land information system: an interoperable framework for high resolution land surface modeling. Environ. Model. Softw. 21 (10), 1402–1415. doi:10.1016/j.envsoft.2005.07.004
Largeron, C., Dumont, M., Morin, S., Boone, A., Lafaysse, M., Metref, S., et al. (2020). Toward snow cover estimation in mountainous areas using modern data assimilation methods: a review. Front. Earth Sci. 8. doi:10.3389/feart.2020.00325
Lievens, H., Brangers, I., Marshall, H.-P., Jonas, T., Olefs, M., and De Lannoy, G. (2022). Sentinel-1 snow depth retrieval at sub-kilometer resolution over the European Alps. Cryosphere 16 (1), 159–177. doi:10.5194/tc-16-159-2022
Lievens, H., Demuzere, M., Marshall, H.-P., Reichle, R. H., Brucker, L., Brangers, I., et al. (2019). Snow depth variability in the Northern Hemisphere mountains observed from space. Nat. Commun. 10 (1), 4629. doi:10.1038/s41467-019-12566-y
Liu, Y., Fang, Y., and Margulis, S. A. (2021). Spatiotemporal distribution of seasonal snow water equivalent in High Mountain Asia from an 18-year Landsat–MODIS era snow reanalysis dataset. Cryosphere 15 (11), 5261–5280. doi:10.5194/tc-15-5261-2021
Lu, Y., James, T., Schillaci, C., and Lipani, A. (2022). Snow detection in alpine regions with Convolutional Neural Networks: discriminating snow from cold clouds and water body. GIScience Remote Sens. 59 (1), 1321–1343. doi:10.1080/15481603.2022.2112391
Malnes, E., Buanes, A., Nagler, T., Bippus, G., Gustafsson, D., Schiller, C., et al. (2015). User requirements for the snow and land ice services – CryoLand. Cryosphere 9 (3), 1191–1202. doi:10.5194/tc-9-1191-2015
Mankin, J. S., Viviroli, D., Singh, D., Hoekstra, A. Y., and Diffenbaugh, N. S. (2015). The potential for snow to supply human water demand in the present and future. Environ. Res. Lett. 10 (11), 114016. doi:10.1088/1748-9326/10/11/114016
Margulis, S. A., Girotto, M., Cortés, G., and Durand, M. (2015). A particle batch smoother approach to snow water equivalent estimation. J. Hydrometeorol. 16 (4), 1752–1772. doi:10.1175/JHM-D-14-0177.1
Marti, R., Gascoin, S., Berthier, E., de Pinel, M., Houet, T., and Laffly, D. (2016). Mapping snow depth in open alpine terrain from stereo satellite imagery. Cryosphere 10 (4), 1361–1380. doi:10.5194/tc-10-1361-2016
Masson, T., Dumont, M., Mura, M. D., Sirguey, P., Gascoin, S., Dedieu, J.-P., et al. (2018). An assessment of existing methodologies to retrieve snow cover fraction from MODIS data. Remote Sens. 10 (4), 619. doi:10.3390/rs10040619
Monteiro, D., and Morin, S. (2023). Multi-decadal analysis of past winter temperature, precipitation and snow cover data in the European Alps from reanalyses, climate models and observational datasets. Cryosphere 17 (8), 3617–3660. doi:10.5194/tc-17-3617-2023
Muhuri, A., Gascoin, S., Menzel, L., Kostadinov, T. S., Harpold, A. A., Sanmiguel-Vallelado, A., et al. (2021). Performance assessment of optical satellite-based operational snow cover monitoring algorithms in forested landscapes. IEEE J. Sel. Top. Appl. Earth Observations Remote Sens. 14, 7159–7178. doi:10.1109/JSTARS.2021.3089655
Nagler, T., and Rott, H. (2000). Retrieval of wet snow by means of multitemporal SAR data. IEEE Trans. Geoscience Remote Sens. 38 (2), 754–765. doi:10.1109/36.842004
Nagler, T., Rott, H., Ripper, E., Bippus, G., and Hetzenecker, M. (2016). Advancements for snowmelt monitoring by means of sentinel-1 SAR. Remote Sens. 8 (4), 348. doi:10.3390/rs8040348
National Academies of Sciences, Engineering, and Medicine (2018). Thriving on our changing planet: a decadal strategy for Earth observation from space. National Academies Press. doi:10.17226/24938
Niffenegger, C. A., Schano, C., Arlettaz, R., and Korner-Nievergelt, F. (2023). Nest orientation and proximity to snow patches are important for nest site selection of a cavity breeder at high elevation. J. Avian Biol. 2023 (3–4), e03046. doi:10.1111/jav.03046
Nolin, A. W. (2010). Recent advances in remote sensing of seasonal snow. J. Glaciol. 56 (200), 1141–1150. doi:10.3189/002214311796406077
Nolin, A. W., Dozier, J., and Mertes, L. A. K. (1993). Mapping alpine snow using a spectral mixture modeling technique. Ann. Glaciol. 17, 121–124. doi:10.3189/S0260305500012702
Notarnicola, C. (2020). Hotspots of snow cover changes in global mountain regions over 2000–2018. Remote Sens. Environ. 243, 111781. doi:10.1016/j.rse.2020.111781
Orsolini, Y., Wegmann, M., Dutra, E., Liu, B., Balsamo, G., Yang, K., et al. (2019). Evaluation of snow depth and snow cover over the Tibetan Plateau in global reanalyses using in situ and satellite remote sensing observations. Cryosphere 13 (8), 2221–2239. doi:10.5194/tc-13-2221-2019
Painter, T. H., Berisford, D. F., Boardman, J. W., Bormann, K. J., Deems, J. S., Gehrke, F., et al. (2016). The Airborne Snow Observatory: fusion of scanning lidar, imaging spectrometer, and physically-based modeling for mapping snow water equivalent and snow albedo. Remote Sens. Environ. 184, 139–152. doi:10.1016/j.rse.2016.06.018
Painter, T. H., Rittger, K., McKenzie, C., Slaughter, P., Davis, R. E., and Dozier, J. (2009). Retrieval of subpixel snow covered area, grain size, and albedo from MODIS. Remote Sens. Environ. 113 (4), 868–879. doi:10.1016/j.rse.2009.01.001
Pepin, N. C., Arnone, E., Gobiet, A., Haslinger, K., Kotlarski, S., Notarnicola, C., et al. (2022). Climate changes and their elevational patterns in the mountains of the world. Rev. Geophys. 60 (1), e2020RG000730. doi:10.1029/2020RG000730
Picard, G., Sandells, M., and Löwe, H. (2018). SMRT: an active–passive microwave radiative transfer model for snow with multiple microstructure and scattering formulations (v1.0). Geosci. Model Dev. 11 (7), 2763–2788. doi:10.5194/gmd-11-2763-2018
Pomeroy, J., Bernhardt, M., and Marks, D. (2015). Research network to track alpine water. Nature 521 (7550), 32. doi:10.1038/521032c
Qin, Y., Abatzoglou, J. T., Siebert, S., Huning, L. S., AghaKouchak, A., Mankin, J. S., et al. (2020). Agricultural risks from changing snowmelt. Nat. Clim. Change 10, 459–465. doi:10.1038/s41558-020-0746-8
Revuelto, J., Gómez, D., Alonso-González, E., Vidaller, I., Rojas-Heredia, F., Deschamps-Berger, C., et al. (2022). Intermediate snowpack melt-out dates guarantee the highest seasonal grasslands greening in the Pyrenees. Sci. Rep. 12 (1), 18328. doi:10.1038/s41598-022-22391-x
Rittger, K., Painter, T. H., and Dozier, J. (2013). Assessment of methods for mapping snow cover from MODIS. Adv. Water Resour. 51, 367–380. doi:10.1016/j.advwatres.2012.03.002
Rott, H., and Nagler, T. (1995). Monitoring temporal dynamics of snowmelt with ERS-1 SAR. 3, 1747–1749.
Saavedra, F. A., Kampf, S. K., Fassnacht, S. R., and Sibold, J. S. (2018). Changes in Andes snow cover from MODIS data, 2000–2016. Cryosphere 12 (3), 1027–1046. doi:10.5194/tc-12-1027-2018
Santi, E., Pettinato, S., Paloscia, S., Pampaloni, P., Fontanelli, G., Crepaz, A., et al. (2014). Monitoring of Alpine snow using satellite radiometers and artificial neural networks. Remote Sens. Environ. 144, 179–186. doi:10.1016/j.rse.2014.01.012
Shi, M., Yuan, Z., Hong, X., and Liu, S. (2022). Spatiotemporal variation of snow cover and its response to climate change in the source region of the yangtze river, China. Atmosphere 13 (8), 1161. doi:10.3390/atmos13081161
Stillinger, T., Rittger, K., Raleigh, M. S., Michell, A., Davis, R. E., and Bair, E. H. (2023). Landsat, MODIS, and VIIRS snow cover mapping algorithm performance as validated by airborne lidar datasets. Cryosphere 17 (2), 567–590. doi:10.5194/tc-17-567-2023
Stillinger, T., Roberts, D. A., Collar, N. M., and Dozier, J. (2019). Cloud masking for landsat 8 and MODIS terra over snow-covered terrain: error analysis and spectral similarity between snow and cloud. Water Resour. Res. 55 (7), 6169–6184. doi:10.1029/2019WR024932
Sturm, M., Goldstein, M. A., and Parr, C. (2017). Water and life from snow: a trillion dollar science question. Water Resour. Res. 53 (5), 3534–3544. doi:10.1002/2017WR020840
Tarricone, J., Webb, R. W., Marshall, H.-P., Nolin, A. W., and Meyer, F. J. (2023). Estimating snow accumulation and ablation with L-band interferometric synthetic aperture radar (InSAR). Cryosphere 17 (5), 1997–2019. doi:10.5194/tc-17-1997-2023
Tsai, Y.-L. S., Dietz, A., Oppelt, N., and Kuenzer, C. (2019). Remote sensing of snow cover using spaceborne SAR: a review. Remote Sens. 11 (12), 1456. doi:10.3390/rs11121456
Tsang, L., Durand, M., Derksen, C., Barros, A. P., Kang, D.-H., Lievens, H., et al. (2022). Review article: global monitoring of snow water equivalent using high-frequency radar remote sensing. Cryosphere 16 (9), 3531–3573. doi:10.5194/tc-16-3531-2022
Viviroli, D., Kummu, M., Meybeck, M., Kallio, M., and Wada, Y. (2020). Increasing dependence of lowland populations on mountain water resources. Nat. Sustain. 3, 917–928. doi:10.1038/s41893-020-0559-9
Wang, H., Zhang, X., Xiao, P., Che, T., Zheng, Z., Dai, L., et al. (2023). Towards large-scale daily snow density mapping with spatiotemporally aware model and multi-source data. Cryosphere 17 (1), 33–50. doi:10.5194/tc-17-33-2023
Wang, L., Forman, B. A., and Kim, E. (2022). Exploring the spatiotemporal coverage of terrestrial snow mass using a suite of satellite constellation configurations. Remote Sens. 14 (3), 633. doi:10.3390/rs14030633
Wayand, N. E., Marsh, C. B., Shea, J. M., and Pomeroy, J. W. (2018). Globally scalable alpine snow metrics. Remote Sens. Environ. 213, 61–72. doi:10.1016/j.rse.2018.05.012
Xin, Q., Woodcock, C. E., Liu, J., Tan, B., Melloh, R. A., and Davis, R. E. (2012). View angle effects on MODIS snow mapping in forests. Remote Sens. Environ. 118, 50–59. doi:10.1016/j.rse.2011.10.029
Keywords: snow, remote sensing, mountain, review, snow water equivalent, snow cover, snow hydrology, mountain ecology
Citation: Gascoin S, Luojus K, Nagler T, Lievens H, Masiokas M, Jonas T, Zheng Z and De Rosnay P (2024) Remote sensing of mountain snow from space: status and recommendations. Front. Earth Sci. 12:1381323. doi: 10.3389/feart.2024.1381323
Received: 03 February 2024; Accepted: 23 April 2024;
Published: 10 May 2024.
Edited by:
Alexander Kokhanovsky, German Research Centre for Geosciences, GermanyReviewed by:
Haokui Xu, University of Michigan, United StatesCopyright © 2024 Gascoin, Luojus, Nagler, Lievens, Masiokas, Jonas, Zheng and De Rosnay. This is an open-access article distributed under the terms of the Creative Commons Attribution License (CC BY). The use, distribution or reproduction in other forums is permitted, provided the original author(s) and the copyright owner(s) are credited and that the original publication in this journal is cited, in accordance with accepted academic practice. No use, distribution or reproduction is permitted which does not comply with these terms.
*Correspondence: Simon Gascoin, c2ltb24uZ2FzY29pbkB1bml2LXRsc2UzLmZy
Disclaimer: All claims expressed in this article are solely those of the authors and do not necessarily represent those of their affiliated organizations, or those of the publisher, the editors and the reviewers. Any product that may be evaluated in this article or claim that may be made by its manufacturer is not guaranteed or endorsed by the publisher.
Research integrity at Frontiers
Learn more about the work of our research integrity team to safeguard the quality of each article we publish.