- 1Key Laboratory of Enhanced Oil Recovery of Carbonate Fractured-vuggy Reservoir, Sinopec, Urumqi, Xinjiang, China
- 2Exploration and Development Research Institute of Northwest Oilfield Branch of Sinopec, Urumqi, China
- 3School of Geosciences, Yangtze University, Wuhan, China
- 4State Key Laboratory of Oil and Gas Resources and Exploration, China University of Petroleum, Beijing, China
- 5Research Institute of Exploration and Development, Petro China Huabei Oilfield Company, Renqiu, China
The complexity and strong heterogeneity of carbonate reservoirs with fracture-vuggy structures present significant challenges in reservoir characterization. To address these challenges, we propose a novel multi-element information fusion modeling approach. This approach is designed to integrate multiple methods and incorporate multi-probability fusion at various facies and scales, thereby bridging the gap between geological information and reservoir modeling. Our methodology involves four key steps. First, the statistics between frequency of karst and geological information are acquired, and we quantify the statistics to regression equations. Second, these regression equations are transferred to probability bodies. The probability bodies can be applied in modeling as a soft control. But just one single body can be input in modeling process. Third, multiple probability bodies are fused into a fusion probability body by a probability fusion algorithm, which can keep the potential information of probability bodies. Finally, we apply the probability body in modeling workflow. By this way, the fusion method bridges the gap between geological information and modeling. The model established through our proposed method showed a significant level of consistency with reservoir re-evaluation, achieving an impressive 90% degree of alignment. Furthermore, the history match analysis revealed a high correlation, indicating the model's reliability. The method effectively integrates various scales and types of geological information, offering an accurate approach to complex carbonate reservoir modeling.
1 Introduction
Fracture-vuggy carbonate reservoirs, which account for a large proportion of oil and gas reserves, are important targets of oil and gas exploration and development. However, fracture-vuggy carbonate reservoirs have undergone a serious late-stage transformation, with various reservoir types and complex development laws. Among them, the main reservoir is the ancient karst fracture-vuggy system, which is more difficult to characterize than pore-type and fracture-type carbonate reservoirs (Hou et al., 2012). Therefore, we have to improve the modeling method to combine with the geological knowledge in fracture-vuggy carbonate reservoir characterization (Yang et al., 2016; Li, 2013; Yang).
At present, a series of mature modeling ideas or methods have been proposed by researchers for fracture-vuggy carbonate reservoirs, which are hereby divided into three categories: deterministic modeling methods based on interpretation results (Zhang et al., 2020; Lu et al., 2021), stochastic modeling methods based on geostatistics (Xie et al., 2023), and modeling methods based on machine learning (Suihong et al., 2022). Among deterministic modeling methods, the seismic attribute-based geological body carving method is a commonly used fracture-vuggy characterization method. In geostatistical modeling methods, the sequential indicator simulation method, for example, expresses the correlation of two points in space through a variogram function, and interpolates the underground reservoir space. Multi-point geostatistics (MPS) randomly generates data events at unevaluated points by scanning the data events in training images (Deutsch, 2002; Mariethoz et al., 2010; He et al., 2014; Wang X. et al., 2022), which is capable of generating fracture-vuggy models. On this basis, geologists have added some constraints of geological and geophysical information, including constraints on the causes of formation of fractures and faults, and constraints on seismic attributes. In this way, the quality of the stochastic models has been greatly improved. The deep learning algorithm has also been applied to carbonate modeling thanks to the development of computer hardware and software. As the most advanced image generation algorithm, the generative adversarial network can learn from a large number of training images, and the trained network can reproduce the geological model and greatly accelerate the model generation speed (Xie et al., 2022). The above mentioned modeling methods still have limitations in practical application. Despite good modeling results achieved by advanced machine learning-based modeling methods, machine learning needs a large amount of training data (Song et al., 2021a), while fracture-vuggy carbonate reservoirs have few outcrops on the ground, so it is difficult to obtain a large amount of training data. Moreover, a lot of computing resources are needed in the training process, and suitable conditions are usually unavailable in practical work. In practice, the fracture-vuggy models are still established by combining deterministic modeling methods, the two-point statistics modeling method, and the MPS method. The existing information constraint methods can introduce geological prior knowledge into the modeling process, but the focus is always on the controlling effect of a single factor on a single reservoir, while reservoirs of the same type are generally related to various factors. For example, the development of karst caves is affected by faults, denudation surfaces, etc., accompanied by relatively stable seismic response characteristics. The single facies control or probabilistic volume can no longer meet the requirements of multi-information fusion.
With the Ordovician fracture-vuggy carbonate reservoir in Area 12 of Tahe Oilfield as an example, the geological situation of the study area was introduced in Section 2; the basic principle of the proposed method was presented in Section 3; in Section 4, the discrete models of different types of reservoirs in the study area were established based on their causes of formation by combining multiple modeling methods with the multi-information probabilistic volume fusion as the soft constraint on stochastic modeling, and then fused by conforming to geological and statistical laws, thus establishing a refined geological model of this fracture-vuggy carbonate reservoir; In Section 5, the final model was subjected to oil reservoir model test.
2 Geological setting
Tahe Oilfield (Figure 1A), which is located in the south-central part of Akekule convex, Shaya uplift, Tarim Basin, adjoins Caohu depression in the east, Halahatang depression in the west, Mangar depression in the south, and Yakela-Luntai fault-convex in the north. The Ordovician carbonate formation in this oilfield is the main horizon with the development of paleokarsts and marine fracture-vuggy oil reservoirs (Zhang et al., 2004; Qiang and Fei, 2013; Liu YM. et al., 2018; Shang et al., 2020; Lu et al., 2021), being a rarely seen complex reservoir on a global scale. It has experienced multi-period tectonic movements and late karst reformation, resulting in uneven fracture-vuggy development and very strong heterogeneity. The main effective reservoir space is the ancient karst fracture-vuggy system (Qiang et al., 2015; Li et al., 2016; Liu Y. et al., 2018), which can be divided into three major classes of reservoirs according to the space size, morphology, and causes of formation, namely, underground rivers formed by fluctuating corrosion of groundwater systems (Yan-feng et al., 2021), fault-controlled karsts formed by corrosion along fault zones (Changcheng et al., 2016), and dissolution caves formed by weathering and leaching (Yang and Qiang, 2016). However, fractures contribute little to oil and gas accumulation and mainly act as seepage channels to transport oil and gas, and they can be divided into large-scale fractures, medium-scale fractures, and small-scale fractures according to their size. Among them, dissolution caves and small-scale fractures are strongly weathered and leached, which can be called weathered crust reservoirs. Underground rivers, fault-controlled karsts, and dissolution cave reservoirs store 95% of oil and gas production, thus being the focus of reservoir development.
This study area is the middle part of the Area 12 in Tahe Oilfield (Figure 1B), which is divided into four fracture-vuggy units, among which TH12208 and TH12201 are the main fracture-vuggy units (Figure 1C). There are a total of 24 development wells, including 22 vertical wells and 2 horizontal wells, with an accumulated oil production of 198 × 104 t. In addition, this area is rich in seismic data (dominant frequency: 28 Hz, sampling interval: 2 ms, group interval: 15 m) as well as well logging and drilling data, which lays a good foundation for modeling.
3 Multi-information fusion modeling method
The workflow of the multi-information fusion modeling method for fracture-vuggy carbonate reservoirs is described as follows: all the factors that affect reservoir development are considered and digitalized into probabilistic information, then multi-information is integrated by means of probabilistic fusion (Allard et al., 2012) as a constraint condition between wells in the modeling process, so that the established geological model conforms to geological information and geophysical information. According to the types and scales of reservoirs, discrete models of large-scale fractures, medium-scale fractures, underground rivers, fault-controlled karsts, dissolution caves, and small-scale fractures are established, among which dissolution caves and small-scale fractures constitute weathered crust reservoirs. Large-scale fractures are established deterministically by seismic fracture interpretation results, and medium-scale fractures are modeled deterministically based ant tracking and identification results. The underground rivers are subjected to geological body carving according to the seismic response characteristics and the geological mode of underground river outcrops. For fault-controlled karst reservoirs, faults control the developments of karsts, the control of karst by the distance from faults can be represented in a probability body. The seismic attributes indicate the distribution, we establish a probability body based on the seismic attribute. The outcrops show the geometry of the reservoir. This kind of reservoir can be modeling by object-based method to represent the geometry. The weak conditioning of object-based modeling method makes it difficult to meet the well data, and we apply it to establish a training image. Thus, the MPS method is used to combine the probability body and training image to generate the fault-controlled karst reservoirs. For dissolution caves, constraints between wells are applied according to the distance to the unconformity surface and seismic attributes, and a model is constructed through the sequential indicator method. Small-scale fractures are constrained according to the fracture development density (correlates with a seismic attribute), and simulated using the object-based method.
3.1 Large-medium fracture models
The fracture system in the study area is mainly controlled by the early Hercynian tectonic movement, along with the evident multi-level nature. Faults (large-scale fractures) constitute an important factor controlling the development of other reservoirs and also the first modeling step. Given the obvious controlling effect of the large-scale fracture on the medium-scale fracture, a large-scale fracture model was firstly established by following the principle of hierarchical modeling. Then, a medium-scale fracture model was established. Both of them were “discrete fracture network (DFN)” models, that is, the fracture distribution in the reservoir was characterized by a large number of discrete fracture pieces differing in the direction, length, shape, dip angle, and azimuth angle.
Large-scale fractures present strong seismic response and unique response laws, and reliable fracture interpretation results can be obtained through manual interpretation (Ma et al., 2014), while medium-scale fractures are controlled by large-scale fractures and have the system characteristics of large-scale fractures. The response characteristics of fractures are strengthened through coherent volume interpretation, and ant tracking technology is used to automatically identify fractures based on the attributes of seismic coherent volumes. The obtained ant body attributes can be matched, supplemented, and corrected according to the fracture system information of large-scale fractures, and the medium-scale fracture results can be acquired conforming to seismic data and geological cognition, so the discrete models of large-scale and medium-scale fractures are modeled by deterministic modeling methods.
3.2 Underground river reservoirs
An underground river system includes chamber caverns and main channel caverns, whose development, from the cause analysis, is controlled by multiple factors such as groundwater supply, fractures, water tables, and lithological differences (Popov et al., 2009; Fairchild, 2012; Li et al., 2020). Underground river karst caves show a unique development law, which is branched and banded on the plane, continuous or discontinuous, with an overall large scale, multi-layer or single-layer distribution on the cross section, and a complex structure, and filled with a large amount of collapsed breccia or sand mud. Due to the protection of cave walls, the fillings inside the caves are weakly mechanically compacted and remain good porosity (Qi and Lu, 2010), so they can be used as effective storage spaces. In the drilling process, emptying and leakage phenomena are common, the beaded seismic reflection characteristics, low resistivity, and extremely low density are manifested, and attenuation occurs frequently during imaging logging.
According to the laser scanning results (Figure 2B) of Longwang Cave (Figure 2A), Hunan Province, it is found that, different from the sand body overlapping and large-scale development of conventional rivers, underground rivers are isolated and developed on a small scale. Therefore, only a small number of wells have encountered underground river reservoirs during drilling in the study area, which leads to the scarcity of condition points, insufficient acquisition of cross-well rules, and it is difficult to obtain a convincing geological model by the stochastic modeling method. Despite the smaller scale of underground rivers than that of conventional river channels, underground rivers are different from the matrix and karst caves, and the resolution of their seismic data can meet the identification requirements. The main body of an underground river can be identified by seismic interpretation, and the identification results can be re-sampled into three-dimensional grids for deterministic modeling. Based on the principle of “well-seismic combination, geological constraint,” human-machine interactive correction was performed considering the underground river characteristics exhibited by outcrops, and an underground river model was finally established.
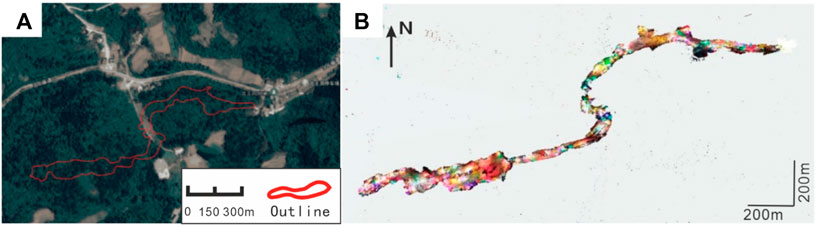
FIGURE 2. Modern underground river structure (Qinghua et al., 2019). (A) are the outline and location from the image. (B) is the laser scanning result.
3.3 Fault-controlled karst reservoirs
Fault-controlled karst reservoirs mostly develop near faults, which are formed by the vertical flow of surface water along faults. Different from underground river caves, they exist as isolated caves or cave groups in morphology, and their development is closely related to faults and fractures. On the whole, however, fault-controlled karsts are important effective reservoirs because of their strong development randomness, major changes in the scale difference, and a low filling degree. Moreover, their logging characteristics resemble those of underground rivers, but their seismic characteristics are different. Generally, the seismic characteristics of karsts extend on a small scale and exist in an isolated state.
From the cause analysis, the development of fault-controlled karst reservoirs is controlled by faults. The statistical fault-controlled karst caves in Bachu outcrop (Figure 3) can be divided into multiple types based on their morphologies, for example, circular, rectangle, ellipse, etc. The object-based modeling method is a stochastic simulation method applicable to target bodies with specific spatial forms and a modeling method suitable for fault-controlled karst reservoirs, but it fails to reflect the influence of faults on karst processes. The closer a fault-controlled karst is to the fault, the greater the karst development scale, and the higher the frequency, and different zones vary in frequency and scale constraint. Therefore, the training image was established through the multi-point geostatistics method and the object method, its spatial geometrical morphological characteristics were reserved, and the relationships of the cave height and development density of fault-controlled karsts with the distance to the fault were respectively determined. Then, multiple fault-controlled karst zones were divided on the plane according to statistical laws. Next, different scales of fault-controlled karst training images were established based on different development scales of fault-controlled karsts in different zones. Moreover, the developmental probabilistic volume based on the distance to the fault was established in line with the relationship between the distance to the fault and the development frequency. Finally, the formation causes constraint was added to the multi-point statistical modeling process by combining multiple zones, multiple training images, and the probabilistic volume, thus realizing the modeling process constrained by double formation causes.
Among them, the probabilistic volume can not only integrate cause information but also other information. For example, fault-controlled karst reservoirs can also be characterized by geophysical information, and usually, fault-controlled karsts on a certain scale present “beaded” reflection characteristics as manifested in their seismic data (Xinbian et al., 2014; Shang et al., 2020). Hence, a good correlation is observed between seismic attributes and fault-controlled karst reservoirs. However, the modeling process can only be controlled by one probabilistic volume (such as Sneism (Mariethoz and Caers, 2014)and Simpat (Strebelle, 2002)), so it is necessary to fuse multiple probabilistic volumes through a fusion algorithm, so as to obtain the probabilistic volume of multi-information fusion and realize multi-information constraints.
For the probabilistic fusion method, the permanence of ratios (PR) model (Allard et al., 2012) is selected, which has weak preconditions, considering both the independence of different data sources and the redundancy of information. This model, which is improved from the conventional probabilistic multiplication method, has been widely used in the field of earth sciences. The PR model performs multiplication of multiple probabilities, with its formula as follows:
Where F is the distance to the fault, S represents the seismic attribute, C represents fault-controlled karst, P{C│F,S} is the fusion probability of fault-controlled karst development under the multi-factor influence, P{C} is the prior probability of fault-controlled karst development, P{C│S} denotes the cave development probability under the condition of distance to the fault, and P{C|F} stands for the cave development probability under the condition of the seismic attribute.
3.4 Weathered crust reservoirs
Weathered crusts are mainly stored in dissolution caves and small-scale cracks and formed mainly due to weathering and leaching actions. In the process of surface water flowing, undercutting channels, swallow holes, etc. are formed. When water flows into soluble karst, bedding dissolution pores, cracks, and so on (dissolution caves) are generated. High-angle dissolution fractures (small-scale fractures) develop at faults, tectonic fractures, and weathered fractures. From the aspect of logging, weathered crusts are characterized by slightly low density, increased acoustic time difference, and decreased gamma ray, and, from the perspective of imaging logging, they present the obvious characteristics of dark star points, which are often densely developed with large porosity and can be used as effective reservoirs.
According to the outcrop data, dissolution caves develop under the weathering front, and according to their development causes, they are irregular pores and caves formed by dissolution, so they are controlled by the weathering denudation surface. On the whole, the dissolution caves are widely distributed, and they are distributed in a plane shape under the unconformity surface (Figure 4A). Locally, such dissolution caves randomly develop in the reservoir space without fixed morphological characteristics (Figures 4B,C) and serve as good oil-bearing reservoir space and an important oil-gas migration channel. According to their characteristics of uniform random distribution and no fixed morphology, the dissolution caves are modeled using the sequential Gaussian method, which, however, is of uncertainties. By reference to the idea of fault-controlled karsts, therefore, the cause information and geophysical information were integrated into the probabilistic volume of reservoir development, specifically as follows: 1) The closer to the top weathered crust, the more obvious the weathering and dissolution, dissolution caves develop, the distance to the weathered crust and the development probability of dissolution caves are calculated, and a causes-controlled probabilistic volume is established; 2) the areas with dense development of dissolution pores and caves show event seismic attribute response characteristics, so seismic information is correlated with dissolution caves to some extent, and a probabilistic volume controlled by seismic information can be established. Here, the aforesaid PR probability fusion model was also adopted to finally obtain a probabilistic volume with multi-information fusion.
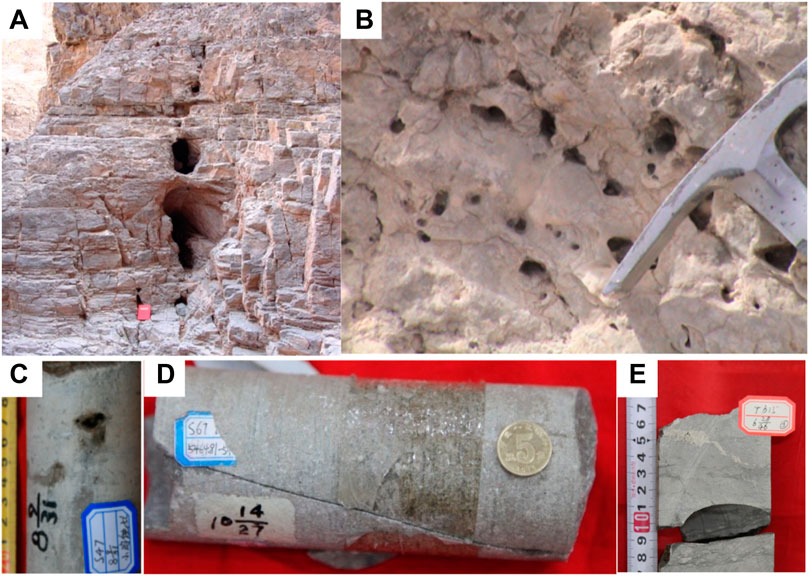
FIGURE 4. (A) shows that dissolved caves correlate the weather crusts horizon, (B) are small scale dissolved caves in a high dense, (C, D and E) are different caves in core.
Small-scale fractures are composed of tectonic fractures and dissolution fractures. The original structural fractures (Figure 4D) are often corroded and enlarged to form dissolution fractures (Figure 4E) after being corroded by groundwater. In other words, affected by large-scale faults, the zoning and orientation of such fractures are controlled by the development characteristics of the original fractures. Moreover, they are randomly distributed in the whole reservoir, and can serve as channels connecting caves, with a small scale (usually 1–5 mm wide and 5–50 cm long) and extensive development. Since the resolution of seismic data interpretation is exceeded (main seismic frequency is 28 Hz), it is impossible to obtain deterministic results from seismic information, but sections with dense fracture distribution are apparently correlated with the coherent volume attribute. Therefore, the fracture characteristics, including the fracture type, dip angle, azimuth angle, fracture development density, and other parameters, were determined by rock core and logging data, and models were established using the object simulation method under coherent volume attribute constraints.
4 Modeling of different types of karst reservoirs
4.1 Large and medium-scale fracture modeling
Through artificial interpretation and identification of seismic data, there are 6 major faults in the study area, with a fault distance of about 5–30 m, a dip angle of about 80°, and an extension length of 500–3,000 m. The distribution model of a large-scale fracture (fault) was established by the deterministic modeling method (Figure 5A).
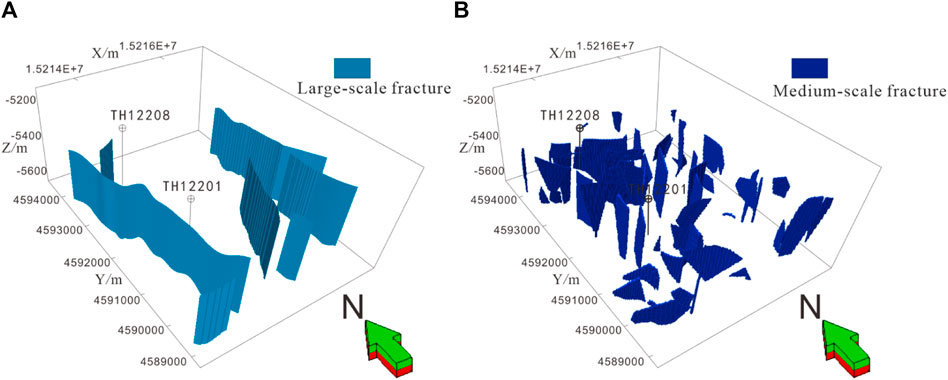
FIGURE 5. (A) is the large-scale fracture model of Area 12, (B) is the medium-scale fracture model of Area 12.
The information of fault formation systems (NE, NNW, and NS fault formation systems) could be obtained according to the interpretation results of large-scale fractures. Then, the artificially interpreted earthquake faults were supplemented and corrected using automatically extracted fracture information by means of man-machine interaction, and 36 medium-scale fractures were automatically picked up by ant bodies in the study area. Finally, a deterministic discrete distribution model of medium-scale fractures was established according to the interpretation results of ant bod (Figure 5B).
4.2 Modeling of underground river reservoirs
According to the logging data of TH12207 well (Figure 6A), the target section exhibits the obvious characteristics of underground rivers, which present a high gamma (GR) value that changes suddenly and a low resistivity (RT) value in logging, indicating serious mud filling. Moreover, evident attenuation is observed through imaging logging. Based on the correlation analysis between the plane seismic attributes and the well logging interpretation results, the correlation between the instantaneous earthquake frequency is considered high. Therefore, the instantaneous earthquake frequency attribute (Figure 6B) of the river channel was tracked starting from the underground river encountered during TH12207 well drilling, so as to obtain the plane distribution pattern of this river channel (Figure 6C). Next, a 3D prediction model of the underground river (Figure 6D) was depicted through the Geobody seismic carving method in Petrel software on basis of the wave impedance attribute. The channel distribution characteristics of the predicted underground river were clear and coincided with the characteristics of the underground river reflected by outcrop data as a whole. Furthermore, the underground river structure was corrected with the mode dependence on outcrop characteristics (Figure 2).
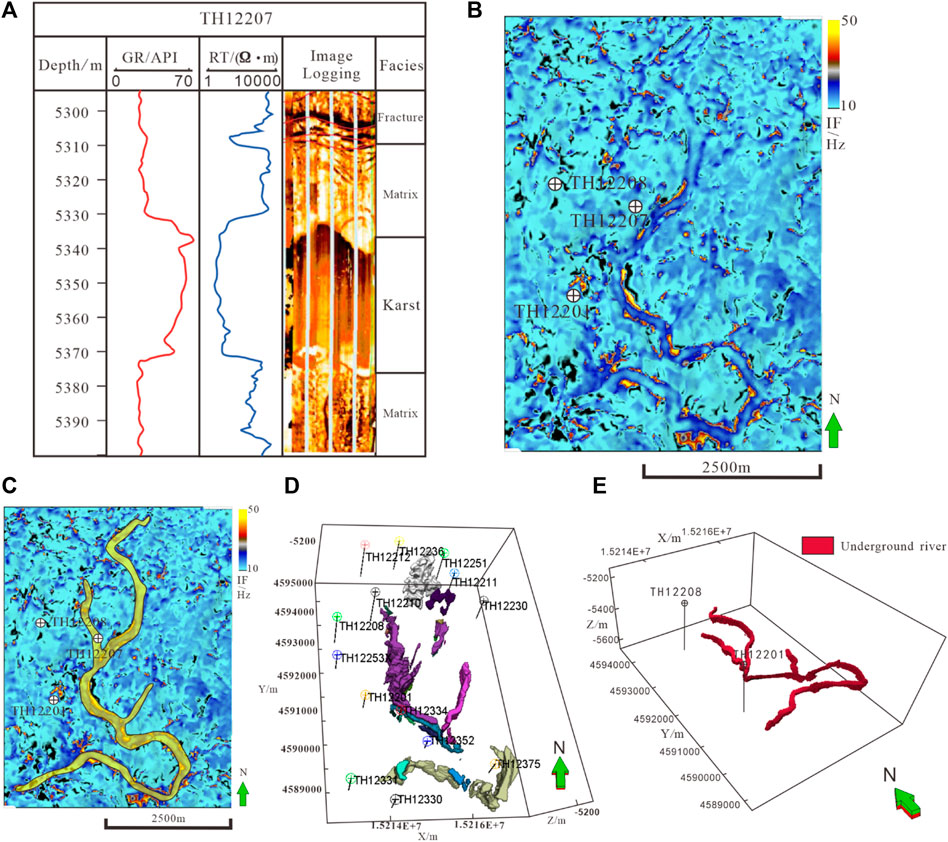
FIGURE 6. (A): the well log interpretation of Well TH12207. (B): the IF attribute slice if Area 12. (C): the boundry of IF attribute slice. (D): the geobody is a prediction based on a cut-off value and the initial seismic attribute. (E): a predicted model of underground river by manual revision.
Figure 6E shows the distribution model of underground rivers in TH12208 and TH12201 fracture-vuggy units after manual revision. The results show that the underground river develops from north to south, with a total length of about 10 km. Two small underground river branches with an average thickness of about 30 m develop on both sides of the main underground river. Besides, the underground river is high in the south and low in the middle.
4.3 Modeling of fault-controlled karst reservoirs
According to the logging information and drilling leakage information, fault-controlled karsts can be identified, with a height of 2–16 m and a length and width of 2–10 m. Based on the outcrop shape, the target body was established, and three zones were established according to the distance to the fault and the frequency range of karst development: 0–80 m from the fault, 80–250 m from the fault, and more than 250 m from the fault. Next, three training images were stablished through the object method to characterize the scale and frequency of different karst bodies. Afterward, and formation cause mechanism of faults was integrated into the training images so that the modeling process could be simulated synchronously in different zones.
The corresponding training images were used for multi-point geostatistical simulation in different zones. However, the three training images could not reflect the gradual relationship between karst development and faults, earthquake constraint and fault distance constraint were required. The fault distance constraint was input in the form of probabilistic volumes. Among 26 karst reservoirs identified through well logging, those within 400 m range from the fault accounted for over 70%, while a few karst reservoirs were distant from the fault. Accordingly, the relationship between fault-controlled karsts and the distance to the fault could be acquired. Then, based on this, the probability formula between karst caves and the distance to the fault was solved (Figure 6A), and the probabilistic volume of karst development controlled by the corresponding fault was established (Figure 7A). The specific process of earthquake constraint was described as follows: after time-depth conversion of seismic data, the above-ground karst and seismic attributes were determined based on the drilling leakage results and the corresponding seismic attributes. The results show that the seismic spectrum energy in seismic attributes is favorably correlated with fault-controlled karsts. The functional relationship between seismic spectrum energy and fault-controlled karst development frequency was obtained through the neural network algorithm (Figure 7B), and the corresponding karst development probabilistic volumes were established on this basis (Figure 7D). Finally, the two probabilistic volumes were fused by the probabilistic multiplication method (Formula 1) to obtain a comprehensive probabilistic volume (Figure 7E).
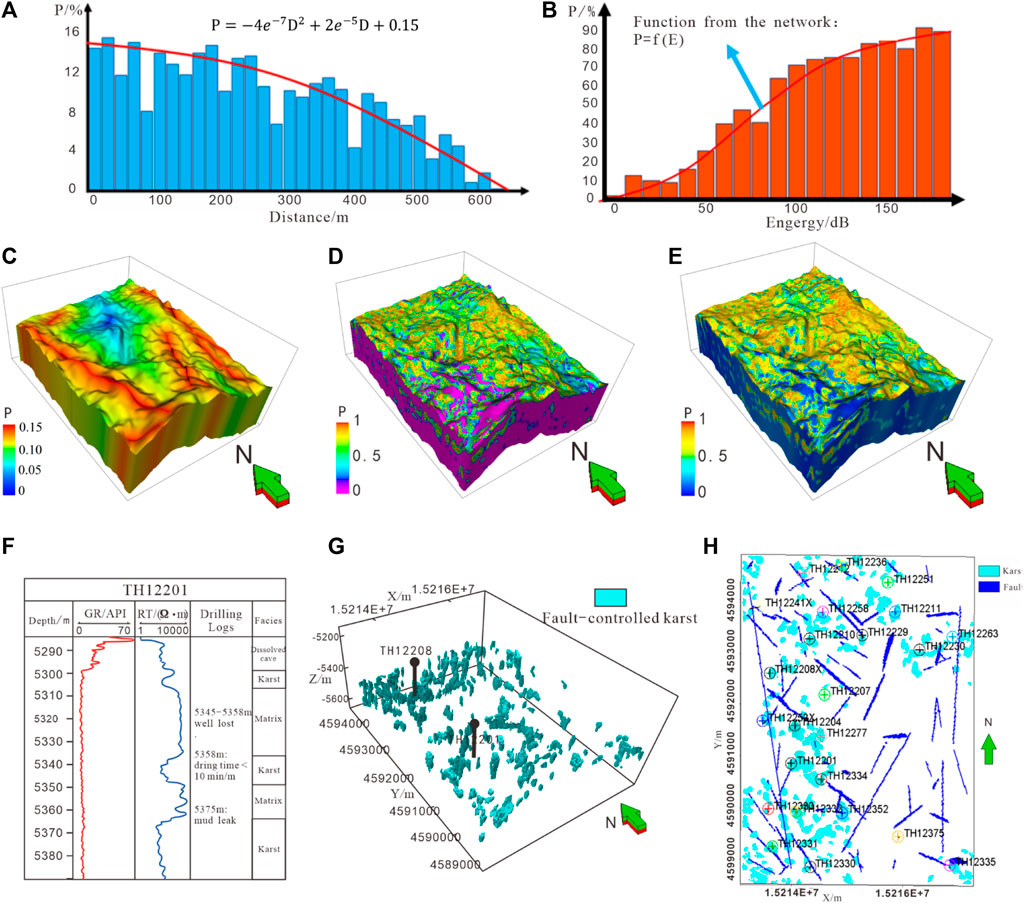
FIGURE 7. (A, B) represent the correlation analysis of developed probability-distance from fault and developed probability-energy seismic attribute. (C, D) are probability model from the correlation of fig 7A and 7B. The (E) is a fused model of fig 7C and 7D. The (F) is a interpretation result of fault-controlled karst in Well TH12201, based on this result, the karst model is simulated by geostatistical modeling method. the top-view map is shown as (H).
Under the constraint of comprehensive probabilistic volume and training images and taking the well point interpretation results (Figure 7F) as the conditional data, the multi-point simulation scanning template was set to 15 × 15 × 3, the multi-grid level was set to 3, and the maximum number of known neighborhood nodes was 8. Three corresponding training images were input in three zones, and the fault-controlled karst model with formation cause mechanism and karst geometry (Figure 7G) was finally obtained by combining the boundary control of fracture-vuggy units. The results show that fault-controlled karst reservoirs were mainly distributed near the NNW major fault in the west of TH12208 and TH12201 fracture-vuggy units, with the cave size ranging from 8 m to 30 m, mainly being vertical and horizontal types, and the closer to the fault, the larger the cave size, and with the increase in the distance to the fault, the cave morphology gradually transits from the vertical type to the horizontal type. It is worth noting that according to the production dynamics data of the oilfield, the effective reservoirs do not develop outside the fracture-vuggy units. To conform to the actual production situation, the fracture-vuggy unit boundary was adopted for control, so the reservoirs were mainly controlled by the large faults in the west (Figure 7H), while the northeastern faults generated a minor influence on the karst body, with a low karst development efficiency, which accorded with the actual situation of effective reservoirs not encountered during well drilling in this area.
4.4 Modeling of weathered crust reservoirs
Dissolution caves and small-scale fractures constitute the weathered crust reservoir, which is the communication channel between the caves. According to the core and imaging logging results (Figure 8A), the small-scale fractures are mainly high-angle tectonic shear fractures, with the extension length mainly distributed in 0.5–50 cm; based on the fracture occurrence statistics of imaging logging in the study area (Figure 8B), there are three fracture formation systems in the study area, including NE, NW, and NS. The fracture development density is established through the statistical dip angle and fracture development frequency (Figures 8C,D). Different well locations display different azimuth angles and development frequencies, and the dip angle of fractures in the study area is mainly 75°–90°. Based on the statistical parameters and development density of small-scale fractures, the discrete model of small-scale fractures was established by the object method under constraints of coherent volume attributes and probabilistic volumes (Figure 9G).
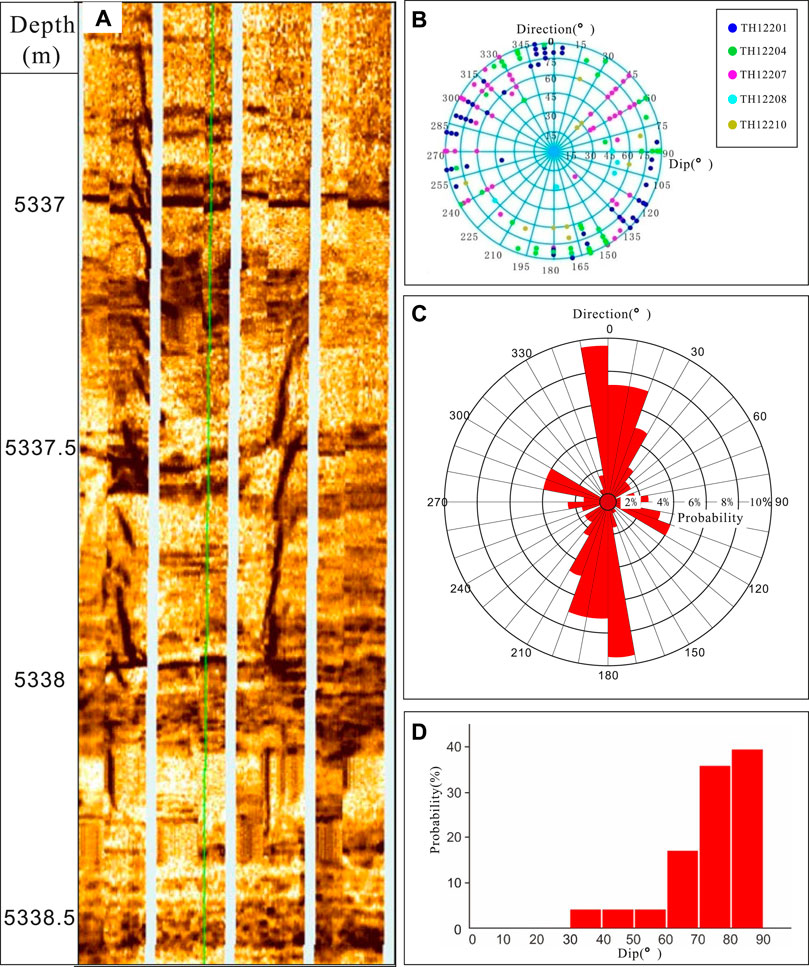
FIGURE 8. Small-scale fractures occurrence and density statistics. (A) is an example of image logging. Based on logging data, the statistics of fractures direction distribution and density are acquired in (B, C, D).
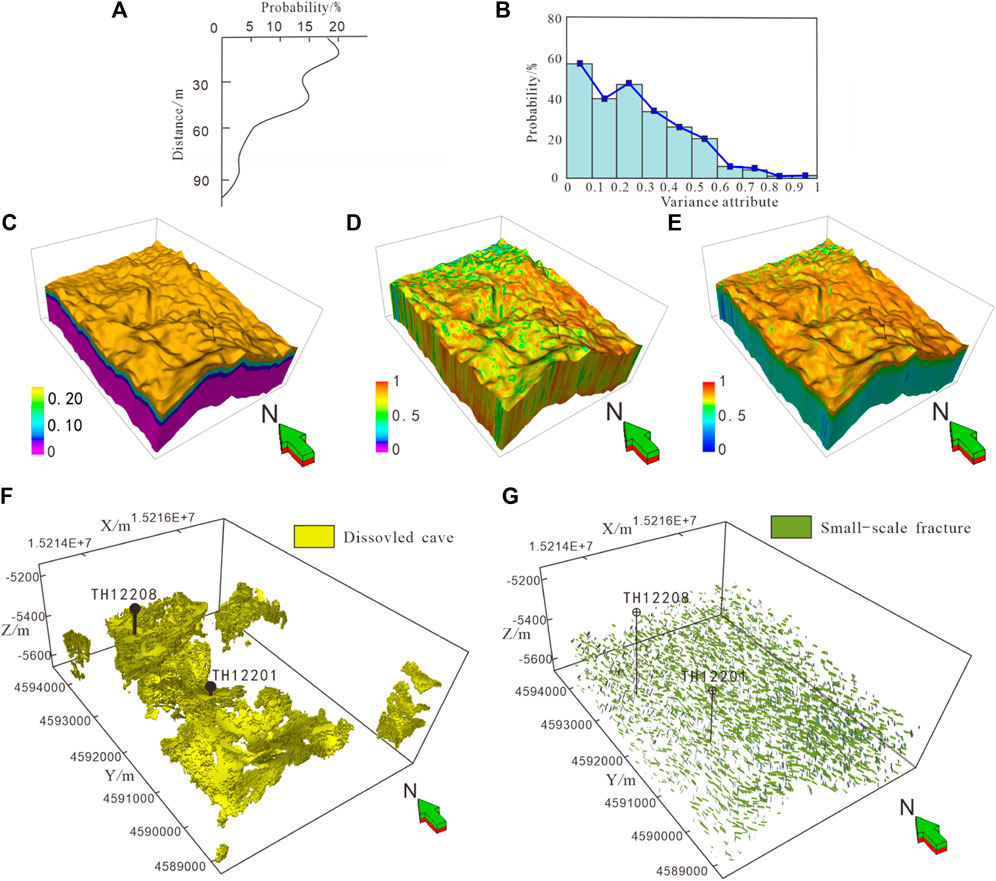
FIGURE 9. (A, B) are correlations of dissolved fracture probability-distance from the crust horizon and dissolved fracture probability-variance attribute, The probability model (C, D) are generated base on A, B. The figure 9C is a fused probability model by PR model. The (F) is a dissolved cave and (G) is a small-scale fracture model.
According to the well data, a total of 11 development sections of dissolution caves were identified in 7 wells of fracture-vuggy units TH12208 and TH12201, among which the development frequency of those within 30 m from the unconformity surface was 42% and that within 30 m from the unconformity surface was 58%. The development sections of dissolution caves mainly developed near 90 m range of the Ordovician unconformity surface. Based on the statistical results, the depth of the top unconformity surface and the vertical development probability of dissolution caves could be obtained (Figure 9A), and a development probabilistic volume was established accordingly (Figure 9C). The developmental probabilistic volume (9d) based on seismic attributes could be established given the high correlation between the coherent volume attribute in seismic attributes and the dissolution caves (9b). The above two probabilistic volumes were subjected to probabilistic fusion to obtain a comprehensive development probabilistic volume of dissolution caves (9e).
The relevant parameters in the sequential indicator simulation modeling were set as follows: the main variation direction of the variogram function was 330° with a variation range of 2,000 m, the secondary variation direction was 240° with a variation range of 1,500 m, and the vertical variation range was 10 m. An exponential model was used as the variogram function model. Then, simulation was performed using the sequential indicator simulation method with trends, and a dissolution cave reservoir model was acquired with the probabilistic volume of dissolution cave development between wells as the constraint data (Figure 9F). From the established dissolution cave reservoir model, dissolution cave reservoirs presented a local flaky distribution and mainly developed within a certain depth range beneath the Ordovician unconformity surface.
Finally, the dissolution cave model and small-scale fracture model were superposed to acquire a weathered crust distribution model in the study area (Figure 9H).
4.5 Model fusion optimization
Because the discrete distribution models of underground rivers, fault-controlled karsts, and dissolution cave reservoirs and multi-scale fracture models were established independently, and the stochastic modeling method was used in both reservoir and small-scale fracture modeling, the same grid might be used as an effective grid in different models when all discrete distribution models were fused, but this was inconsistent with the actual geological conditions. To solve the conflict among reservoirs characterized by grids, a certain fusion principle was put forward.
(1) From a reservoir capacity and model uncertainty standpoint, underground rivers and fault-controlled karst reservoirs possess robust reservoir capacities, contributing significantly to cumulative output. However, underground rivers present greater uncertainty than fault-controlled karst reservoirs, warranting a higher prioritization. Conversely, weathered crust reservoirs, characterized by minute fractures and dissolution caves, exhibit the poorest physical properties and highest randomness among effective reservoirs, thus receiving the lowest priority. Regarding fractures, large-scale fractures (faults) are delineated through deterministic interpretation, medium-scale fractures via a blend of random extraction and human-computer interaction, and small-scale fractures through stochastic simulation. Consequently, the prioritization for integration is large-scale fractures first, followed by medium and small-scale fractures, respectively. In the final stage, small-scale fractures, medium-scale fractures, large-scale fractures, dissolution caves, fault-controlled karst, and underground rivers are sequentially incorporated into the study area’s three-dimensional space. In overlapping zones, reservoirs assigned later will be preserved.
(2) The relationship between fractures and caves in reservoir configurations can generally be categorized into three types: karst cave-large fracture, karst cave-fracture-karst cave, and dissolution cave-small fracture. Separate models for karst caves and fractures are developed based on their respective geological model constraints and statistical laws. However, during the fusion process, the specific fracture-karst cave configuration pattern is not taken into account, except for the statistical law constraint applied to the fracture model. To address this, the fracture-vuggy relationship is fine-tuned using the simulated annealing method (Xie et al., 2022). The simulated annealing method is a probabilistic technique used for finding an approximate solution to an optimization problem, particularly those involving a large search space. It's inspired by the process of annealing in metallurgy, a technique involving heating and controlled cooling of a material to increase the size of its crystals and reduce their defects. This adjustment involves altering the orientation and position of fractures (as illustrated in Figure 10A), which enhances reservoir connectivity in a manner that aligns with dynamic data. Ultimately, the fracture model that best conforms to the fracture-vuggy configuration relationship is preferentially selected.
(3) Probabilistic similarity, in the context of geological modeling, refers to ensuring that the final reservoir model accurately represents the statistical characteristics of the actual geological formations. After initial adjustments to models (as per steps 1 and 2), the original karst or dissolution cave reservoir model might be significantly altered when fused with other reservoir models, leading to substantial changes. This can result in the volume proportions of various reservoirs in the new model not aligning with the original statistical data.
After the above three operation steps are completed, the fusion model of karst, fracture, and cave reservoirs in the study area is finally obtained (Figure 10), in which underground rivers are continuously distributed, fault-controlled karsts mainly develop near faults, and dissolution caves and small-scale fractures (weathered crust) are widely developed as connecting channels. The development characteristics of fracture-vuggy units are well reflected by this fusion model.
5 Model test
Based on the final fusion geological model, the oil reservoir reserves in the study area are calculated as 1,507 × 104 t. Therein, the reserves of underground rivers, fault-controlled karsts, and weathered crusts are 417 × 104 t, 479 × 104 t, and 310 × 104 t, respectively, among which the reserves of dissolution caves, small-scale fractures, and large and medium-scale fracture models are 302 × 104 t, 8 × 104 t, and 1 × 104 t, respectively. By comparing the well-controlled reserves and production dynamic reserves of the model (Figures 11A,B), it can be seen that the calculation results of reserves of each well in TH12201 and TH12208 fracture-vuggy unit models basically accord with the actual recalculation results of single-well dynamic reserves, which indirectly verifies the effectiveness of the model.
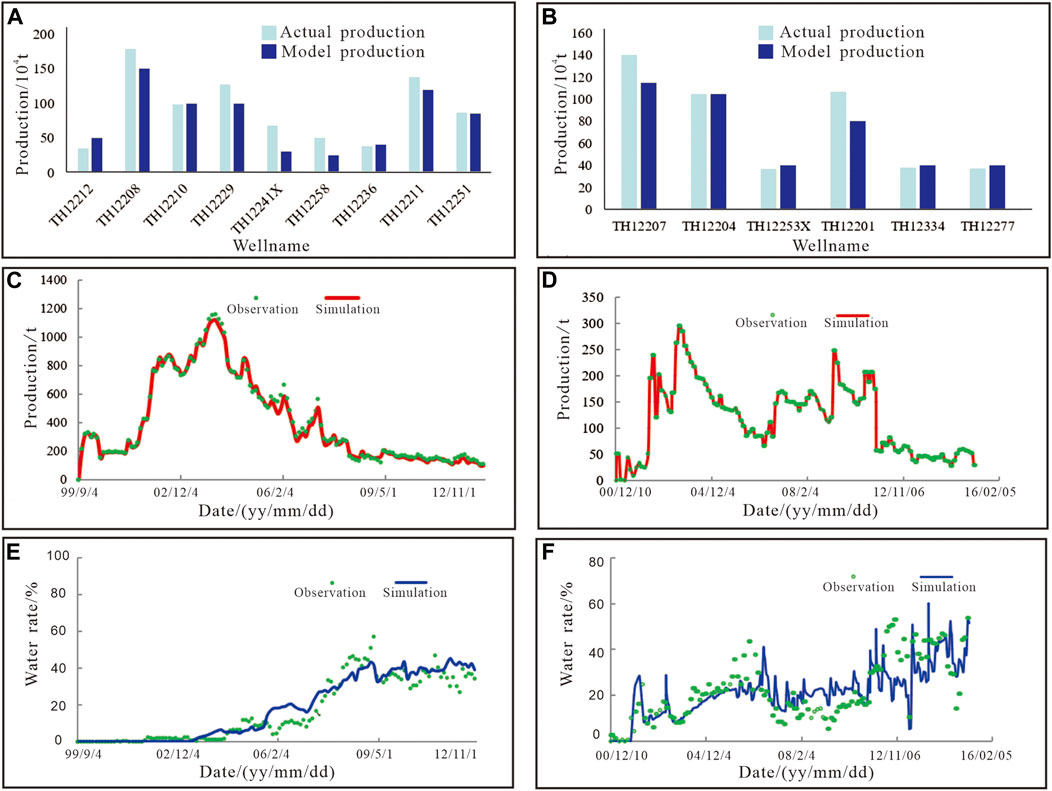
FIGURE 11. (A, B) are comparations of real production oil and model reserves in units TH12201, TH12208. (C-F) are correlation analysis of the simulated oil production and water cut in TH12201, TH12208 unit.
On the basis of the three-dimensional geological model, the numerical simulation was carried out for the oil reservoirs in fracture-vuggy units TH12201 and TH12208 (Figures 11C–F), and the coincidence rate of both the simulated oil production and water cut was greater than 90%. The model simulation results showed a high degree of fitting with the actual production results, proving the high precision of the geological model and according with the actual underground situation, and the favorable position of the remaining oil distribution could be determined. Based on this model, an infill well has been implemented in the unit TH12201, the average daily oil production reaches 45 t, the cumulative oil production up to now is 7.167 × 104 t, and only 0.079 t of water is produced.
6 Discussion
In this paper, we introduced a fracture-vuggy modeling method that leverages multiple geological data points, treating these pieces of geological information as probability models within our modeling approach. Probability models enable us to mathematically constrain the modeling scope, reducing uncertainties in the geological modeling process. However, it's important to note that reservoir behavior is influenced by a multitude of factors. To address this complexity, our approach fuses these factors using a PR model, allowing us to overcome the limitations associated with models controlled by a single probability parameter. Undoubtedly, our method represents significant progress in improving model quality. Yet, challenges persist in its practical application. In this process, all information is utilized as soft data (probability models), and the accuracy of simulation is constrained by correlation relationships. When there are insufficient sample points to establish robust correlation coefficients, our method may introduce high uncertainty in the soft data. The reservoir is influenced by many geological factors, for example, the rock physical property decides the distribution karst, the stronger fragility of rock, the easier generation of karst or fracture. Thus, more and more information need to be added in limit factors. It makes difficult to keep the potential information of so many data. Maybe we need a better fusion algorithm to solve this problem. For example, neural network algorithms can get the optional solution by many times iteration. The solution can extract the non-linear relation between probability bodies and keep principal components of multiple factors, which may improve the quality of fusion.
The reservoir is influenced by numerous geological factors. For instance, the physical properties of the rock determine the distribution of karst; the more fragile the rock, the more susceptible it is to the formation of karst or fractures. Consequently, there is a growing need to include additional limiting factors, which poses challenges in preserving the potential information contained in this wealth of data. Perhaps a more effective fusion algorithm is required to address this issue. For example, neural network algorithms can iteratively generate an optimal solution (Dorrington and Link, 2004; Li et al., 2019). The solution have the capacity to extract nonlinear relationships among probability models and retain the principal components of multiple factors, potentially enhancing the quality of fusion.
Fracture-vuggy reservoirs exhibit pronounced heterogeneity and non-stationarity. In this context, we generate karst reservoirs using the MPS modeling method. MPS modeling involves pattern extraction from scanned data events, essentially reproducing patterns by copying and pasting (Song et al., 2021b; Wang L. et al., 2022; Chen et al., 2022). Clearly, characterizing the non-stationarity of fracture-vuggy reservoirs through MPS modeling is challenging. To capture global information, we must explore methods for extracting potential data from the training image. Machine learning methods have proven to be at the forefront of pattern recognition, making machine learning algorithms a viable solution for uncovering abstract geological features. This approach represents a novel way to incorporate abstract geological knowledge into the modeling process. For instance, geologists (Mosser et al., 2018; Zhang et al., 2019; Song et al., 2022; Liao et al., 2023) have successfully applied generative adversarial networks (GANs), a generative model algorithm, to learn geological patterns and reproduce them in geological modeling. Researchers have used seismic data and sand fraction data to control channel geometry and the number of channels. By combining the strengths of seismic inversion and machine learning methods, we can harness abstract geological knowledge to characterize the structure of underground rivers and fault-controlled karst formations. When apply the machine learning method to modeling works, the challenges we may face include enough and convincing training data, the computing hardware and computer knowledge.
7 Conclusion
The modeling of carbonate reservoirs with fracture-vuggy structures, characterized by diverse storage types with varied origins, shapes, and sizes, requires a nuanced approach. Initially, the modeling process involves categorizing and classifying the different storage types. Based on the characteristics of each storage type, suitable methods are selected: object or multipoint statistical methods for storage bodies with specific forms and random distributions, sequential indicator simulation methods for those with non-specific forms and random distributions, and deterministic methods for specific geological bodies. The integration of geological and geophysical information through multi-probability body fusion maximizes the contributions of different data types to the model, enhancing multi-information driving, reducing uncertainty, and resulting in a refined 3D geological model. This model adheres to three fusion principles: isotope priority for hierarchical fusion based on causal relationships, fracture relationship configuration with iterative correction using simulated annealing, and probability similarity for volume adjustments to match statistical patterns. Validated through numerical simulation and drilling tests, this model effectively informs adjustments in development plans for such complex reservoirs.
Data availability statement
The original contributions presented in the study are included in the article/Supplementary material, further inquiries can be directed to the corresponding author.
Author contributions
YW: Writing–review and editing, Funding acquisition. PX: Writing–original draft, Writing–review and editing. HZ: Data curation, Formal Analysis, Writing–review and editing. YL: Funding acquisition, Writing–review and editing. AY: Visualization, Writing–review and editing.
Funding
The author(s) declare financial support was received for the research, authorship, and/or publication of this article. This research was funded by Sinopec Key Laboratory Open Fund Project for Enhancing Recovery in Fractured Reservoirs, grant number 34400000-22-ZC0607-0002. This research was supported by Key Laboratory for EOR of Fractured-Vuggy Carbonate Reservoir and National Natural Science Foundation of China (No. 42172154).
Conflict of interest
The authors YW and HZ were employed by the company Sinopec. The author AY was employed by the Petro China Huabei Oilfield Company.
The remaining authors declare that the research was conducted in the absence of any commercial or financial relationships that could be construed as a potential conflict of interest.
The study received funding from Sinopec Key Laboratory Open Fund Project for Enhancing Recovery in Fractured Reservoirs. The funder had the following involvement in the study: study design.
Publisher’s note
All claims expressed in this article are solely those of the authors and do not necessarily represent those of their affiliated organizations, or those of the publisher, the editors and the reviewers. Any product that may be evaluated in this article, or claim that may be made by its manufacturer, is not guaranteed or endorsed by the publisher.
References
Allard, D., Comunian, A., and Renard, P. (2012). Probability aggregation methods in geoscience. Math. Geosci. 44 (5), 545–581. doi:10.1007/s11004-012-9396-3
Changcheng, H., Chengyan, L., Xinbian, L., Lihua, R., Ting, W., Xianguo, Z., et al. (2016). Characterization and genesis of fault-controlled karst reservoirs in ordovician carbonate karst slope of tahe oilfield, tarim basin. Oil Gas Geol. 37 (5), 644–652. doi:10.11743/ogg20160504
Chen, M., Wu, S., and Bedle, H. (2022). Modeling of subsurface sedimentary facies using self-attention generative adversarial networks (SAGANs). J. Petroleum Sci. Eng., 214. doi:10.1016/j.petrol.2022.110470
Dorrington, K. P., and Link, C. A. (2004). Genetic-algorithm/neural-network approach to seismic attribute selection for well-log prediction. GEOPHYSICS 69 (1), 212–221. doi:10.1190/1.1649389
He, X. L., Sonnenborg, T. O., Jørgensen, F., and Jensen, K. H. (2014). The effect of training image and secondary data integration with multiple-point geostatistics in groundwater modelling. Hydrology earth Syst. Sci. 18 (8), 2943–2954. doi:10.5194/hess-18-2943-2014
Hou, J.-G., Jiang, X. M., and Yu, m. (2012). Modeling of carbonate fracture-vuggy reservoir, a case study of Ordovician reservoir of 4th Block in Tahe Oilfield. Earth Sci. Front. 19 (02), 59–66.
Li, S., Kang, Z., Feng, X. T., Pan, Z., Huang, X., and Zhang, D. (2020). Three dimensional hydrochemical model for dissolutional growth of fractures in karst aquifers. Water Resour. Res. 56 (3), e2019WR025631. doi:10.1029/2019wr025631
Li, W., Yue, D., Wang, W., Wang, W., Wu, S., Li, J., et al. (2019). Fusing multiple frequency-decomposed seismic attributes with machine learning for thickness prediction and sedimentary facies interpretation in fluvial reservoirs. J. Petroleum Sci. Eng. 177, 1087–1102. doi:10.1016/j.petrol.2019.03.017
Li, Y. (2013). The theory and method for development of carbonate fracture-cavity reservoir in Tahe oilfield. Acta pet. Sin. 34 (01), 115–121. doi:10.7623/syxb201301013
Li, Y., Hou, J., and Ma, X. (2016). Data integration in characterizing a fracture-cavity reservoir, Tahe oilfield, Tarim basin, China. Arabian J. Geosciences 9 (8), 532. doi:10.1007/s12517-016-2562-z
Liao, B., Wang, J., Sun, J., Lv, K., Liu, L., Wang, Q., et al. (2023). Microscopic insights into synergism effect of different hydrate inhibitors on methane hydrate formation: experiments and molecular dynamics simulations. Fuel 340, 127488. doi:10.1016/j.fuel.2023.127488
Liu, Y., Hou, J., Li, Y., Dong, Y., Ma, X., and Wang, X. (2018b). Characterization of architectural elements of ordovician fractured-cavernous carbonate reservoirs, Tahe oilfield, China. J. Geol. Soc. India 91 (3), 315–322. doi:10.1007/s12594-018-0856-3
Liu, Y. M., Hou, J. G., and Li, Y. Q. (2018a). A multi-constrained modeling method for paleokarst carbonate reservoirs: an application to the Ordovician reservoir in the Tahe Oilfield. Petroleum Sci. Bull. 3 (02), 125–133.
Lu, X. R., Sun, J. F., and Wu, X. W. (2021). Internal architecture characterization of fractured-vuggy carbonate reservoirs: a case study on the Ordovician reservoirs, Tahe Unit S67, Tarim Basin. OIL GAS Geol. 42 (03), 728–737.
Ma, L. J., Kong, Q. Y., and Liu, K. Y. (2014). Weak reflection characteristics and its formation mechanism of rdovician reservoir in Tahe Oilfield. OPG 49 (02), 338–343 222.
Mariethoz, G., and Caers, J. (2014). Multiple-point geostatistics: stochastic modeling with training images.
Mariethoz, G., Renard, P., and Straubhaar, J. (2010). The Direct Sampling method to perform multiple-point geostatistical simulations. Water Resour. Res. - WATER RESOUR RES 46 (11). doi:10.1029/2008wr007621
Mosser, L., Olivier, D., and Martin, J. B. (2018). Conditioning of three-dimensional generative adversarial networks for pore and reservoir-scale models. 80th EAGE Conference and Exhibition 2018, 1–5. doi:10.3997/2214-4609.201800774
Popov, P., Qin, G., Bi, L., Enfendiev, Y., Kang, Z., Li, J., et al. (2009). Multiphysics and Multiscale Methods for Modeling Fluid Flow Through Naturally Fractured Carbonate Karst Reservoirs. Spe Technical Conference and Exhibition 12, 218–231. doi:10.2118/105378-MS
Qi, L., and Lu, Y. (2010). Development characteristics and main controlling factors of the Ordovician carbonate karst in Tahe oilfield. Oil Gas Geol. 31 (1), 1–12. doi:10.11743/ogg20100101
Qiang, J., and Fei, T. (2013). Investigation of fracture-cave constructions of karsted cabonate reservoirs of ordovician in Tahe oilfield, Tarim Basin. J. China Univ. Petroleum Ed. Nat. Sci. 37 (5), 15–21. doi:10.3969/j.issn.1673-5005.2013.05.003
Qiang, J., Fei, T., and Hongfang, Z. (2015). Comprehensive evaluation of fracture-cave units in karst carbonates in Tahe Oilfield, Tarim Basin. Petroleum Geol. Exp. 37 (3), 272–279. doi:10.7603/s40972-015-0042-2
Qinghua, C., Ming, C., and Du, H. (2019). Application of 3D digital outcrop characterization technology based on laser scanning in modern karst research. Henan Sci. 37 (3), 422–428. doi:10.3969/j.issn.1004-3918.2019.03.017
Shang, X. F., Duan, T. Z., and Zhang, W. B. (2020). Characterization of dissolution facies belt in fracture-cavity carbonate rocks mainly controlled by fault-controlling karst: a case study of Ordovician reservoirs in the Block 10 of Tahe oilfield. ACTA PET. SIN. 41 (03), 329–341. doi:10.7623/syxb202003007
Song, S., Mukerji, T., and Hou, J. (2021a). GANSim: conditional facies simulation using an improved progressive growing of generative adversarial networks (GANs). Math. Geosci. 53 (7), 1413–1444. doi:10.1007/s11004-021-09934-0
Song, S., Mukerji, T., and Hou, J. (2021b). Geological Facies modeling based on progressive growing of generative adversarial networks (GANs). Comput. Geosci. 25 (3), 1251–1273. doi:10.1007/s10596-021-10059-w
Song, S., Zhang, D., and Mukerji, T. (2022). GANSim-surrogate: an integrated framework for conditional geomodelling and uncertainty analysis. J. Hydrology 620B, 129493. doi:10.1016/j.jhydrol.2023.129493
Strebelle, S. (2002). Conditional simulation of complex geological structures using multiple-point statistics. Math. Geol. 34, 1–21. doi:10.1023/a:1014009426274
Suihong, S., Yanqing, S., and Jiagen, H. (2020). Review of a generative adversarial networks (GANs)-based geomodel-ling method. Petroleum Sci. Bull. 7 (1), 34–49. doi:10.3969/j.issn.2096-1693.2022.01.004
Wang, L., Yin, Y., Zhang, C., Feng, W., Li, G., Chen, Q., et al. (2022b). A MPS-based novel method of reconstructing 3D reservoir models from 2D images using seismic constraints. J. Petroleum Sci. Eng. 209, 109974. doi:10.1016/j.petrol.2021.109974
Wang, X., Yu, S., Li, S., and Zhang, N. (2022a). Two parameter optimization methods of multi-point geostatistics. J. Petroleum Sci-ence Eng. 208, 109724. doi:10.1016/j.petrol.2021.109724
Xie, P., Hou, J., Yin, Y., Chen, Z., Chen, M., and Wang, L. (2022). Seismic inverse modeling method based on generative adversarial networks. J. Petroleum Sci. Eng. 215, 110652. doi:10.1016/j.petrol.2022.110652
Xie, P. F., Hou, J. G., and Wang, Y. (2023). Application of multi-information fusion modeling of fracture-vuggy reservoir in Ordovician reservoir of 12th block in Tahe Oilfield. J. China Univ. Petroleum Ed. Nat. Sci. 47 (03), 1–14. doi:10.3969/j.issn.1673-5005.2023.03.001
Xinbian, L., Chengjiang, H., and Guangxiao, D. (2014). Development features of karst ancient river system in Ordovician reservoirs, Tahe Oil Field. Petroleum Geol. Exp. 3, 268–274. doi:10.11781/sysydz201403268
Yan-feng, L., Wen-biao, Z., Tai-zhong, D., and Hua-wei, Z. (2021). Numerical simulation method for the formation of fault-karst carbonate reservoir process based on cellular automaton. Sci. Technol. Eng. 21 (9), 3550–3555. doi:10.3969/j.issn.1671-1815.2021.09.017
Yang, L., and Qiang, J. (2016). Karst zonings and fracture-cave structure characteristics of Ordovician reservoirs in Tahe oilfieid, Tarim Basin. Acta Pet. Sin. 37 (3), 289–298. doi:10.7623/syxb201603001
Yang, L. I. (2012). Ordovician carbonate fracture-cavity reservoirs identification and quantitative characterization in Tahe Oilfield. J. China Univ. Petroleum Ed. Nat. Sci. 36 (1), 1–7. doi:10.3969/j.issn.1673-5005.2012.01.001
Yang, L. I., Jiagen, H., and Yongqiang, L. I. (2016). Features and hierarchical modeling of carbonate fracture-cavity reservoirs. Petroleum Explor. Dev. 43 (4), 600–606. doi:10.11698/PED.2016.04.12
Zhang, S., Jin, Q., and Cheng, F. Q. (2020). Genesis relation of surface and underground rivers and reservoir charac⁃ teristics in paleokarst drainage systems:A case study of Ordovician karst in the Tahe oilfield. CARSOLOGICA Sin. 39 (06), 900–910. doi:10.11932/karst2020y37
Zhang, T. F., Peter, T., Dupont, E., Zhu, L. C., Liang, L., and Bailey, W. (2019). Generating geologically realistic 3D reservoir facies models using deep learning of sedimentary architecture with generative adversarial networks. Petroleum Sci. 16 (3), 541–549. doi:10.1007/s12182-019-0328-4
Keywords: carbonate reservoir, Fractured-vuggy reservoir, geological modeling, probability fusion, fracture
Citation: Wang Y, Xie P, Zhang H, Liu Y and Yang A (2024) Fracture-vuggy carbonate reservoir characterization based on multiple geological information fusion. Front. Earth Sci. 11:1345028. doi: 10.3389/feart.2023.1345028
Received: 27 November 2023; Accepted: 21 December 2023;
Published: 11 January 2024.
Edited by:
Wenguang Wang, Northeast Petroleum University, ChinaReviewed by:
Muming Wang, University of Calgary, CanadaYuping Wu, Xi’an Shiyou University, China
Bo Liao, China University of Petroleum (East China), China
Copyright © 2024 Wang, Xie, Zhang, Liu and Yang. This is an open-access article distributed under the terms of the Creative Commons Attribution License (CC BY). The use, distribution or reproduction in other forums is permitted, provided the original author(s) and the copyright owner(s) are credited and that the original publication in this journal is cited, in accordance with accepted academic practice. No use, distribution or reproduction is permitted which does not comply with these terms.
*Correspondence: Pengfei Xie, xie.pengfei@ucalagry.ca