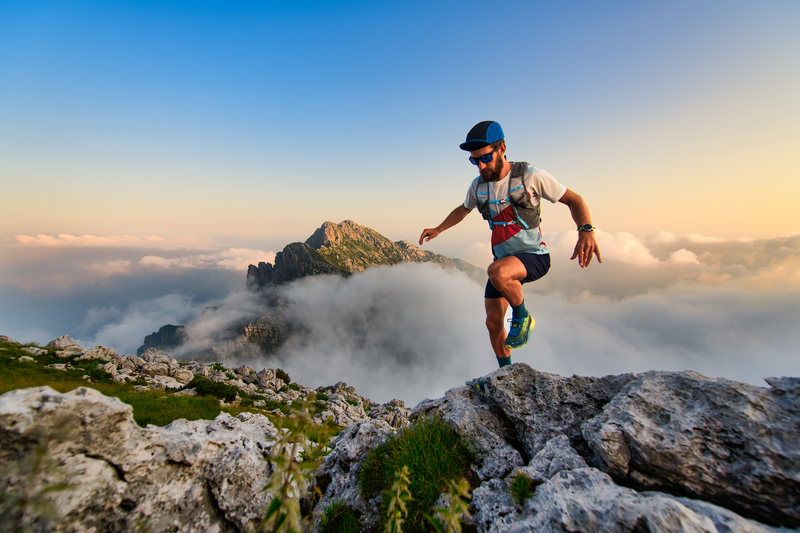
94% of researchers rate our articles as excellent or good
Learn more about the work of our research integrity team to safeguard the quality of each article we publish.
Find out more
REVIEW article
Front. Earth Sci. , 25 January 2024
Sec. Environmental Informatics and Remote Sensing
Volume 11 - 2023 | https://doi.org/10.3389/feart.2023.1230973
Geosciences modelling and 3D geovisualization is growing and evolving rapidly. Driven by commercial urgency and an increase in data from sensor-based sources, there is an abundance of opportunities to analyze geosciences data in 3D and 4D. Geosciences modelling is developing in GIS based systems, 3D modelling through both game engines and custom programs, and the use of extended reality to further interact with data. The key limitations that are currently prevalent in 3D geovisualization in the geosciences are GIS representations having difficulty displaying 3D data and undergoing translations to pseudo-3D, thus losing fidelity, financial and personnel capital, processing issues with the terabytes worth of data and limited computing, digital occlusion and spatial interpretation challenges with users, and matching and alignment of 3D points. The future of 3D geovisualization lies in its accelerated growth, data management solutions, further interactivity in applications, and more information regarding the benefits and best practices in the field.
Mapping and modelling in the geosciences field have grown rapidly in the past 75 years (Mossa et al., 2019). The field has evolved from predominantly 2D cartography moving into 3D geovisualization (Kraak, 2003). Geovisualizations are created using spatial tools and algorithms with spatial data (Papadopoulou et al., 2021); it is a visual tool for data exploration (Forsythe et al., 2021). This paper identifies limitations in the research and commercial applications of geovisualization while offering an evolving framing of geovisualization and interfaces and how they may grow into opportunities for geoscience to progress. The paper offers a road map vision of future opportunity for geovisualization in the context of the geosciences.
The Earth is not a 2D object - its structures and systems are geometrically three-dimensional, and four-dimensional when one includes change over time. These propositions may seem obvious, but they provide a context that led to the emergence of ‘geovisualization’ and subsequently, ‘geovisual analytics’—fields that focused on developing multidimensional, interactive methods to support the analytical needs of 3- and 4-D spatial data analysis and visualization. (See (Robinson 2017) for an overview of this evolution).
The earliest definition of geovisualization comes from (MacEachren and Monmonier, 1992) and is defined as “direct depiction of movement and change, multiple views of the same data, user interaction with maps, realism (through three dimensional stereo views and other techniques), false realism (through fractal generation of landscapes), and the mixing of maps with other graphics, text and sound”.
As cartography and mapping evolved, the lines of definitions began to overlap and the boundaries of cartography blurred (Dykes et al., 2005). MacEachren and Kraak noted that “at one level, all mapping can be considered a kind of visualization - in the sense of making visible” (Maceachren and Menno-Jan Kraak, 1997), aligning with the MacEachren and Monmonier statement in 1992 that “all map use involves both visualization and communication”. As cartography evolved, so did geovisualization, as it is founded on cartography.
Propelled by the use of technology and increases of data, geovisualization found its foothold, drawing from the disciplines of cartography, scientific visualization, data analytics, and GIScience. The links between modern computing and visualization date back to the early 1990s (MacEachren and Monmonier, 1992) and continue to become more evident through the early 2000s as experience was acquired from geovisual software and new instruments (Dykes et al., 2005), and new advantages were gained through higher resolution data and faster computing (Mitasova et al., 2012). This has continued to grow as vast sets of data are acquired and computing continues to advance (Carbonell-Carrera and Hess-Medler, 2019).
Influenced by technological advances, and increasing volumes of data, geovisualization, and its definition, has evolved throughout the years. Evolving from the definition given by (MacEachren and Monmonier, 1992) geovisualization grew to be more about the process and the acquisition of knowledge, as opposed to the strict definition of information and graphics. (Dykes et al., 2005). defines geovisualization as being about “people, maps, process, and the acquisition of information and knowledge” and can “lead to enlightenment, decision making”. The boundaries of cartography, and the nature of map use and visualizations broaden and welcome in a new era and definition of geovisualization as experience through technology and new volumes of data is gained (Dykes et al., 2005).
The emergence of visual analytics (Wong and Thomas, 2004; Thomas and Cook, 2005; Keim, 2008) resonated with the field of geovisualization, and its scope from the early 2000s onwards. In the foundational volume on visual analytics (VA) Thomas and Cook envisioned VA as “the science of analytical reasoning facilitated by interactive visual interfaces.” (2005).
Much of what VA embodied and strived for, was familiar to the geovisualization community, but it helped to legitimize and integrate a set of burgeoning subfields of geovisualization research that were on the rise (such as immersive interfaces, and new directions in interface-based spatial cognition). It made total sense that geovisualization scholars initiated geovisual analytics (geoVA) to make a significant and distinct contribution to this movement (Andrienko and Andrienko, 2007).
The need for visualization to extend beyond traditional methods and to combine with computational analysis, and other computer based operations is noted in (Andrienko and Andrienko, 2007). Visualization is shown to play an increasingly important role, as it allows for background knowledge of the human analyst to be used (Andrienko and Andrienko, 2007). Reaching beyond just geovisualization, and into scientific visualizations, this importance of visualization is shown to aid in analysis and communication (Mitasova et al., 2012). These improvements continue into present day, where it is shown that geovisualization can improve spatial thinking and decision making (Carbonell-Carrera and Hess-Medler, 2019).
As boundaries are pushed and technology grows, the volumes of data increase and geovisualization has evolved to become a cornerstone to decision making and spatial thinking. Drawing from many disciplines, including cartography, it has grown beyond its roots of a “direct depiction of movement of change” and the “mixing of maps with other graphics” (MacEachren and Monmonier, 1992) to include the people, processes and decision making aspects (Dykes et al., 2005), and become founded on the evolution of computation and data (Andrienko and Andrienko, 2007; Mitasova et al., 2012).
The 30-plus year evolution of geovisualization has always been driven by a need for spatially analytical visualizations that support ideation, analysis, interpretation and communication., informed by both deep roots in analytical cartography, and spatial analysis with mainstream GIS. But what geovisualization offers now, versus 18 years ago, let alone at its inception 30 years ago, far exceeds the scope of its formative context. Advances in data acquisition/generation technologies have transformed our ability to record and reconstruct geographic spaces and phenomena. More recently, the latest wave of spatial interface technologies (virtual reality, augmented reality, mixed reality, extended reality, have delivered far more mature and durable platforms on which to iterate and develop meaningful geovisual information experiences.
As illustrated above, geovisualization has become far more than “simply a synthesis of the long-developed visual communication of cartography with current digital analytical technologies, principally GIS” (Smith, 2022). The preceding snapshot of the scope, context and evolution of geovisualization are not intended as an exhaustive review. Rather, our aim is to illustrate how contemporary geovisualization, and the many facets of it, are the result of an ongoing, dynamic evolution. And that the scope and definition of geovisualization are in constant flux. This quote is not intended to criticise the interest, but to offer an even deeper framing of geovisualization, that has utility for Earth sciences.
Advances in the capabilities, integration and application of enabling technologies have in turn led to an expansion in analytical methods. Perhaps one of the most exciting parts of this evolving geovisualization ‘landscape’, has been the way in which new opportunities with visualization technologies and methods, has led to exciting new vectors in spatial information design, spatial interface technology design, interaction design and information experience design applied to spatial phenomena (Lonergan and Hedley, 2014; Fenech et al., 2017; Rydvanskiy and Hedley, 2020; Lochhead and Hedley, 2021). These have all led to a new expanding Frontier of variously immersive and interactive geovisualizations (Coltekin et al., 2019). These developments have in turn elevated interest and research into the relationship between users and spatial phenomena - mediated by their perceptual experience with spatial data in new spaces of analytical visualization (Lochhead et al., 2022).
Geovisualization offers methods through which geoscientific research and communication may be enhanced–through their ability to deliver rigorous, analytical multidimensional visualizations of geoscience data and phenomena at any spatial or temporal scale. While legacy planar mapping methods may suit some applications, 3D/4D geovisualization approaches have considerable value for fields such as geological mapping, rock fall mechanics, and flood plain visualization–where an ability to analyze structure and dynamics in three and four dimensions is a core need.
In the context of this paper, the geosciences are framed as a research endeavour where physical Earth systems are being studied. It is important to note that these definitions and disciplines overlap frequently with geographical research domains, and in real life situations such as in riverbed morphology or natural hazard studies. This paper explores geovisualization in the geosciences in the context of characterizing and studying the Earth and Earth systems. As the data with which we characterize 3 and 4D has become more sophisticated, so too have the opportunities to utilize these data not just in visualizations but in analytical simulations (and that can be experienced using a range of interfaces). Exploratory work of this type is increasingly being conducted by practitioners at the overlapping borders of the geosciences and geography.
Geovisualization is critically valuable to geoscience modelling is necessary for analysis and communication of data, encompasses a broad range of formats and visual representations, and goes beyond data representation into the exploration of data through “interactive and dynamic interfaces” (Marmo et al., 2010). Geovisualizations are used to enhance visual thinking about geospatial relationships (Kraak, 2003). Geovisualization can reach beyond the formal definitions such as those listed above from Marmo, Cartwright and Yuille and Kraak. At its heart it is the visualization of geospatial data and works to enable simulation and exploration of said data. It has many defining characteristics and in the context of this paper, geovisualization as the general simulation and exploration of geospatial data to enhance thinking will be explored. It enables and develops spatial thinking which can furthermore benefit the analysis of complex geoscience situations such as rockslides, flood mitigation, and contamination migration (Carbonell-Carrera and Hess-Medler, 2019). Unlike generic use of spatial analytical software to output a standard visualizations of inputted data, 3D (and 4D) geovisualizations can be intentionally designed and tuned to the specific characteristics of a geoscience phenomenon, to the needs of users across the whole scientific process. Geovisualization outputs (and the information science behind their implementation) therefore, powerfully support the communication of data, geovisual analysis exchange of data and information between stakeholder groups including public officials, citizens, and experts in the field (Jacquinod and Julia Bonccorsi, 2019).
Traditionally, geoscience data were represented in the form of 2D maps, but has been evolving to different representations. Data collected for pseudo 3D is being represented in the form of digital elevation models (DEM), hillshades, slope maps, triangular irregular networks (TIN), among others. However, 3D data is now being represented with all three coordinates (x, y, z) in the form of point clouds, generally collected using Light Detection and Ranging (LiDAR) (Sarakinou et al., 2016).
The evolution of 3D/4D spatial data acquisition technologies (multibeam, LiDAR, MVS-SfM photogrammetry) has created immense opportunities for geoscientists. As a result, 4D spatial reality capture, data production and geovisualization (though not always labelled ‘geovisualization’) have become key pieces of geoscience characterization, analysis and communication.
Contemporary sensors and technologies offer methods that have the capability to record and reconstruct the 3D structure (and 4D change/dynamics) of geoscience phenomena with greater representational fidelity than the inferential dimensionality of pseudo 3D, and abstracted representations of historical 2D planar methods (that were largely inherited from cartography).
2D and pseudo 3D models have several shortcomings in the geosciences. Pseudo 3D is less effective in communicating complex terrain information than 3D (Forsythe et al., 2021), a shortcoming that occurs when the data is transformed. 3D features undergo distortion through transformation, which can affect accuracy and analysis of the results (Ahmed et al., 2021). Difficulties exist when presenting complex information using 2D methods (Juřík et al., 2020). Additionally, historic 2D data, both in maps and databases, can lack precision and accuracy. Previous cartographic models for geoscience applications can lack precision and the ability to communicate and analyze complex information; this has given rise to the popularity of 3D geovisualization.
Note that the authors recognize that the performance of contemporary methods over traditional ones, is not only the result of technology. It is also a function of the quality of information design, execution of the methods, as well as understanding the needs and capabilities of end users. These and other considerations must form part of a commensurate 3D/4D spatial information science that informs the integration and use of cutting-edge geovisual technology and methods in the geosciences.
The paper reviews the methods used to select and analyze the papers, provides an overview of the current state of practice and applications, reviews the limitations and key issues in the current state of practice and then moves to future developments in the field, followed by conclusions.
The following methods were used to select papers relevant to the literature review regarding the current state of practice of geovisualization in the geosciences. The literature review strategy involved searching databases using keyword retrieval, content review and assessment of whether the paper met the grounds for inclusion.
The purpose of the approach used was to begin by selecting a wide range of papers at the intersection of geovisualization and the geosciences. Our aim was to capture a broad spectrum of papers, through which to be able to capture the evolution of geovisualization while identifying potential linked opportunities in the geosciences and limitations of practices in the field. A road map of past and present opportunity has been established by taking in evolving research, industry standards, best practices, and past evolution.
The databases we used are central to the fields of geosciences and emerging interfaces (and common in scientific disciplines). These databases were: Web of Science (WoS), Elsevier Science Direct, GeoRef, GEOSCAN, and SpringerLink.
The search for literature papers involved used keywords from two lists (List I and List II) to identify intersections for relevant papers. List I was comprised on geoscience related keywords and List II was comprised of geovisualization related keywords. The lists are shown in Table 1 in the Tables section. The search results included combination of words from List I and List II using “and” to ensure both categories were included. This helped target potential papers in the selected database, which were then further vetted using the grounds for inclusion.
The rationale for the search term selection was to attempt to encompass all potential geovisualization and geosciences papers by utilizing a broad range of search terms. A wide net of terms was intentionally cast to avoid disqualifying potential candidates prior to reading their abstracts. By using interlinked key words that are tangentially related to geovisualization and the geosciences, a variety of papers were selected to frame the evolution of geovisualization in the context of the geosciences.
The grounds for inclusion are based upon five main rules. Firstly, the publication must be in the field of geosciences. Secondly, geovisualization or emerging interfaces must be a central theme to the paper to ensure it is relevant to the current state of practice for geovisualization. Geovisualization or synonyms had to be in the title and substantively in the abstract. Thirdly, it must fall in the time of 2003-Present, to be based on the current state of practice while also covering the emergence of geovisualization in the early to mid 2000s. Fourth, the publication must include the shortcomings and issues of the technique or emerging technology and potential future developments to target key issues in the field and upcoming progress. Finally, the publication must either have contributed to the emergence of the field of geovisualization or be representative of the current state of practice.
In total, 50 papers were selected for the review. This is out of 160 articles total where at minimum, the abstract was read. The distribution of papers included, and total papers are shown in Figure 1 and Figure 2 in the Figures section. Each paper in the List I figure corresponds to a paper in the List II figure as it must meet both search terms to be eligible for review. The total inclusion rate is 31.2% over 160 articles. This does not include articles that did not meet grounds for inclusion based on title. The references include all articles selected, in addition to supplementary literature to provide background in the field and definition of key terms.
The literature was filtered and selected, read and processed, then the relevant information was extracted. The cited papers were then cross-referenced and synthesized to identify the current state of practice, limitations, and key issues, as well as future developments in the field.
Thematic coding was used to identify prevalent themes in the qualified literature. The main priority was to find patterns in the current state of practice and link the themes through themes of limitations, key issues, and future development. The main concepts focussed on were data acquisition, sensor mapping, geoscience modelling, and extended reality all through the lens of geovisualization and geosciences. These concepts were then linked and analyzed while highlighting limitations, issues, and opportunities in development.
The majority of themes were identified prior to thematic coding, by selecting key concepts at the intersection of geovisualization and the geosciences. Emerging themes that developed during the processing of literature were added to the review. The papers were classed into theme-based subsections and synthesized within each theme. The applicable themes to geovisualization and the geosciences were then cross-referenced to demonstrate their interconnectedness and generate an overall evaluation.
The geosciences acquire data from a number of sources including photogrammetry and LiDAR (Light Detection And Ranging) to analyze the morphological and geological landscapes (Guerin et al., 2020; Westoby, 2020; Romeo et al., 2021).
Geovisualization and modelling has come a considerable way in the past 70 years, particularly since GIS took hold in the 1990s and SAR monitoring gained popularity in the past 2 decades (Kraak, 2003). In direct linkages, geological modelling has followed this evolution to become more advanced. The advances in geosciences and geological modelling are greatly spurred by the evolvement of LiDAR, photogrammetry, and other sensing techniques, generating increased volumes of data to analyze (Havenith et al., 2019).
These sensors advance the field at large and provide new opportunities to manipulate and analyze data in 3D and 4D environments (Havenith et al., 2019). Geomodelling is frequently being used to understand and interpret geological hazards such as rockfalls, floods, and earthquakes. The majority of these models use data derived from a combination of SAR measurements and field work, then rely on numerical modelling and qualitative assessments to determine hazard risk (Jordan et al., 2017; Francioni et al., 2018; García et al., 2019; Yao et al., 2021). Though these solutions exist there is a need for more dynamic interpretation methods. The capabilities of GIS and numerical modelling are limited in both the temporal realm and 3D space (Havenith et al., 2019). GIS predominantly uses pseudo-3D modelling as opposed to real 3D and therefore, cannot capture the complexities of real 3D space and data, in instances such as flood mapping where the transformation of 3D data to pseudo-3D can affect the flow patterns and results. Similarly, numerical modelling is but a snapshot in time and fails to capture long term progression or time-lapse simulations the way a physics game engine can simulate. GIS and numerical modelling pose limitations when working with real 3D space and time lapsed activities such as rock falls or tsunamis.
Autonomous vehicles have been adopting LiDAR to sense their surroundings in urban environments (Wang et al., 2019). However, sensor technology can also be used to map remote areas (such as by using a specialized off-road vehicle) and gather data to be used in geomorphological studies.
Studies are being conducted using Mobile LiDAR Sensors (MLS) that are ground-based, airborne, and water-based (e.g., boats). Several studies involve the mounting of MLS on boats to acquire topographic data for fluvial research, creating a spatially dense point cloud by integrating navigational and data acquisition sensors (Alho et al., 2009). MLS on boats can reach accuracies of 1–4 cm (Vaaja et al., 2013). MLS requires efficient and automatic processing, which can be done through noise point filtering, and automatic identification and point classification (Vaaja et al., 2013). Boat based LiDAR can also monitor coastal sites where airborne LiDAR does not offer adequate coverage. The near horizontal view of the laser allows for high coverage and accuracy of steep topographical surfaces (Kaminsky et al., 2014). An MLS has also been employed to map railways, as a specialized mobile mapping tool that also functions for general surveying.
Mounting sensors to vehicles on rail networks has proven to be a powerful way to generate datasets along a fixed, and repeatable, path. The RailMapper managed to execute its mapping with a high level of accuracy and a precise location identification system using a coupled Global Navigation Satellite System (GNSS)—Inertial Measurement Unit (IMU) system (Kremer and Grimm, 2012).
MLS is also being used in ground-based systems to evaluate slope mass rating and monitor rockfalls. Existing approaches involve the combination of multiple remote sensing methods, including LiDAR and photogrammetry, to scan slopes. While Terrestrial Lidar Sensors (TLS) and Airborne Lidar Sensors (ALS) are increasingly being used, minimal real time processing has been conducted, leading to gaps in the use of MLS and ALS for hazard monitoring. However, MLS is increasingly being used to monitor slopes and reach inaccessible areas through ground based exploration, to support development of a rockfall monitoring system (Romeo et al., 2021). Ground based systems are also being used to map forests (Pierzchała et al., 2018; Meadows et al., 2019; Mokroš et al., 2021). TLS is time-consuming, labour intensive, and computationally demanding when developing a forest inventory but the use of MLS in combination with SLAM is being explored to measure key variables, such as number and size of trees, and while immature, poses a strong future (Pierzchała, Giguère, and Astrup, 2018).
MLS is providing better opportunities for inaccessible areas in comparison to ALS and TLS by allowing movement not tethered to a static object or only gaining an airborne angle. TLS allows the collection of data at a higher resolution but poses spatial limitations, while ALS can cover a wide area but does not have the same high point density as TLS. MLS combines these strengths, measuring objects with an accuracy of a few cm to tens of meters and presenting the ability to cover large spaces (Alho et al., 2009). In addition, ALS has poor coverage on vertical surfaces, while MLS can cover high relief topography with greater ease (Kaminsky et al., 2014). While ALS is the most widely used method, and has good visibility, it also comes with a high price tag, MLS provides similar potential range capabilities with a lower cost attached (Kremer and Grimm, 2012). TLS can be time consuming and labour intensive when gathering a high point density, while MLS is immature and has less studies, it can maximize the density to the same level as a TLS survey (Pierzchała, Giguère, and Astrup, 2018). MLS has been developing rapidly, and while it has several issues with regards to its extensive processing and analysis due to high volumes of data and noise, as well as its object recognition, it provides promising opportunities for monitoring in fluvial and geomorphological environments (Vaaja et al., 2013; Kaminsky et al., 2014; Wang et al., 2019; Romeo et al., 2021).
In order to successfully integrate sensors with vehicles for effective mapping and modelling capabilities, the analytical data visualization must be agile, and be able to connect to different programs and operate in a variety of scenarios. Existing research demonstrates that interconnected visualization infrastructure can enable new forms of geovisualization analysis. Immersive data exploration on Mars (Lochhead and Hedley, 2021), coastal environment simulations (Lonergan and Hedley, 2015; Lonergan, Hedley, and Clague, 2015), and mixed reality flood simulations (Rydvanskiy and Hedley, 2020) present the diversity of scenarios where emerging geovisualization technology can aid in understanding. Sensors and data can prove key to the operator’s situational awareness in vehicle based sensing, as well as benefitting data collection and interpretation, as shown in the Mars rover project (Helmick, Angelova, and Matthies, 2009). Integration is essential to successful operation and providing elevated geovisual analysis methods.
GIS-based software such as ArcGIS and QGIS can be used to create both 2D and 3D visualizations. GIS can take in multiple data types including point clouds collected from LiDAR, historical maps, shapefiles, among others. The simulations can be interactive or static and have a variety of applications. GIS-based 3D visualizations have been used for mapping alluvial sites, modelling elevation and topography, measuring landslide vulnerability, and mapping water depth (Masse and Christophe, 2015; Heitzler et al., 2017a; Mossa et al., 2019; Wahyudi et al., 2020; Ahmed, Mahmud, and Tuya, 2021). These techniques using GIS have demonstrated that 3D visualizations can provide new insight alongside their 2D counterparts (Mossa et al., 2019), can provide additional geographic data, and model virtual representations of real events (Wahyudi et al., 2020).
3D modelling software is being used more frequently, moving beyond the realm of GIS. It takes in point cloud or image data through methods such as LiDAR and photogrammetry and generally produces interactive visualizations. There are many different types of 3D modelling software from game engines such as Unity, applications such as AutoDesk or SketchUp, FARV3DPT, Spaceyes 3D, and Agisoft Metashape. These tools provide a wide range of options to explore three-dimensional data.
A wider range of applications, particularly those with a temporal component, have been used in 3D modelling software, as opposed to GIS. Modelling software has been used for active events and simulations such as landslides, flooding mitigation, and rockfalls (Jacquinod and Julia Bonaccorsi, 2019; Forsythe et al., 2021; Nordvik et al., 2009; G; Zhang et al., 2020). It has also been used in static instances to create more in depth maps and 3D recreations of coastlines, bathymetry, and terrain (Sarakinou et al., 2016; Papakonstantinou et al., 2018; Papadopoulou et al., 2021; de Magalhaes et al., 2020).
There are numerous benefits to using 3D modelling software over existing GIS techniques. These include the use of a physics engine in game engines to model collisions and liquid behaviour (Sala, Hutchinson, and Harrap, 2019). In addition, they utilize real 3D over the use of pseudo 3D which can be beneficial in understanding complex shapes and generating spatial awareness (Juřík et al., 2020; Wahyudi, Ramdani, and Bachtiar, 2020). Both GIS-based and 3D-model software are viable options for generating geoscience models, and in combination they can be used even more powerfully.
The current state of practice in extended reality geoscience modelling has been advancing rapidly. Extended reality aids in the effective communication of complex three-dimensional data. Geological data is complex and layered and loses fidelity and becomes more difficult to understand when communicated in 2D. Extended reality helps communicate 3D information naturally, in an efficient way, to experts and laypeople alike (Janeras et al., 2022).
Virtual mapping and monitoring in conjunction with extended reality have been used in several studies. For instance, the RIMS system, Real-time Interactive Mapping Software, generates 3D models from DEM and texture data and allows measurements and geological reconstructions in an interactive 3D environment (Bernardin et al., 2006) and has been used to model the region surrounding the epicentre of the 2010 Haiti earthquake for practical fault analysis (Cowgill et al., 2012). Augmented reality has been used to model fluids in real time over the Fraser River watershed (Rydvanskiy and Hedley, 2020), and developing mobile augmented reality system for on site analysis of the Snowy River flood plains (Haynes, Hehl-Lange, and Lange, 2018). Augmented reality has also been used to model rock slopes and fuse geoinformation virtually into the slope environment, allowing identification of structural planes and potential rockfall hazard zones (Y. Zhang et al., 2019). Despite having the ability to map rock slopes, the majority of studies do not integrate a dynamic component, instead overlaying static visualizations onto the area.
Extended reality has also been used to communicate data for teaching and learning purposes. XR offers the ability to view remote and inaccessible locations such as volcanic sites, glaciers, and underground caverns or mines for teaching purposes (Janeras et al., 2022). For instance, at the University of Georgia they have developed the 3DIG, 3D Immersion and Geovisualization, to be used for Earth science teaching and learning. It uses a combination of image acquisition, VR, MR, and 2D panels. The 3DIG effectively communicated Earth science information to students and helped enrich their understanding of complex geological environments (Bernardes et al., 2018). In another instance, the Montserrat massif was studied through complex 3D models visualized using the Microsoft HoloLens 2 and Clino’s software (Janeras et al., 2022). The system effectively and naturally communicated complex 3D information to experts in the consulting field (Janeras et al., 2022).
XR offers a rich opportunity to facilitate the understanding of multi-faceted 3D environments. The world of geosciences is 3D, often multi-layered, with many complexities. XR provides the ability to communicate this information for mapping and monitoring, teaching and learning, and general understanding.
Many advancements have been occurring in GIS representation including the emergence of cloud computing, the rise of big data analytics, open standards and interoperability, and the emergence of mobile and web GIS. Though advancements have been taking place, there are still many challenges plaguing the field. GIS has difficulties displaying 3D data beyond current applications that use DEMs, and both numerical modelling and GIS has issues displaying temporal changes (Havenith, Cerfontaine, and Mreyen, 2019). Though in recent years, applications such as ESRI’s ArcGIS Pro have been adding LiDAR integration and features (Price, 2018).
When data is converted from 3D to the 2.5D (pseudo-3D) that is prevalent in GIS, representations become distorted (Ahmed, Mahmud, and Tuya, 2021). The transition from 3D to 2.5D can cause a loss of detail and the 2D projections can add increased mental load as the user attempts to interpret the information from a flat screen (de Magalhaes et al., 2020; Zhang et al., 2020). The increased mental workload coupled with the loss of detail can reduce interaction and limit the communicability of the data.
GIS can also rely on 2D visualizations, which can mask features. 2D GIS features can become indistinguishable; sills and steep slopes in bathymetry, for instance, can be difficult to interpret (Forsythe et al., 2021).
On the whole, information produced and displayed in 2.5D must undergo transformations which can alter its fidelity and accuracy, in comparison to 3D data, and thus lacks effectiveness in comparison (Juřík et al., 2020). Real 3D visualizations demonstrate the capability to increase spatial thinking and interpretation skills in comparison to 2.5D or 2D visualizations that GIS displays (Juřík et al., 2020; Wahyudi et al., 2020).
GIS poses limitations when displaying 3D data, particularly its distortion when converting to 2.5D, and reliance on the 2D or 2.5D data presentations. This leads to less significant data display, in terms of accuracy, which ultimately affects decision making, and lacks full interpretation and interactivity abilities that are capable in real 3D visualizations.
Virtual reality is rarely used in the geosciences due to both its high cost and the required computing knowledge to correctly apply the emerging interfaces to geoscience applications.
Financial capital can be expended through imagery techniques or personnel. Imagery, including LiDAR, UAV, and MLS can be costly, particularly if data must be collected on an as-needed basis (Ahmed et al., 2021). It is also difficult to acquire high resolution data that is applicable to rural landscapes that are not accessible by roads; this can make accurate modelling a challenge (Francioni et al., 2018).
The lack of data sharing in the industry and academia has been raised, which may cause additional costs when developing systems to exchange terabytes of 3D data. Personnel and risks gathering data, such as traversing an area with natural hazards or poor weather conditions, can also drive up costs (Rydvanskiy and Hedley, 2020). There is a knowledge capital required via personnel, as 3D tasks generally take longer to solve (Juřík et al., 2020) and the spatial thinking skills must be learned in order to effectively analyze and interpret 3D data.
When high resolution data are acquired, processing speeds are slower and real time processing is difficult but may be able to be completed with effective cloud computing (Havenith et al., 2019). Real time processing could aid in efficiency when identifying issues such as runoff and unstable slopes, however, the large volumes of data such as those in the intricacies of a rock mass, can lead to longer processing times.
3D data has additional complexity which may lead to issues in rendering efficiency, particularly if the data is of a finer resolution. In addition, Cartesian coordinates are not transformed automatically when input into a 3D engine (G. Zhang et al., 2020) which can lead to a longer processing time while the coordinate system is converted. The multi-dimensional data requires an effective data management system moving forward (Núñez-Andrés et al., 2022).
Processing times can also be longer due to the large data pool. Data reaches up to terabyte levels quickly when collecting in three dimensions and the computing power can be a limitation in processing. When collecting geosciences data for geovisualizations it often contains high complexity and large-scale areas to gain a full understanding. For example, when modelling a large outcrop formation, surrounding data covering many square kilometers is needed to establish a full picture. Past trends have shown that computing and processing capabilities are consistently behind the increase of data volume and complexity (Li et al., 2011). A consistent limitation has been processing time and computing power as data grows in this era of geovisualization.
In the realm of augmented reality, digital occlusion is an issue that plagues many simulations and users can experience motion sickness or experience data interpretation issues (V Juřík et al., 2016; Lonergan and Hedley, 2014). The synchronization and rendering is a key piece, particularly as users may experience motion sickness due to frame rate or unsynchronized interaction (Havenith et al., 2019). Occlusion has been shown to be an issue when modelling digital earth (G. Zhang et al., 2020). Digital earth has been used to model earth systems and when utilizing digital earth to perform flood visualizations, occlusion was a prevalent issue. When the viewpoint moves and the flood object is occluded, the flood may not be rendered and understood correctly (G. Zhang et al., 2020).
Users are not only subject to occlusion in simulation but also need to apply spatial thinking skills to interpret visualizations. 3D geovisualizations cannot be rapidly understood without explanation or previous spatial thinking skills (Jacquinod and Julia Bonaccorsi, 2019). When viewing the Earth in a VR setting, as many as one in ten users experienced motion sickness (Havenith et al., 2019). 3D geovisualizations and communicability of information therefore relies heavily on the user themselves; including their spatial thinking skills, which can be heavily impacted by issues with motion sickness, and reaction to frame rate or occlusion.
Another predominant issue in AR is tracking, registration, and rendering; specifically, linking 1:1 relations in real time between the virtual environment and real world (Lonergan and Hedley, 2014; Zhang et al., 2019). Despite being a challenge, it is being solved using edge tracking and camera posing and has the potential to be overcome in AR environments.
The accuracy in matching points to their true location can depend on the techniques used and the surveyor’s skill leaving room for uncertainty and errors, which can lead to misaligned data. This is noted when modelling 3D landforms to enhance spatial thinking (Carbonell-Carrera and Hess-Medler, 2019). Matching issues can also occur during the transformation in the Cartesian alignment of space (Rydvanskiy and Hedley, 2020). When modelling floods, transformation issues with the Cartesian alignment can affect the flood paths and ultimately, the result (Rydvanskiy and Hedley, 2020). This demonstrates the need for proper matching and alignment in geoscience applications such as flood visualization. Object tracking methods and recognition, including point matching, is an issue that persists (Jian et al., 2017). Furthermore, misalignment leads to errors and uncertainties in data location that can cause interpretation issues and incorrect conclusions drawn from data.
There are other prevalent issues that exist with specific data acquisition techniques. Each data collection technique has its advantages and disadvantages; for instance, LiDAR cannot reach underwater (Mossa, Chen, and Wu, 2019) while high resolution InSAR data can be difficult to acquire. For instance, LiDAR DSM and SfM DSM have similar results in bare plots but SfM is inaccurate in forested areas and cannot penetrate the vegetation (Hua et al., 2021). Data resolution is another prevalent issue where surficial data is dense but subsurface or underwater data is lacking, leading to misinterpretations (Nordvik et al., 2009; Mossa et al., 2019). The significance of resolution lies in its ability to provide finer details which can aid in effective decision making when using the data.
The future of 3D geovisualization still has many unknowns but is moving towards increasing interactivity, studies exploring its uses, and data management.
Interactivity and immersion are becoming more popular, particularly in the field of extended reality. Immersive navigation has been shown, as of late, to increase recognition and overall spatial awareness, and increase performance when interpreting complex terrain (Nordvik et al., 2009; Ahmed, Mahmud, and Tuya, 2021). Interaction and collaboration are current obstacles as it is difficult to create real time, collaborative interfaces, however, emerging interfaces are offering better options to improve the interactivity of ‘geovisualized’ data (Havenith et al., 2019; Rydvanskiy and Hedley, 2020).
New methods are also being developed to manage the increasingly large amounts of data. As terabytes of data are being produced through photogrammetry and LiDAR, among other recording techniques, data management becomes more integral. Computing and processing continually lag behind the development of data collection methods, leaving a gap between the data management and processing and the collection itself (Li et al., 2011). The development of algorithms to reduce data size is a strong avenue for future development (Heitzler et al., 2017b).
There are still many unknowns in the use of 3D and its effectiveness in increasing decision making in geosciences applications. Results have been contradictory, some showing that 3D methods do not necessarily communicate risk area more effectively than 2D solutions (Wahyudi et al., 2020) and that its use may simply be a novelty. Lab studies do not reflect usability in the real world, and its uses remain ambiguous, not yet being analyzed critically (Havenith et al., 2019; Juřík et al., 2016). The benefits of 3D visualizations are not yet clear in geoscience applications, and the lack of evidence for efficiency could negatively impact long-term growth of 3D geovisualization techniques and applications (Nordvik et al., 2009; Rydvanskiy and Hedley, 2020).
Driven by commercial urgency and the growth of computing technology, 3D geovisualization and its applications are rapidly evolving. Future developments will have a strong focus on interactivity, data management, and determining the benefits and best practices for use of 3D geovisualization.
Geosciences modelling has made great strides, particularly in the past few decades, being driven by large increases in data which are providing new opportunities to analyze data in 3D and 4D. The geosciences have been acquiring data from an increasing number of sources, and as more data becomes available through methods such as LiDAR, photogrammetry, and InSAR, there are more opportunities to utilize the data in 3D and 4D settings. Geoscience modelling is using said data in GIS based programs and experimenting with their newer pseudo-3D interfaces, in 3D modelling in game engines and custom-built programs, and in extended reality interfaces with increasing interactivity.
Though research and commercial use in the field of geovisualization is increasing, there are several key limitations that remain. In GIS representations there is a difficulty displaying 3D data and it is frequently translated to pseudo-3D, which can mask features and cause distortion when translating points. There is also financial, and personnel capital being used due to the novelty of 3D geovisualization and the cost of gathering data, which oftentimes cannot be gathered on demand. Processing issues remain prevalent as computing power, related to CPU and GPU use, is lagging behind data volumes leading to limited speeds when processing the large data pool. There are additional issues with newer interfaces and users such as digital occlusion and spatial interpretation of the user potentially causing motion sickness and limiting understanding. Matching and alignment issues with 3D points are another limitation. On the whole, the large data pool and translation of 3D data is causing a barrier but is being explored in both research and commercial sectors.
The development of 3D geovisualization is evolving rapidly, with a focus on interactivity, data management, and identifying the benefits and best practices. Both industry and academia are attempting to overcome key limitations while interfaces expand, and interactivity becomes more prevalent. The future of 3D geovisualization is growing quickly and moving to tackle more geoscientifically complex applications and address limitations.
MF and NH contributed to the conception and design of this review. MF reviewed and selected applicable papers. MF wrote the first draft of the manuscript. All authors contributed to the article and approved the submitted version.
The authors declare that the research was conducted in the absence of any commercial or financial relationships that could be construed as a potential conflict of interest.
All claims expressed in this article are solely those of the authors and do not necessarily represent those of their affiliated organizations, or those of the publisher, the editors and the reviewers. Any product that may be evaluated in this article, or claim that may be made by its manufacturer, is not guaranteed or endorsed by the publisher.
Ahmed, R., Khandakar, H. M., and Jannatun, H. T. (2021). A GIS-based mathematical approach for generating 3D terrain model from high-resolution UAV imageries. J. Geovisualization Spatial Analysis 5, 24. doi:10.1007/s41651-021-00094-7
Alho, P., Kukko, A., Hyyppä, H., Kaartinen, H., Hyyppä, J., and Jaakkola, A. (2009). Application of boat-based laser scanning for river survey. Earth Surf. Process. Landforms 34 (13), 1831–1838. doi:10.1002/esp.1879
Andrienko, N., and Andrienko, G. (2007). Designing visual analytics methods for massive collections of movement data. Cartographica 42 (2), 117. –138. doi:10.3138/carto.42.2.117
Bernardes, S., Madden, M., Knight, A., Neel, N., Morgan, N., Cameron, K., et al. (2018). “A multi-component system for data acquisition and visualization in the geosciences based on UAVs, augmented and virtual reality,” in The international archives of the photogrammetry, remote sensing and spatial information sciences XLII–4 (Copernicus GmbH: IEEE), 45–49. doi:10.5194/isprs-archives-XLII-4-45-2018
Bernardin, T., Cowgill, E., Gold, R., Hamann, B., Kreylos, O., and Schmitt, A. (2006). Interactive mapping on 3-D terrain models: INTERACTIVE TERRAIN MAPPING. Geochem. Geophys. Geosystems G3 7 (10). doi:10.1029/2006GC001335
Carbonell-Carrera, C., and Hess-Medler, S. (2019). 3D landform modeling to enhance geospatial thinking. Isprs Int. J. Geo-Information 8 (2), 65. doi:10.3390/ijgi8020065
Coltekin, A., Griffin, A., Aidan Slingsby, , Robinson, A., Christophe, S., Rautenbach, V., et al. (2019). Geospatial information visualization and extended reality displays.
Cowgill, E., Bernardin, T. S., Oskin, M. E., Bowles, C., Burak Yikilmaz, M., Kreylos, O., et al. (2012). Interactive terrain visualization enables virtual field work during rapid scientific response to the 2010 Haiti earthquake. Geosph. (Boulder, Colo.) 8 (4), 787. –804. doi:10.1130/GES00687.1
de Magalhaes, H., Marques, D., and Ana Clara Mourao, M. (2020). “Aerial images and three-dimensional models generated by RPA to support geovisualization in geodesign workshops,” in Computational science and its applications, iccsa 2020, Part Iv. Editors O. Gervasi, S. Misra, C. Garau, I. Blecic, D. Taniar, B. O. Apduhanet al. (Cham: Springer International Publishing Ag), 296–309. doi:10.1007/978-3-030-58811-3_21
Fenech, A., Chen, A., Clark, A., and Hedley, N. (2017). Building an adaptation tool for visualizing the coastal impacts of climate change on prince edward island, Canada. Clim. Change Adapt. N. Am. 225. doi:10.1007/978-3-319-53742-9_14
Forsythe, K. W., Danielle, E., Ford, C. H., Shaker, R. R., MacDonald, M. W., and Wilkinson, R. (2021). Multivariable 3D geovisualization of historic and contemporary lead sediment contamination in lake erie. Pollutants 1 (1), 29. –50. doi:10.3390/pollutants1010004
Francioni, M., Coggan, J., Eyre, M., and Stead, D. (2018). A combined field/remote sensing approach for characterizing landslide risk in coastal areas. Int. J. Appl. Earth Observation Geoinformation 67 (May), 79–95. doi:10.1016/j.jag.2017.12.016
García, H., Jesús, A., Marchamalo, M., Martínez, R., González-Rodrigo, B., and González, C. (2019). Integrating geotechnical and SAR data for the monitoring of underground works in the Madrid urban area: application of the persistent scatterer interferometry technique. Int. J. Appl. Earth Observation Geoinformation 74 (February), 27–36. doi:10.1016/j.jag.2018.08.025
Guerin, A., Stock, G. M., Radue, M. J., Jaboyedoff, M., Collins, B. D., Matasci, B., et al. (2020). Quantifying 40 years of rockfall activity in yosemite valley with historical structure-from-motion photogrammetry and terrestrial laser scanning. Geomorphology 356, 107069. doi:10.1016/j.geomorph.2020.107069
Havenith, H.-B., Philippe, C., and Mreyen, A.-S. (2019). How virtual reality can help visualise and assess geohazards. Int. J. Digital Earth 12 (2), 173–189. doi:10.1080/17538947.2017.1365960
Haynes, P., Hehl-Lange, S., and Lange, E. (2018). Mobile augmented reality for flood visualisation. Environmental modelling and software: with environment data news. Elsevier Ltd. 109, 380. –389. doi:10.1016/j.envsoft.2018.05.012
Heitzler, M., Hackl, J., Adey, B. T., and Hurni, L. (2017a). A simulation and visualization environment for spatiotemporal disaster risk assessments of network infrastructures. Cartogr. Int. J. Geogr. Inf. Geovisualization 52 (4), 349–363. doi:10.3138/cart.52.4.2017-0009
Heitzler, M., Hackl, J., Adey, B. T., and Hurni, L. (2017b). GPU-accelerated rendering methods to visually analyze large-scale disaster simulation data. J. Geovisualization Spatial Analysis 1 (1–2), 3. doi:10.1007/s41651-017-0004-4
Helmick, D., Angelova, A., and Matthies, L. (2009). Terrain adaptive navigation for planetary rovers. J. Field Robotics 26 (4), 391–410. doi:10.1002/rob.20292
Hua, J., Zhou, J., and Yang, W. (2021). Comparing LiDAR and SfM digital surface models for three land cover types. Open Geosci. 13 (May), 497–504. doi:10.1515/geo-2020-0257
Jacquinod, F., and Julia Bonaccorsi, H. (2019). Studying social uses of 3D geovisualizations: lessons learned from action-research projects in the field of flood mitigation planning. ISPRS Int. J. Geo-Information 8 (2), 84. doi:10.3390/ijgi8020084
Janeras, M., Roca, J., Gili, J. A., Pedraza, O., Magnusson, G., Amparo Núñez-Andrés, M., et al. (2022). Using mixed reality for the visualization and dissemination of complex 3D models in geosciences—application to the Montserrat massif (Spain). Geosciences 12 (10), 370. doi:10.3390/geosciences12100370
Jian, H., Liao, J., Fan, X., and Xue, Z. (2017). Augmented virtual environment: fusion of real-time video and 3D models in the digital earth system. Int. J. Digital Earth 10 (12), 1177–1196. doi:10.1080/17538947.2017.1306126
Jordan, H., Cigna, F., and Bateson, L. (2017). Identifying natural and anthropogenically-induced geohazards from satellite ground motion and geospatial data: stoke-on-trent, UK. Int. J. Appl. Earth Observation Geoinformation 63 (December), 90–103. doi:10.1016/j.jag.2017.07.003
Juřík, V., Herman, L., Kubíček, P., Stachoň, Z., and Šašinka, Č. (2016). “COGNITIVE ASPECTS OF COLLABORATION IN 3D VIRTUAL ENVIRONMENTS,” in International archives of the photogrammetry, remote sensing and spatial information sciences (Gottingen: Copernicus GmbH), XLI-B2, 663–670. doi:10.5194/isprs-archives-XLI-B2-663-2016
Juřík, V., Herman, L., Snopková, D., Stachoň, Z., Chmelík, J., Kubíček, P., et al. (2020). The 3D hype: evaluating the potential of real 3D visualization in geo-related applications. PLoS one. Public Libr. Sci. 15 (5), e0233353. doi:10.1371/journal.pone.0233353
Kaminsky, G. M., Baron, H. M., Hacking, A., and McCandless, D. (2014). Mapping and monitoring bluff erosion with boat-based LIDAR and the development of a sediment budget and erosion model. Washington: Washington State Department of Ecology.
Keim, D. A. (2008). “Florian mansmann, jörn schneidewind, jim Thomas, and hartmut ziegler,” in Visual data mining. Editors S. J. Simoff, M. H. Böhlen, and A. Mazeika (Berlin, Heidelberg: Springer Berlin Heidelberg). doi:10.1007/978-3-540-71080-6_6
Kraak, M.-J. (2003). Geovisualization illustrated. ISPRS J. Photogrammetry Remote Sens. 57 (5), 390–399. doi:10.1016/S0924-2716(02)00167-3
Kremer, J., and Grimm, A. (2012). The railmapper – a dedicated mobile lidar mapping system for railway networks. International archives of the photogrammetry. Remote Sens. Spatial Inf. Sci., 477. doi:10.5194/isprsarchives-XXXIX-B5-477-2012
Li, J., Wu, H., Yang, C., Wong, D. W., and Xie, J. (2011). Visualizing dynamic geosciences phenomena using an octree-based view-dependent LOD strategy within virtual globes. Comput. Geosciences 37 (9), 1295–1302. doi:10.1016/j.cageo.2011.04.003
Lochhead, I., and Hedley, N. (2021). Designing virtual spaces for immersive visual analytics. KN - J. Cartogr. Geogr. Inf. 71 (4), 223–240. doi:10.1007/s42489-021-00087-y
Lochhead, I., Hedley, N., Çöltekin, A., and Fisher, B. (2022). The immersive mental rotations test: evaluating spatial ability in virtual reality. Front. Virtual Real. 3. Front. Media S.A. doi:10.3389/frvir.2022.820237
Lonergan, C., and Hedley, N. (2014). Flexible mixed reality and situated simulation as emerging forms of geovisualization. Cartographica 49 (3), 175. –187. doi:10.3138/carto.49.3.2440
Lonergan, C., and Hedley, N. (2015). Navigating the future of tsunami risk communication: using dimensionality, interactivity and situatedness to interface with society. Nat. Hazards 78 (1), 179–201. doi:10.1007/s11069-015-1709-7
Lonergan, C., Hedley, N., and Clague, J. J. (2015). A visibility-based assessment of tsunami evacuation signs in seaside, Oregon. Nat. Hazards Dordr. 78 (1), 41. doi:10.1007/s11069-015-1696-8
Maceachren, A. M.Menno-Jan Kraak (1997). Exploratory cartographic visualization: advancing the agenda. Comput. Geosciences, Explor. Cartograpic Vis. 23 (4), 335–343. doi:10.1016/S0098-3004(97)00018-6
MacEachren, A. M., and Monmonier., M. (1992). Introduction. Cartography and geographic information systems. Taylor Francis 19 (4), 197. doi:10.1559/152304092783721303
Marmo, C., Cartwright, W., and Yuille, J. (2010). Knowledge in (Geo)Visualisation: the relationship between seeing and thinking. Available at: https://www.semanticscholar.org/paper/Knowledge-in-(Geo)Visualisation%3A-The-relationship-Marmo-Cartwright/428144de6b135b0bfccb77924d5ad3d60b2680db.
Masse, A., and Christophe, S. (2015). Homogeneous geovisualization of coastal areas from heterogeneous spatio-temporal data. Int. Archives Photogrammetry, Remote Sens. Spatial Inf. Sci. XL, 509–516. doi:10.5194/isprsarchives-XL-3-W3-509-2015
Meadows, W., Hudson, C., Goodin, C., Dabbiru, L., Powell, B., Matt, D., et al. 2019. “Multi-LiDAR placement, calibration, Co-registration, and processing on a subaru forester for off-road autonomous vehicles operations, 11009:110090J–110111J. SPIE. doi:10.1117/12.2518915
Menno-Jan Kraak (2005). in Exploring geovisualization. Editors J. Dykes,, and A. M. MacEachren Germany, (Elsevier).
Mitasova, H., Harmon, R. S., Weaver, K. J., Lyons, N. J., and Overton, M. F. (2012). Scientific visualization of landscapes and landforms. Geomorphol. Geospatial Technol. Geomorphol. Mapp. Proc. 41st Annu. Binghamt. Geomorphol. Symposium 137 (1), 122–137. doi:10.1016/j.geomorph.2010.09.033
Mokroš, M., Mikita, T., Singh, A., Tomaštík, J., Chudá, J., Wężyk, P., et al. (2021). Novel low-cost mobile mapping systems for forest inventories as terrestrial laser scanning alternatives. Int. J. Appl. Earth Observation Geoinformation 104 (December), 102512. doi:10.1016/j.jag.2021.102512
Mossa, J., Chen, Y.-H., and Wu, C.-Yu (2019). Geovisualization geoscience of large river floodplains. J. Maps 15 (3), 75–91. doi:10.1080/17445647.2019.1584129
Nordvik, T., Grøneng, G., Nilsen, B., and Harding, C. (2009). Geovisualization, geometric modelling and volume estimation of the åknes rockslide, western Norway. Bull. Eng. Geol. Environ. 68 (2), 245–256. doi:10.1007/s10064-009-0198-x
Núñez-Andrés, L., Amparo, M., Zarzosa, N. L., and Martínez-Llario, J. (2022). Spatial data infrastructure (SDI) for inventory rockfalls with fragmentation information. Nat. Hazards 112 (3), 2649–2672. doi:10.1007/s11069-022-05282-2
Papadopoulou, E.-E., Papakonstantinou, A., Zouros, N., and Soulakellis, N. (2021). Scale-variant flight planning for the creation of 3D geovisualization and augmented reality maps of geosites: the case of voulgaris gorge, lesvos, Greece. Appl. Sciences-Basel 11 (22), 10733. doi:10.3390/app112210733
Papakonstantinou, A., Doukari, M., Roussou, O., Ch Drolias, G., Chaidas, K., Moustakas, A., et al. (2018). “UAS multi-camera rig for post-earthquake damage 3D geovisualization of vrisa village,” in Sixth international conference on remote sensing and geoinformation of the environment (Rscy2018). Editors K. Themistocleous, G. Papadavid, S. Michaelides, V. Ambrosia, and D. G. Hadjimitsis (Bellingham: Spie-Int Soc Optical Engineering). UNSP 1077315. doi:10.1117/12.232617310773
Pierzchała, M., Giguère, P., and Astrup, R. (2018). Mapping forests using an unmanned ground vehicle with 3D LiDAR and graph-SLAM. Comput. Electron. Agric. 145 (February), 217–225. doi:10.1016/j.compag.2017.12.034
Price, M. (2018). Mapping and modeling lidar data with ArcGIS Pro. Available at: https://www.esri.com/about/newsroom/arcuser/mapping-and-modeling-lidar-data-with-arcgis-pro/.
Robinson, A. (2017). Geovisual analytics. GIS&T body of knowledge. Available at: https://gistbok.ucgis.org/bok-topics/geovisual-analytics.
Romeo, S., Cosentino, A., Giani, F., Mastrantoni, G., and Mazzanti, P. (2021). Combining ground based remote sensing tools for rockfalls assessment and monitoring: the poggio baldi landslide natural laboratory. Sensors (Basel, Switz. 21 (8), 2632. doi:10.3390/s21082632
Rydvanskiy, R., and Hedley, N. (2020). 3D geovisualization interfaces as flood risk management platforms: capability, potential, and implications for practice. Cartogr. Int. J. Geogr. Inf. Geovisualization 55 (4), 281. –290. doi:10.3138/cart-2020-0003
Sala, Z., Jean Hutchinson, D., and Harrap, R. (2019). Simulation of fragmental rockfalls detected using terrestrial laser scans from rock slopes in south-central British columbia, Canada. Nat. Hazards Earth Syst. Sci. 19 (11), 2385. –2404. doi:10.5194/nhess-19-2385-2019
Sarakinou, I., Papadimitriou, K., Georgoula, O., and Patias, P. (2016). Underwater 3D modelling: image enhancement and point cloud filtering. Int. Archives Photogrammetry, Remote Sens. Spatial Inf. Sci. XLI-B2, 441–447. doi:10.5194/isprs-archives-XLI-B2-441-2016
Smith, M. (2022). Treatise on geomorphology. Available at: http://www.sciencedirect.com:5070/referencework/9780128182352/treatise-on-geomorphology.
Vaaja, M., Kukko, A., Kaartinen, H., Kurkela, M., Kasvi, E., Flener, C., et al. (2013). Data processing and quality evaluation of a boat-based mobile laser scanning system. Sensors (Basel, Switz. 13 (9), 12497. –12515. doi:10.3390/s130912497
Wahyudi, H. B., and Ramdani, F.Fitra Abdurrachman Bachtiar (2020). 2D and 3D geovisualization: learning user preferences in landslide vulnerability. JITeCS J. Inf. Technol. Comput. Sci. 5 (1). 75–85. doi:10.25126/jitecs.202051167
Wang, Y., Chen, Qi, Zhu, Q., Liu, L., Li, C., and Zheng, D. (2019). A survey of mobile laser scanning applications and key techniques over urban areas. Remote Sens. (Basel, Switz. 11 (13), 1540. doi:10.3390/rs11131540
Westoby, M. (2020). Decoding complex erosion responses for the mitigation of coastal rockfall hazards using repeat terrestrial LiDAR. Remote Sens. 12 (16), 2620. doi:10.3390/rs12162620
Wong, P. C., and Thomas, J. (2004). Visual analytics. IEEE Comput. Graph. Appl. 24 (5), 20–21. doi:10.1109/MCG.2004.39
Yao, X., Chen, Y., Liu, D., Zhou, Z., Liesenberg, V., and Li, J. (2021). Average-DInSAR method for unstable escarpments detection induced by underground coal mining. Int. J. Appl. Earth Observation Geoinformation 103 (December), 102489. doi:10.1016/j.jag.2021.102489
Zhang, G., Gong, J., Li, Yi, Sun, J., Xu, B., Zhang, D., et al. (2020). An efficient flood dynamic visualization approach based on 3D printing and augmented reality. Int. J. Digital Earth 13 (11), 1302. doi:10.1080/17538947.2019.1711210
Keywords: geovisualization, geoscience, 3D modelling, geosciences modelling, 3D
Citation: Fitzpatrick M and Hedley N (2024) Review of the state of practice in geovisualization in the geosciences. Front. Earth Sci. 11:1230973. doi: 10.3389/feart.2023.1230973
Received: 29 May 2023; Accepted: 18 December 2023;
Published: 25 January 2024.
Edited by:
Ali Khenchaf, De la Communication et de la Connaissance (LAB-STICC), FranceReviewed by:
Robert Newell, Royal Roads University, CanadaCopyright © 2024 Fitzpatrick and Hedley. This is an open-access article distributed under the terms of the Creative Commons Attribution License (CC BY). The use, distribution or reproduction in other forums is permitted, provided the original author(s) and the copyright owner(s) are credited and that the original publication in this journal is cited, in accordance with accepted academic practice. No use, distribution or reproduction is permitted which does not comply with these terms.
*Correspondence: Mia Fitzpatrick, bWZhODFAc2Z1LmNh
Disclaimer: All claims expressed in this article are solely those of the authors and do not necessarily represent those of their affiliated organizations, or those of the publisher, the editors and the reviewers. Any product that may be evaluated in this article or claim that may be made by its manufacturer is not guaranteed or endorsed by the publisher.
Research integrity at Frontiers
Learn more about the work of our research integrity team to safeguard the quality of each article we publish.