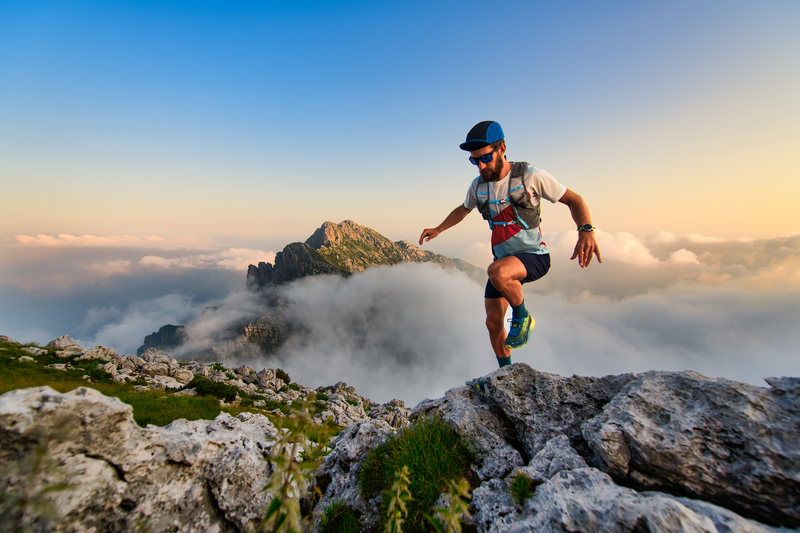
95% of researchers rate our articles as excellent or good
Learn more about the work of our research integrity team to safeguard the quality of each article we publish.
Find out more
ORIGINAL RESEARCH article
Front. Earth Sci. , 26 March 2024
Sec. Geoscience and Society
Volume 12 - 2024 | https://doi.org/10.3389/feart.2024.1353572
This article is part of the Research Topic Near-Surface Geophysics in Latin American Contexts: Its Applications, Education and Societal Perspectives as a whole View all 6 articles
Trees sequester carbon dioxide from the atmosphere through photosynthesis, storing it in branches, stems, and roots, where the belowground carbon fraction, approximately ¼ of the total amount, exhibits significant interspecies root biomass variability. Estimating the amount of carbon stored in tree roots of different species is key to understanding an important aspect of climate change and exploring how natural forests, urban tree planting policies, and reforestation projects might help to address it. In this context, one of the most prominent Non-Destructive Testing methods capable of estimating the diameter and length of roots at different depths is ground penetrating radar (GPR). It has been widely used for geological, archaeological, and geotechnical studies due to its accuracy in locating buried material in different contexts, although standards for the correct management of datasets related to belowground root systems are still been developed. This paper reports a GPR signal processing flow to estimate the root diameter of three species of tropical forest trees, and to demonstrate the method’s viability, a dataset was collected in a study area with a 900 MHz shielded antenna. A multi-stage data processing flow is then presented, including raw data, file format conversion, zero-time adjustment, background removal, signal gain, Stolt FK migration, and time-to-depth conversion with hyperbolic adjustment velocity. The resulting data were converted from true amplitude data to a trace envelope. High amplitudes on the envelope section, with lateral continuity in parallel sections, were interpreted as roots. However, the interpretation of outcomes encounters notable complexities, primarily attributable to the intricate nature of subsurface root architectures, the soil matrix characterized by significant clay content, and the co-occurrence of buried materials proximate to the arboreal subjects. Consequently, amplitudes discerned within ground penetrating radar (GPR) 2D sections necessitate cautious interpretation, as they are not immediately indicative of subsurface roots. To overcome this difficulty, this study used direct measurements of the roots in the field, to confirm the GPR data. Despite these complexities, the study demonstrates GPR’s efficacy, particularly in the uppermost soil layer-a pivotal carbon reservoir with a 96% correlation (R2) between GPR-derived coarse-root diameter estimates and field measurements.
In recent decades, anthropic actions have triggered a series of climate changes with the increase in greenhouse gases, and other activities intensified since the Industrial Revolution with the burning of fossil fuels (Houghton, 2003; Carvalho et al., 2010).
The increase in greenhouse gas concentration has been the focus of several international conferences and conventions such as United Nations Framework Convention on Climate Change (UNFCCC) in 1992 and the Kyoto Protocol in 1997, REDD++ program in 2009, IPCC in 2014, the Paris Agreement in 2015, among others. The main objective is to find strategies to reduce greenhouse gas emissions into the atmosphere. This has motivated the demand for knowledge about the ability of different ecosystems to capture and fix carbon from the atmosphere in their biomass.
In this context, forests are an important provider of environmental services to mitigate climate change. The carbon fixed by the reservoir of living biomass (above and below ground) can represent between 70% and 95% of the sequestered CO2 during the growth of a tropical forest (Centro de Gestão e Estudos Estratégicos, 2008), the rest is represented by the litter, dead wood and carbon in the soil.
Evaluating the biomass distribution on the planet is important to estimate carbon sequestration stocks, for the modeling of biogeochemical cycles and for understanding the historical effects and future impacts of anthropic actions. Subsurface biomass represents 40% of the planet’s total biomass and is estimated at 230 Gt C (Bar-On et al., 2018).
According to Grace et al. (2006), the productivity of the biomass below the soil contained in the roots of the savanna ecosystem is often underestimated due to the difficulty of quantifying, since destructive methodologies require a large amount of time and service, making the activity quite costly. According to Qureshi et al. (2012), the quantification of carbon stock in forests is a challenging task, that involves a high level of uncertainties and discrepancies.
An alternative to the destructive method is the indirect method using allometric equations to estimate root biomass. However, it is rare in the literature that have adjusted allometric models to obtain root biomass of a natural ecosystem (Kuyah et al., 2012; Cornet et al., 2015; Kuyah et al., 2016; Koala et al., 2017).
As a promising alternative to indirect techniques, ground penetration radar (GPR), a geophysical method for subsoil mapping, has been studied since Hruska et al. (1999) for underground biomass imaging. GPR is a geophysical method that uses radar pulses to image the subsurface. It is a non-invasive technique that allows for the detection and imaging of buried objects, structures, or geological features beneath the ground surface. The use of GPR has shown good results in imaging the behavior of root patterns and is still being studied to be established as a traditional methodology (Butnor et al., 2001; Butnor et al., 2003; Butnor et al., 2005; Vianden et al., 2010; Tanikawa et al., 2013; Borden et al., 2014; Guo et al., 2015; Bain, 2016; Nichols et al., 2017; Agbona et al., 2021).
Coarse root investigation by GPR is being tested for different types of soil and plant species (Butnor et al., 2001; Guo et al., 2015; Altdorff et al., 2019; Lantini et al., 2020). The feasibility and accuracy of diameter estimations based on radargrams - the graphical representation of the data collected by a GPR - are still in discussion in the literature (Butnor et al., 2001, Guo et al., 2015; Lantini et al., 2020; Alani and Lantini, 2020; Wang et al., 2020). Many parameters such as local soil conditions, electromagnetic root properties, and GPR antenna frequency can impact the results. Field data collection protocols are not yet well established (Guo et al., 2015, Wang et al., 2020; Alani and Lantini, 2020). In particular, studies of biomass estimation with GPR on South American tropical soils are absent, as far as we know.
The studied area is located in Brasilia, Brazil (Figure 1). The climate of the region is characterized by long interspersed periods of drought and rain (INMET, 2019). The region’s main soil is the Oxisols (Haridasan, 1994), and we chose three species of trees for our study. The trees were planted in garden soil inside the University of Brasilia. The studied trees include: the Schizolobium parahyba, the Mangifera indica, and the Acacia farnesiana (L.) Willd.
Figure 1. Location map of the trees studied inside the University of Brasilia/Darcy Ribeiro Campus. (A) Schizolobium parahyba; (B) Mangifera indica; (C) Acacia farnesiana (L.) Willd.
The GPR method has been confirmed in recent years as an efficient methodology for root determination (Butnor et al., 2016; Delgado et al., 2017; Almeida et al., 2018; Linna et al., 2022), since underground biomass is the most time-consuming and challenging component to be quantified in any forest ecosystem. Furthermore, direct biomass estimation methods are generally not standardized (Britez et al., 2006).
Coarse root investigation by GPR is commonly conducted from the interpretation of reflection waveform indexes (Butnor et al., 2001; Butnor et al., 2003; Guo et al., 2013), or reflection amplitude intensity indexes (Guo et al., 2013).
This case study aims to evaluate the possibility of estimating the actual size of a coarse root diameter with GPR data and Hilbert Transform, following the data processing steps proposed by Wielopolski et al., 2000. We estimated the diameter of the detected roots by analyzing their waveforms, and the distance between the top, and bottom reflection of a root within 2D GPR data. The first framework of root diameter estimation through GPR data was proposed by Butnor et al. (2001).
The objective of this work is to verify the diameter of tree’ roots indirectly, by using GPR data to improve the estimation of underground biomass, following the protocols suggested by Butnor et al., 2005; Guo et al., 2015. This is a case study work to evaluate the efficacy of these protocols in a tropical context, within Brazilian soil. We show that with adequate parameters tunning, it is possible to retrieve coarse root diameters. To evaluate results, direct verification with in situ methodologies is used to compare the image results.
The GPR is a geophysical method that records high frequency (10–2,600 MHz) electromagnetic waves artificially irradiated to media. The electromagnetic waves propagate in the medium and depending on the electrical characteristics of the structures present in the medium, the signal returns in the form of reflected, diffracted and refracted waves (Annan and Chua, 1992). The electrical signal is converted into a digital signal and arranged in traces that represent variations in the amplitude of the electromagnetic signal as a function of travel time. The set of traces obtained along a path or time, with the same distance between the antennas, is called a radargram (Figure 2). The main components of the electromagnetic field come from the Fresnel zone (Zhang et al., 2019).
Figure 2. (A) Graphic representation of the propagation of the electromagnetic wave of the GPR in a homogeneous medium with the presence of roots. (B) GPR pulse recorded in the presence of a root. (C) Radargram with the indication of a hyperbola related to a root.
The main factor controlling the equipment signal is the electromagnetic frequency (Annan and Chua, 1992). Thus, the choice of the central frequency of GPR should always be preceded by soil identification since the amount of clay, salt content, or water can directly influence radar signal attenuation (Doolittle and Butnor, 2009).
According to Table 1, the dielectric constant represents the particular physical characteristic of the medium in which the radar pulse travels. The dielectric constant plays a prominent role when working with high-frequency electromagnetic methods (>1 MHz). For frequencies between 1 MHz and 2.6 GHz, the water content governs the dielectric properties of the materials since the relative water permissiveness value is ten times greater than the characteristic permittivity of the roots.
Table 1. Interval of dielectric constant, electrical conductivity and magnetic permeability, (Annan and Chua, 1992).
Antennas of high frequencies provide better results in dry, and electrically resistive soils because the attenuation is directly proportional to the electrical conductivity of the medium. The higher the conductivity in the soil, the higher the attenuation of the electromagnetic wave (Annan and Chua, 1992).
In this way, the GPR method has limitations on the imaging and characterization of belowground biomass in high conductivity soils. In this case study, the soil is characterized as an oxidized soil, Oxisols (Haridasan, 1994). This type of soil has a high content of clay, and high conductivity, making the radargram processing a challenging task. Nevertheless, we found suitable parameters for locating the position, and dimensions of the roots of trees in a non-invasive way. The drought season is the most convenient period for GPR data acquisition.
Antennas with frequencies between 900 MHz and 1.5 MHz have been used for shallow investigations and sandy soils. In soils of middle attenuation, where the penetration of the signal at depth is quite limited, these antennas of high frequency provide a depth of investigation comparable to the low-frequency antennas (Loewer et al., 2016). However, the antennas most used for soil surveys have a central frequency between 100 and 500 MHz because they reach greater depth than high-frequency antennas. Organic soils are responsible for a high attenuation of the electromagnetic signal (Van Dam, 2014). When considerable depths of research are necessary, low-frequency antennas between 70 and 200 MHz are commonly used (Doolittle and Butnor, 2009).
We used the GPR model SIR-3000 (manufactured by Geophysical Survey Systems, Inc.) coupled with a pair of shielded antennas with a central frequency of 900 MHz and an odometrical wheel for data acquisition. The data were acquired through the common offset technique (fixed distance between antennas), where a pair of antennas (one transmitter and one receiver) is simultaneously moved along each profile (Jonard et al., 2019), allowing real-time visualization of the reflections in the subsoil.
The data were collected along 4 m profiles, equispaced in 5 cm, where the three species were in the center of an area of 16 m2, for each of the surveys (Figure 3). The set of 2D GPR sections obtained with this small spacing between profiles allows the interpolation of all traces in 3D GPR volumes (Grasmueck et al., 2005). The data was collected during the dry season in September, when the Oxisols have higher resistivity, providing a better GPR image. The trees are placed in the garden of the University of Brasília, Darcy Ribeiro Campus.
Figure 3. Picture shows the GPR system used in data acquisition and the survey lines for Schizolobium parahyba. Mangifera indica, and Acacia farnesiana (L.) Willd. survey follow similar patterns.
The root diameters were measured on the 2D GPR radargrams and then measured through the in situ methodology, the traditional excavation method, as shown in Figure 4. Single-tree excavation methods consist of removing the tree root system from the soil and tracing each root individually from the stump to root tip (Pierret et al., 2005). Allometric equations (Kuyah et al., 2012; Koala et al., 2017) can also be used to evaluate root biomass, but such models need to be calibrated on large amounts of data collected by reliable excavation methods (Saint-André et al., 2005).
Figure 4. Picture shows the in situ methodology, the excavation method used for root diameter measurements.
We excavated the roots after the GPR acquisition. We first identified the correct location of root samples belowground using the 3D GPR radargram slices. Then, we measured the diameter of each sample to further correlate with estimated diameters from GPR data.
After the exposure of the target root defined by the 3D, and 2D GPR images, the root diameters were measured for each tree. For this preliminary work, the in situ measurements collected consisted of 10 samples of root diameter for each tree through the excavation method.
Forward modeling provides a faster alternative to study the limiting factors of GPR root investigation when compared to real data. Since all the variables in the synthetic model can be precisely controlled, it is possible to differentiate and detect the various limiting factors (Guo et al., 2013).
The synthetic models were generated using the software ReflexW, version 7.5 (Sandmeier, 2015). The software simulates the propagation of the electromagnetic waves using input values for dielectric conductivity and permittivity for each x, and z coordinate. The modeling algorithm uses a time domain finite difference method for solving Maxwell’s equations. We generated 16 different models, where we varied the values of some physical properties of the dielectric medium such as presented in Table 2, the dielectric constant of the soil, electrical conductivity of the soil, root diameter, and root depth. We varied these values to build a synthetic controlled experiment of the root’s complex organic system presented in the real dataset.
Table 2. Dielectric constant and electrical conductivity values, recorded in the field and in the laboratory, and used to generate the synthetic models in the ReflexW software.
For the generation of a finite difference model with numerical efficiency it is necessary to define the horizontal spatial sampling (dx) as a function of vertical sampling (dz). The spatial increment dx depends on the shortest wavelength λ as defined in Equation 1:
Where the central frequency is f = 900 MHz and c = vacuum velocity (0.3 m/ns). To determine the time increment dt that respects numerical stability, we can use the Equation 2 (Taflove and Hagness, 1995):
We set the time increment dt = 0.0046s and the spatial increment dx = 0.0028 m. We defined the edge conditions as a linear absorbing range, in which the edges of the model absorb the energy of the waves arriving at them, avoiding spurious reflections. Also, we defined the target as an exploding reflector (Yilmaz, 2001) to simulate that waves are emitted directly by the target towards the surface. We used a Kuepper plane wave as the source, with a 900 MHz central frequency, simulating the antenna we used for the real data survey. The root target was defined as a circular layer at various depths (20 cm, 30 cm, 40 cm, and 50 cm). The root target was defined as a circular layer with different diameters (10 cm and 20 cm).
After the data processing, the same indexes were extracted from the synthetic radargrams and analyzed similarly to the real data surveyed.
We processed the data in the software ReflexW, version 7.5, Sandmeier (2015). Although environment conditions are different in each case study on GPR applied to the identification of belowground biomass, data processing routines have been gradually established, including GPR radargram standardization, noise reduction, migration, and Hilbert transformation (Wielopolski et al., 2000; Butnor et al., 2003; Stover et al., 2007; Dannoura et al., 2008; Butnor et al., 2008; Hirano et al., 2009, Guo et al., 2015).
The signal processing workflow is exemplified in Figure 5. It consisted of file format conversion of (A) raw data (B) zero time adjustment (C) background removal (D) gain - declipping, and (E) Stolt or fk migration. The time to depth conversion was done with hyperbolic adjustment velocity found at roots of 0.12 m/ns. The resulting data is (F) converted from the true amplitude data to a trace envelope.
Figure 5. (A) Raw data; (B) Radargram after zero time correction; (C) Background removal; (D) Gain (declipping); (E) Stolt or fk migration; (F) Hilbert Transform (Trace Envelope).
According to Sandmeier (2015), stolt migration works in the frequency wavenumber (fk) domain. The migration aims to trace the reflected and diffracted energy back to the source (Figure 5E). Alani and Lantini (2020) suggested that the application of Fk or Stolt migration significantly improved the effectiveness of root detection and quantification algorithms, as it decreased the errors in identifying the roots’ positions.
The trace envelope is the amplitude of the Hilbert transform. High amplitudes on the envelope section are interpreted as roots. Through the trace envelope, the roots are measured (distance between top, and bottom of a root) using the depth scale to convert it to centimeters. Note that in 2D sections, it is not possible to distinguish a small rock from a root transversal cut, for example,. For proper analysis of tree roots, it is necessary to check 3D GPR radargram slices.
Estimated diameters from radargrams and actual measures of root diameters were correlated using a linear regression model and the coefficient of determination. This statistical method can assess how strong the linear relationship is between two variables, it is a measure that represents the proportion of the variance for a dependent variable that is explained by an independent variable or variables in a regression model (Butnor et al., 2003).
Figure 6 shows the data acquired with the 900 MHz GPR antenna. On the left column, it is possible to identify hyperbolic diffractions related to the roots of trees, with the adjustment of the penetration of the signal to the maximum depth of 2 m. On the right column, it is possible to observe the results of the Hilbert Transform, and we identified the detected roots with a black line.
Figure 6. 2D GPR signal after migration step for (A1) Schizolobium parahyba; (B1) Mangifera indica; (C1) Acacia farnesiana (L.); Willd. Hilbert transforms for (A2) Schizolobium parahyba; (B2) Mangifera indica; (C2) Acacia farnesiana (L.); Willd. Velocity used for time-depth conversion: 0.12 m/ns.
Figure 7 illustrates the estimated diameter measured from the radargrams by each trace. The estimated diameter is an important variable to be consistently compared to the real diameter. The dielectric constant of the soil plays an important role when estimating the root diameter. The dielectric constant of the soil influences the electromagnetic wave propagation, changing the waveform to be analyzed.
Figure 7. Illustration of ɸe (estimated diameter) extratcted from radargrams measured by trace for (A) Schizolobium parahyba; (B) Mangifera indica; (C) Acacia farnesiana (L.); Willd.
Figure 8 exemplifies the differences between the estimated diameter (cm) by depth (cm) for dry soil (A) and humid soil (B). It is possible to visualize that the estimated diameter varies in comparison to the real diameter, overestimating or underestimating it. The graph also demonstrates how the diameter estimation is better for dry soil environment when analyzing deeper roots.
Figure 8. Graph illustrates the synthetic data generated. The differences between the estimated diameter (cm) by depth (cm) for dry (A) and humid soil (B).
Soil is a complex substance made up of different components. It contains different solid materials, air, free and bound water. There are different types of soil covering the surface of the earth. It is useful to separate the dry and wet components of soil while estimating soil’s dielectric properties. The permittivity of dry soil is better understood than the wet soil because dielectric losses can lead to water contribution.
Figure 9 exemplifies how the maximum amplitude (dB) value by depth changes for humid soil and dry soil. It is possible to verify from the synthetic data in this graph that humid soil will generated root anomalies with considerably higher amplitude. It is possible to identify how the maximum amplitude diminishes when depth increases due to the geometric dispersion and backscattering of propagating electromagnetic waves.
Figure 9. Graph illustrates the synthetic data generated. Max amplitude (dB) by Depth (cm) with different dielectric constants of soil (dry or humid soil).
Figure 10 presents depth slices in 3D GPR blocks of the three species, which allows the visualization of the roots distribution patterns, and root diameters. The GPR radargrams were correlated with in situ methodology.
Figure 10. 3D GPR signal with Hilbert Transform (A) Schizolobium parahyba’s slice at 0.17 m depth; (B) Mangifera indica’s slice at 0.08 m depth; (C) Acacia farnesiana (L.); Willd.'s slice at 0.15 m depth.
Each studied tree was excavated at 20 cm depth, and the in situ methodology was tested with 10 root samples for each tree, totalizing 30 samples. The root diameter (or thickness) was measured with the GPR radargrams and its results compared to the in situ measurement through the conventional excavation method.
We used the Python package Statsmodel (Seabold and Perktold, 2010) to run an ordinary least squares regression model, with the in situ measured diameters as dependent variable, and the estimated root diameters from GPR data as the explanatory variable. The model was built from the 30 samples that were collected in the field. Raw data provided nonlinear and heteroscedastic residual errors, which would invalidate linear regression models.
The White test for heteroscedasticity of residuals provided an LM test’s p-value of 0.0003, so we rejected the hypothesis of the test that residual errors were homoscedastic. The Lagrange Multiplier (LM) test, also known as the score test, is a hypothesis test used to check whether some parameter restrictions are violated. This test can be performed after estimating the parameters by maximum likelihood.
Furthermore, the F-test p-value was also practically null, as we can see in Table 3. An F-test is any statistical test used to compare the variances of two samples or the ratio of variances between multiple samples.
Table 3. OLS summary for raw data and exponential transformed data set, and White test on residual errors results.
To dampen heteroscedasticity effects and remove nonlinearity, we transformed the data by applying an exponential on the dependent variable. We built a primary model by fitting an OLS linear regression model to the exponential-transformed dataset. In statistics, the Ordinary Least Squares (OLS) is a type of linear least squares method for choosing the unknown parameters in a linear regression model by the principle of least squares. Based on the model assumptions, it is possible to derive estimates on the intercept and slope that minimize the sum of squared residuals (SSR). Minimizing the SSR is a desired result, since we want the error between the regression function and sample data to be as small as possible. The coefficient estimates that minimize the SSR are called the Ordinary Least Squares (OLS).
Then we evaluated heteroscedasticity on the residual errors by running the White Test on a secondary model, in which the dependent variable was the square of the residuals from the primary model, and the explanatory variables were the “Real root diameter” and its square. The White test for heteroscedasticity on the residual errors returned a much higher p-value for F-statistic, of 0.421 than for the previous raw data, which confirmed the null hypothesis of the test that there was no heteroscedasticity in the residual errors. Furthermore, the LM test statistics’ p-value (0.394) was much higher than the previous result. Overall, we concluded that the residual errors for the exponential transformed dataset were homoscedastic. The linear regression for the exponential transformed dataset returned an R2 of 0.965, indicating that we can trust predictions of root diameters by GPR images, when it is possible to reach the ideal conditions (Figure 11).
Figure 11. Correlation graph between measured root thickness with 2D GPR Signal, and measured root thickness on the field. The error bars represent the error percentage on each measure.
The ideal conditions to successfully measure the root diameter, and consequently the root volume belowground, are dependable on the root geometry, root depth, root thickness, root water content (Guo et al., 2013), cross angles between root and survey line (Hirano et al., 2009; Tanikawa et al., 2013, Guo et al., 2015), soil humidity, and others. Nevertheless, it is possible to estimate coarse root diameter using GPR under certain conditions: shallow coarse roots, roots containing water in its composition.
In this case study, S. parahyba presents longer and thicker roots than other species, they are sparse and present a horizontal growth pattern (at the same depth).
Mangifera indica presents an interwinted growth pattern with shorter and thinner roots in general and intense variation in root depth, causing several diffraction patterns. Acacia farnesiana (L.) Willd. has the less reliable correlation results of this case study because it has an intertwined growth pattern with intense variation in root depth. In addition, A. farnesiana (L.) Willd.'s roots are also thinner. Future studies might focus on more modern data processing methods for super-resolution imaging.
We observed that the 3D GPR provides better results than the 2D GPR. It is necessary to consider the root growth pattern and root identification on the map belowground. Despite being useful for estimating root diameters, the 2D GPR profile alone can not allow the interpretation of the root angles and their root continuity.
The 3D GPR provided a fast way to identify the root patterns and the roots targeted as root samples for the diameter measurement.
The best framework for the quantification of roots with the use of GPR is still being studied (Guo et al., 2013, Guo et al., 2015, Altdorff et al., 2019; Wang et al., 2020, Alani and Lantini, 2020; Lantini et al., 2020).
GPR has proven to be an efficient method to identify roots of trees in different environments, although the identification of roots of trees in Brazil has not been studied yet. Figure 11 presents the linear R-squared correlation results for the 2D GPR data.
This preliminary study shows the strong correlation between the GPR images, and the real root diameter.
Soils with high content of clay are more challenging to detect roots than sandy soils. We showed that it is possible to characterize roots up to 1 m depth in Oxisols using 2D and 3D sections. The interpretation of roots in radargram is extremely subjective in 2D sections, because root systems are extremely complex and unpredictable. Furthermore, high amplitudes in radargrams cannot be immediately interpreted as roots. Roots patterns are more interpretable in 3D sections, which are the most recommended for belowground biomass studies with GPR. This study presents the strong correlation between the root diameter measured with the GPR and the root diameter measured with the excavation method. In future work, using software development, we pretend to make it possible to estimate the volume of belowground biomass using measurements of root diameters, 2D GPR data, and 3D GPR data.
Indirect belowground carbon estimation is a methodology that requires further research, and the development of new GPR applications in this area is promising. There are several open topics in this research field, such as how the water content within the soil, and within the roots, impact results; the differences in the root depth, root thickness, and root cross angles between the survey lines, among others.
The GPR radargrams are better trustworthy when correlated with other direct measurements taken at field level. Further development of post-processing software tools is recommended to integrate the field data, and the GPR data to develop better statistical solutions, using volume estimation, and the pixel intensity values.
It is possible to evaluate the validity of the real data surveyed and processed with the synthetic data. The graphs show the distribution parameters of the differences in amplitude according to the differences in the dielectric constant in the different humidity values in the soil.
The raw data supporting the conclusion of this article will be made available by the authors, without undue reservation.
AA: Conceptualization, Data curation, Formal Analysis, Investigation, Methodology, Project administration, Software, Validation, Visualization, Writing–original draft, Writing–review and editing. WB: Conceptualization, Data curation, Funding acquisition, Project administration, Resources, Software, Supervision, Validation, Visualization, Writing–review and editing. MG: Funding acquisition, Project administration, Resources, Supervision, Validation, Visualization, Writing–review and editing. FF: Investigation, Validation, Visualization, Writing–review and editing. SR: Supervision, Validation, Visualization, Writing–review and editing. JD: Funding acquisition, Methodology, Resources, Investigation, Writing–review and editing, Conceptualization, Data curation, Project administration, Supervision, Validation, Visualization. TB: Funding acquisition, Investigation, Methodology, Writing–review and editing.
The author(s) declare that no financial support was received for the research, authorship, and/or publication of this article.
We would like to acknowledge the Seismological Observatory of the University of Brasilia - ObSIS (www.obsis.unb.br) for the support on the development and conclusion of this project.
Author JD was employed by GITEC-IGIP GmBH.
The remaining authors declare that the research was conducted in the absence of any commercial or financial relationships that could be construed as a potential conflict of interest.
All claims expressed in this article are solely those of the authors and do not necessarily represent those of their affiliated organizations, or those of the publisher, the editors and the reviewers. Any product that may be evaluated in this article, or claim that may be made by its manufacturer, is not guaranteed or endorsed by the publisher.
Agbona, A., Teare, B., Ruiz-guzman, H., Dobreva, I. D., Everett, M. E., Adams, T., et al. (2021). Prediction of root biomass in cassava based on ground penetrating radar phenomics. Remote Sens. 13, 4908. doi:10.3390/rs13234908
Alani, A. M., and Lantini, L. (2020). Recent advances in tree root mapping and assessment using non-destructive testing methods: a focus on ground penetrating radar. Surv. Geophys 41, 605–646. doi:10.1007/s10712-019-09548-6
Almeida, E. R., Porsani, J. L., Booth, A., Brunello, A. T., and Sarkinen, T. (2018). “Analysis of GPR field parameters for root mapping in Brazil’s caatinga environment,” in 2018. 17th International Conference on Ground Penetrating Radar (GPR). doi:10.1109/icgpr.2018.8441547
Altdorff, D., Botschek, J., Honds, M., van der Kruk, J., and Vereecken, H. (2019). In situ detection of tree root systems under heterogeneous anthropogenic soil conditions using ground penetrating radar. J. Infrastructure Syst. 25 (3), 05019008. doi:10.1061/(asce)is.1943-555x.0000501
Annan, A. P., and Chua, L. T. (1992). Ground penetrating radar performance predictions: the geological survey of Canada, 5–13. Paper 90-4.
Bain, J. C. (2016). Coarse root biomass and architecture: applications of ground penetrating radar. Doctor of Philosophy (PhD), Dissertation. Biological Sciences, Old Dominion University. doi:10.25777/rbc1-d155https://digitalcommons.odu.edu/biology_etds/15
Bar-On, Y. M., Phillips, R., and Milo, R. (2018). The biomass distribution on Earth. PNAS Latest Articles. doi:10.1073/pnas.1711842115
Borden, K. A., Marney, E. I., Thevathasan, N. V., Gordon, A. M., and Thomas, S. C. (2014). Estimating coarse root biomass with ground penetrating radar in a tree-based intercropping system. Agrofor. Syst. 88 (4), 657–669. doi:10.1007/s10457-014-9722-5
Britez, R. M. D. E., Borgo, M., Tiepolo, G., Ferretti, A., Calmon, M., and Higa, R. (2006). Estoque e incremento de carbono em florestas e povoamentos de espécies arbóreas com ênfase na Floresta Atlântica do Sul do Brasil. Colombo: embrapa Florestas; Curitiba. Sociedade de Pesquisa em Vida Selvagem e Educação Ambiental, 165p. Available at: https://ainfo.cnptia.embrapa.br/digital/bitstream/doc/313733/1/EmbrapaFlorestas-2006-EstoqueIncrementoCarbonoFlorestas.pdf
Butnor, J. R., Doolittle, J. A., Johnsen, K. H., Samuelson, L., Stokes, T., and Kress, L. (2003). Utility of ground penetrating radar as a root biomass survey tool in forest systems. Soil Sci. Soc. Am. J. 67, 1607–1615. doi:10.2136/sssaj2003.1607
Butnor, J. R., Doolittle, J. A., Kress, L., Cohen, S., and Johnsen, K. H. (2001). Use of ground-penetrating radar to study tree roots in the southeastern United States. Tree Physiol. 21. Victoria: Canada, 1269–1278.
Butnor, J. R., Roth, B., and Johnsen, K. H. (2005). “Feasibility of using ground-penetrating radar to quantify root mass in Florida''s intensively managed pine plantations,” in Forest biology research cooperative report # 38 (FL: Gainsesville), 13.
Butnor, J. R., Samuelson, L. J., Stokes, T. A., Johnsen, K. H., Anderson, P. H., and GonzálezBenecke, C. A. (2016). Surface-based GPR underestimates below-stump root biomass. Plant Soil 402, 47–62. doi:10.1007/s11104-015-2768-y
Butnor, J. R., Stover, D. B., Roth, B. E., Johnsen, K. H., Day, F. P., and McInnis, D. (2008). “Using ground-penetrating radar to estimate tree root mass comparing results from two Florida surveys,” in Handbook of agricultural Geophysics. Editors B. J. Allred, J. J. Daniels, and M. R. Ehsani (Boca Raton: CRC Press), 375–382.
Carvalho, J. L. N., Avanzi, J. C., Silva, M. L. N., Mello, C. R., and Cerri, C. E. P. (2010). Potencial de sequestro de carbono em diferentes biomas do Brasil. Rev. Bras. Ciência do Solo 34, 277–290. doi:10.1590/s0100-06832010000200001
Centro de Gestão e Estudos Estratégicos (CGEE) (2008). Manual de Capacitação sobre Mudança do Clima e Projetos de Mecanismo de Desenvolvimento Limpo (MDL). Brasília, 276p.
Cornet, D., Sierra, J., and Tournebize, R. (2015). Assessing allometric models to predict vegetative growth of yams in different environments. Agron. J. 107, 241–248. doi:10.2134/agronj14.0370
Dannoura, M., Hirano, Y., Igarashi, T., Ishii, M., Aono, K., Yamase, K., et al. (2008). Detection of Cryptomeria japonica roots with ground penetrating radar. Plant Biosyst. 142, 375–380. doi:10.1080/11263500802150951
Delgado, A., Hays, D. B., Bruton, R. K., Ceballos, H., Novo, A., Boi, E., et al. (2017). Ground penetrating radar: a case study for estimating root bulking rate in cassava (Manihot esculenta Crantz). Plant Methods 13 (1), 65. doi:10.1186/s13007-017-0216-0
Doolittle, J. A., and Butnor, J. R. (2009). “Ground penetrating radar: theory and applications,” in Chapter 6: soils, peatlands, and biomonitoring. Editor H. M. Jol (Elsevier Science).
Grace, J., San Jose, J., Meir, P., Miranda, H. S., and Montes, R. A. (2006). Productivity and carbon fluxes of tropical savannas. J. Biogeogr. 33, 387–400. doi:10.1111/j.1365-2699.2005.01448.x
Grasmueck, M., Weger, R., and Horstmeyer, H. (2005). Full-resolution 3D GPR imaging. Geophysics 70 (1), 12–19. doi:10.1190/1.1852780
Guo, L., Lin, H., Fan, B., Cui, X., and Chen, J. (2013). Impact of root water content on root biomass estimation using ground penetrating radar: evidence from forward simulations and field controlled experiments. Plant Soil 371, 503–520. doi:10.1007/s11104-013-1710-4
Guo, L., Wu, Y., Chen, J., Hirano, Y., Tanikawa, T., Li, W., et al. (2015). Calibrating the impact of root orientation on root quantification using ground-penetrating radar. Plant Soil 395, 289–305. doi:10.1007/s11104-015-2563-9
Haridasan, M. (1994). “Solos do distrito federal. ” in Cerrado: caracterização, ocupação e perspectivas – O caso do Distrito Federal. 2aed. Editor M. Novaes-Pinto (Brasília: Editora Universidade de Brasília/Sematec), 681. 321-344.
Hirano, Y., Dannoura, M., Aono, K., Igarashi, T., Ishii, M., Yamase, K., et al. (2009). Limiting factors in the detection of tree roots using ground-penetrating radar. Plant Soil 319, 15–24. doi:10.1007/s11104-008-9845-4
Houghton, R. A. (2003). Revised estimates of the annual net flux of carbon to the atmosphere from changes in land use and land management 1850-2000. Tellus Ser. B-Chemical Phys. Meteorology 55 (2), 378–390. doi:10.3402/tellusb.v55i2.16764
Hruska, J., Čermák, J., and Sustek, S. (1999). Mapping tree root systems with ground-penetrating radar. Tree Physiol. 19, 125–130. doi:10.1093/treephys/19.2.125
INMET (Instituto Nacional de Meteorologia) (2019). Prognóstico climático primavera. Available on: https://portal.inmet.gov.br/uploads/notastecnicas/PROGNOSTICO_CLIMATICO_DE_PRIMAVERA_2019.pdf (Accessed June 30, 2021).
Jonard, F., Bogena, H., Caterina, D., Garré, S., Klotzsche, A., Monerris, A., et al. (2019). Ground-based soil moisture determination. Ecohydrology, 29–70. doi:10.1007/978-3-662-48297-1_2
Koala, J., Sawadogo, L., Savadogo, P., Aynekulu, E., Heiskanen, J., and Saïd, M. (2017). Allometric equations for belowground biomass of four key woody species in West African savanna-woodlands. Silva Fenn. 51. 3 article id 1631. doi:10.14214/sf.1631
Kuyah, S., Dietz, J., Muthuri, C., Jamnadass, R., Mwangi, P., Coe, R., et al. (2012). Allometric equations for estimating biomass in agricultural landscapes: II. Belowground biomass. Belowgr. biomass. Agric. Ecosyst. Environ. 158, 225–234. doi:10.1016/j.agee.2012.05.010
Kuyah, S., Sileshi, G. W., and Rosenstock, T. S. (2016). Allometric models based on Bayesian frameworks give better estimates of aboveground biomass in the Miombo Woodlands. Forests 7, 13. doi:10.3390/f7020013
Lantini, L., Tosti, F., Giannakis, I., Zou, L., Egyir, D., Mortimer, D., et al. (2020). Health assessment of trees using GPR-derived root density maps. IEEE Radar Conf. (RadarConf20), 1–5. doi:10.1109/RadarConf2043947.2020.9266447
Linna, P., Halla, A., and Narra, N. (2022). “Ground-penetrating radar-mounted drones in agriculture,” in New developments and environmental applications of drones. Editors T. Lipping, P. Linna, and N. Narra (Cham: Springer). doi:10.1007/978-3-030-77860-6_8
Loewer, M., Igel, J., and Wagner, N. (2016). Spectral decomposition of soil electrical and dielectric losses and prediction of in situ GPR performance. IEEE J. Sel. Top. Appl. Earth Observations Remote Sens. 9 (1), 212–220. doi:10.1109/jstars.2015.2424152
Nichols, P., Mccallum, A., and Lucke, T. (2017). Using ground penetrating radar to locate and categorise tree roots under urban pavements. Urban For. Urban Green. 27, 9–14. doi:10.1016/j.ufug.2017.06.019
Pierret, A., Moran, C. J., and Doussan, C. (2005). Conventional detection methodology is limiting our ability to understand the roles and functions of fine roots. New Phytol. 166, 967–980. doi:10.1111/j.1469-8137.2005.01389.x
Qureshi, A., Pariva, , Badola, R., and Hussain, S. A. (2012). A review of protocols used for assessment of carbon stock in forested landscapes. Environ. Sci. Policy 16, 81–89. doi:10.1016/j.envsci.2011.11.001
Roitman, I., Bustamante, M. M. C., Haidar, R. F., Shimbo, J. Z., Abdala, G. C., Eiten, G., et al. (2018). Optimizing biomass estimates of savanna woodland at different spatial scales in the Brazilian Cerrado: Re-evaluating allometric equations and environmental influences. PLoS ONE 13 (8), e0196742. doi:10.1371/journal.pone.0196742
Saint-André, L., Thongo M'Bou, A., Mabiala, A., Mouvondy, W., Jourdan, C., Roupsard, O., et al. (2005). Age-related equations for above and belowground biomass of a Eucalyptus hybrid in Congo. For. Ecol. Manag. 205, 199–214. doi:10.1016/j.foreco.2004.10.006
Sandmeier, K. J. (2015). REFLEXW. Version 6.0. WindowsTM 9x/NT/2000/XP/7 – a program for the processing of seismic, acoustic or electromagnetic reflection, refraction, and transmission data. Manual, 516p.
Seabold, S., and Perktold, J. S. (2010). Econometric and statistical modeling with Python. in Proc. OF the 9th PYTHON in science CONF. SCIPY.
Stover, D. B., Day, F. P., Butnor, J. R., and Drake, B. G. (2007). Effect of elevated Co-2 on coarse-root biomass in Florida scrub detected by ground-penetrating radar. Ecology 88, 1328–1334. doi:10.1890/06-0989
Taflove, A., Hagness, S. C., and Piket-May, M. (2005). Computational electromagnetics: the finite-difference time-domain methodThe Electrical Engineering Handbook. Academic Press, 629–670. doi:10.1016/B978-012170960-0/50046-3
Tanikawa, T., Hirano, Y., Dannoura, M., Yamase, K., Aono, K., Ishii, M., et al. (2013). Root orientation can affect detection accuracy of ground-penetrating radar. Plant Soil 373, 317–327. doi:10.1007/s11104-013-1798-6
Van Dam, R. L. (2014). Calibration functions for estimating soil moisture from GPR dielectric constant measurements. Commun. Soil Sci. Plant Analysis 45 (3), 392–413. doi:10.1080/00103624.2013.854805
Vianden, J., Mitja, , Weihs, U., Kuhnke, F., and Rust, S. (2010). Non-destructive tree root detection with geophysical methods in urban soils. Geophys. Res. Abstr. 12, 3963–3. Available at: https://www.researchgate.net/publication/238537657_Non-destructive_tree_root_detection_with_geophysical_methods_in_urban_soils#full-text
Wang, M., Wen, J., and Li, W. (2020). Qualitative research: the impact of root orientation on coarse roots detection using ground-penetrating radar (GPR). BioRes 15 (2), 2237–2257. doi:10.15376/biores.15.2.2237-2257
Wielopolski, L., Hendrey, G., Daniels, J., and McGuigan, M. (2000). “Imaging tree root systems in situ,” in Gpr 2000: proceedings of the eighth international conference on ground penetrating radar. Editors D. A. Noon, G. F. Stickley, and D. Longstaff (Proceedings of the Society of Photo- Optical Instrumentation). 4084.
Keywords: indirect measurement, root systems, ground penetrating radar (GPR), 3D GPR, belowground imaging
Citation: Almeida Rocha A, Borges WR, Giannoccaro Von Huelsen M, Ferreira Rodrigues de Oliveira e Sousa FR, Ramalho Maciel ST, de Almeida Rocha J and Baiocchi Jacobson TK (2024) Imaging tree root systems using ground penetrating radar (GPR) data in Brazil. Front. Earth Sci. 12:1353572. doi: 10.3389/feart.2024.1353572
Received: 13 December 2023; Accepted: 15 February 2024;
Published: 26 March 2024.
Edited by:
Marcelo Cohen, Federal University of Pará, BrazilReviewed by:
Fikret Dogru, Atatürk University, TürkiyeCopyright © 2024 Almeida Rocha, Borges, Giannoccaro Von Huelsen, Ferreira Rodrigues de Oliveira e Sousa, Ramalho Maciel, de Almeida Rocha and Baiocchi Jacobson. This is an open-access article distributed under the terms of the Creative Commons Attribution License (CC BY). The use, distribution or reproduction in other forums is permitted, provided the original author(s) and the copyright owner(s) are credited and that the original publication in this journal is cited, in accordance with accepted academic practice. No use, distribution or reproduction is permitted which does not comply with these terms.
*Correspondence: Amanda Almeida Rocha, YW1hbmRhN2tAZ21haWwuY29t
Disclaimer: All claims expressed in this article are solely those of the authors and do not necessarily represent those of their affiliated organizations, or those of the publisher, the editors and the reviewers. Any product that may be evaluated in this article or claim that may be made by its manufacturer is not guaranteed or endorsed by the publisher.
Research integrity at Frontiers
Learn more about the work of our research integrity team to safeguard the quality of each article we publish.