- 1WSL Institute for Snow and Avalanche Research SLF, Davos, Switzerland
- 2Eastern Switzerland University of Applied Sciences, Rapperswil, Switzerland
- 3CNRS, Centre d’Études de la Neige, University Grenoble Alpes, Université de Toulouse, Grenoble, France
- 4Department of Geosciences, University of Oslo, Oslo, Norway
The seasonal evolution of snow cover has significant impacts on the hydrological cycle and microclimate in mountainous regions. However, snow processes also play a crucial role in triggering alpine mass movements and flooding, posing risks to people and infrastructure. To mitigate these risks, many countries use operational forecast systems for snow distribution and melt. This paper presents the Swiss Operational Snow-hydrological (OSHD) model system, developed to provide daily analysis and forecasts on snow cover dynamics throughout Switzerland. The OSHD system is a sophisticated snow hydrological model designed specifically for the high-alpine terrain of the Swiss Alps. It leverages exceptional station data and high-resolution meteorological forcing data, as well as various reanalysis products to combine snow modeling with advanced data assimilation and meteorological downscaling methods. The system offers models of varying complexity, each tailored to specific modeling strategies and applications. For snowmelt runoff forecasting, monitoring snow water resources, and research-grade purposes, the OSHD system employs physics-based modeling chains. For snow climatological assessments, a conceptual model chain is available. We are pleased to present two comprehensive datasets from the conceptual and physics-based models that cover the entirety of Switzerland. The first dataset comprises a snow water equivalent climatology spanning 1998–2022, with a spatial resolution of 1 km. The second dataset includes snow distribution and snow melt data spanning 2016–2022 at a high spatial resolution of 250 m. To meet the needs of a multi-purpose snow hydrological model framework, the OSHD system employs various strategies for process representation and sub-grid parameterizations at the snow-canopy-atmosphere interface, particularly in complex terrain. Recent and ongoing model developments are aimed at accounting for complex forest snow processes, representing slope and ridge-scale precipitation and snow redistribution processes, as well as improving probabilistic snow forecasts and data assimilation procedures based on remote sensing products.
1 Introduction
The distribution of snow in space and time is of paramount importance to the Earth system, as it plays a fundamental role in driving the hydrological cycle (Viviroli et al., 2007; Clark et al., 2011; Vionnet et al., 2019) and influencing the climate of cold regions (Beniston et al., 2018). In mountainous regions, the timing and intensity of snowmelt can have significant impacts on extreme events such as rain-on-snow flooding (e.g., Würzer and Jonas, 2018; Li et al., 2019) and droughts (e.g., Barnett et al., 2005; Dierauer et al., 2018).
The hydrological response of mountain catchments is driven by a range of snow accumulation and ablation processes (Freudiger et al., 2017) that operate at multiple temporal and spatial scales (Blöschl, 1999; Clark et al., 2011). Accurately estimating snow accumulation and ablation is critical for reducing uncertainties in snow-hydrological modelling (Raleigh et al., 2015; Vionnet et al., 2022) and presents a challenge for snow models used in avalanche hazard forecasting (Morin et al., 2020) and hydrological predictions (Brauchli et al., 2017). The accumulation of snow in complex terrain is mainly governed by atmospheric processes, which can range from orographic precipitation enhancement at large spatial scales to preferential deposition and redistribution of snow at finer scales (Gerber et al., 2019; Vionnet et al., 2021). During the ablation season, the melt rates vary spatially due to differences in solar irradiance resulting from variations in aspect, shading, and albedo (e.g., Marks and Dozier, 1992; Dumont et al., 2011; Schirmer and Pomeroy, 2020), turbulent fluxes of sensible and latent heat (e.g., Winstral and Marks, 2014; Garvelmann et al., 2015; Mott et al., 2019), and heat advection processes during patchy snow cover conditions (e.g., Essery et al., 2006; Pohl et al., 2006; Mott et al., 2016; Schlögl et al., 2016; Harder et al., 2017; Mott et al., 2017).
Furthermore, the complex interactions between the snow and canopy, such as attenuation of shortwave radiation and enhancement of longwave radiation (Hardy et al., 2004; Sicart et al., 2006; Pomeroy et al., 2009; Musselman and Pomeroy, 2017; Webster et al., 2017; Malle et al., 2019), wind attenuation (Mahat et al., 2013; Roth and Nolin, 2017), interception of snowfall (Hedstrom and Pomeroy, 1998; Moeser et al., 2015; Roth and Nolin, 2019; Helbig et al., 2020), and subsequent unloading or sublimation of canopy snow (Pomeroy et al., 1998; Mahat and Tarboton, 2014), pose significant challenges for hydrological and land surface models. These challenges make snow-hydrological predictions even more complex for forested landscapes (Essery et al., 2009; Rutter et al., 2009), and accurate representation of snow accumulation and ablation dynamics in both open and forested areas is crucial for reliable snow-hydrological predictions.
Temperature-index models have been widely adopted in modeling snow and meltwater in mountain catchments due to their low computational costs and ability to run on simple, widely available input data (Hock, 1999; Jost et al., 2012; Tobin et al., 2013; Farinotti et al., 2012; Girons Lopez et al., 2020; Parajuli et al., 2020). However, such models fail to capture spatial differences in snow melt rates and runoff among slopes with different aspects, which are critical in representing the seasonal runoff dynamics of alpine catchments (Dornes et al., 2008a; Dornes et al., 2008b). DeBeer and Pomeroy (2017) contend that temperature-index model approaches may yield acceptable results in certain topographic settings and climatic conditions, but the spatial variability in the snowpack energy balance must be considered, especially in cold regions, windy conditions, and increasingly complex terrain. Therefore, distributed energy- and mass-balance snow cover models are increasingly being applied in snow hydrological simulations (Bavay et al., 2013; Warscher et al., 2013; Gallice et al., 2016; Painter et al., 2016; Brauchli et al., 2017; Magnusson et al., 2017; Hedrick et al., 2018; Shakoor et al., 2018; Carletti et al., 2022). In particular, the consideration of temporally and spatially varying surface energy fluxes is crucial for snow-hydrological simulations in complex terrain, extreme weather events, and glacierized catchments, where the presence of local wind systems enhances the importance of turbulent heat exchange for the energy balance of snow and ice surfaces (Shea and Moore, 2010; Sauter and Galos, 2016; Freudiger et al., 2017; Mott et al., 2020).
The frequency and intensity of extreme weather events are projected to increase at high elevations, leading to potential flooding from heavy rainfall events in spring or rain-on-snow events in winter (Surfleet and Tullos, 2013; Beniston and Stoffel, 2016; Morán-Tejeda et al., 2016; Musselman et al., 2018). However, accurately simulating the complex snow-atmosphere interactions during rain-on-snow events with temperature-based solutions is challenging, as turbulent heat fluxes play a significant role in high snow melt intensities (Dyer and Mote, 2002; Dadic et al., 2013; Garvelmann et al., 2014). Therefore, it is increasingly important to rely on physically-based snow-hydrological models to accurately predict the effects of extreme weather events on snowmelt and runoff.
Operational physical-based snow cover models have limitations due to their high computational demands and dependence on spatially distributed meteorological input variables obtained from regional weather models in high temporal and spatial resolution (Anderson, 1976; Schlögl et al., 2016; Hock et al., 2017). While these regional weather models can resolve coarser processes such as orographic enhancement, they fail to capture ridge and slope-scale processes (Dujardin and Lehning, 2022). To overcome this limitation, downscaling techniques are needed to bridge the scale gap between forcing data and the spatial resolution at which snow models are run (Kruyt et al., 2022). The choice of downscaling methods for different meteorological variables is especially important in modeling mountain snow cover dynamics, as several studies have shown that resolving terrain effects on wind, radiation, and precipitation is crucial in simulating seasonal snow cover dynamics on the mountain slope scale (Schneiderbauer and Prokop, 2011; Musselman et al., 2015; Vionnet et al., 2017; Mott and Lehning, 2010; Reynolds et al., 2020; Carletti et al., 2022). Therefore, downscaling techniques need to be carefully chosen to accurately represent the impact of topography on snow accumulation and ablation, especially in complex mountainous regions.
Various modeling strategies have been developed to account for snow accumulation on mountain slopes at different resolutions, ranging from hundreds of meters to a few meters (e.g., Winstral and Marks, 2002; Musselman et al., 2015). Some models use parameterizations to adjust snowfall based on topographic parameters (Winstral and Marks, 2002; Helbig et al., 2015), while others explicitly represent snowfall and redistribution processes (Essery et al., 1999; Durand et al., 2005; Pomeroy et al., 2007; Liston et al., 2007; Lehning et al., 2008; Sauter et al., 2013; Vionnet et al., 2014; Comola et al., 2019; Marsh et al., 2020; Vionnet et al., 2021). However, explicit representation of wind-induced snow accumulation processes can be computationally expensive and not practical for operational snow-hydrological models, which need to run multiple times a day on large model domains with short computation times. Therefore, a balance needs to be struck between physics-based process representation and model efficiency. To address this, sub-grid parameterizations have been proposed to represent snow depth variability at the mountain ridge and slope scale for snow cover models operating at kilometer scales. These approaches have been applied in various applications to improve the representation of snow depth variability (Liston, 2004; Lehning et al., 2011; Helbig and van Herwijnen, 2017; He and Ohara, 2019).
Data assimilation is a valuable tool for improving the accuracy of snow cover predictions in operational snow cover models. It allows for the integration of observational snow data into the model to correct for biases and errors in the observed precipitation field, which can significantly enhance the accuracy of snow model predictions (Magnusson et al., 2014; Griessinger et al., 2016; Winstral et al., 2019). One of the key advantages of data assimilation is its ability to combine model predictions with observational data, thereby reducing uncertainties and improving the overall reliability of the model output. This approach enables the model to account for the limitations of the observational network and to estimate the snow cover conditions in areas with limited or no observations.
We would like to introduce a model framework that has been developed for operational snow hydrological modeling in Switzerland. This framework provides input data for national and regional flood and avalanche forecasting and is also used for various research applications. It is a prime example of a snow hydrological model that combines downscaling strategies, process representation and data assimilation to optimize the accuracy of model predictions while maintaining computational efficiency.
The framework comprises models of different complexities that are dedicated to different modeling strategies and purposes. In this context, we will present the strategies for data assimilation, downscaling techniques, and sub-grid parametrizations of relevant processes that are used to achieve an optimal balance between process representation and computational requirements in an operational snow hydrological model setting.
The model framework is used operationally to forecast snow cover dynamics in Switzerland. However, the individual model components are also utilized for many different research applications (e.g., Griessinger et al., 2016; Arnoux et al., 2021; Schirmer et al., 2022). This highlights the versatility of the framework and the importance of developing models that can meet multiple requirements simultaneously. It is noteworthy that the success of this model framework is attributed to its ability to incorporate multiple techniques into one cohesive framework, which results in improved model accuracy and efficiency.
2 The operational snow-hydrological model framework
The Swiss Operational Snow-Hydrological Service (OSHD) was originally established to meet the demand for Swiss-wide daily forecasts on snow distribution and snow melt, which are essential for national flood forecasting in Switzerland. In addition, the OSHD has been at the forefront of model development, and its framework and resulting datasets have been used for various research applications. Overall, the OSHD has become an invaluable resource for flood forecasting in Switzerland and has contributed significantly to scientific knowledge in the field of snow hydrology.
The development of a snow hydrological model system in Switzerland was made possible by several key factors, including the high-alpine terrain of the Swiss Alps, the availability of exceptional station data, especially the high density of high-quality snow stations at mid to high elevations, and access to high-resolution meteorological input data from the Swiss regional weather model and various reanalysis products provided by the Federal Office of Meteorology and Climatology MeteoSwiss. Based on these prerequisites a OSHD modular model framework was developed that combines snow modeling with data assimilation techniques and downscaling methods.
This section will provide a comprehensive overview of the OSHD model framework, including its individual aspects. We will begin by introducing the physics-based OSHD model chain (Section 2.1), which represents the benchmark for this publication and has undergone several years of development to reach its latest stage. Next, we will provide detailed information on the representation and parameterizations for snowpack processes (Section 2.1.1), snow cover fraction (Section 2.1.2), and forest snow processes (Section 2.1.3). Additionally, we will describe the climatological model chain (Section 2.2) in detail to provide a comprehensive understanding of the OSHD model framework.
2.1 The physics-based OSHD model chain for seasonal snow cover modeling
The physics-based FSM2oshd model (https://github.com/oshd-slf/FSM2oshd) solves the complete mass and energy balance of the snowpack for open and forested areas (Mazzotti et al., 2020a; Mazzotti et al., 2020b) at an hourly temporal resolution (Figure 1, FSM2oshd). FSM2oshd was originally based on the FSM2 snow cover model (Flexible snow model, https://github.com/RichardEssery/FSM2), with adapted snowpack process parameterizations and new model components for snow cover fraction (Helbig et al., 2021a) and for forest processes (Mazzotti et al., 2021; Mazzotti et al., 2023; Webster et al., 2023). In its operational setting the physics based model chain is run at a horizontal resolution of 250 m, but is also applied at higher resolutions (up to 25 m) for research purposes. The current physics-based OSHD model chain was initiated by Magnusson et al. (2015) who built a Swiss snow model framework suitable for predicting daily SWE and snowmelt using the first multi-model framework of physically based energy-balance models, JULES Investigation model (JIM), from Essery et al. (2013). The spatialized version of JIM was set up to run with downscaled numerical weather prediction data (NWP) and became the first operational OSHD version.
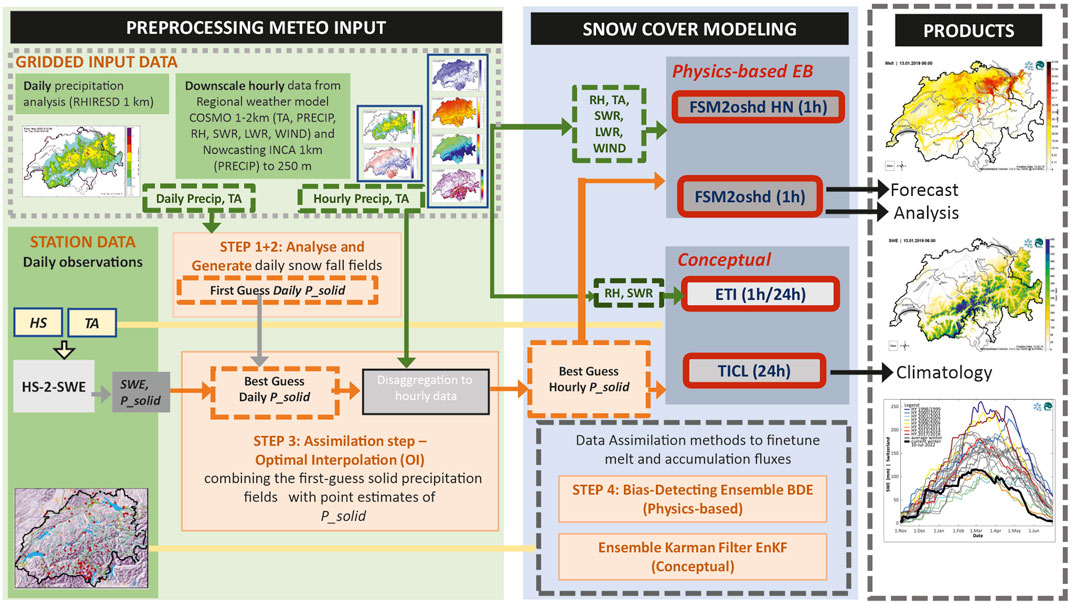
FIGURE 1. General OSHD model framework including data assimilation (orange boxes) for solid precipitation (P_solid), snow depth (HS) and air temperature (TA) and meteorological input from regional weather and nowcasting models such as relative humidity (RH), short- and longwave radiation (SWR, LWR), precipitation and wind. Input sources are shown for the physics-based multi-layer snow model (FSM2oshd, FSM2-HN) combined with Bias-Detecting Ensemble (BDE) and the conceptual models based on temperature index models (TICL, ETI) and the Ensemble Kalman filter (EnKF).
The performance of JIMoshd was evaluated in a study by Griessinger et al. (2019), who ran the latest version of the model at a spatial resolution of 250 m and temporal resolution of 1 h. The input was then fed into hydrological StreamFlow model simulations for 25 catchments in Switzerland. The study found that daily discharge simulations using StreamFlow were more accurate when JIMoshd simulations were updated with snow model mass and energy fluxes using snow observations with optimal interpolation (OI; Magnusson et al., 2014) or the bias-detecting ensemble approach (BDE; Winstral et al., 2019). In another evaluation, JIMoshd simulated snow cover evolution at a spatial resolution of 1 km and showed good seasonal agreement with various spatial snow cover data. This ranged from airborne-acquired fine-scale snow depth data to satellite and terrestrial imagery for Switzerland (Helbig et al., 2021b).
Essery (2015) developed the initial version of the Factorial Snow Model (FSM) to provide a more organized approach to the development of the snow model suite JIM. To enhance the model’s physics, particularly adding the representation of forest snow processes (for more details see Section 2.1.3) and output options, Essery (2015) introduced the Flexible Snow Model FSM2. The FSMoshd, originally based on FSM2, is capable of running on both a list of points and a regular grid simultaneously. Grid cells are divided into separate tiles, including forest-covered, open, and glacierized fractions (Figure 2, left), with fractions covered by large bodies of water where snow does not accumulate being excluded. The states and fluxes of each tile are computed as weighted averages of the respective states and fluxes of the grid cell. FSM2oshd includes local canopy cover fraction, hemispherical sky view fraction, wind attenuation, spatial patterns of shortwave and longwave radiation transfer through the canopy, and preferential deposition of snow in canopy gaps for the forest tiles, which have been improved by Mazzotti et al. (2020a), Mazzotti et al. (2020b). Station locations are linked to simulations at the point scale to assimilate local observations into the model (see Section 3.1).
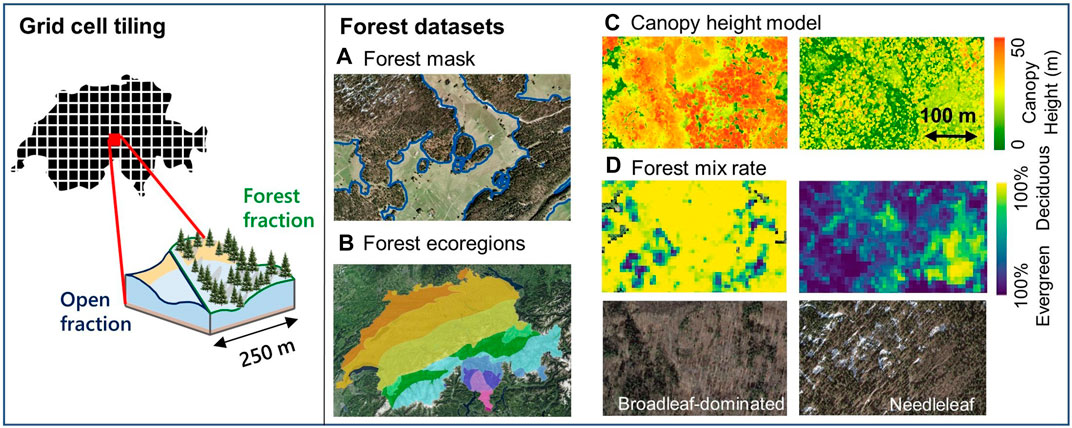
FIGURE 2. Conceptual sketch of a FSM2oshd model grid cell split into open and forest fractions (left) and forest datasets used to characterize the canopy in the forest fraction (right), including forest mask (A), the forest ecoregions map (B), and examples of canopy height model (C) and forest mix rates (D) for corresponding true color images for broadleaf (left examples) and needleleaf (right examples) stands.
The FSM2oshd model, which is based on physics, is currently being used for seasonal runs of recent water years (since WY 2016) to analyze and predict the spatio-temporal dynamics of snow water equivalent (SWE), snow depth (HS), snow melt runoff (SMR), and snow state (based on liquid water content) on a daily and hourly basis (Figure 3). Additionally, a new snow height model, FSM2oshd-HN, has been implemented to explicitly model the height of new snow over defined time frames, taking into account snowpack processes such as melting and compaction (Figure 4). The HN model simulates a HN board, where snow accumulates and settles over a specific time period. At the beginning of the accumulation period, the bottom boundary condition of the snowpack is initialized based on snow surface temperatures at the initialization times. In forecast mode, the HN model runs for lead times of 12, 24, 48, and 72 h, driven by COSMO forecasts (Figure 4). Due to the settling of the snowpack, the HN may be smaller after 48 h than after 24 h, despite continued snowfall. Furthermore, the HN model is run in the hindcast, using the data assimilation scheme described in Sect three. To allow for an optimal transition between hindcast and forecast data, precipitation is obtained from the nowcasting product INCA (Integrated Nowcasting through Comprehensive Analysis) (Meteoswiss, 2020) to drive the FSM2oshd-HN model. All other meteorological variables are forced by the COSMO analysis or forecast models. Within the operational model framework, the HN model is updated whenever new forecast and analysis data from COSMO becomes available.
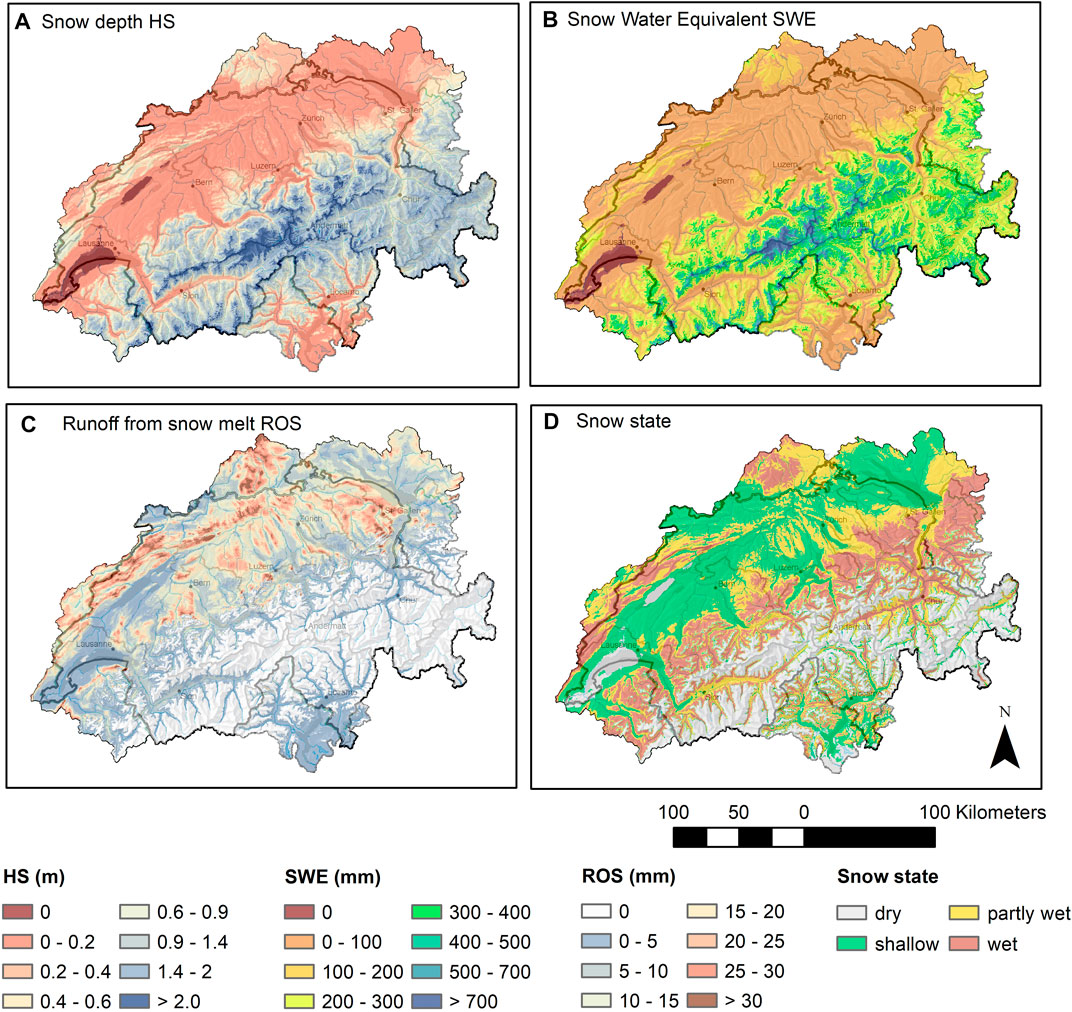
FIGURE 3. Main OSHD products (A) snow depth (HS), (B) snow water equivalent (SWE, (C) runoff from snowmelt (ROS) and (D) snow state, obtained from the physics-based model chain for a rain-on-snow event on 28 January 2021 (after a rain-on-snow event).
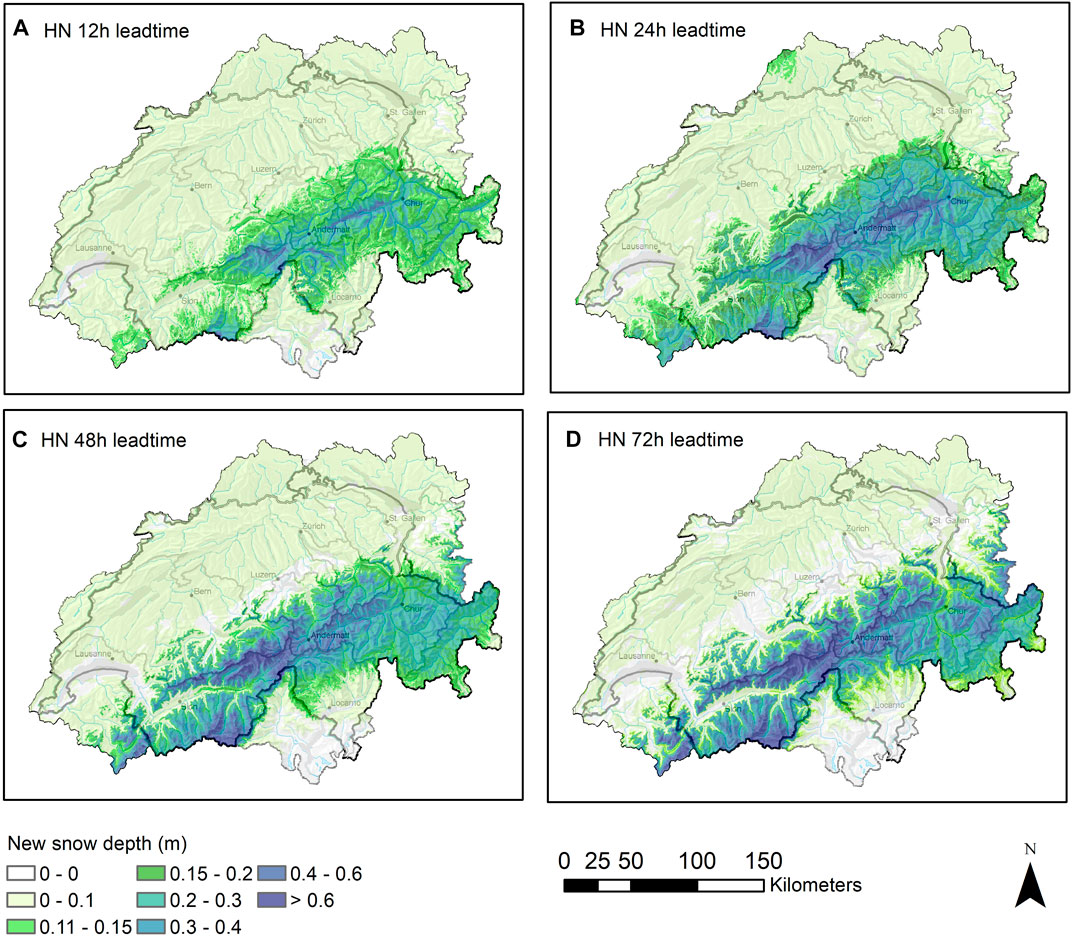
FIGURE 4. Maps of forecasted new snow depths for lead times of 12 (A), 24 (B), 48 (C) and 72 (D) h (8 December 2021).
The physics-based OSHD model chain has a wide range of applications, including operational use as well as in various research projects. These projects aim to study high-resolution snow melt processes in alpine terrain (Griessinger et al., 2019) and forested areas (Mazzotti et al., 2023), and to project future snow water resources and rain-on-snow events (e.g., Arnoux et al., 2021; Schirmer et al., 2022). The advantage of using a physics-based model is that it allows for more precise predictions of snow water inputs during snow melt events, particularly those driven by turbulent heat fluxes such as rain-on-snow situations. Additionally, the model can resolve radiation differences between slopes, which significantly increases the spatial variability of snow melt in the spring season (see Figure 5). Compared to temperature-index based models, which typically predict much lower snow melt during rain-on-snow events (as shown in Figure 6), the physics-based model chain appears to better capture snow melt processes during complex meteorological conditions.
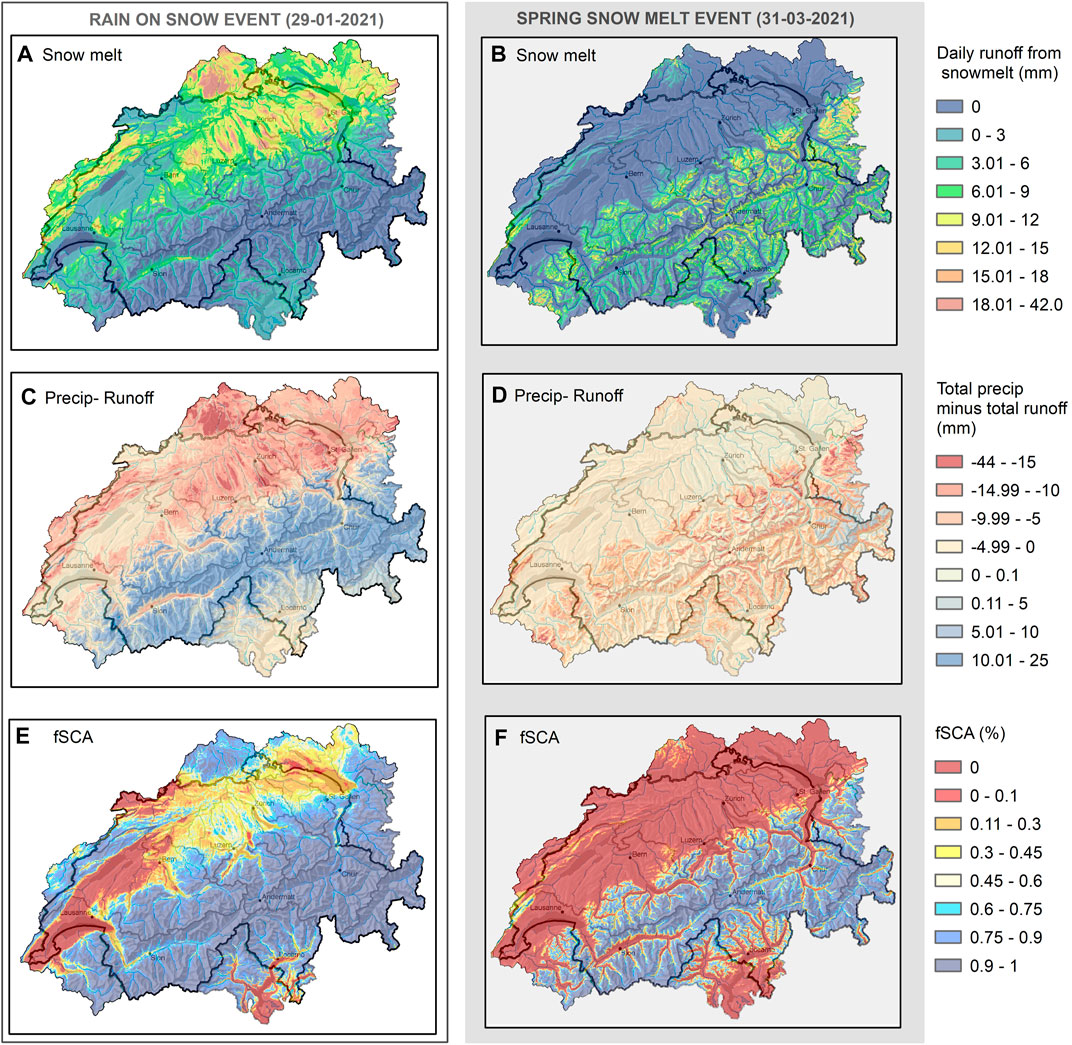
FIGURE 5. Model output of the physics-based OSHD model chain after a rain-on-snow event (A,C,E) and for a spring snowmelt event (B,D,F). Model results present runoff from snowmelt (A,B), total precipitation minus total runoff where positive values indicate that precipitation is stored as snow and negative values indicate that precipitation contributes to total runoff (C,D) and fraction of snow covered areas fSCA (E,F).
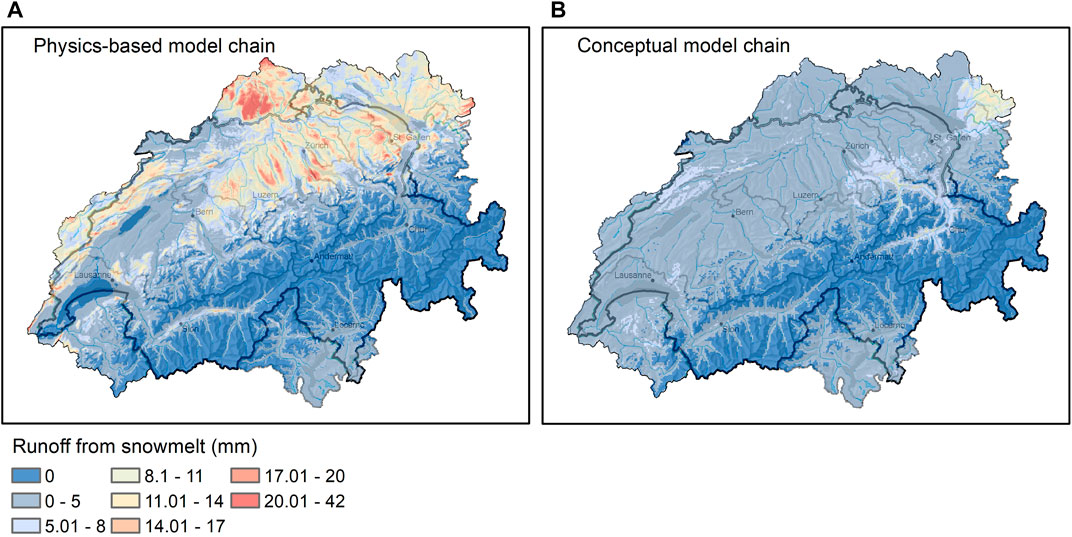
FIGURE 6. Runoff from snowmelt for a rain-on-snow event predicted by the physics-based (A) and the conceptual (B) model chain.
The physics-based model chain used for snowpack simulation relies heavily on precise and high-resolution information about the local meteorological conditions that affect the mass and energy balance of the snowpack, both spatially and temporally. For instance, the FSM2oshd model requires hourly data on various parameters such as air temperature, air pressure, relative humidity, total precipitation, wind speed, shortwave and longwave radiation, for each grid cell (as shown in Figure 1). Although such detailed information can be obtained by downscaling the high-resolution (1–2 km) COSMO forcings, it is important to note that these forcings may still contain errors inherited from the atmospheric models. This is especially true for mountainous regions, where NWP models often lack assimilation of surface observations.
To minimize errors in the simulation, snow data assimilation can be used to improve the accuracy of solid precipitation by correcting it before running the snowpack model (front-end) or during the execution of FSMoshd (back-end), utilizing the wealth of snow depth and 2 m air temperature data available in Switzerland and neighboring countries. The procedure for solid precipitation data assimilation is detailed in Section 3 (as shown in Figure 1).
2.1.1 Snowpack process parameterizations
A suitable combination of parameterizations is chosen in FSM2oshd for predicting snow water equivalent and snow melt based on the study of Magnusson et al. (2015) and recent results presented by Mazzotti et al. (2020a), Mazzotti et al. (2020b). For further optimization, available FSM2 parameterizations were either adapted to our specific needs, or new parameterizations were implemented as outlined below.
We use or adapted the following choice of snowpack process parameterizations in FSM2oshd which were originally implemented in FSM2:
We model the thermal conductivity of the snowpack using the methods of Douville et al. (1995) as originally implemented in FSM2.
We compute the turbulent exchange of heat and moisture between the snow and atmosphere using the original implementation of the Richardson parameterization (FSM2) following Louis (1979). However, we have made an important modification to this parameterization by introducing a maximum threshold of 0.2 for the bulk Richardson number. This is crucial to prevent the stability-induced shutdown of turbulent fluxes and the resulting unrealistic low surface temperatures that can occur during calm wind conditions (Martin and Lejeune, 1998; Vionnet et al., 2012; Lafaysse et al., 2017). Our careful adaptation of the Richardson parameterization ensures that our model produces highly accurate results that are consistent with real-world observations.
We have implemented the following choices of snowpack process parameterizations in FSM2oshd:
Snow compaction: we have incorporated a modified version of the physically-based method from the Crocus snowpack model (Vionnet et al., 2012) into the FSM2oshd model. The purpose of this modification is to simulate the increase in snow density for each snow layer resulting from the weight of overlying snow and the presence of liquid water. Our adaptation of the method takes into account the effects of liquid water on the snowpack, while keeping the factor that accounts for grain metamorphosis constant. This approach ensures that the simulation accurately reflects the effects of both overlying snow and liquid water on the snowpack density.
We have incorporated a fine-tuned version of the latest new snow density method used in the Crocus snowpack model (Vionnet et al., 2012) into our FSM2oshd software. This method takes into account air temperature and wind speed to accurately simulate the density of newly fallen snow. We carefully calibrated this function specifically for FSM2oshd by conducting an assessment against measurements of fresh snow that had settled over 24 h, while also considering the elevation.
We implemented a parameterization for the decay of snow albedo based on the empirical method following Douville et al. (1995). This method was tuned for FSM2oshd with an elevation dependency of the albedo for fresh snow, through an assessment against measured snow depletion rates in springtime.
We model snow hydraulics by implementing a bucket approach for routing liquid water through the snowpack following the methods of Boone and Etchevers (2001).
2.1.2 Snow cover fraction parameterizations
The spatial variability of snow cover varies significantly throughout a winter season, making it essential to consider the fraction of a grid, that is, covered by snow when modeling snow (as depicted in Figures 5E, F). A seasonal and scale-independent algorithm for the fraction of snow-covered area (fSCA) has been developed for open areas by Helbig et al. (2015); Helbig et al. (2021a); Helbig et al. (2021b). This algorithm tracks the history of snow depth (HS) and snow water equivalent (SWE) and takes into account the alternating accumulation and ablation phases (Helbig et al., 2021b).
The algorithm utilizes a subgrid parameterization of the spatial snow depth variability at the peak of winter based on the mean snow depth, mean-squared slope, and the subgrid correlation length of characteristic topographic features (Helbig et al., 2015). The subgrid parameterization was re-evaluated with high-resolution spatial snow depth data sets from seven independent geographic regions acquired at the peak of winter and demonstrated good performance but with scale-dependent results (Helbig et al., 2021a).
To address this, Helbig et al. (2021a) introduced scale-dependent parameters in the peak of winter parameterization of Helbig et al. (2015) to account for the maximum spatial snow depth variability. This adjustment allows the hyperbolic tangent fSCA parameterization to be applied reliably for grid cell sizes ranging from 200 m to 5 km.
The current approach for estimating the fraction of snow-covered areas in forested regions uses the simple hyperbolic tangent model, which was first implemented in FSM2 (Essery, 2015). Although this approach works reasonably well, it does not take into account the complex structure of forests, and therefore there is a need for a more refined parametrization method. To address this limitation, we plan to enhance the model in the future by developing a fSCA parametrization approach that explicitly accounts for forest structure.
2.1.3 Forest snow process representations
Simulations of the forest-covered grid cell fraction with FSM2oshd include all processes by which the presence of a forest canopy affects mass and energy exchange between the atmosphere and the sub-canopy snowpack: Interception of snowfall in the canopy and its subsequent sublimation to the atmosphere or unloading to the ground, transmission of shortwave radiation through and enhancement of longwave radiation by the canopy, and wind attenuation. The forest canopy is represented as a one-layer canopy whose energy balance is coupled to that of the below-canopy snowpack via a canopy air space (i.e., the vegetation and canopy air space temperatures and the canopy air space specific humidity are additional model state variables). This is common in land surface models, for example, CLASS (Bartlett et al., 2006), JULES (Best et al., 2011), CLM (Lawrence et al., 2019), ISBA (Boone et al., 2017), Noah-MP (Niu et al., 2011). The parametrizations of canopy-mediated processes used in FSM2oshd are well-established in literature: treatment of canopy snow interception and unloading relies on the Hedstrom and Pomeroy (1998) model, transmission of diffuse shortwave radiation and enhancement of longwave radiation are dictated by bulk canopy transmissivity properties (as, e.g., in Bewley et al., 2010), and turbulent exchange is treated with a bulk aerodynamic scheme including a composite exp-log wind profile to account for attenuation by the canopy (as, e.g., in Mahat et al., 2013). The representation of canopy structure, however, was adapted to allow for more realistic dependencies of individual processes on local canopy characteristics by using diversified, process-specific canopy metrics, as described in Mazzotti et al. (2020a), Mazzotti et al. (2020b); Mazzotti et al. (2021). This is especially important in heterogeneous forests.
To characterize horizontal, vertical, local, and stand-scale canopy features at each modeled location, canopy structure input to FSM2oshd comprises canopy height (Hc), canopy cover fraction (Fc), sky-view fraction (Vf), and leaf area index (LAI, parametrized from Hc, Fc and maximum LAI values specific to forest type and ecoregions). In particular, Mazzotti et al. (2020b) highlighted the benefit of additionally providing a time-varying transmissivity for direct shortwave radiation through the canopy (Td(t)) as input FSM2oshd. Transmissivity time series are computed by an external radiation transfer model that solves the process explicitly. Incorporating these in FSM2oshd thus avoids the simplification of the process representation in the model through a parameterization.
Mazzotti et al. (2021) further showed that accurate process representation at intermediate model resolution can be achieved by using canopy descriptors and time-varying transmissivities computed at high spatial resolution and subsequently averaged (arithmetic mean) over the entire (forest fraction of each) grid cell. Canopy structure input computed at ‘evaluation points’ with 10 m spacing is used as the basis for aggregating over the entire grid cells. The canopy structure descriptors Fc and Hc are computed from a high-resolution canopy height model (see below) over areas of 5- and 50-m radii around each evaluation point as described in Mazzotti et al. (2020a). Vf and Td(t) are calculated using the model CanRad (Webster et al., 2023). CanRad calculates synthetic hemispheric images at each evaluation point, by first determining the height of the canopy horizon line around the evaluation point from the canopy height model, and subsequently binarising a probability of Td(t) below the horizon line based on canopy thickness across the hemisphere and tree crown volume density. Vf is calculated using the methods described in Essery et al. (2008) and Td(t) is calculated by overlaying the image with the position of the complete annual solar track as in Jonas et al. (2020) and Webster et al. (2020). The use of spatially averaged, time-varying transmissivities is efficient because computationally expensive transmissivity calculations at the evaluation points can be performed offline, without compromising operational model run times.
The national forest datasets used to derive canopy structure metrics across the OSHD domain (Figure 6) are described in detail by Waser et al. (2015), Waser et al. (2017). A canopy height model (Figure 2C) was calculated at 1 m resolution based on LiDAR data covering all Switzerland. The data consists of a nationwide acquisition from 2003 and an ongoing campaign (2017–2023), with supplementary data from individual cantonal acquisitions. LiDAR point density ranges from 1 to 30 pt/m2. The canopy height model is continually updated as new lidar data is acquired. Partitioning of grid cells into open and forest-covered fractions is based on a forest mask (Figure 2A), with open areas influenced by the presence of forest included in the forest fraction. Further datasets were used to include information on canopy properties across the domain, which affect leaf area index and tree crown densities. A nationwide forest mix rate dataset (Figure 2D) calculated using machine learning and Sentinel 1 and 2 images served to discriminate between deciduous and evergreen forest types (Waser et al., 2017). Whether forest is needleleaf or broadleaf was determined based on a combination of the Swiss forest ecoregions dataset (Figure 2B) (https://opendata.swiss/en/dataset/waldstandortsregionen), the Copernicus forest type product (https://land.copernicus.eu/pan-european/high-resolution-layers/forests), and elevation (Figure 2). Species-specific LAI values are based on a product derived from Sentinel-3 (https://land.copernicus.eu/global/products/lai).
2.1.4 Sub-grid parameterizations of meteorological processes for larger-scale modeling at the kilometer scale
FSM2oshd was advanced by integrating sub-grid parameterization for unresolved processes for coarser-scale applications and downscaling schemes for research applications at higher resolutions (Table 1).
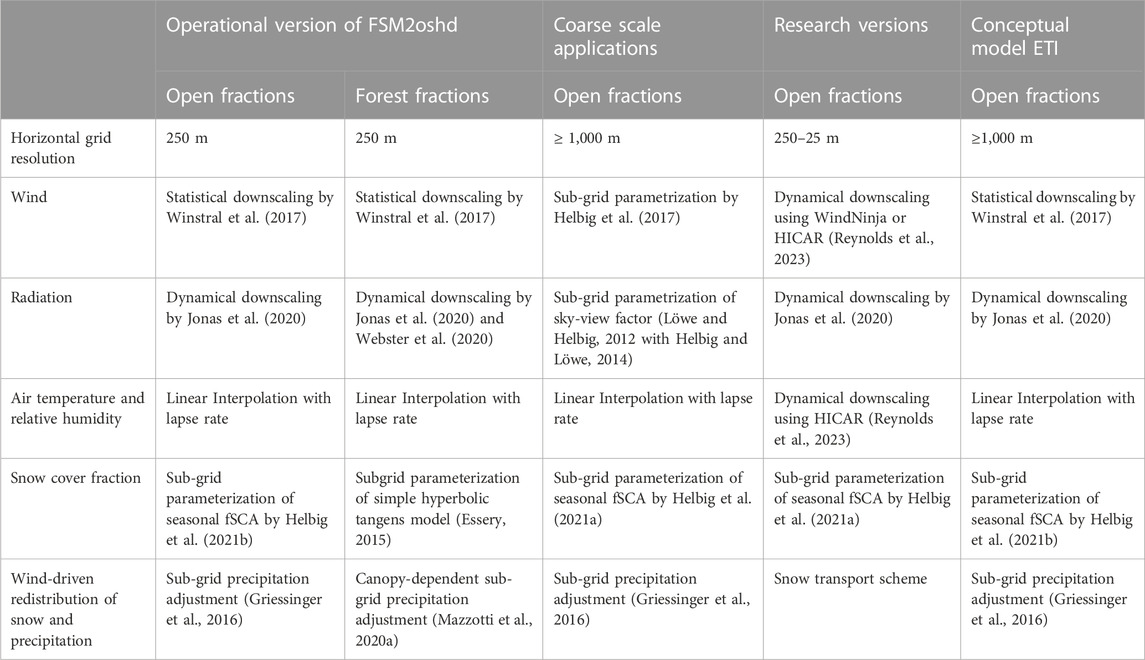
TABLE 1. Downscaling methods and parameterization options used in the OSHD model framework for different model chains and modeling purposes.
2.1.4.1 Radiation
In case of coarser scale simulations (kilometer scale) when radiation is not dynamically downscaled, the subgrid influence of topography on radiative fluxes is described using a quasi-analytical method presented in Löwe and Helbig (2012). First-order terrain reflections, partial shading, and limited sky are parameterized subgrid topographic impacts using the radiative flux components. Only a subgrid sky view factor, spatial mean-squared slope, and mean albedo, as well as the Sun elevation angle, are needed in the grid cell of the coarse-scale model. If only global radiation is available for the coarse grid cell, the radiative flux components, shortwave direct and diffuse sky radiation, are obtained from applying a decomposition model at the coarse scale as described in Helbig et al. (2010). For the subgrid sky view factor the formulation of Helbig and Löwe (2014) was used, which describes the spatially averaged sky view factor for a given grid cell over complex topography. The parameterization is solely based on computationally cheap terrain parameters, namely, the correlation length of sub-grid topographic features and the mean-squared slope in the grid cell of the coarse-scale model.
2.1.4.2 Wind
For larger-scale applications when statistical downscaling of wind is not suitable, the FSM2oshd model further allows for parameterizing the sub-grid effect of terrain on the local wind speeds (Helbig et al., 2017). The subgrid parameterization describes terrain drag for surface wind speed based on systematically analyzed wind fields over many synthetic topographies. To describe the unresolved topographic impacts in coarse-scale wind speed, the sub-grid parametrization of the sky view fraction (Helbig and Löwe, 2014) was used as a topographic sub-grid scaling parameter.
2.2 The conceptual model chain for snow climatological modeling
The conceptual model chain was developed within the OSHD model chain (Figure 1, OSHD_TICL) for climatological runs to compare snow water resources in Switzerland of water years since 1998 at a horizontal resolution of 1,000 m (Figure 5). The conceptual model is based on an efficient temperature-index (TI) snow model with SWE and liquid water as main state variables and only requires precipitation and temperature as forcing data, that is, available for a longer historical period than the physics-based model chain. The model is described in detail in Magnusson et al. (2014); Griessinger et al. (2016). The conceptual models include a density model (HS-2-SWE, see Section 3.1.1) for converting observed changes in snow depth to solid precipitation fluxes and considering decreasing snow depth due to compaction (Jonas et al., 2009). The temperature index method is used to compute the daily amount of snow melt occurring.
Our model framework uses an Ensemble Kalman filter (EnKF) to update simulated melt rates and two model state variables (SWE and liquid water content) based on observed snow water equivalents computed from snow depth records. We run 100 ensemble members for the point observation sites and the model grid, using interpolated input data perturbed with spatially correlated errors (Magnusson et al., 2014).
The model framework employs two conceptual snow models, OSHD_TICL (Climatological Temperature Index Model) and OSHD_ETI (Enhanced Temperature Index Model). In our operational setting, we use OSHD_TICL, which compares SWE of different years using the same data sources available since 1998 (Figure 7). We ensure maximum temporal constancy between individual water years since 1998 by considering only stations and data sources that feature data since 1998. Thus, OSHD_TICL only uses gridded daily precipitation data (RHIRESD; MeteoSwiss, 2019) and observed solid precipitation fluxes. Further details on data assimilation procedures for precipitation are provided in Section 3. To account for the sub-grid spatial variability of snow, we use the seasonal and scale-independent algorithm for the fraction of snow-covered area (fSCA) developed by Helbig et al. (2015) (see Section 2.1.2).
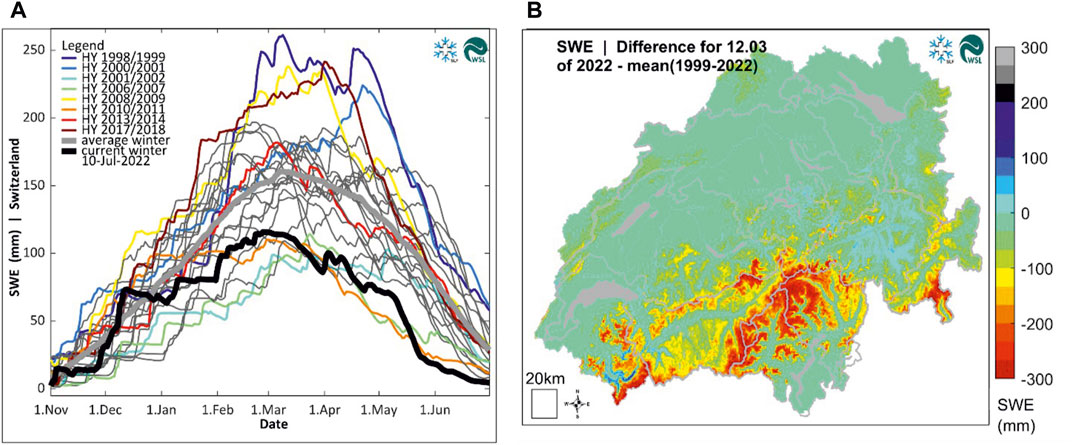
FIGURE 7. Main products from the conceptual model (TICL). Yearly evolution of Snow Water Equivalent (SWE) of different years (A). Map of the difference between the SWE and the mean SWE for the years 1999-2022 (B).
We also developed the non-operational ETI model to improve the representation of the precipitation phase. This model uses hourly air temperature and precipitation data to discriminate between rain and snowfall. Forcing data for air temperature, relative humidity, and shortwave radiation is downscaled from respective fields from COSMO (see Section 4).
A full data set for climatological runs, providing maps of snow water equivalent and snow cover fraction from water year 1998–2022 from the OSHD_TICL model, is available at EnviDat. doi:10.16904/envidat.401.
3 Data assimilation procedure
Within the OSHD model framework, different data assimilation methods for precipitation exist which are used depending on the model requirements of the physics-based and conceptual model chains (Figure 1). The challenge of data assimilation within the OSHD framework is to make optimal use of available point observation of snow depth and air temperature, gridded products of precipitation and air temperature, and analysis and forecast products of the regional weather model. The data assimilation of point scale snow depth data and gridded precipitation products requires four steps of input and output processing (Figure 1): 1) inferring daily snowfall estimates from snow depth observations at stations, 2) providing an optimal first-guess solid precipitation field from gridded air temperature and precipitation products, 3) an assimilation step combining the first-guess solid precipitation fields with the point estimates of solid precipitation, and 4) updating calculated mass and energy fluxes with snow observations.
3.1 Inferring solid precipitation rates from observed snow depths
In order to gain information on daily solid precipitation rates, we convert daily snow depth records (HS) to snow water equivalent (SWE) using a density model (HS-2-SWE) (step 1, Figure 1). This method uses station data (Figure 8) from IMIS (Intercantonal Measurement and Information System) snow monitoring networks including automatic stations and manual observer data or from SwissMetNet (MeteoSwiss). Furthermore, data from DWD (Deutscher Wetterdienst, German national meteorological service), ZAMG (Zentralanstalt für Meteorologie und Geodynamik, Austrian national meteorological service) and MeteoFrance (French national meteorological service) are operationally used for regions outside the Swiss border. The station network consists of 444 stations measuring snow depths (HS stations) and 256 stations measuring air temperature (TA-stations). For the climatological runs the number of HS stations is reduced to 349 stations and TA-stations to 255 providing a full time-series of quality-controlled data from WY 1998 until today. Snow depth and air temperature data are extracted at daily timesteps and are quality checked.
We convert daily point measurements of snow depth to water equivalent using a model based on the methods presented by Martinec and Rango (1991). The adapted model describes the accumulation and settling of the snowpack for each layer. The density model applied for each layer has been calibrated based on data from the over 10,000 snow profiles used in the study by Jonas et al. (2009). We can compute snowfall and melt rates from the increments/decrements in snow water equivalent. For an example of the computed solid precipitation and melt time series, see Figure 2 in Magnusson et al. (2014). Note that melting and accumulation cannot occur simultaneously with this simplified description of the snowpack development. At the same time, this model only requires daily snow depth records without the need for any meteorological data.
3.2 Combining available gridded precipitation products
To carry out the assimilation outlined in Step 3 of Figure 1, obtaining the best possible prior estimate for solid precipitation on the model grid is crucial (Step 2, Figure 1). To achieve this, we gather data from various sources, including gridded daily precipitation products (RhiresD) from MeteoSwiss and interpolated temperature fields based on large station datasets. RhiresD provides a spatial analysis of daily precipitation over the OSHD domain, utilizing data from heated rain gauges, typically 430 within and 220 outside of Switzerland daily. The daily temperature interpolation follows the methods presented by Frei (2014) and employs data from over 300 stations covering most of the elevation range given by the OSHD domain. We combine these daily products with hourly data from COSMO 1 E to improve, for instance, precipitation phase determination to obtain the best possible priors of daily snowfall on the OSHD grid, which are further used in the optimal interpolation step described below.
3.3 Optimal interpolation of solid precipitation estimates at stations
The gauge network utilized in the gridded precipitation products of MeteoSwiss has a limited representation of elevations above 1,200 m. Additional snow depth data from the snow observation networks, particularly the IMIS network, are incorporated to enhance the accuracy of solid precipitation information at high altitudes. The first-guess or background fields of daily snowfall obtained in the previous step (refer to Section 3.2) serve as the basis for assimilating point solid precipitation rates inferred from snow depth measurements (refer to Section 4.1). An optimal interpolation scheme (OI) is employed for the assimilation, efficiently updating the background field utilizing observations (step3, Figure 1). The OI method considers apriori-defined covariances between errors in the gridded input fields and point observations, accounting for observation errors. We assume errors in the precipitation input fields to be spatially correlated, while observation errors are assumed to be spatially uncorrelated (Magnusson et al., 2014). The optimal interpolation scheme generates a best-guess daily solid precipitation field, incorporating information from the snow depth stations.
To account for the effects of terrain on precipitation and snow redistribution by wind and avalanches, distributed precipitation multipliers are utilized as snow observations are commonly conducted at flat field sites. To implement the sub-grid precipitation adjustment, snow depths obtained from airborne lidar acquisitions in the European Alps, as presented in Grünewald and Lehning (2015), are employed. The scaling method, following the iterative scaling approach proposed by Vögeli et al. (2016), corrects for snow accumulation and is based on slope calculations conducted at 25 m and then upscaled to a resolution of 250 m. It should be noted that the scaling method is only applied to solid precipitation.
In Figure 9, the impact of the assimilation procedure described above on the accuracy of snow simulations at 45 measurement locations spanning an elevation range of 1,195–2,690 m.a.s.l. from 2015-09-02 to 2022-8-01 is presented. The optimal interpolation step leads to a reduction in the root-mean-squared error and bias for all elevation bands. It should be noted that the error reduction is smallest for the two highest altitude bands, likely due to lower station density at these elevations and, therefore, fewer data available for assimilation. In Figure 10, we present a comparison between the time series of modelled snow depths with and without applying OI technique, based on observations for Water Year (WY) 2022. To ensure robust analysis, station data is aggregated for 500 m elevation bands. The results clearly highlight the significant benefits of employing optimal interpolation in operational snow modeling, particularly in reducing errors associated with solid precipitation. These findings further corroborate and validate the earlier conclusions reported in studies conducted by Magnusson et al. (2014) and Griessinger et al. (2019).
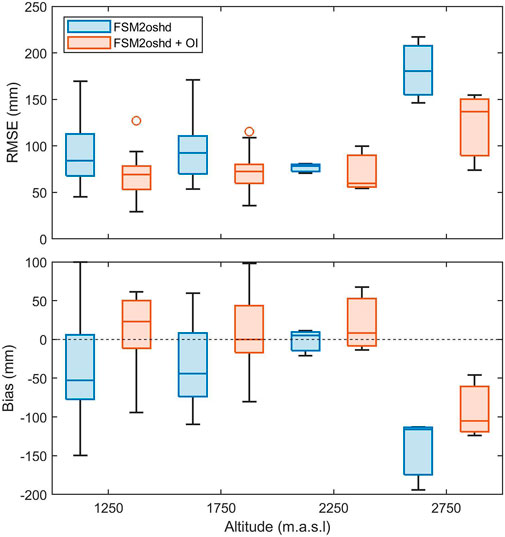
FIGURE 9. Root-mean-squared-error and bias for snow water equivalent at 45 observation sites covering an altitude range from 1,195 to 2,690 m.a.s.l. for the open loop (FSM2oshd) and data assimilation run using optimal interpolation (FSM2oshd + OI). The optimal interpolation step reduces the errors in all elevation bands showing the added value of this assimilation procedure to the operational system.
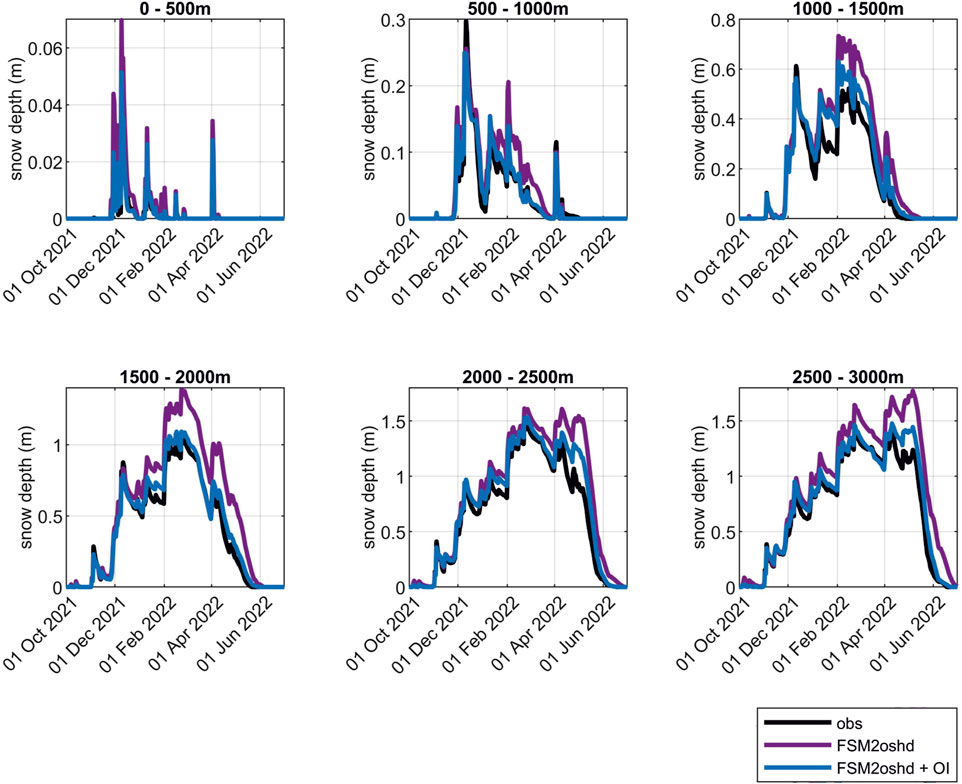
FIGURE 10. Comparison of modelled snow depths at stations aggregated for 500 m elevation bands using FSMoshd with and without Optimal Interpolation (OI) against measured snow depths for Water Year 2022 (WY 2022). The figure demonstrates the impact of OI on improving the accuracy of snow depth estimates.
3.4 The Bias-Detecting Ensemble (step 4)
The OSHD-EB model chain employs the Bias-Detecting Ensemble (BDE) method to enhance snow accumulation and depletion predictions by updating mass and energy fluxes with snow observations (Winstral et al., 2019). The BDE method evaluates the entire modeling chain to identify potential biases in the meteorological driving data and snow model, serving as an indirect approach for detecting biases in solid precipitation and surface energy inputs to the model.
In the first step, the BDE algorithm determines whether accumulation, melting or settling dominates the observed snow depth development. An ensemble of simulations is then conducted and evaluated against the snow observations, with precipitation perturbations evaluated on snow days and only energy flux perturbations on melt days. Daily history of the best-performing biases based on simulated and measured snow depths at each site is updated, with the assumption that these biases exhibit temporal coherence. Daily values are back-averaged and spatially interpolated to the modeling grid (Step 4, Figure 1).
Griessinger et al. (2019) have demonstrated that the use of the BDE method significantly improves the model performance of runoff modeling results for energy balance snow simulations.
4 Downscaling methods of input data
The physics-based snow cover model requires total precipitation, air temperature, incoming shortwave radiation, incoming longwave radiation, wind velocity, air pressure and relative humidity at an hourly resolution and spatial resolutions at the sub-kilometer scale. As COSMO1 data at a horizontal resolution of 1 km is not resolving the effect of high-resolution terrain on wind and radiation, statistical and dynamical downscaling schemes were developed. The operationally used downscaling schemes for radiation and wind are a promising alternative to subgrid parameterization, which is primarily used for larger-scale applications (Section 5). The operational version of FSMoshd applies downscaling methods for meteorological parameters as detailed in Table 1.
4.1 Radiation
For model applications with target resolutions at the sub-kilometer scale, radiation is dynamically downscaled using topographical information from a 25-m resolution digital elevation model accounting for topographic shading and the inclination of the respective grid cell following Jonas et al. (2020). To this end, the DEM is evaluated for each location of interest and point in time to determine whether the Sun is visible (weather permitting) or obscured by terrain. This enables calculating incoming shortwave radiation within terrain from either the diffuse component or the diffuse and direct components of the above-terrain radiation. The local hemispherical sky view fraction is used to constrain diffuse shortwave radiation and partition incoming longwave radiation into the fractions sourced from the sky and the terrain. In the operational setting, radiation per inclined surface is re-upscaled from 25 m to the current target model resolution of 250 m (Figure 11).
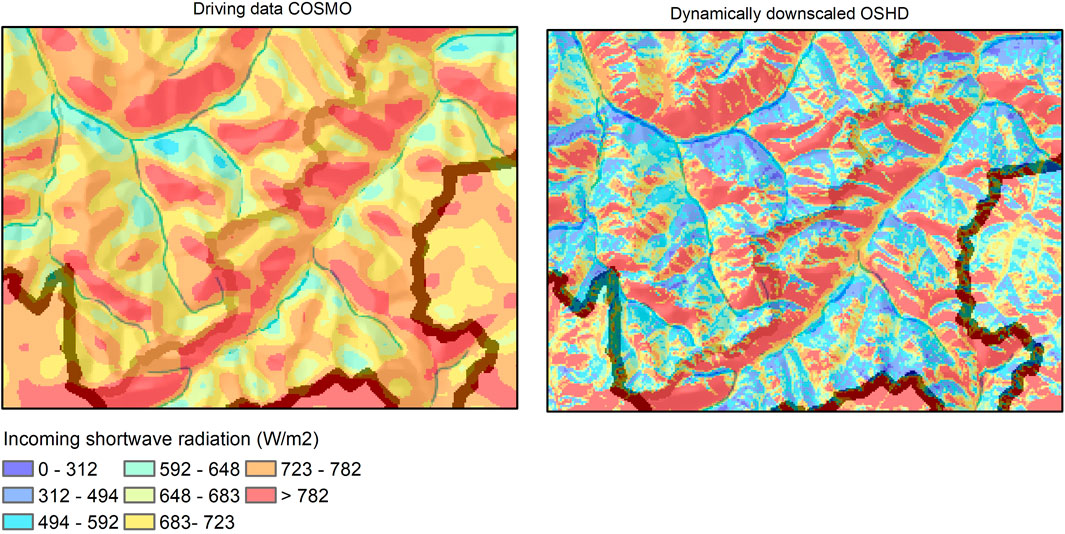
FIGURE 11. Incoming shortwave radiation obtained from COSMO and dynamically downscaled within the OSHD model framework applying the HPEval model (Jonas et al., 2020).
4.2 Wind
For higher-resolution model applications (sub-kilometre scale), two wind downscaling methods are available within the OSHD model framework. The two statistical approaches (Helbig et al., 2017; Winstral et al., 2017) aim to downscale gridded coarse-scale wind data to finer grids using information from the local topography. However, these two methods differ in the required wind data and topographic parameters. In the current operational model framework, the approach suggested by Winstral et al. (2017) is used.
The statistical approach, Winstral et al. (2017) developed uses local topographic information at a much higher resolution than present in the forecast wind data to delineate the spatial organization of biases in the forecast products. The local terrain is statistically linked with observed forecast biases at stations located at different terrain shading locations. The sub-grid or high-resolution terrain information is determined from a 25 m resolution DEM using two established terrain parameters: 1) the topographic position indices (TPI), which describes elevation relative to its surroundings, independent from wind direction (Jenness 2006); 2) the terrain parameter Sx which is a direction-dependent, slope-based assessment of topographic shelter and exposure capable of differentiating such slopes based on given wind directions. The Sx parameter has been used successfully to distribute hourly observed wind speeds across a modeling domain (Winstral and Marks, 2002; Winstral et al., 2009; Winstral et al., 2013; Fiddes and Gruber, 2014). The resulting wind exposure metrics effectively segregated high (ridges), moderate (slopes), and low wind speed sites (valleys). An optimization scheme is applied to further improve downscaled wind distributions, nudging the coarse-scale wind distributions from COSMO data to better match the observed wind speeds at stations (distributions and variability). The optimization method requires a reasonable number of wind stations in the domain and a new optimization whenever new forecast data is used.
As an alternative approach, the statistical downscaling scheme, described by Helbig et al. (2017), is available in the OSHD model framework. This method can downscale coarse-scale wind speeds from numerical weather prediction models to points or high-resolution grids by describing the impact of the surrounding resolved topography. Easily derived terrain parameters using the mean squared slope for each fine-scale grid and a parameter related to the Laplacian of terrain elevations are used to calculate a local topographic downscaling factor. Both terrain parameters relate to the sheltering and exposure of grid cells. Depending on the two terrain parameters, the downscaling scheme is based on millions of simulated ARPS (Advanced Regional Prediction System) fine-scale wind speed values on model topographies (Gaussian random fields), depicting a wide range of realistic terrain characteristics.
5 Discussion, conclusion and outlook
We have presented a multi-modular model framework for modeling snow cover dynamics. The model framework was developed for the purpose of operational snow hydrological modeling in Switzerland and is also used for providing daily new snow forecasts for avalanche warning and weather predictions. The model framework and associated data have been utilized in a variety of research applications. For instance, they have been used to study droughts (Toreti et al., 2023), project future snow water resources (Lüthi et al., 2019) and their hydrological implications (Floriancic et al., 2020), and project future rain-on-snow events (Schirmer et al., 2022). Additionally, they have been employed in studies examining the impact of snow cover dynamics on catchment hydrology (Freudiger et al., 2016; Griessinger et al., 2016; Jenicek et al., 2016; Arnoux et al., 2021) and mountain ecosystems (Xie et al., 2018; Schano et al., 2021), as well as the importance of snow melt dynamics in triggering cascading processes in high-alpine terrain under changing climate conditions (https://ccamm.slf.ch/de/cascading-processes.html).
The operational snow hydrological model framework consists of models of different complexity, which are dedicated to different modeling strategies and purposes. The physics-based model chain allows to capture aspect related snowmelt differences as well as high spatio-temporal melt dynamics during extreme weather situations such as rain-on snow or strong wind events. However, the conceptual model chain is still required for climatological runs to compare snow water resources of the last decades when atmospheric forcing data was not available. While the OSHD model framework makes optimal use of the exceptional data availability in Switzerland, the OSHD developments also accounted for the challenges involved with the large fraction of high-alpine terrain in Switzerland inducing a high complexity of snow-atmosphere interactions. We have thus demonstrated strategies for data assimilation, downscaling techniques and sub-grid parametrizations of relevant processes at the snow-atmosphere interface in order to capture relevant snow processes at the catchment and ridge scale. At the same time the model is designed to optimize computational efficiency to meet the requirements of an operational snow hydrological service in an alpine country.
The FSMoshd model is a leading-edge snow hydrological model that accurately captures forest snow processes, as demonstrated in recent studies (Mazzotti et al., 2020a; Mazzotti et al., 2023). However, there is still room for improvement in the model’s representation of slope and ridge-scale snow processes, such as preferential deposition of snowfall, snow drift, and avalanching. To address this, we are coupling the FSMoshd model with a new, high-resolution variant of the intermediate complexity atmospheric research model HICAR (Gutmann et al., 2016; Kruyt et al., 2022; Reynolds et al., 2023).
By incorporating the atmospheric model, we can better represent the interaction between ridge-scale terrain-induced winds, the advection of falling snow particles, and local-scale micro-physical processes. This coupling will allow us to account for the changing snow coverage and its impact on near-surface air temperatures. Moreover, recent advances in atmospheric measurement techniques (Haugeneder et al., 2022) have provided valuable insights into the dynamics of heat transport processes over patchy snow covers, which we will integrate into our model system to parameterize the effect of heat advection processes. In addition, coupling the model with a snow transport model will enable us to explicitly account for snow redistribution processes by wind and avalanches.
Physics-based models for snow hydrological modeling heavily rely on accurate meteorological input data. However, due to the inherent uncertainty in weather forecasting, ensemble weather forecasting has become a promising development for snow hydrological modeling. By providing probabilistic forecasts through ensemble simulations, decision-makers can gain valuable insights and make informed decisions based on the added value of the OSHD products (Buizza, 2008; Ramos et al., 2013). These approaches have also been proven to have scientific applications (Dumont et al., 2020). To fully account for all sources of uncertainty in snowpack modeling, it is essential to use meteorological ensemble input in combination with an ensemble of snowpack models (Vernay et al., 2015; Lafaysse et al., 2017; Aalstad et al., 2018; Kim et al., 2019). Integrating multiple sources of uncertainty can significantly improve the accuracy and reliability of snow hydrological modeling (Raleigh et al., 2015).
The model system can significantly benefit from the latest advancements in remote sensing products that provide high-resolution data on snow coverage, snow wetness, snow depths, and forest structures (Dozier et al., 2016; Lievens et al., 2019; Lievens et al., 2022). With the integration of data assimilation methods, the model system can effectively propagate information into underserved areas, particularly with particle filter-based approaches (Cluzet et al., 2022; Odry et al., 2022), by incorporating various measurement types, such as gauges, radars, satellites, and snow observations (Lundquist et al., 2019). This integration improves the accuracy of data assimilation procedures and enhances the representation of forest snow processes. Moreover, it allows for precise spatial validation of the model results, particularly crucial in mountainous terrains. Overall, the combination of remote sensing and data assimilation can steadily improve the model system.
Data availability statement
We are pleased to present two comprehensive datasets from the conceptual and physics-based models that cover the entirety of Switzerland at the envidat repository. The first dataset comprises the climatology on snow water equivalent, snow melt runoff and snow fraction spanning 1998-2022, with a spatial resolution of 1 km and daily temporal resolution (EnviDat. https://www.doi.org/10.16904/envidat.401). The second dataset includes snow depth (m), snow water equivalent (mm), runoff from snow melt (mm) and snow cover fraction. The data is spanning the water years 2016-2022 at a high spatial resolution of 250 m. Data are stored as daily results (EnviDat. https://www.doi.org/10.16904/envidat.404). The FSM2oshd code is available at https://github.com/oshd-slf/FSM2oshd.
Author contributions
RM prepared the manuscript and figures with contributions from all co-authors. All authors considerably contributed to the development of the OSHD model framework. TJ has played a pivotal role in establishing the OSHD and has consistently been at the forefront, leading the way in driving its model developments since its inception. All authors contributed to the article and approved the submitted version.
Funding
The OSHD model development and implementation was largely funded by the Swiss Federal Office for the Environment (FOEN). Part of the work was funded by the WSL Institute for Snow and Avalanche Research SLF. Open access funding by Swiss Federal Institute for Forest, Snow and Landscape Research (WSL).
Acknowledgments
We thank Christian Ginzler for processing the canopy height model and providing access to auxiliary vegetation datasets. We appreciate the discussions with Richard Essery related to the FSM2 model code. We thank the Federal Office of Meteorology and Climatology (MeteoSwiss) for providing COSMO and INCA data and daily precipitation data.
Conflict of interest
The authors declare that the research was conducted in the absence of any commercial or financial relationships that could be construed as a potential conflict of interest.
Publisher’s note
All claims expressed in this article are solely those of the authors and do not necessarily represent those of their affiliated organizations, or those of the publisher, the editors and the reviewers. Any product that may be evaluated in this article, or claim that may be made by its manufacturer, is not guaranteed or endorsed by the publisher.
References
Aalstad, K., Westermann, S., Schuler, T. V., Boike, J., and Bertino, L. (2018). Ensemble-based assimilation of fractional snow-covered area satellite retrievals to estimate the snow distribution at Arctic sites. Cryosphere 12, 247–270. doi:10.5194/tc-12-247-2018
Arnoux, M., Brunner, P., Schaefli, B., Mott, R., Cochand, F., and Hunkeler, D. (2021). Low-flow behavior of alpine catchments with varying quaternary cover under current and future climatic conditions. J. Hydrology 592, 125591. doi:10.1016/j.jhydrol.2020.125591
Barnett, T. P., Adam, J. C., and Lettenmaier, D. P. (2005). Potential impacts of a warming climate on water availability in snowdominated regions. Nature 438, 303–309. doi:10.1038/nature04141
Bartlett, P. A., MacKay, M. D., and Verseghy, D. L. (2006). Modified snow algorithms in the Canadian land surface scheme: Model runs and sensitivity analysis at three boreal forest stands. Atmosphere-Ocean 44 (3), 207–222. doi:10.3137/ao.440301
Bavay, M., Grünewald, T., and Lehning, M. (2013). Response of snow cover and runoff to climate change in high Alpine catchments of Eastern Switzerland. Adv. Water Resour. 55, 4–16. doi:10.1016/j.advwatres.2012.12.009
Beniston, M., and Stoffel, M. (2016). Rain-on-snow events, floods and climate change in the Alps: Events may increase with warming up to 4 C and decrease thereafter. Sci. total Environ. 571, 228–236. doi:10.1016/j.scitotenv.2016.07.146
Beniston, M., Farinotti, D., Stoffel, M., Andreassen, L. M., Coppola, E., Eckert, N., et al. (2018). The European mountain cryosphere: A review of its current state, trends, and future challenges. Cryosphere 12, 759–794. doi:10.5194/tc-12-759-2018
Best, M. J., Pryor, M., Clark, D. B., Rooney, G. G., Essery, R. L. H., Ménard, C. B., et al. (2011). The joint UK land environment simulator (JULES), model description – Part 1: Energy and water fluxes. Geosci. Model. Dev. 4, 677–699. doi:10.5194/gmd-4-677-2011
Bewley, D., Essery, R., Pomeroy, J., and Ménard, C. (2010). Measurements and modelling of snowmelt and turbulent heat fluxes over shrub tundra. Hydrol. Earth Syst. Sci. 14, 1331–1340. doi:10.5194/hess-14-1331-2010
Blöschl, G. (1999). Scaling issues in snow hydrology. Hydrol. Process 13, 2149–2175. doi:10.1002/(sici)1099-1085(199910)13:14/15<2149::aid-hyp847>3.0.co;2-8
Boone, A., and Etchevers, P. (2001). An intercomparison of three snow schemes of varying complexity coupled to the same land surface model: Local-scale evaluation at an Alpine site. J. Hydrometeorol. 2 (4), 374–394. doi:10.1175/1525-7541(2001)002<0374:aiotss>2.0.co;2
Boone, A., Samuelsson, P., Gollvik, S., Napoly, A., Jarlan, L., Brun, E., et al. (2017). The interactions between soil–biosphere–atmosphere land surface model with a multi-energy balance (ISBA-MEB) option in SURFEXv8 – Part 1: Model description. Model. Dev. 10, 843–872. doi:10.5194/gmd-10-843-2017
Brauchli, T., Trujillo, E., Huwald, H., and Lehning, M. (2017). Influence of slope-scale snowmelt on catchment response simulated with the Alpine3D model. Water Resour. Res. 53, 10723–10739. doi:10.1002/2017WR021278
Buizza, R. (2008). The value of probabilistic prediction. Atmosph. Sci. Lett. 9, 36–42. doi:10.1002/asl.170
Carletti, F., Michel, A., Casale, F., Burri, A., Bocchiola, D., Bavay, M., et al. (2022). A comparison of hydrological models with different level of complexity in Alpine regions in the context of climate change. Hydrology Earth Syst. Sci. 26 (13), 3447–3475. doi:10.5194/hess-26-3447-2022
Clark, M. P., Hendrikx, J., Slater, A. G., Kavetski, D., Anderson, B., Cullen, N. J., et al. (2011). Representing spatial variability of snow water equivalent in hydrologic and land-surface models: A review. Water Res. Res. 47, W07539. doi:10.1029/2011WR010745
Cluzet, B., Lafaysse, M., Deschamps-Berger, C., Vernay, M., and Dumont, M. (2022). Propagating information from snow observations with CrocO ensemble data assimilation system: A 10-years case study over a snow depth observation network. Cryosphere 16, 1281–1298. doi:10.5194/tc-16-1281-2022
Comola, F., Giometto, M. G., Salesky, S. T., Parlange, M. B., and Lehning, M. (2019). Preferential deposition of snow and dust over hills: Governing processes and relevant scales. J. Geophys. Res. Atmos. 124, 7951–7974. doi:10.1029/2018JD029614
Dadic, R., Mott, R., Lehning, M., Carenzo, M., Anderson, B., and Mackintosh, A. (2013). Sensitivity of turbulent fluxes to wind speed over snow surfaces in different climatic settings. Adv. Water Resour. 55, 178–189. doi:10.1016/j.advwatres.2012.06.010
DeBeer, C. M., and Pomeroy, J. W. (2017). Influence of snowpack and melt energy heterogeneity on snow cover depletion and snowmelt runoff simulation in a cold mountain environment. J. Hydrol. 553, 199–213. doi:10.1016/j.jhydrol.2017.07.051
Dierauer, J. R., Whitfield, P. H., and Allen, D. M. (2018). Climate controls on runoff and low flows in mountain catchments of Western North America. Water Resour. Res. 54 (10), 7495–7510. doi:10.1029/2018WR023087
Dornes, P. F., Pomeroy, J. W., Pietroniro, A., Carey, S. K., and Quinton, W. L. (2008a). Influence of landscape aggregation in modelling snow-cover ablation and snowmelt runoff in a sub-arctic mountainous environment. Hydrol. Sci. J. 53, 725–740. doi:10.1623/hysj.53.4.725
Dornes, P. F., Pomeroy, J. W., Pietroniro, A., and Verseghy, D. L. (2008b). Effects of spatial aggregation of initial conditions and forcing data on modeling snowmelt using a land surface scheme. J. Hydrometeorol. 9, 789–803. doi:10.1175/2007JHM958.1
Douville, H., Royer, J. F., and Mahfouf, J. F. (1995). A new snow parameterization for the Meteo-France climate model. Clim. Dyn. 12 (1), 21–35. doi:10.1007/bf00208760
Dozier, J., Bair, E. H., and Davis, R. E. (2016). Estimating the spatial distribution of snow water equivalent in the world’s mountains. WIREs Water 3, 461–474. doi:10.1002/wat2.1140
Dujardin, J., and Lehning, M. (2022). Wind-Topo: Downscaling near-surface wind fields to high-resolution topography in highly complex terrain with deep learning. Q.J.R. Meteorol. Soc. 148, 1368–1388. doi:10.1002/qj.4265
Dumont, M., Sirguey, P., Arnaud, Y., and Six, D. (2011). Monitoring spatial and temporal variations of surface albedo on Saint Sorlin Glacier (French Alps) using terrestrial photography. Cryosphere 5, 759–771. doi:10.5194/tc-5-759-2011
Dumont, M., Tuzet, F., Gascoin, S., Picard, G., Kutuzov, S., Lafaysse, M., et al. (2020). Accelerated snow melt in the Russian Caucasus mountains after the Saharan dust outbreak in March 2018. J. Geophys. Res. Earth Surf. 125, e2020JF005641. doi:10.1029/2020JF005641
Durand, Y., Guyomarc'h, G., Mérindol, L., and Corripio, J. G. (2005). Improvement of a numerical snow drift model and field validation. Cold regions Sci. Technol. 43 (1-2), 93–103. doi:10.1016/j.coldregions.2005.05.008
Dyer, J. L., and Mote, T. L. (2002). Role of energy budget components on snow ablation from a midlatitude prairies nowpack. Polar Geogr. 26 (2), 87–115. doi:10.1080/789610133
Essery, R., Li, L., and Pomeroy, J. (1999). A distributed model of blowing snow over complex terrain. Hydrol. Process. 13 (14-15), 2423–2438. doi:10.1002/(sici)1099-1085(199910)13:14/15<2423::aid-hyp853>3.0.co;2-u
Essery, R., Granger, R., and Pomeroy, J. W. (2006). Boundary-layer growth and advection of heat over snow and soil patches: Modelling and parameterization. Hydrol. Process. 20, 953–967. doi:10.1002/hyp.6122
Essery, R., Pomeroy, J., Ellis, C., and Link, T. (2008). Modelling longwave radiation to snow beneath forest canopies using hemispherical photography or linear regression. Hydrological Process. An Int. J. 22 (15), 2788–2800. doi:10.1002/hyp.6930
Essery, R., Rutter, N., Pomeroy, J., Baxter, R., Staehli, M., Gustafsson, D., et al. (2009). SNOWMIP2: An evaluation of forest snow process simulations. Bull. Am. Meteorological Soc. 90 (8), 1120–1136. doi:10.1175/2009BAMS2629.1
Essery, R., Morin, S., Lejeune, Y., and Ménard, C. B. (2013). A comparison of 1701 snow models using observations from an alpine site. Adv. water Resour. 55, 131–148. doi:10.1016/j.advwatres.2012.07.013
Essery, R. (2015). A factorial snowpack model (FSM 1.0). Model. Dev. 8, 3867–3876. doi:10.5194/gmd-8-3867-2015
Farinotti, D., Usselmann, S., Huss, M., Bauder, A., and Funk, M. (2012). Runoff evolution in the Swiss Alps: projections for selected high-alpine catchments based on ENSEMBLES scenarios. Hydrol. Process 26, 1909–1924. doi:10.1002/hyp.8276
Fiddes, J., and Gruber, S. (2014). TopoSCALE v. 1.0: Downscaling gridded climate data in complex terrain. Geosci. Model. Dev. 7 (1), 387–405. doi:10.5194/gmd-7-387-2014
Floriancic, M. G., Berghuijs, W. R., Jonas, T., Kirchner, J. W., and Molnar, P. (2020). Effects of climate anomalies on warm-season low flows in Switzerland. Earth Syst. Sci. 24, 5423–5438. doi:10.5194/hess-24-5423-2020
Frei, C. (2014). Interpolation of temperature in a mountainous region using non-linear profiles and non-Euclidean distances. Int. J. Climatol. 34, 1585–1605. doi:10.1002/joc.3786
Freudiger, D., Frielingsdorf, B., Stahl, K., Steinbrich, A., Weiler, M., Griessinger, N., et al. (2016). Das Potential meteorologischer Rasterdatensätze für die Modellierung der Schneedecke alpiner Einzugsgebiete. Hydrol. Wasserbewirtsch. 60 (6), 353–367. doi:10.5675/HyWa_2016,6_1
Freudiger, D., Kohn, I., Seibert, J., Stahl, K., and Weiler, M. (2017). Snow redistribution for the hydrological modeling of alpine catchments. WIREs Water 4, e1232. doi:10.1002/wat2.1232
Gallice, A., Bavay, M., Brauchli, T., Comola, F., Lehning, M., and Huwald, H. (2016). StreamFlow 1.0: An extension to the spatially distributed snow model Alpine3D for hydrological modelling and deterministic stream temperature prediction. Geosci. Model. Dev. 9, 4491–4519. doi:10.5194/gmd-9-4491-2016
Garvelmann, J., Pohl, S., and Weiler, M. (2014). Variability of observed energy fluxes during rain-on snow and clear sky snow melt in a midlatitude mountain environment. J. Hydrometeorol. 15 (3), 1220–1237. doi:10.1175/JHM-D-13-0187.1
Garvelmann, J., Pohl, S., and Weiler, M. (2015). Spatio-temporal controls of snowmelt and runoff generation during rain-on-snow events in a mid-latitude mountain catchment. Hydrol. Process. 29, 3649–3664. doi:10.1002/hyp.10460
Gerber, F., Mott, R., and Lehning, M. (2019). The importance of near-surface winter precipitation processes in complex alpine terrain. J. Hydrometeorol. 20 (2), 177–196. doi:10.1175/JHM-D-18-0055.1
Girons Lopez, M., Vis, M. J. P., Jenicek, M., Griessinger, N., and Seibert, J. (2020). Assessing the degree of detail of temperature-based snow routines for runoff modelling in mountainous areas in central Europe. Earth Syst. Sci. 24, 4441–4461. doi:10.5194/hess-24-4441-2020
Griessinger, N., Seibert, J., Magnusson, J., and Jonas, T. (2016). Assessing the benefit of snow data assimilation for runoff modeling in Alpine catchments. Hydrol. Earth Syst. Sci. 20, 3895–3905. doi:10.5194/hess-20-3895-2016
Griessinger, N., Schirmer, M., Helbig, N., Winstral, A., Michel, A., and Jonas, T. (2019). Implications of observation-enhanced energy-balance snowmelt simulations for runoff modeling of Alpine catchments. Adv. Water Resour. 133, 12, 103410. doi:10.1016/j.advwatres.2019.103410
Grünewald, T., and Lehning, M. (2015). Are flat-field snow depth measurements representative? A comparison of selected index sites with areal snow depth measurements at the small catchment scale. Hydrol. Process. 29, 1717–1728. doi:10.1002/hyp.10295
Harder, P., Pomeroy, J. W., and Helgason, W. (2017). Local-scale advection of sensible and latent heat during snowmelt. Geophys. Res. Lett. 44, 9769–9777. doi:10.1002/2017GL074394
Hardy, J. P., Melloh, R., Koenig, G., Marks, D., Winstral, A., Pomeroy, J. W., et al. (2004). Solar radiation transmission through conifer canopies. Agric. For. Meteorology 126 (3-4), 257–270. doi:10.1016/j.agrformet.2004.06.012
Haugeneder, M., Lehning, M., Reynolds, D., Jonas, T., and Mott, R. (2022). A novel method to quantify near-surface boundary-layer dynamics at ultra-high spatio–temporal resolution. Bound.-Lay.-Meteorol. 186, 177–197. doi:10.1007/s10546-022-00752-3
He, S., and Ohara, N. (2019). Modeling subgrid variability of snow depth using the Fokker-Planck equation approach. Water Resour. Res. 55 (4), 3137–3155. doi:10.1029/2017wr022017
Hedrick, A. R., Marks, D., Havens, S., Robertson, M., Johnson, M., Sandusky, M., et al. (2018). Direct insertion of NASA Airborne Snow Observatory-derived snow depth time series into the iSnobal energy balance snow model. Water Resour. Res. 54 (10), 8045–8063. doi:10.1029/2018wr023190
Hedstrom, N., and Pomeroy, J. W. (1998). Measurements and modelling of snow interception in the boreal forest. Hydrol. Process. 12, 1611–1625. doi:10.1002/(SICI)1099-1085(199808/09)12:10/11<1611::AID-HYP684>3.0.CO;2-4
Helbig, N., and Löwe, H. (2014). Parameterization of the spatially averaged sky view factor in complex topography. J. Geophys. Res. Atmos. 119 (8), 4616–4625. doi:10.1002/2013jd020892
Helbig, N., and van Herwijnen, A. (2017). Subgrid parameterization for snow depth over mountainous terrain from flat field snow depth. Water Resour. Res. 53 (2), 1444–1456. doi:10.1002/2016wr019872
Helbig, N., Löwe, H., Mayer, B., and Lehning, M. (2010). Explicit validation of a surface shortwave radiation balance model over snow-covered complex terrain. J. Geophys. Res. 115, D18113. doi:10.1029/2010JD013970
Helbig, N., van Herwijnen, A., Magnusson, J., and Jonas, T. (2015). Fractional snow-covered area parameterization over complex topography. Hydrol. Earth Syst. Sci. 19, 1339–1351. doi:10.5194/hess-19-1339-2015
Helbig, N., Mott, R., Van Herwijnen, A., Winstral, A., and Jonas, T. (2017). Parameterizing surface wind speed over complex topography. J. Geophys. Res. Atmos. 122 (2), 651–667. doi:10.1002/2016jd025593
Helbig, N., Moeser, D., Teich, M., Vincent, L., Lejeune, Y., Sicart, J.-E., et al. (2020). Snow processes in mountain forests: Interception modeling for coarse-scale applications. Earth Syst. Sci. 24, 2545–2560. doi:10.5194/hess-24-2545-2020
Helbig, N., Bühler, Y., Eberhard, L., Deschamps-Berger, C., Gascoin, S., Dumont, M., et al. (2021a). Fractional snow-covered area: Scale-independent peak of winter parameterization. Cryosphere 15, 615–632. doi:10.5194/tc-15-615-2021
Helbig, N., Schirmer, M., Magnusson, J., Mäder, F., van Herwijnen, A., Quéno, L., et al. (2021b). A seasonal algorithm of the snow-covered area fraction for mountainous terrain. Cryosphere 15, 4607–4624. doi:10.5194/tc-15-4607-2021
Hock, R., Hutchings, J. K., and Lehning, M. (2017). Grand challenges in cryospheric sciences: Toward better predictability of glaciers, snow and sea ice. Front. Earth Sci. 5, 64. doi:10.3389/feart.2017.00064
Hock, R. (1999). A distributed temperature-index ice- and snow melt model including potential direct solar radiation. J. Glaciol. 46, 101–111. doi:10.3189/s0022143000003087
Jenicek, M., Seibert, J., Zappa, M., Staudinger, M., and Jonas, T.(2016). Importance of maximum snow accumulation for summer low flows in humid catchments. Earth Syst. Sci. 20, 859–874. doi:10.5194/hess-20-859-2016
Jonas, T., Marty, C., and Magnusson, J. (2009). Estimating the snow water equivalent from snow depth measurements in the Swiss Alps. J. Hydrology 378 (1-2), 161–167. doi:10.1016/j.jhydrol.2009.09.021
Jonas, T., Webster, C., Mazzotti, G., and Malle, J. (2020). HPEval: A canopy shortwave radiation transmission model using high-resolution hemispherical images. Agric. For. Meteorology 284, 107903. doi:10.1016/j.agrformet.2020.107903
Jost, G., Moore, R. D., Smith, R., and Gluns, D. R. (2012). Distributed temperature-index snowmelt modelling for forested catchments. J. Hydrol. 420–421, 87–101. doi:10.1016/j.jhydrol.2011.11.045
Kim, R. S., Durand, M., Li, D., Baldo, E., Margulis, S. A., Dumont, M., et al. (2019). Estimating alpine snow depth by combining multifrequency passive radiance observations with ensemble snowpack modeling. Remote Sens. Environ. 226, 1–15. doi:10.1016/j.rse.2019.03.016
Kruyt, B., Mott, R., Fiddes, J., Gerber, F., Sharma, V., and Reynolds, D. (2022). A downscaling intercomparison study: The representation of slope- and ridgeridge-scale processes in models of different complexity. Front. Earth Sci. 10, 789332. doi:10.3389/feart.2022.789332
Lafaysse, M., Cluzet, B., Dumont, M., Lejeune, Y., Vionnet, V., and Morin, S. (2017). A multiphysical ensemble system of numerical snow modelling. Cryosphere 11, 1173–1198. doi:10.5194/tc-11-1173-2017
Lawrence, D. M., Fisher, R. A., Koven, C. D., Oleson, K. W., Swenson, S. C., Bonan, G., et al. (2019). The Community Land Model version 5: Description of new features, benchmarking, and impact of forcing uncertainty. J. Adv. Model. Earth Syst. 11, 4245–4287. doi:10.1029/2018MS001583
Lehning, M., Grünewald, T., and Schirmer, M. (2011). Mountain snow distribution governed by an altitudinal gradient and terrain roughness. Geophys. Res. Lett. 38, L19504. doi:10.1029/2011GL048927
Lehning, M., Löwe, H., Ryser, M., and Raderschall, N. (2008). Inhomogeneous precipitation distribution and snow transport in steep terrain. Water Resour. Res. 44, W07404. doi:10.1029/2007WR006545
Li, D., Lettenmaier, D. P., Margulis, S. A., and Andreadis, K. (2019). The role of rain-on-snow in flooding over the conterminous United States. Water Resour. Res. 55, 8492–8513. doi:10.1029/2019WR024950
Lievens, H., Demuzere, M., Marshall, H. P., Reichle, R. H., Brucker, L., Brangers, I., et al. (2019). Snow depth variability in the Northern Hemisphere mountains observed from space. Nat. Commun. 10, 4629. doi:10.1038/s41467-019-12566-y
Lievens, H., Brangers, I., Marshall, H.-P., Jonas, T., Olefs, M., and De Lannoy, G. (2022). Sentinel-1 snow depth retrieval at sub-kilometer resolution over the European alps. Cryosphere 16, 159–177. doi:10.5194/tc-16-159-2022
Liston, G. E. (2004). Representing subgrid snow cover heterogeneities in regional and global models. J. Clim. 17 (6), 1381–1397. doi:10.1175/1520-0442(2004)017<1381:rsschi>2.0.co;2
Liston, G. E., Haehnel, R. B., Sturm, M., Hiemstra, C. A., Berezovskaya, S., and Tabler, R. D. (2007). Simulating complex snow distributions in windy environments using SnowTran-3D. J. Glaciol. 53, 241–256. doi:10.3189/172756507782202865
Louis, J. F. (1979). A parametric model of vertical eddy fluxes in the atmosphere. Boundary-Layer Meteorol. 17 (2), 187–202. doi:10.1007/bf00117978
Löwe, H., and Helbig, N. (2012). Quasi-analytical treatment of spatially averaged radiation transfer in complex terrain: Averaged radiation in complex terrain. J. Geophys. Res. 17, D19101. doi:10.1029/2012JD018181
Lundquist, J., Hughes, M., Gutmann, E., and Kapnick, S. (2019). Our skill in modeling mountain rain and snow is bypassing the skill of our observational networks. Bull. Am. Meteorological Soc. 100 (12), 2473–2490. Retrieved Aug 31, 2022, from. doi:10.1175/bams-d-19-0001.1
Lüthi, S., Ban, N., Kotlarski, S., Steger, C. R., Jonas, T., and Schär, C. (2019). Projections of alpine snow-cover in a high-resolution climate simulation. Atmosphere 10 (8), 463. doi:10.3390/atmos10080463
Magnusson, J., Gustafsson, D., Husler, F., and Jonas, T. (2014). Assimilation of point SWE data into a distributed snow cover model comparing two contrasting methods. Water Resour. Res. 50, 7816–7835. doi:10.1002/2014WR015302
Magnusson, J., Wever, N., Essery, R., Helbig, N., Winstral, A., and Jonas, T. (2015). Evaluating snow models with varying process representations for hydrological applications. Water Resour. Res. 51, 2707–2723. doi:10.1002/2014WR016498
Magnusson, J., Winstral, A., Stordal, A. S., Essery, R., and Jonas, T. (2017). Improving physically based snow simulations by assimilating snow depths using the particle filter. Water Resour. Res. 53, 1125–1143. doi:10.1002/2016WR019092
Mahat, V., and Tarboton, D. G. (2014). Representation of canopy snow interception, unloading and melt in a parsimonious snowmelt model. Hydrol. Process. 28 (26), 6320–6336. doi:10.1002/hyp.10116
Mahat, V., Tarboton, D. G., and Molotch, N. P. (2013). Testing above- and below-canopy representations of turbulent fluxes in an energy balance snowmelt model. Water Resour. Res. 49, 1107–1122. doi:10.1002/wrcr.20073
Malle, J., Rutter, N., Mazzotti, G., and Jonas, T. (2019). Shading by trees and fractional snow cover control the subcanopy radiation budget. J. Geophys. Res. Atmos. 124, 3195–3207. doi:10.1029/2018JD029908
Marks, D., and Dozier, J. (1992). Climate and energy exchange at the snow surface in the alpine region of the sierra Nevada: 2. Snow cover energy balance. Water Resour. Res. 28 (11), 3043–3054. doi:10.1029/92wr01483
Marsh, C. B., Pomeroy, J. W., Spiteri, R. J., and Wheater, H. S. (2020). A finite volume blowing snow model for use with variable resolution meshes. Water Resour. Res. 56 (2), e2019WR025307. doi:10.1029/2019wr025307
Martin, E., and Lejeune, Y. (1998). Turbulent fluxes above the snow surface. Ann. Glaciol. 26, 179–183. doi:10.3189/1998aog26-1-179-183
Martinec, J., and Rango, A. (1991). “Indirect evaluation of snow reserves in mountain basins,” in Proceedings: Snow, Hydrology and Forest in High Alpine Areas. Editors H. Bergmann, H. Lang, W. Frey, D. Issler, and B. Salm, (Vienna: IAHS Publication) 205, 111–120.
Mazzotti, G., Essery, R., Moeser, C. D., and Jonas, T. (2020a). Resolving small-scale forest snow patterns using an energy balance snow model with a one-layer canopy. Water Resour. Res. 56 (1), e2019WR026129. doi:10.1029/2019WR026129
Mazzotti, G., Essery, R. L. H., Webster, C., Malle, J., and Jonas, T. (2020b). Process-level evaluation of a hyper-resolution forest snow model using distributed multi-sensor observations. Water Resour. Res. 56 (9), e2020WR027572. doi:10.1029/2020WR027572
Mazzotti, G., Webster, C., Essery, R., and Jonas, T. (2021). Increasing the physical representation of forest-snow processes in coarse-resolution models: Lessons learned from upscaling hyper-resolution simulations. Water Resour. Res. 57 (5), e2020WR029064. doi:10.1029/2020WR029064
Mazzotti, G., Webster, C., Quéno, L., Cluzet, B., and Jonas, T. (2023). Canopy structure, topography and weather are equally important drivers of small-scale snow cover dynamics in sub-alpine forests. Hydrol. Earth Syst. Sci. Discuss., 2099–2121 [preprint] in press. 10.5194/hess-27-2099-2023, 2023
MeteoSwiss, 2019: MeteoSwiss. Available at: https://www.meteoswiss.admin.ch/content/dam/meteoswiss/de/service-und-publikationen/produkt/raeumliche-daten-niederschlag/doc/ProdDoc_RhiresD.pdf (Accessed: 27 August 2022), 2019.
MeteoSwiss (2020). MeteoSwiss. Available at: https://www.meteoschweiz.admin.ch/wetter/warn-und-prognosesysteme/nowcasting.html.
Moeser, D., Morsdorf, F., and Jonas, T. (2015). Novel forest structure metrics from airborne LiDAR data for improved snow interception estimation. Agric. For. Meteorology 208, 40–49. doi:10.1016/j.agrformet.2015.04.013
Morán-Tejeda, E., López-Moreno, J. I., Stoffel, M., and Beniston, M. (2016). Rain-on-snow events in Switzerland: Recent observations and projections for the 21st century. Clim. Res. 71 (2), 111–125. doi:10.3354/cr01435
Morin, S., Horton, S., Techel, F., Bavay, M., Coléou, C., Fierz, C., et al. (2020). Application of physical snowpack models in support of operational avalanche hazard forecasting: A status report on current implementations and prospects for the future. Cold Reg. Sci. Tech. 170, 102910. doi:10.1016/j.coldregions.2019.102910
Mott, R., and Lehning, M. (2010). Meteorological modeling of very high resolution wind fields and snow deposition for mountains. J. Hydromet. 11, 934–949. doi:10.1175/2010JHM1216.1
Mott, R., Paterna, E., Horender, S., Crivelli, P., and Lehning, M. (2016). Wind tunnel experiments: Cold-air pooling and atmospheric decoupling above a melting snow patch. Cryosphere 10, 445–458. doi:10.5194/tc-10-445-2016
Mott, R., Schlögl, S., Dirks, L., and Lehning, M. (2017). Impact of extreme land surface heterogeneity on micrometeorology over spring snow cover. J. Hydrometeor. 18, 2705–2722. doi:10.1175/JHM-D-17-0074.1
Mott, R., Wolf, A., Kehl, M., Kunstmann, H., Warscher, M., and Grünewald, T. (2019). Avalanches and micrometeorology driving mass and energy balance of the lowest perennial ice field of the alps: A case study. Cryosphere 13 (4), 1247–1265. doi:10.5194/tc-13-1247-2019
Mott, R., Stiperski, I., and Nicholson, L. (2020). Spatio-temporal flow variations driving heat exchange processes at a mountain glacier. Cryosphere 14, 4699–4718. doi:10.5194/tc-14-4699-2020
Musselman, K. N., and Pomeroy, J. W. (2017). Estimation of needleleaf canopy and trunk temperatures and longwave contribution to melting snow. J. Hydrometeorol. 18 (2), 555–572. doi:10.1175/jhm-d-16-0111.1
Musselman, K. N., Pomeroy, J. W., Essery, R. L., and Leroux, N. (2015). Impact of windflow calculations on simulations of alpine snow accumulation, redistribution and ablation. Hydrol. Process. 29 (18), 3983–3999. doi:10.1002/hyp.10595
Musselman, K. N., Lehner, F., Ikeda, K., Clark, M. P., Prein, A. F., Liu, C., et al. (2018). Projected increases and shifts in rain-on-snow flood risk over Western North America. Nat. Clim. Change 8 (9), 808–812. doi:10.1038/s41558-018-0236-4
Niu, G.-Y., Yang, Z. L., Mitchell, K. E., Chen, F., Ek, M. B., Barlage, M., et al. (2011). The community Noah land surface model with multiparameterization options (Noah-MP): 1. Model description and evaluation with local-scale measurements. J. Geophys. Res. 116, D12109. doi:10.1029/2010JD015139
Odry, J., Boucher, M.-A., Lachance-Cloutier, S., Turcotte, R., and St-Louis, P.-Y. (2022). Large-scale snow data assimilation using a spatialized particle filter: Recovering the spatial structure of the particles. Cryosphere 16, 3489–3506. doi:10.5194/tc-16-3489-2022
Painter, T. H., Berisford, D. F., Boardman, J. W., Bormann, K. J., Deems, J. S., Gehrke, F., et al. (2016). The Airborne Snow Observatory: Fusion of scanning lidar, imaging spectrometer, and physically-based modeling for mapping snow water equivalent and snow albedo. Remote Sens. Environ. 184, 139–152. doi:10.1016/j.rse.2016.06.018
Parajuli, A., Nadeau, D. F., Anctil, F., Schilling, O. S., and Jutras, S. (2020). Does data availability constrain temperature-index snow models? A case study in a humid boreal forest. Water 12, 2284. doi:10.3390/w12082284
Pohl, S., Marsh, P., and Liston, G. E. (2006). Spatial-temporal variability in turbulent fluxes during spring snowmelt. Arct. Antarct. Alp. Res. 38, 136–146. doi:10.1657/1523-0430(2006)038[0136:SVITFD]2.0.CO;2
Pomeroy, J., Parviainen, J., Hedstrom, N., and Gray, D. (1998). Coupled modelling of forest snow interception and sublimation. Hydrol. Process. 12 (15), 2317–2337. doi:10.1002/(SICI)1099-1085(199812)12:15<2317::AID-HYP799>3.0.CO;2-X
Pomeroy, J. W., Gray, D. M., Brown, T., Hedstrom, N. R., Quinton, W. L., Granger, R. J., et al. (2007). The cold regions hydrological model: A platform for basing process representation and model structure on physical evidence. Hydrological Process. An Int. J. 21 (19), 2650–2667. doi:10.1002/hyp.6787
Pomeroy, J., Marks, D., Link, T., Ellis, C., Hardy, J., Rowlands, A., et al. (2009). The impact of coniferous forest temperature on incoming longwave radiation to melting snow. Hydrol. Process. 23 (17), 2513–2525. doi:10.1002/hyp.7325
Raleigh, M. S., Lundquist, J. D., and Clark, M. P. (2015). Exploring the impact of forcing error characteristics on physically based snow simulations within a global sensitivity analysis framework. Hydrology Earth Syst. Sci. 19 (7), 3153–3179. doi:10.5194/hess-19-3153-2015
Ramos, M. H., van Andel, S. J., and Pappenberger, F. (2013). Do probabilistic forecasts lead to better decisions? Hydrol. Earth Syst. Sci. 17, 2219–2232. doi:10.5194/hess-17-2219-2013
Reynolds, D., Pflug, J. M., and Lundquist, J. D. (2020). Evaluating wind fields for use in basin-scale distributed snow models. Water Resour. Res. 57, e2020WR028536. doi:10.1029/2020WR028536
Reynolds, D. S., Gutmann, E., Kruyt, B., Haugeneder, M., Jonas, T., Gerber, F., et al. (2023). The high-resolution intermediate complexity atmospheric research (HICAR v1.0) model enables fast dynamic downscaling to the hectometer scale. Geosci. Model. Dev. Discuss. [preprint] in review, 2023. doi:10.5194/gmd-2023-16
Roth, T. R., and Nolin, A. W. (2017). Forest impacts on snow accumulation and ablation across an elevation gradient in a temperate montane environment. Hydrology Earth Syst. Sci. 21 (11), 5427–5442. doi:10.5194/hess-21-5427-2017
Roth, T. R., and Nolin, A. W. (2019). Characterizing maritime snow canopy interception in forested mountains. Water Resour. Res. 55 (6), 4564–4581. doi:10.1029/2018WR024089
Rutter, N., Essery, R., Pomeroy, J., Altimir, N., Andreadis, K., Baker, I., et al. (2009). Evaluation of forest snow processes models (SnowMIP2). J. Geophys. Res. 114, D06111. doi:10.1029/2008JD011063
Sauter, T., and Galos, S. P. (2016). Effects of local advection on the spatial sensible heat flux variation on a mountain glacier. Cryosphere 10, 2887–2905. doi:10.5194/tc-10-2887-2016
Sauter, T., Möller, M., Finkelnburg, R., Grabiec, M., Scherer, D., and Schneider, C. (2013). Snowdrift modelling for the Vestfonna ice cap, north-eastern Svalbard. Cryosphere 7 (4), 1287–1301. doi:10.5194/tc-7-1287-2013
Schano, C., Niffenegger, C., Jonas, T., and Korner-Nievergelt, F. (2021). Hatching phenology is lagging behind an advancing snowmelt pattern in a high-alpine bird. Sci. Rep. 11, 22191. doi:10.1038/s41598-021-01497-8
Schirmer, M., and Pomeroy, J. W. (2020). Processes governing snow ablation in alpine terrain – detailed measurements from the Canadian Rockies. Earth Syst. Sci. 24, 143–157. doi:10.5194/hess-24-143-2020
Schirmer, M., Winstral, A., Jonas, T., Burlando, P., and Peleg, N. (2022). Natural climate variability is an important aspect of future projections of snow water resources and rain-on-snow events. Cryosphere 16, 3469–3488. doi:10.5194/tc-16-3469-2022
Schlögl, S., Marty, C., Bavay, M., and Lehning, M. (2016). Sensitivity of Alpine3D modeled snow cover to modifications in DEM resolution, station coverage and meteorological input quantities. Environ. Model. Softw. 83, 387–396. doi:10.1016/j.envsoft.2016.02.017
Schneiderbauer, S., and Prokop, A. (2011). The atmospheric snow-transport model: SnowDrift3D. J. Glaciol. 57 (203), 526–542. doi:10.3189/002214311796905677
Shakoor, A., Burri, A., Bavay, M., Ejaz, N., Ghumman, A. R., Comola, F., et al. (2018). Hydrological response of two high altitude Swiss catchments to energy balance and temperature index melt schemes. Polar Sci. 17, 1–12. doi:10.1016/j.polar.2018.06.007
Shea, J., and Moore, R. (2010). Prediction of spatially distributed regional-scale fields of air temperature and vapor pressure over mountain glaciers. J. Geophys. Res. 113, D23107. doi:10.1029/2010JD014351
Sicart, J. E., Pomeroy, J. W., Essery, R. L. H., and Bewley, D. (2006). Incoming longwave radiation to melting snow: Observations, sensitivity and estimation in northern environments. Hydrol. Process. 20 (17), 3697–3708. doi:10.1002/hyp.6383
Surfleet, C. G., and Tullos, D. (2013). Variability in effect of climate change on rain-on-snow peak flow events in a temperate climate. J. Hydrology 479, 24–34. doi:10.1016/j.jhydrol.2012.11.021
Tobin, C., Schaefli, B., Nicótina, L., Simoni, S., Barrenetxea, G., Smith, R., et al. (2013). Improving the degree-day method for sub-daily melt simulations with physically-based diurnal variations. Adv. Water Resour. 55, 149–164. doi:10.1016/j.advwatres.2012.08.008
Toreti, A., Bavera, D., Acosta Navarro, J., Arias-Muñoz, C., Avanzi, F., Marinho Ferreira Barbosa, P., et al. (2023). Drought in europe march 2023, EUR 31448 EN. Luxembourg: Publications Office of the European Union. ISBN 978-92-68-01068-6 10.2760/998985, JRC133025.
Vernay, M., Lafaysse, M., Mérindol, L., Giraud, G., and Morin, S. (2015). Ensemble forecasting of snowpack conditions and avalanche hazard. Cold Reg. Sci. Technol. 120, 251–262. doi:10.1016/j.coldregions.2015.04.010
Vionnet, V., Martin, E., Masson, V., Lac, C., Durand, Y., Naaim-Bouvet, F., et al. (2012). Simulation of wind-driven snow redistribution at a high-elevation alpine site with a mesoscale atmospheric model.
Vionnet, V., Martin, E., Masson, V., Guyomarc'h, G., Naaim-Bouvet, F., Prokop, A., et al. (2014). Simulation of wind-induced snow transport and sublimation in alpine terrain using a fully coupled snowpack/atmosphere model. Cryosphere 8 (2), 395–415. doi:10.5194/tc-8-395-2014
Vionnet, V., Martin, E., Masson, V., Lac, C., Naaim Bouvet, F., and Guyomarc'h, G. (2017). High-resolution large eddy simulation of snow accumulation in Alpine Terrain. J. Geophys. Res. Atmos. 122, 11005–11021. doi:10.1002/2017JD026947
Vionnet, V., Six, D., Auger, L., Dumont, M., Lafaysse, M., Quéno, L., et al. (2019). Sub-kilometer precipitation datasets for snowpack and glacier modeling in alpine terrain. Front. Earth Sci. 7, 182. doi:10.3389/feart.2019.00182
Vionnet, V., Marsh, C. B., Menounos, B., Gascoin, S., Wayand, N. E., Shea, J., et al. (2021). Multi-scale snowdrift-permitting modelling of mountain snowpack. Cryosphere 15 (2), 743–769. doi:10.5194/tc-15-743-2021
Vionnet, V., Verville, M., Fortin, V., Brugman, M., Abrahamowicz, M., Lemay, F., et al. (2022). Snow level from post-processing of atmospheric model improves snowfall estimate and snowpack prediction in mountains. Water Resour. Res. 58, e2021WR031778. doi:10.1029/2021WR031778
Viviroli, D., Dürr, H. H., Messerli, B., Meybeck, M., and Weingartner, R. (2007). Mountains of the world, water towers for humanity: Typology, mapping, and global significance. Water Resour. Res. 43 (7). doi:10.1029/2006wr005653
Vögeli, C., Lehning, M., Wever, N., and Bavay, M. (2016). Scaling precipitation input to spatially distributed hydrological models by measured snow distribution. Front. Earth Sci. 4, 108. doi:10.3389/feart.2016.00108
Warscher, M., Strasser, U., Kraller, G., Marke, T., Franz, H., and Kunstmann, H. (2013). Performance of complex snow cover descriptions in a distributed hydrological model system: A case study for the high alpine terrain of the berchtesgaden alps. Water Resour. Res. 49 (5), 2619–2637. doi:10.1002/wrcr.20219
Waser, L. T., Fischer, C., Wang, Z., and Ginzler, C. (2015). Wall-to-wall forest mapping based on digital surface models from image-based point clouds and a NFI forest definition. Forests 6 (12), 4510–4528. doi:10.3390/f6124386
Waser, L. T., Ginzler, C., and Rehush, N. (2017). Wall-to-wall tree type mapping from countrywide airborne remote sensing surveys. Remote Sens. 9 (8), 766. doi:10.3390/rs9080766
Webster, C., Rutter, N., and Jonas, T. (2017). Improving representation of canopy temperatures for modeling subcanopy incoming longwave radiation to the snow surface. J. Geophys. Res. Atmos. 122 (17), 9154–9172. doi:10.1002/2017JD026581
Webster, C., Mazzotti, G., Essery, R. L. H., and Jonas, T. (2020). Enhancing airborne LiDAR data for improved forest structure representation in shortwave transmission models. Remote Sens. Environ. 249, 112017. doi:10.1016/j.rse.2020.112017
Webster, C., Essery, R., Mazzotti, G., and Jonas, T. (2023). Using just a canopy height model to obtain lidar-level accuracy in 3D forest canopy shortwave transmissivity estimates. Agric. For. Meteorology 338, 109429. doi:10.1016/j.agrformet.2023.109429
Winstral, A., and Marks, D. (2002). Simulating wind fields and snow redistribution using terrain-based parameters to model snow accumulation and melt over a semi-arid mountain catchment. Hydrol. Process. 16 (18), 3585–3603. doi:10.1002/hyp.1238
Winstral, A., and Marks, D. (2014). Long-term snow distribution observations in a mountain catchment: Assessing variability, time stability, and the representativeness of an index site. Water Resour. Res. 50, 293–305. doi:10.1002/2012WR013038
Winstral, A., Marks, D., and Gurney, R. (2009). An efficient method for distributing wind speeds over heterogeneous terrain. Hydrological Process. An Int. J. 23 (17), 2526–2535. doi:10.1002/hyp.7141
Winstral, A., Marks, D., and Gurney, R. (2013). Simulating wind-affected snow accumulations at catchment to basin scales. Adv. Water Resour. 55, 64–79. doi:10.1016/j.advwatres.2012.08.011
Winstral, A., Jonas, T., and Helbig, N. (2017). Statistical downscaling of gridded wind speed data using local topography. J. Hydrometeorol. 18 (2), 335–348. doi:10.1175/jhm-d-16-0054.1
Winstral, A., Magnusson, J., Schirmer, M., and Jonas, T. (2019). The bias-detecting ensemble: A new and efficient technique for dynamically incorporating observations into physics-based, multilayer snow models. Water Resour. Res. 55, 613–631. doi:10.1029/2018WR024521
Würzer, S., and Jonas, T. (2018). Spatio-temporal aspects of snowpack runoff formation during rain on snow. Hydrol. Process. 32, 3434–3445. doi:10.1002/hyp.13240
Keywords: snow hydrology, snow processes, forest snow processes, snow-atmosphere interactions, meteorological downscaling, snow model, data assimilation
Citation: Mott R, Winstral A, Cluzet B, Helbig N, Magnusson J, Mazzotti G, Quéno L, Schirmer M, Webster C and Jonas T (2023) Operational snow-hydrological modeling for Switzerland. Front. Earth Sci. 11:1228158. doi: 10.3389/feart.2023.1228158
Received: 24 May 2023; Accepted: 04 July 2023;
Published: 25 July 2023.
Edited by:
Alun Hubbard, University of Oulu, FinlandReviewed by:
Juraj Parajka, Vienna University of Technology, AustriaRensheng Chen, Chinese Academy of Sciences (CAS), China
Copyright © 2023 Mott, Winstral, Cluzet, Helbig, Magnusson, Mazzotti, Quéno, Schirmer, Webster and Jonas. This is an open-access article distributed under the terms of the Creative Commons Attribution License (CC BY). The use, distribution or reproduction in other forums is permitted, provided the original author(s) and the copyright owner(s) are credited and that the original publication in this journal is cited, in accordance with accepted academic practice. No use, distribution or reproduction is permitted which does not comply with these terms.
*Correspondence: Rebecca Mott, mott@slf.ch