- 1China Construction Civil Construction Co., Ltd., Beijing, China
- 2School of Intelligent Transportation and Engineering, Guangzhou Maritime University, Guangzhou, China
- 3School of Intelligent Transportation and Engineering, Guangzhou Jiaotong University, Guangzhou, China
- 4School of Integrated of Circuits, Guangdong University of Technology, Guangzhou, China
Introduction: The study proposes a method for evaluating stress distribution in high-altitude Tibetan Plateau railway tunnels using high-precision radar satellite time-series interferometric synthetic aperture radar technology.
Methods: To effectively monitor and prevent geological hazards during the construction process, this method i employed, as it serves as a component of advanced geological prediction and surrounding rock deformation monitoring technology for high-altitude tunnels, particularly in the Dongelu Tunnel of the China–Tibet Railway. The study utilizes time-series interferometric synthetic aperture radar to obtain deformation information for Dongelu Tunnel area between 2022 and 2023 from Sentinel- 1A orbit images. This quantitatively investigates the upper mountain body and line-of-sight direction along the tunnel. The deformation characteristics are correlated with high-frequency and high-precision automated vertical displacement monitoring results, determining the spatiotemporal distribution of tunnel deformation. In this study, a model that determines the vertical stress state of the Dongelu Tunnel under loading near the entrance and evaluates its health status was established.
Results: The results show that the surface deformation of the mountain above the tunnel axis develops slowly and is relatively small, with a maximum vertical deformation rate of 1–3 mm/year. The average stress on the tunnel arch is 5.54 MPa, with a fluctuation range of 0.01 MPa. Temporal Q9 changes in various parts of the tunnel are periodic, with maximum fluctuations observed in December 2022. The study reveals inconsistent surface settlement of the tunnel arch and mountain above it, causing minor vertical stress changes. As the tunnel construction progresses, vertical stress variation shows periodicity because of an initial imbalance in internal stress within the mountain. Stress fluctuations near the tunnel entrance occur during the initial excavation phase, gradually diminishing as the project progresses and internal stress stabilizes.
Discussion: The proposed tunnel monitoring and stability assessment method can reduce its impact on engineering construction and provide guidance for advanced geological prediction.
1 Introduction
The range of economic activities gradually expands with population increase, and railway transportation—which makes up the majority of land transportation—has made a substantial and lasting contribution to the advancement of human development. All countries have begun building the plateau railway to develop the economy, consolidate national defense, promote ethnic unity, and economic development in the plateau area, considering it as a strategic artery for national defense security (Jianbing et al., 2020). The engineering construction is considerably more difficult than the usual railway engineering because of the unique and complicated geological structure of the plateau area. In particular, geological disasters must be adequately monitored and avoided. The railway area experiences mixed formation lithology, new tectonic movement, deep activity faults, and strong earthquakes. It also experiences large-magnitude collapses, landslides, debris flow, and other giant geological bodies, as well as special rock and soil development.
During the engineering design stage, numerous research works on the geological elements that influence the route direction scheme are conducted. Geological line selection is a set of concepts and principles that are proposed for specific geological issues that include active faults and high ground temperatures. Nevertheless, despite the presence of several geological difficulties, current research methods employed to perform qualitative and quantitative analyses of safety and stability are few. In terms of regional security stability, there are two types of stability: the stability of the internal dynamic crust, which primarily pertains to new tectonic movement, and the stability of the rock, soil, and mountain slopes under the influence of internal and external forces, which primarily manifest as ground collapse and mountain disasters (Zhang et al., 2016). Thus, more stringent guidelines for the sophisticated geological prediction of tunnels must be developed, the impact associated with these geological calamities on engineering development should be minimized, and problems must be monitored and regulated effectively. One of the most efficient approaches to geological catastrophe mitigation and prevention is early detection. However, identifying landslides in complicated terrain regions, particularly the buried landslides in the plateau railway building area situated in the valley and mountains, in a timely manner has been challenging (Zhang et al., 2024a; Fang et al., 2024). Landslides, being the most prevalent type of geological hazard, are widely distributed, frequently occur, and pose notable threats to human life and property safety (Zhang et al., 2024b).
Interferometric synthetic aperture radar (InSAR) technology has recently been widely employed for high-precision monitoring of slope deformations, which is critical for analyzing dangers including landslides above high-altitude railway tunnels (De-hong, 2019; Xinghong et al., 2020). The permanent scatterer InSAR and small baseline subset synthetic aperture radar (SBAS-InSAR) techniques mainly have been utilized to mitigate the atmospheric effects and temporal and spatial decorrelation inherent in traditional interferometric measurement methods, as well as to overcome the limitations of temporal decorrelation in the early detection of landslides. These developments provide precise and continuous land deformation results in space and time, which is a dependable method for enhancing the accuracy of landslide identification and monitoring. The permanent scatterer synthetic aperture radar and SBAS-InSAR technologies have been widely applied to early identification of landslide hazards and have yielded successful outcomes in some present studies (Sun et al., 2016; Daqing et al., 2019; Keren et al., 2019; Dai et al., 2020). Chaoying et al. (2019) used InSAR technology to carry out a multitemporal cataloging, long-term monitoring, and instability mode recognition investigation of possible loess landslides in the Heifang area of Gansu Province. The excellent dependability of landslides detected by InSAR technology was confirmed by field studies. Huiyan et al. (2019) used high-resolution optical remote sensing image visual interpretation and SBAS-InSAR technology to identify landslide hazards within a 30-km upstream and 100-km downstream range of the Jinsha River Basin, a critical area for Sichuan–Tibet Railway. Using SBAS-InSAR technology, Hong et al. (2021) investigated the subsidence rates and sequences in VV polarization and VH polarization in the Liaoning Province encompassing regions of Panjin, Yingkou, Haicheng, and Anshan. Moreover, they examined and forecasted the subsidence along the passenger lines of Panjin-Yingkou and Ha-Da Railway, which traverse the research area.
Through the advantages of wide-area identification, InSAR technology has been widely validated for effective early identification of construction areas in plateau regions, particularly landslide hazards (Zhao et al., 2012). However, problems with data processing, including decorrelation and shadowing, could result in omitted or inaccurate results. Sentinel-1 (an ascending orbit) and RADARSAT-2 (a descending orbit) data combined in a complimentary fashion can effectively solve the issue of landslide dangers along Lancang River section of Sichuan–Tibet Railway (Shi et al., 2016). Using the SBAS-InSAR technique, Jiajia et al. (2021) combined data in a complimentary way from the descending orbit RADARSAT-2 satellite and the ascending orbit Sentinel-1 satellite. In the Lancang River section of the Qinghai–Tibet Railway, this method successfully avoids shadow overlap areas and allows for the early identification of landslide hazards. To demonstrate the specific geometric distortion areas and the applicability of Sentinel-1 ascending and descending orbit data in high-mountain canyon areas, Keren et al. (2020) employed time-series InSAR technology to conduct broad-area early identification of landslide hazards in Ya-Luo River Basin from Ya County to Muli County.
To solve the concerns raised above, this work focuses on improved geological prediction and rock deformation monitoring technologies for plateau tunnels. For this study’s deformation monitoring research using InSAR technology, Dongelu Tunnel on China–Tibet Railway was observed and analyzed. To assess the stability of the tunnel’s physical condition, the stress condition of the tunnel was forecasted. This approach, which uses time-series InSAR technology, can give some assistance in assessing stress distribution in high-altitude railway tunnels.
2 Engineering background and the data sources
2.1 Research area
Dongelu Tunnel is located on the southern side of the transition from the Sichuan Basin to the Qinghai–Tibet Plateau. The external force is predominantly caused by freezing and thawing, which is accompanied by biological weathering and other influences that are characteristic of plateau landforms. The elevation of the research area spans between 3,468 and 3,802 m, with a relative elevation difference of 334 m and a generally natural slope of 30°–40°. A dirt road leads approximately 520 m south of the tunnel entrance from National Highway 318, with no traffic access; the tunnel exit is located in East Village, Xinduqiao Town, with a cement village road leading about 240 m north of the tunnel exit, with general traffic conditions. The length of the tunnel is 1,163 m. Figure 1 shows the distribution diagram of the landform and railway tunnel.
The research region is located in Hengduan Mountain range, at a high altitude. The complicated topography has altered the latitude climatic evolution sequence, which resulted in a unique plateau continental monsoon climate. Subtropical climatic traits are only seen in valleys with low heights. The high-altitude plateau area includes Xinduqiao area, which is situated at an elevation of more than 3,000 m. This area’s climate is classified as high altitude. The environment is characterized by a chilly temperature, a lengthy frost-free season, and plenty of sunlight. Zheduo Mountain—located in the heart of Daxueshan series, which the main mountain range in the research region—splits the climate in the Xinduqiao area into two sections. The southeast monsoon brings warm, muggy, wet, and concentrated weather to the eastern area. Summer is hot and muggy, whereas winter is frigid. There are four distinct seasons throughout the frost-free period, which vary depending on altitude. Western Zheduo Mountain experiences a year-round climate with plenty of sunshine; low temperatures; long, dry winters; and cool, breezy summers due to the influence of the southwest monsoon (Changyan et al., 2005). The tunnel is situated in western Zheduo Mountain. The yearly average temperature of Xinduqiao region is 5.2°C, with typical temperatures of 3.6°C in January and 12.8°C in July. The extreme maximum temperature is 32.7°C, and the extreme lowest temperature is 8.2°C, based on the data from Xinduqiao weather station, which is located at an altitude of 3,460 m. There is a 922.7-mm annual average rainfall, a height of 146.1 mm per day, 761.7-mm annual average evaporation, 61% relative humidity, 2,525.9 h of sunlight, and 95 days without frost. The schematic layout of the site building in front of the tunnel entrance is depicted in Figure 2.
2.2 Data source
2.2.1 Synthetic aperture radar (SAR) satellite data
Sentinel1 is made up of two European Space Agency–launched and European Space Agency–operated Earth observation satellites that carry C-band SAR with a wavelength of approximately 5.6 cm and a 12-day revisit time. Terrain observation by progressive scanning (TOPS) offers a horizontal single-field coverage of up to 250 km × 170 m, enabling the provision of high-resolution radar data in all weather conditions and during the day. Progressive scanning (TOPS) can offer high-resolution radar satellite data with all-weather, all-day coverage up to 250 km × 170 m. Sentine-1 satellite has been widely utilized in the field of geohazards since it offers the most convenient open SAR data gathering, the greatest coverage area, and the best time resolution in the world (Yafeng et al., 1999; Tomas et al., 2014; Dong et al., 2018).
Herein, the real topography of the mountain above the tunnel, along with the quantitative analysis of the appropriateness of the tunnel axial range in the research region, should be effectively completed. The unique criteria for estimating the slope direction of the mountain in raised orbit data (typically north–south and eastward tendency is appropriate for elevated orbit data) was considered. The present research examines 21-view IW-mode IAR images that were obtained over the course of a year, from November 2022 to June 2023, using SAR images with resolutions of 5 and 20 m, from the Sentinel-1 satellite in an elevated orbit. Figure 3 displays the coverage of the image data. WorldDEM grid interval of 30-m digital elevation model data is used for the topographic and geomorphic feature characteristics. The positioning accuracy of the POD precision orbit data, which is released after 21 days of imaging, might exceed 5 cm. SBAS-InSAR then processes the data. As the experimental area-specific parameters are shown in Table 1 following 21 days of imaging, the elevated track data can fully cover the longitudinal and protibial 10-km range along the tunnel area. The positioning accuracy can reach 5 cm, and SBAS-InSAR is then used to process the data. Table 1 displays the particular specifications of the experimental region that the lifting rail data may cover, which includes the whole longitudinal 10-km range along the tunnel area.
2.2.2 Tunnel internal monitoring data
To deduce the spatial and temporal distribution of tunnel deformation and quantitatively study the correlation between internal and external deformation of the tunnel, the internal monitoring data of the tunnel are mainly the results of high-frequency and high-precision total station automated vertical displacement monitoring at the same time period as InSAR monitoring. The data were measured by China Construction Civil Engineering & Architecture Co., Ltd., in the field; the data collection time was 2022.11–2023.6, and the sampling frequency was two times/day. This study recorded the temperature changes during each observation at the same time as the automated observation of deformation, and the format of its raw data is shown in Table 2. The large temperature difference between day and night in the Tibetan Plateau region is likely to be one of the driving factors for the stress changes inside the tunnel, as shown in the schematic diagram of the automated monitoring inside the tunnel in Figure 4. The raw data should be resampled for the correlation qualitative study’s subsequent phase to synchronize it with the temporal sampling of the external monitoring data.
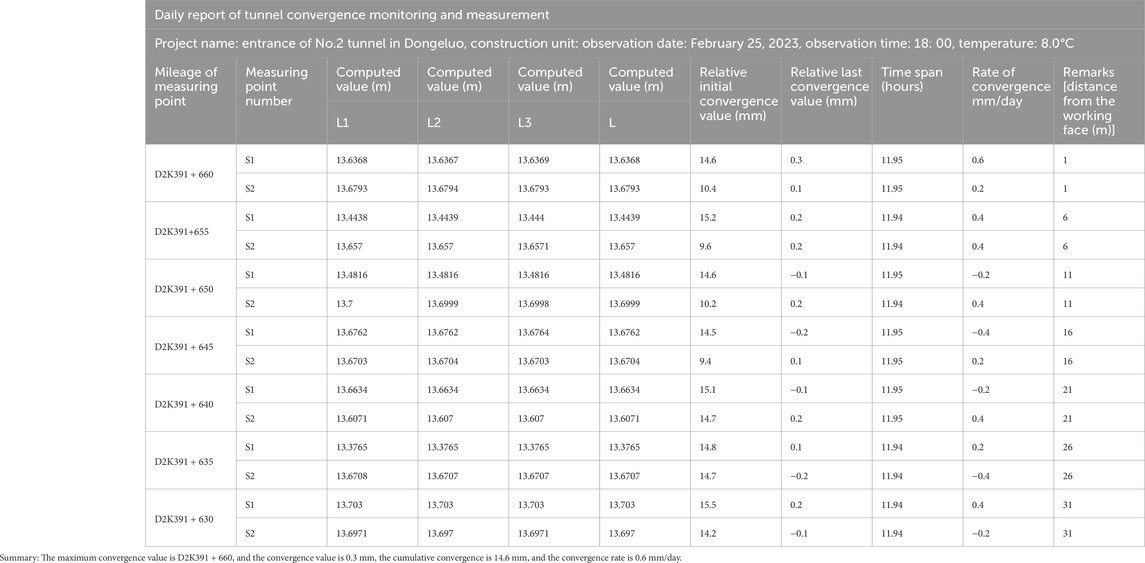
Table 2. Example of raw data for vertical displacement measurement inside the tunnel (observation data at 6:00 on January 14, 2022).
2.2.3 SBAS-InSAR
The SBAS-InSAR technology used in this study was proposed by Berardino et al. (2002). The fundamental idea underlying this technique is to obtain all SAR images and combine the results into a small baseline set, such that the image baseline space within each subset is small. Subsequently, using the least squares method of the images in each subset could compute the surface deformation of the time series in each subset (Nestor et al., 2016; Guitang et al., 2020). The linear deformation model is constructed using a combination of interferograms based on the high coherence sites to acquire the linear deformation rate and elevation error information. Next, the non-linear deformation information is obtained by decomposing the residual error. The specific algorithm is described as follows: After obtaining N + 1 SAR images of the same area, one of the images was selected as the reference image and underwent registration, resampling, leveling, and topographic phase. The M interference images were obtained based on a suitable spatial–temporal baseline threshold, and each interference pair had to satisfy the inequality
Herein,
The reference image is baseline estimated, screened, registered, and resampled with all pictures simultaneously throughout the data processing phase. Subsequently, the remaining phase is eliminated by orbit refining and releveling after the differential interference map and unwrapping map are acquired via decapping, interference processing, and phase unwinding. Further, using the high coherence linear deformation model inversion to obtain linear deformation rate and elevation error information, a 4:1 ratio, a resolution of 15 m, a filter window of 32, and a coherence threshold of 0.3 were selected. The residual error is then used to decompose the non-linear deformation information, and the surface temporal deformation information is obtained using the SVD method, as shown in Figure 5.
Figure 6 shows the reference images that were selected and which date back to April 1, 2023. To invert the initial average deformation rate of high coherence sites and the residual height correction coefficient, the first inversion employs the SVD approach using minimal baseline data and a linear model. To estimate the atmospheric interference phase components, the second inversion employs a first-order linear model. The atmospheric filter eradicates atmospheric interference phase components by using a time high-pass filter and a space low-pass filter. Finally, the sight deformation rate and time-series deformation accumulation are used simultaneously with radar incident data. Eq. 2 represents the angle of sight to the deformation to the vertical deformation. This time-series differential interferogram was analyzed using SBAS-InSAR, as shown in Figure 7. The time-series coherence coefficient that was produced during processing is shown in Figure 8.
In Eq. (2), dLos is the line-of-sight to the deformation results, dv is the vertical direction deformation result, and θ is the radar incident angle, respectively.
2.3 Calculation of tunnel vertical pressure under load
The vertical pressure under the load can be calculated according to formula (3) (Dong et al., 2017; Liu et al., 2018; Meng et al., 2019; Zhenlin et al., 2019), and Figure 9 shows the schematic of boron action (load) of bias tunnel lining.
where
Among them, α: Ground slope Angle
It is also assumed that the bias distribution pattern is consistent with the ground slope.
3 Results and analysis
3.1 Precision validation
The correlation between the spatial monitoring results derived from the SAR data of rising and lowering rail is computed to confirm the validity of the InSAR monitoring findings presented in the present research. As shown in Figure 10, the correlation coefficient is 0.72, and the two monitoring results are highly correlated, which indicates the accuracy of the two methods. However, the majority of points are concentrated, approximately 5–5 mm apart, and a few are spread, which might be due to a different selection procedure for connecting diagrams and geodetic control point, resulting in differing deformation rate estimates. Overall, the cross-validation of SBAS-InSAR monitoring data demonstrated that InSAR monitoring results were reliable in the present study.
3.2 Analysis of mountain deformation of the upper part of the tunnel
3.2.1 Unitary analysis
The vertical surface deformation monitoring data of the mountain surface above the East Aurora 2 Tunnel from October 2022 to May 2023 were obtained using SBAS-InSAR technology based on 21 ascending rail Sentinel-1A images (Figure 11). The mountain surface that covers the tunnel has a maximum vertical settlement rate of approximately 26 mm/year and a maximum vertical uplift rate of 22 mm/year.
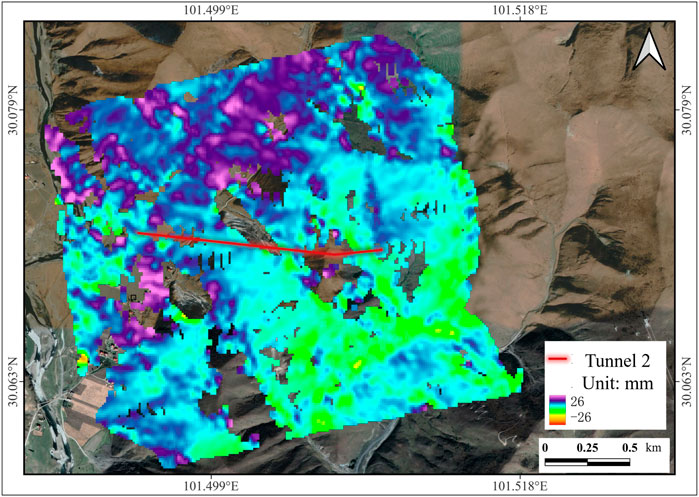
Figure 11. Schematic diagram of the sedimentation rate monitoring of 2022.102–2023.5 InSAR in the study area (mm/year).
The statistical curves for the northwest and southeast directions of the mountain near the tunnel have peaks of approximately −1 mm and −3 mm, respectively, based on the statistical histogram of the four directional variables of the tunnel in Figure 14. According to the InSAR timing, the tunnel southeast of the mountain is expected to settle faster than the northwest by 1–2 mm/year. This phenomenon can be attributed to the fact that the southeast inlet of the tunnel was constructed before the northwest exit tunnel, which indicates that although the total settlement is within a safe range, the cumulative settlement will be higher than the northwest.
3.2.2 Analysis of characteristic point deformation of tunnel axis orientation
Figure 12A displays the cumulative settlement field of the deformation monitoring of the tunnel axis–covered region, as shown by the analysis of the tunnel axis direction feature points 9, and Figure 12B displays the time series of the elevation measurement change at the exit of the axis direction. The time-series deformation trend chart of the nine typical characteristic points selected directly above the tunnel axis is displayed in Figure 12C. The overall result exhibits an obvious seasonal cycle variation, with the uplift range being larger in autumn and winter than in the summer. The seasonal shift brought on by frost is the potential origin of the aforementioned explanation, and the total yearly change is a modest upward tendency.
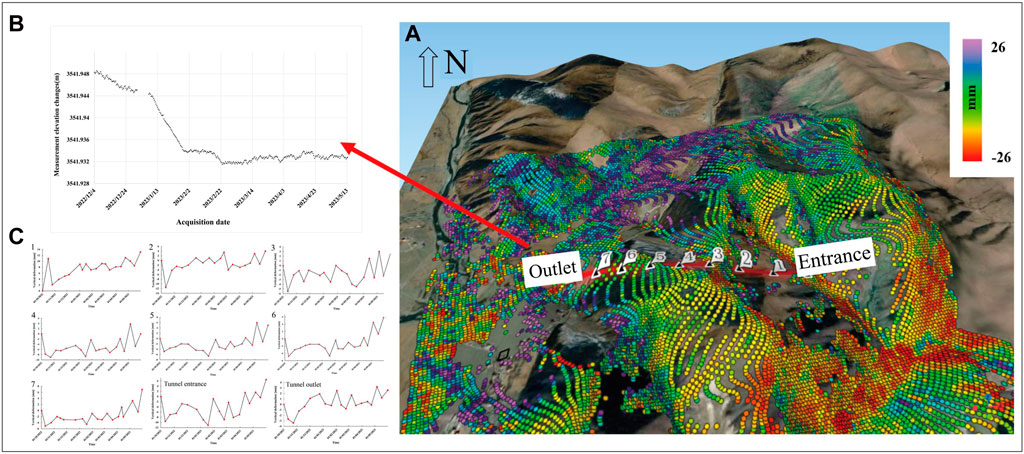
Figure 12. (A) displays the cumulative settlement field of the deformation monitoring of the tunnel axis–covered region, as shown by the analysis of the tunnel axis direction feature points 9, and (B) displays the time series ofthe elevation measurement change at the exit of the axis direction. The time-series deformation trend chart of the nine typical characteristic points selected directly above the tunnel axis is displayed in (C). The overall result exhibits an obvious seasonal cycle variation, with the uplift range being larger in autumn and winter than in the summer. The seasonal shift brought on by frost is the potential origin of the aforementioned explanation, and the total yearly change is a modest upward tendency.
From the tunnel exit section analysis diagram, before 2023, overall deformation changes, because the construction is causing mountain changes. In the middle of February, it began to slowly stabilize and presented small amplitude seasonal cycle changes. Figure 13 displays the tunnel exit D2K391 + 675 mileage pile section deformation analysis diagram. Figure 13A shows the tunnel mountain elevation diagram. Figure 13B presents the section of 11 monitoring point elevation time sequence change trend diagram.
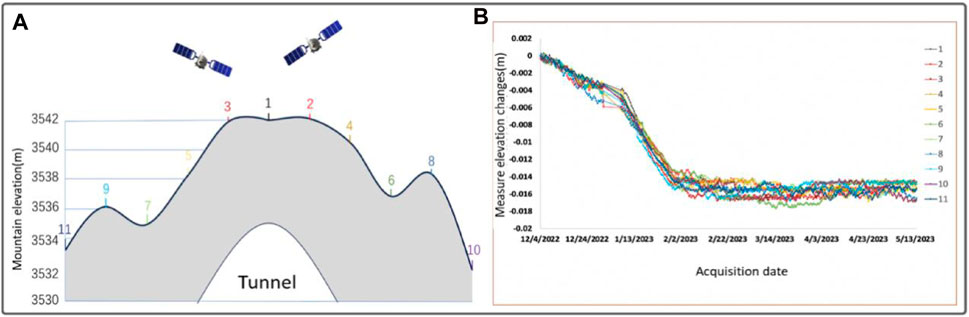
Figure 13. Analysis of typical tunnel section. (A) the tunnel mountain elevation diagram and (B) the section of 11 monitoring point elevation time sequence change trend diagram.
4 Tunnel stress status assessment
Herein, we examined the vertical stress time series (Figure 14) from October 2022 to May 2023 using the interior tunnel parameters that China Construction Group provided (Table 4. The vertical stress on the top of the tunnel can be attributed to the mountain gravity generated by the top of the tunnel. The settlement value of the tunnel vault differs from that of the mountain surface directly above it, suggesting that the tunnel activities are causing periodic variations in the vertical stress of the vault. The vault stress induced by the uneven settlement of the mountain and the tunnel varies slightly, with an average stress of 5.54 MPa (553.5 kN/cm2) and a variation range of 0.01 MPa on the mountain directly above the tunnel.
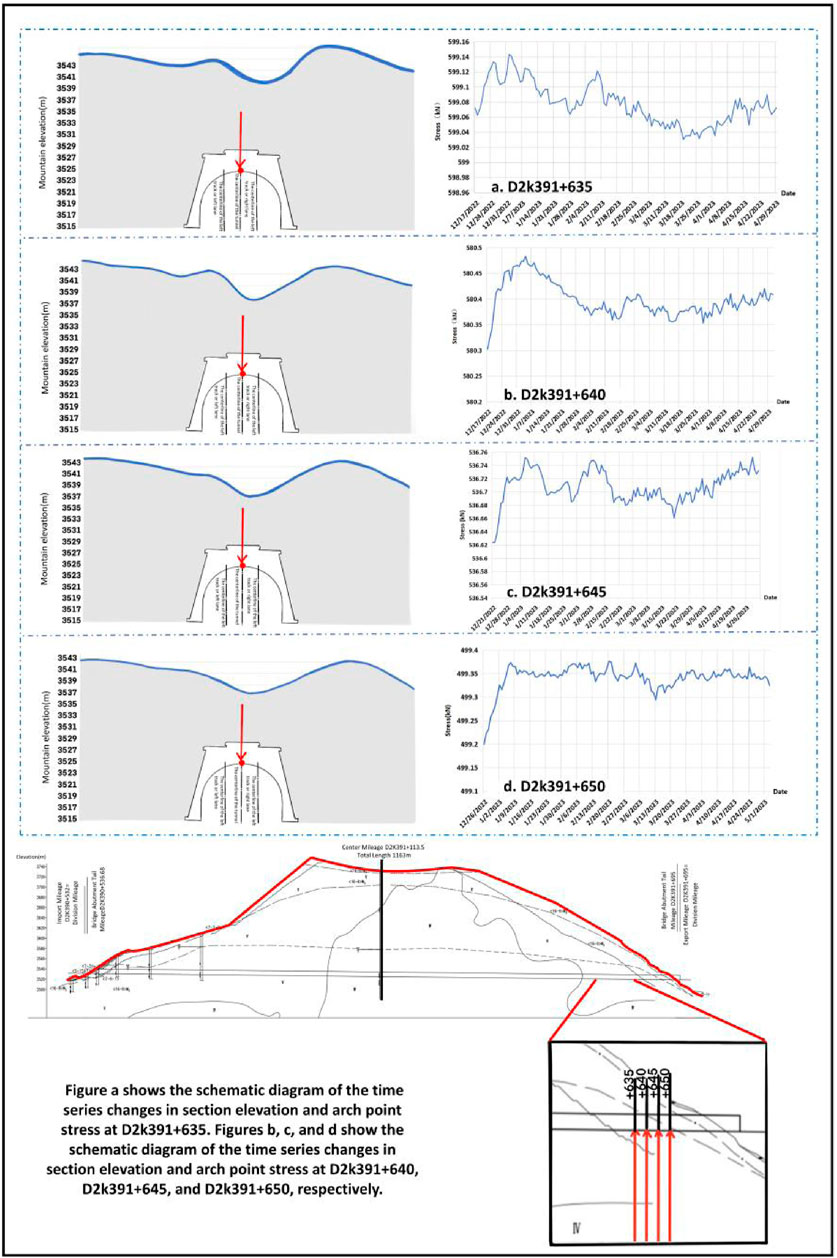
Figure 14. Schematic of the time-series analysis of the changes in section elevation and arch point stress at each mileage stake near the tunnel entrance.
The uneven settlement of the mountain along the tunnel direction has a greater impact on the tunnel’s stress than the change in mountain load. The average stress of the tunnel mouth is 5.88 MPa, whereas the middle tunnel mouth section (650 measuring points) has an average stress of 4.90 MPa (499.32 kN/cm2). The two measuring points are 15 m apart, and the stress difference is 0.98 MPa. This results in an average shear stress of 0.98 MPa on the periphery of the tunnel, affecting the tunnel’s stability. The middle tunnel section and the tunnel mouth have a stress differential of 0.98 MPa in this result, which indicates that the average shear stress on the periphery of the tunnel is 0.98 MPa. Additionally, the stress distribution of each section of the tunnel is simulated using the difference method. The time change of each part of the tunnel has a certain periodicity, and it fluctuates the most in December 2022. It is speculated that the state of the mountain near the tunnel entrance is weak at the beginning of the tunnel project, which causes stress fluctuation at the top of the tunnel. The stress condition of the tunnel eventually tends to settle as tunnel engineering advances and volatility steadily decreases.
5 Conclusion
The plateau area railway has garnered remarkable interest due to its high construction difficulties and strategic importance. Besides causing numerous geological catastrophes along the line, the extraordinarily complex geological environment poses considerable geometric distortion issues for the early detection and monitoring of geological risks in this region.
Herein, a method for evaluating stress distribution in high-altitude Tibetan Plateau railway tunnels using high-precision radar satellite time-series InSAR technology was proposed. The proposed method effectively monitors and prevents geological hazards during the construction process considering it serves as a component of advanced geological prediction and surrounding rock deformation monitoring technology for high-altitude tunnels, particularly in Dongelu Tunnel of the China–Tibet Railway. The results show that the surface deformation of the mountain above the tunnel axis develops slowly and is relatively small, with a maximum vertical deformation rate of 1–3 mm/year. The average stress on the tunnel arch is 5.54 MPa, with a fluctuation range of 0.01 MPa. Temporal changes in various parts of the tunnel are periodic. This study reveals inconsistent surface settlement of the tunnel arch and the mountain above it, causing minor vertical stress changes. As the tunnel construction progresses, vertical stress variation shows periodicity due to an initial imbalance in internal stress within the mountain. Stress fluctuations near the tunnel entrance occur during the initial excavation phase, gradually diminishing as the project progresses and internal stress stabilizes.
Herein, the deformation monitoring of railway tunnels and stability analysis contributes to the accuracy evaluation analysis, which can reduce the influence on engineering construction and difficulty and has important guidance for advanced geological tunnel prediction.
Data availability statement
The original contributions presented in the study are included in the article/supplementary material; further inquiries can be directed to the corresponding author.
Author contributions
BaL: Methodology, Writing–original draft. BoL: Resources, Supervision, Writing–review and editing. BX: Investigation, Writing–original draft. HX: Investigation, Writing–original draft, Methodology. XL: Investigation, Writing–original draft, Resources. ZZ: Supervision, Writing–review and editing. YL: Conceptualization, Writing–original draft. XH: Validation, Writing–original draft. FS: Validation, Writing–original draft
Funding
The authors declare that financial support was received for the research, authorship, and/or publication of this article. This paper was supported by funds from the National Natural Science Foundation of China (Grant No. 61974035), Guangdong Basic and Applied Basic Research Funding (2021A515110638 and 2021A515110674), and Guangzhou Basic and Applied Basic Research Funding Project (202201011145).
Conflict of interest
Authors BaL, BoL, BX, HX, and XL were employed by China Construction Civil Construction Co., Ltd.
The remaining authors declare that the research was conducted in the absence of any commercial or financial relationships that could be construed as a potential conflict of interest.
Publisher’s note
All claims expressed in this article are solely those of the authors and do not necessarily represent those of their affiliated organizations, or those of the publisher, the editors, and the reviewers. Any product that may be evaluated in this article, or claim that may be made by its manufacturer, is not guaranteed or endorsed by the publisher.
References
Berardino, P., Fornaro, G., Lanari, R., and Sansosti, E.(2002) A new algorithm for surface deformation monitoring based on small baseline differential SAR interferograms. IEEE Trans Geosci Remote Sens, 2375. doi:10.1109/TGRS.2002.803792
Changyan, Z., Yueqing, L., Wei, L., and Longxun, C. (2005). Climatological characteristics of water vapor transport over eastern part of qinghai-xizang plateau and its surroundings. Plateau Meteorol. (06), 880–888. doi:10.3321/j.issn:1000-0534.2005.06.006
Chaoying, Z., Xiaojie, L., and Qin, Z. (2019). Research on loess landslide identification, monitoring and failure mode with InSAR technique in heifangtai, Gansu. Geomatics Inf. Sci. Wuhan Univ. 44 (07), 996–1007. doi:10.13203/j.whugis20190072
Dai, K., Li, Z., Xu, Q., Burgmann, R., Milledge, D. G., Tomas, R., et al. (2020). Time to enter the era of Earth-Observation based landslide warning system. IEEE Geoscience Remote Sens. Mag. 8 (1), 136–153. doi:10.1109/MGRS.2019.2954395
Daqing, G., Keren, D., Zhaocheng, G., and Zhenhong, L. (2019). Early identification of serious geological hazards with integrated remote sensing technologies: thoughts and recommendations. Geomatics Inf. Sci. Wuhan Univ. 44 (07), 949–956. doi:10.13203/j.whugis20190094
De-hong, Y. (2019). Analysis of main engineering geological problems in changdu to linzhi section of Sichuan∼Tibet railway. Railw. Stand. Des. 63 (09), 16–22+27. doi:10.13238/j.issn.1004-2954.201811200005
Dong, J., Liao, M., Xu, Q., Zhang, L., Tang, M., and Gong, J. (2018). Detection and displacement characterization of landslides using multitemporal satellite SAR interferometry: a case study of danba county in the dadu River Basin. Eng. Geol. 240, 95–109. doi:10.1016/j.enggeo.2018.04.015
Dong, J., Zhang, L., Li, M., Yu, Y., Liao, M., Gong, J., et al. (2017). Measuring precursory movements of the recent xinmo landslide in mao county, China with Sentinel-1 and ALOS-2 PALSAR-2 datasets. Landslides 15 (1), 135–144. doi:10.1007/s10346-017-0914-8
Fang, K., Dong, Ao, Tang, H., An, P., Wang, Q., Jia, S., et al. (2024). Development of an easy-assembly and low-cost multismartphone photogrammetric monitoring system for rock slope hazards. Int. J. Rock Mech. Min. Sci. 174, 105655. doi:10.1016/j.ijrmms.2024.105655
Guitang, P., Fei, R., Fuguang, Y., Liquan, W., Baodi, W., Dongbing, W., et al. (2020). Key zones of oceanic plate geology and sichuan-tibet railway project. Earth Sci. 45 (07), 2293–2304.
Hong, Y., Hongyan, M., Yongfa, L., Zhihong, W., Lan, L., and Peng, X. (2021). Monitoring and prediction of settlement along high speed railway supported by InSAR technology. Sci. Surv. Mapp. 46 (07), 67–75. doi:10.16251/j.cnki.1009-2307.2021.07.010
Huiyan, L., Wei, L., Qiang, X., Xiujun, D., Cong, D., and Dong, W. (2019). Early detection of landslides in the upstream and downstream areas of the baige landslide, the Jinsha River based on optical remote sensing and InSAR technologies. Geomatics Inf. Sci. Wuhan Univ. 44 (09), 1342–1354. doi:10.13203/j.whugis20190086
Jiajia, Z., Bo, G., Jiankang, L., Long, C., Hai, H., and Jie, L. (2021). Early landslide detection in the lancangjiang region along the sichuan-tibet railway based on SBAS-InSAR technology. Geoscience 35 (01), 64–73. doi:10.19657/j.geoscience.1000-8527.2021.005
Jianbing, P., Peng, C., and Jianqi, Z. (2020). Challenges to engineering geology of Sichuan-Tibet railway. Chin. J. Rock Mech. Eng. 39 (12), 2377–2389. doi:10.13722/j.cnki.jrme.2020.0446
Keren, D., Guanchen, Z., Qiang, X., Zhenhong, L., Wele, L., and WeI, G. (2019). Tracing the pre-failure two-dimensional sirface displacements of nanyu landslide, Gansu Province with radar interferometry. Geomatics Inf. Sci. Wuhan Univ. 44 (12), 1778–1786+1796. doi:10.13203/j.whugis20190092
Keren, D., Yongbo, T., Guanchen, Z., Qiang, X., Ye, F., and Xianlin, S. (2020). Early identification of potential landslide geohazards in alpine-canyon terrain based on SAR interferometrya case study of the middle section of yalong river. J. Radars 9 (03), 554–568. doi:10.12000/JR20012
Liu, X., Zhao, C., Zhang, Q., Peng, J., Zhu, W., and Lu, Z. (2018). Multi-Temporal loess landslide inventory mapping with C-, X- and L-band SAR datasets:A case study of heifangtai loess landslides, China. Remote Sens. 10, 1756. doi:10.3390/rs10111756
Meng, Q., Xu, Q., Wang, B., Li, W., Peng, Y., Peng, D., et al. (2019). Monitoring the regional deformation of loess landslides on the hei- fangtai terrace using the Sentinel-1 time series in terferometry technique. Nat. Hazards 98 (2), 485–505. doi:10.1007/s11069-019-03703-3
Nestor, Y., Pau, P., Fernando, R., Brcic, R., Shau, R., Geudtner, D., et al. (2016). Intererometric processing of Sentinel-1 TOPS data. IEEE Trans. Geoscience Remote Sens. 54 (4). doi:10.1109/TGRS.2015.2497902
Shi, X., Liao, M., Li, M., Zhang, L., and Cunningham, C. (2016). Wide-Area landslide deformation mapping with Multi-Path alos pal- SAR data stacks: a case study of three gorges area, China. Remote Sens. 8 (2), 136. doi:10.3390/rs8020136
Sun, Q., Hu, J., Zhang, L., and Ding, X. (2016). Towards Slow-Moving landslide monitoring by integrating Multi-Sensor in- SAR time series datasets: the zhouqu case study, China. Remote Sens. 8 (11), 908. doi:10.3390/rs8110908
Tomas, R., Li, Z., Liu, P., Singleton, A., Hoey, T., and Cheng, X. (2014). Spatiotemporal characteristics of the huangtupo landslide in the three gorges region(China)constrained by radar interferometry. Geophys. J. Int. 197 (1), 213–232. doi:10.1093/gji/ggu017
Xinghong, L., Xin, Y., Kaitbing, Y., Fuchu, D., Shifeng, W., and Jiaming, Y. (2020). Remote sensing integrated identification of geological hazards in the batang-mangkang sectia of the sichuan-tibet Highway. Adv. Eng. Sci. 52 (06), 49–60. doi:10.15961/j.jsuese.202000242
Yafeng, S., Jijun, L., Bingyuan, L., Tandong, Y., Suming, W., Shijie, L., et al. (1999). Uplift of the qinghai-xizang (Tibetan) plateau and east asia environmental change during late cenozoic. Acta Geogr. Sin. 54 (01), 12–22. doi:10.3321/j.issn:0375-5444.1999.01.002
Zhang, H., Oskin, M., Liu, Z., Zhang, P., Reiners, P. W., and Xiao, P. (2016). Pulsed exhumation of interior eastern tibet: implications for relief generation mechanisms and the origin of high elevation planation surfaces. Earth Planet. Sci. Lett. 449, 176–185. doi:10.1016/j.epsl.2016.05.048
Zhang, J., Lin, C., Tang, H., Wen, T., Tannant, D. D., and Zhang, B. (2024a). Input-parameter optimization using a SVR based ensemble model to predict landslide displacements in a reservoir area-A comparative study. Appl. Soft Comput. 150, 111107. doi:10.1016/j.asoc.2023.111107
Zhang, J., Tang, H., Li, C., Gong, W., Zhou, B., and Zhang, Y. (2024b). Deformation stage division and early warning of landslides based on the statistical characteristics of landslide kinematic features. Landslides 21, 717–735. doi:10.1007/s10346-023-02192-7
Zhang, S. (2016). The construction of Sichuan-Tibet railway with internal and external connectivity has been listed as a key project of the 13th Five-Year plan [N/OL]. Chengdu Business Daily.
Zhao, C., Lu, Z., Zhang, Q., and de la Fuente, J. (2012). Large-Area land- slide detection and monitoring with ALOS/PAL- SAR imagery data over northern California and Southern Oregon, USA. Remote Sens. Environ. 124, 348–359. doi:10.1016/j.rse.2012.05.025
Keywords: time-series interferometric synthetic aperture radar, plateau railway, deformation monitoring, spatiotemporal analysis, stress assessment
Citation: Lyu B, Liu B, Xie B, Xiao H, Liu X, Zhang Z, Li Y, Huang X and Shi F (2024) Study on InSAR deformation information extraction and stress state assessment in a railway tunnel in a plateau area. Front. Earth Sci. 12:1367978. doi: 10.3389/feart.2024.1367978
Received: 09 January 2024; Accepted: 13 June 2024;
Published: 07 August 2024.
Edited by:
Junrong Zhang, China University of Geosciences Wuhan, ChinaReviewed by:
Hong Xu, Changsha University of Science and Technology, ChinaJinxing Lai, Chang’an University, China
Copyright © 2024 Lyu, Liu, Xie, Xiao, Liu, Zhang, Li, Huang and Shi. This is an open-access article distributed under the terms of the Creative Commons Attribution License (CC BY). The use, distribution or reproduction in other forums is permitted, provided the original author(s) and the copyright owner(s) are credited and that the original publication in this journal is cited, in accordance with accepted academic practice. No use, distribution or reproduction is permitted which does not comply with these terms.
*Correspondence: Zhang Ziwen, zhangziwen@gzmtu.edu.cn