- 1School of Architecture and Urban Planning, Huazhong University of Science and Technology, Wuhan, China
- 2Hubei Engineering and Technology Research Center of Urbanization, Wuhan, China
Abstract
Global climate change and the process of urbanization have had a significant impact on land surface temperature (LST). This study selects the Chengdu metropolitan area in China as a typical research subject. Based on the seasonal heterogeneity and spatial distribution characteristics of LST, different types of potential influencing factors are selected for Principal Component Analysis (PCA) to determine the categories of these factors. Subsequently, a multiple linear regression analysis is conducted to explore the relationship between LST and the identified potential influencing factors during different seasons. The findings of this study suggest that the regions with high temperatures and secondary high temperatures in the Chengdu metropolitan area are primarily concentrated in Chengdu and its adjacent localities, exhibiting noticeable seasonal variations. In the summer, high-temperature zone and second high-temperature zone of the LST show a central aggregation pattern. In the transition season, the high-temperature zone of the LST presents a “large dispersion, small aggregation” pattern. In the winter, it presents a dispersed pattern. In terms of influencing factors, elevation, slope, wind speed, humidity, and surface vegetation cover related to natural geographical conditions have a significant impact on LST, reaching a peak during the transition season. Factors associated with social and economic conditions, such as population size, nighttime light index, and road density, have a pronounced effect on LST during the summer season. During winter, LST is mainly influenced by landscape pattern-related factors such as Shannon Diversity Index, Edge Density, Largest Patch Index, and Patch Density. This study not only assesses the seasonal and spatial characteristics of LST in the Chengdu metropolitan area but also provides valuable insights for formulating phased measures to mitigate the Urban Heat Island (UHI) in other regions.
1 Introduction
With the ongoing global climate change and urbanization process, the continuous development of cities worldwide and the concentration of population in urban areas have had a significant impact on the urban ecological environment. The advancing urbanization exerts a strong force on climate change in cities and their surrounding areas. Among these forces, the urban heat island (UHI) effect plays a crucial role. (Grimm et al., 2008). This phenomenon is identified by a considerable rise in air and land surface temperature (LST) within urban regions, contrasting with the adjacent rural surroundings (Thompson and Perry, 1997). The Surface Urban Heat Island (SUHI) effect can be identified using conventional thermal-infrared remote sensing techniques, which can effectively interpret the land-surface energy flow characteristics in terms of numerical values. The SUHI effect exhibits pronounced spatial and temporal variations when compared to the UHI effect described by air temperature. It is also more susceptible to variations in land surface characteristics and human activity. Furthermore, the rise in LST gives rise to an upward movement of air currents, which can provide better insight into the underlying causes of UHI than air temperature-based evaluations (Wang Z. et al., 2022; Hu and Li, 2022). SUHI effect exerts a significant force on human production and living. In recent years, it has drawn attention from various disciplines such as geography, ecology, meteorology, and urban planning. Many scholars have begun to explore the characteristics of LST changes and the mechanisms behind the formation of SUHI(Zakšek and Oštir, 2012). They have been investigating different approaches to mitigate the rise in surface temperature and, consequently, alleviate the impact of UHI on human production and living. Therefore, it is of vital importance to scientifically investigate the spatial and temporal distribution of UHI and its influencing factors to understand the functioning of the urban heat island effect and find ways to mitigate its impact.
Currently, the conventional approach of identifying LST through weather stations has no longer sufficient to meet practical needs. Multivariate remote sensing data, such as Landsat Thematic Mapper, Advanced Very High-Resolution Radiometer (AVHRR) satellite data, Moderate Resolution Imaging Spectroradiometer (Modis), and Advanced Spaceborne Thermal Emission and Radiation (Aster), have revolutionized the field of LST detection. Compared to traditional methods of measuring LST, remote sensing technology has proven to be more reliable and efficient in capturing the complex and dynamic nature of LST variations over time and space (Imhoff et al., 2010). In this study, MODIS11A2 data is utilized, which is captured by long-term surface satellites and processed through techniques such as stitching, projection conversion, and other image processing. This method offers a more accurate and extended detection time range of LST data (Wan, 2008).
Merely examining the spatial distribution of LST might not be adequate in effectively mitigating UHI hazards; it is crucial to investigate the probable factors behind LST changes. Numerous statistical techniques have been utilized for examining the impacts of LST including geographically-weighted regression models to explore the connection between LST variation and its driving forces (Gao et al., 2022), geographic detectors (Geo-detectors) for identifying the impact of surface parameters on LST (Wang W. et al., 2021), and spatial regression models to examine the influence of urban spatial structure on UHI at the community level (Guo A. et al., 2020). The above study explores the spatial characteristics and influencing factors of urban heat islands from different perspectives, providing valuable suggestions for mitigating the urban heat island effect. However, there are several limitations. Firstly, from a temporal perspective, many studies focus on the surface temperature during the summer season, overlooking the seasonal variations and making it difficult to assess the phased characteristics of surface temperature. Secondly, from a spatial perspective, numerous studies concentrate on individual cities or large urban clusters, neglecting the closer connections within urban agglomerations that encompass economic, social, and natural aspects. Furthermore, many studies have focused on the impacts of natural geographical features and human socio-economic activities on urban heat islands (Ward et al., 2016). In recent years, the influence of landscape patterns on urban heat islands has also been gradually addressed. Several studies have indicated a relationship between urban green spaces and urban heat islands (Li et al., 2013). Common landscape indicators are often used to explore the connection between urban thermal environments and landscape pattern factors (Peng et al., 2016; Sun et al., 2022). However, many of these studies overlook the interactions between multiple influencing factors and the seasonal variations in these factors. To address this gap, our research will consider multiple types of influencing factors, emphasizing the elimination of interactions among these factors. This approach allows for a more intuitive identification of the dominant factors influencing urban heat islands at different stages. Additionally, considering the seasonal variability of the driving factors for surface temperature, we will propose tailored mitigation measures. Nevertheless, there are still limitations in the current research regarding this aspect.
Since China’s reform and opening-up, cities have developed rapidly. The continuous advancement of urbanization has put forward new requirements for the construction and development of Chinese cities. Urban agglomerations and metropolitan areas have become important models for the development of Chinese cities. Nevertheless, metropolitan areas exhibit more proximate material and spatial linkages compared to urban agglomerations. Furthermore, they play a crucial role in the evolvement of urban agglomerations (Fang, 2021; Wang Q. et al., 2022). In 2021, the Chinese government released the Chengdu Metropolitan Area Development Plan, designating the Chengdu metropolitan area as a significant growth center in southwest China. However, due to its location in the Sichuan Basin, the area faces challenges in heat dissipation and is prone to forming a heat island. In recent years, the UHI effect in Chengdu has had a stronger influence on people’s production and life, and at the same time, it has posed challenges to the sustainable development of the city (Guo J. et al., 2020; Wu et al., 2021). Currently, there are multiple perspectives in the research on surface temperature in the Chengdu region to explore the urban heat island phenomenon and the driving factors influencing it. These perspectives include examining the relationship between urban land changes and the urban heat island effect (Zhigang et al., 2016; Yu et al., 2022; Zhe et al., 2022), investigating the relationship between the built environment in the Chengdu region and surface temperature (Sun et al., 2022; Luo et al., 2023), exploring the impact of changes in health indices of urban and rural residents in China on surface temperature (Ren et al., 2021), studying the influence of landscape patterns on the intensity of the urban heat island (Sun et al., 2022), and using remote sensing satellite products to investigate the impact of meteorological factors on the intensity of the urban heat island (Lai et al., 2018; Liao et al., 2022). However, the current research on the Chengdu region mostly focuses on the urban heat island characteristics within the city itself, while there is relatively little research on the urban heat island effect in the Chengdu metropolitan area, lacking an exploration of the characteristics of the urban heat island effect within the metropolitan area that spans administrative boundaries and has close interconnections among its elements.
Therefore, this study focuses on the Chengdu metropolitan area, aiming to fill the research gap on urban heat islands in the Chengdu metropolitan area and provide recommendations for mitigating the urban heat island effect. In summary, although there are many studies focusing on exploring the characteristics and influencing factors of the urban heat island effect, this study has several key innovations compared to previous research. Firstly, in terms of the choice of research area, this study takes the perspective of the metropolitan area and selects the Chengdu metropolitan area as the research object. As the first approved metropolitan area in Southwest China and an important growth pole in the region, there is relatively limited research on the Chengdu metropolitan area. Therefore, this study focuses on the Chengdu metropolitan area, which has important theoretical value for mitigating the urban heat island effect at the metropolitan scale. Secondly, in terms of research methods, this study examines the characteristics of the urban heat island in different seasons, conducts correlation analysis to explore potential driving factors influencing surface temperature in the Chengdu metropolitan area, and finally utilizes principal component analysis and multiple linear regression to investigate the relationships among the driving factors, thereby identifying the main factors influencing surface temperature in different seasons in the Chengdu metropolitan area. Thirdly, from a theoretical and practical perspective, this study identifies the dominant factors influencing surface temperature in different seasons and proposes seasonal mitigation measures, providing reference and guidance for local governments and urban planners to a certain extent. This study is of theoretical significance and practical relevance to the construction and development of the Chengdu metropolitan area.
2 Research region
The location of the Chengdu metropolitan area lies in the northwestern vicinity of the Sichuan Basin, specifically positioned within the southwestern boundaries of China’s Sichuan Province (30°04′-31°42′N; 103°50′-105°27′E). The Chengdu metropolitan area, with a total expanse of 33,100 square kilometers, encompasses various counties and urban zones, including the Jingyang District of Deyang City, Shifang City, and Guanghan City, and is primarily centered around Chengdu City. The topography of the region is predominantly flat, with ranges of mountains situated towards the west. The bulk of the metropolitan zone is located within the central subtropical belt (Liu et al., 2021a), and its unique geographic location results in an average temperature of 16.8°C in Chengdu City. The planning area is selected as the study area (Figure 1) to provide a relevant reference for the planning and construction of Chengdu metropolitan area.
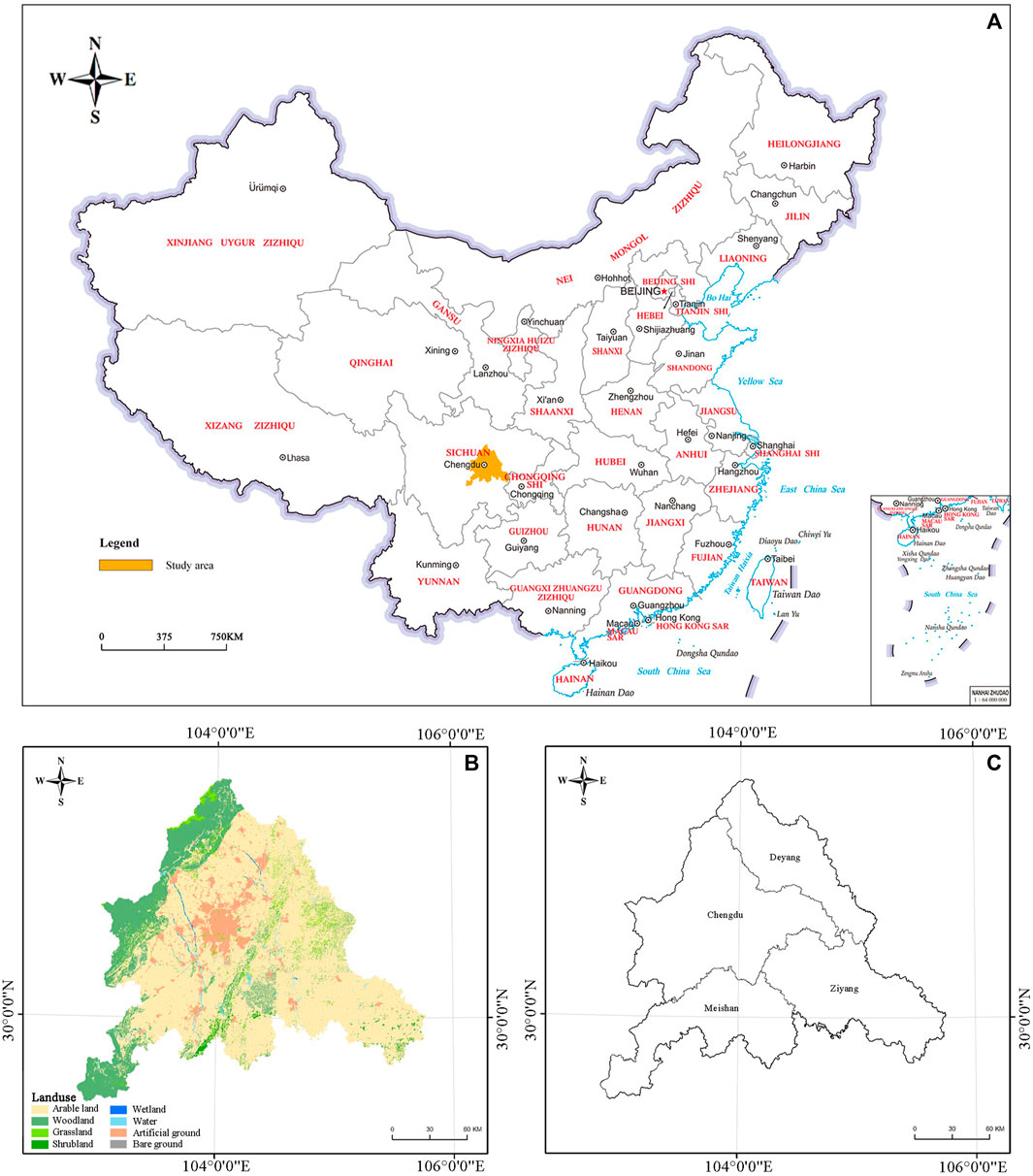
FIGURE 1. (A) Extent of the research region (B) Research region land use (C) Administrative division of the research region.
This study is based on the climatic characteristics of the Chengdu metropolitan area and previous research findings (Wang Z. et al., 2022). We also take into account the geographical environment of the Chengdu metropolitan area. On one hand, we analyze the temperature and precipitation data for each month. On the other hand, we consider the continuity of human society’s seasonal division, resulting in the categorization of the Chengdu metropolitan area into three seasons: summer, transition season, and winter. The Chengdu metropolitan area exhibits distinct subtropical monsoon humid climate characteristics and is mainly situated in the central subtropical region (Figure 2). During June to September, the average temperatures are relatively high and exhibit a stable distribution. The temperature fluctuations during these 4 months are minimal, thus designating them as the summer season. In comparison, the average temperatures from December to March are lower, especially in January. Although the average temperature and precipitation in March are slightly higher than in November, the seasonal classification pattern considers the coherence in month-to-month division. As a result, these 4 months are defined as the winter season. The average temperatures in April-May and October-November are relatively similar, as these months often serve as transitional periods between summer and winter. Therefore, the study defined March, April, May, and October as the transition seasons.
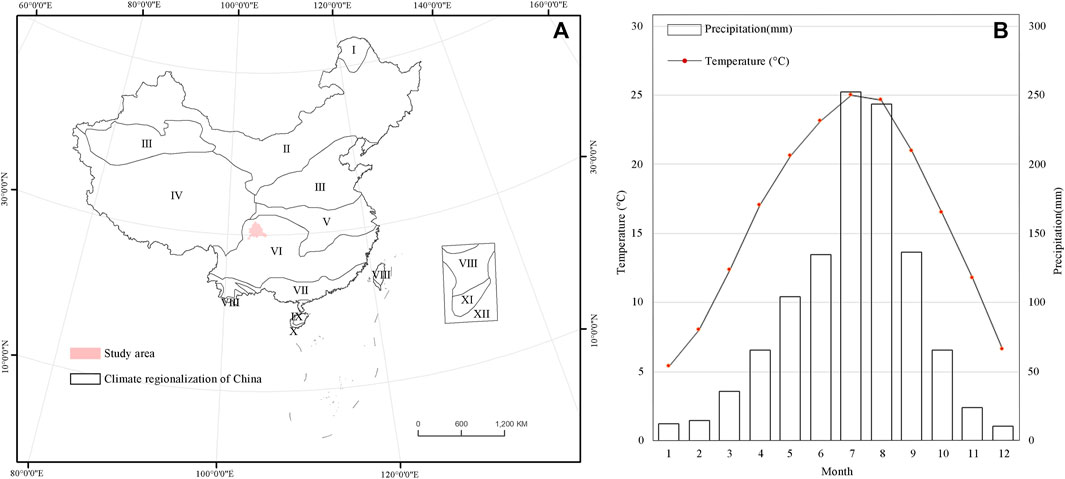
FIGURE 2. (A) The climate zone in which the study area is located (I. Northern Temperate Zone Ⅱ. Central Temperate Zone Ⅲ. Southern Temperate Zone Ⅳ. Highland climate zone V. Northern Subtropical VI. Central subtropics Ⅶ. Southern subtropics VIII. Northern subtropics Ⅸ. Northern subtropics X. Central tropics Ⅺ. Southern tropics Ⅻ. South Sea islands) (B) Average monthly temperature and precipitation in the central subtropics.
3 Data and methods
3.1 Data sources and processing
The data utilized in this study comprise LST data and potential driving force that affect LST. These factors include a digital elevation model, wind speed, humidity, slope, Shannon diversity index, edge density, maximum patch area index, patch density, road density, population density, night lighting, and vegetation cover, resulting in a total of 12 potential driving force (Figure 3). Please refer to (Table 1) for more details. To characterize the distribution characteristics of various influencing factors, this study employed the natural breakpoint method to reclassify the potential influencing factors into five levels ranging from 1 to 5. A higher level indicates a higher numerical value, and a more pronounced spatial expression of the corresponding potential influencing factor.
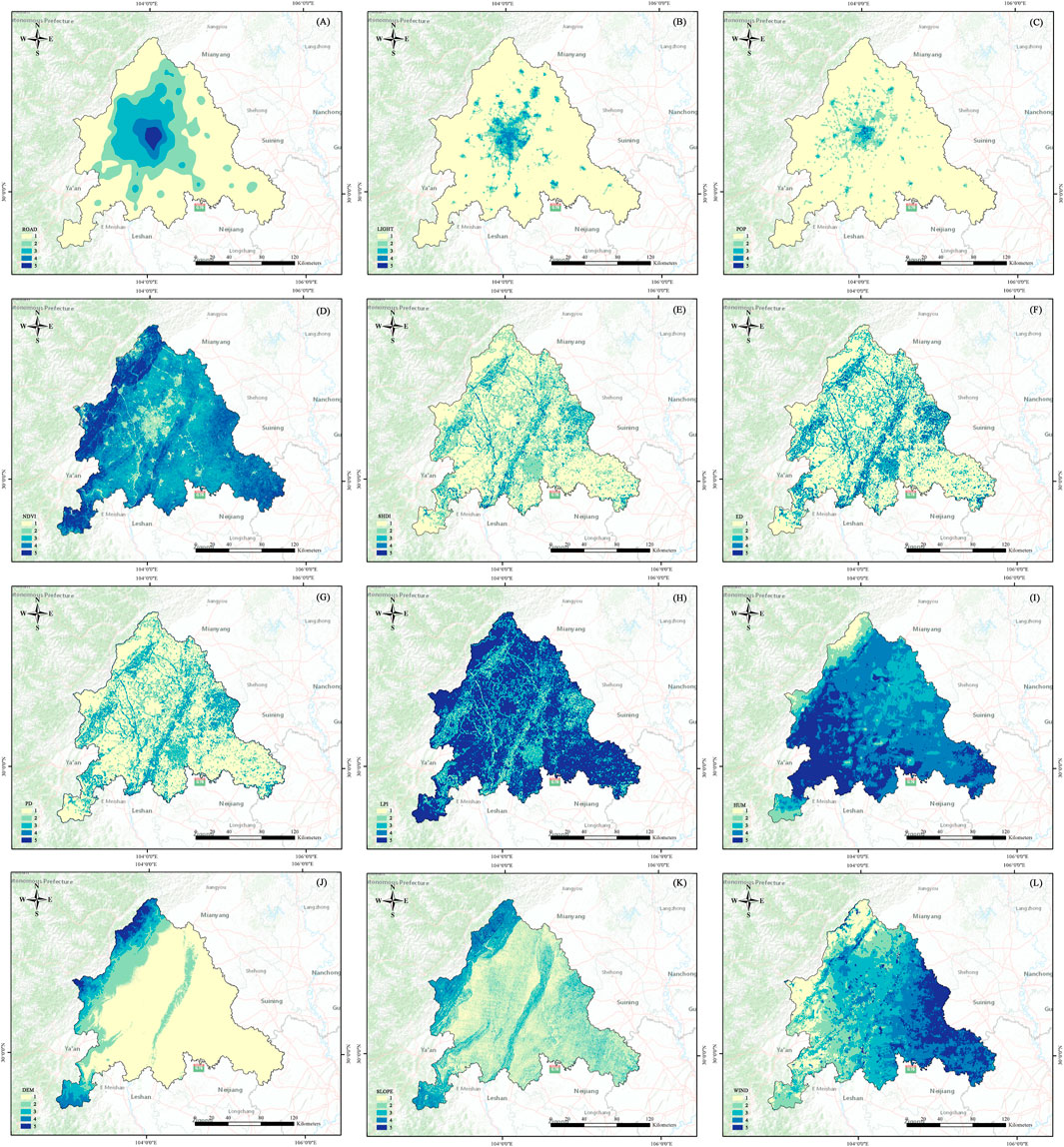
FIGURE 3. Potential driving force affecting LST change in the Chengdu metropolitan area (A) ROAD (B) LIGHT (C) POP (D) NDVI (E) SHDI (F) ED (G) PD (H) LPI (I) HUM (J) DEM (K) SLOPE (L) WIND.
The study utilized MODIS11A2 data obtained from the Terra and Aqua satellites, which are equipped with the important MODIS sensor. The sensor allows for the acquisition of LST data at four daily intervals, it was determined that MODIS11A2 data offers higher accuracy (Wan, 2008). Therefore, for this study, the 2020 MODIS11A2 data was utilized to obtain processed LST data for the Chengdu metropolitan area, which was sourced from LAADS DAAC.
3.2 Research methodology
The logical framework for the research presented is illustrated in the following (Figure 4). The main purpose is to summarize the LST distribution characteristics of Chengdu metropolitan area by using LST characteristics in different seasons, and 12 potential driving force are selected to analyze the potential factors affecting the spatial distribution pattern of heat islands in Chengdu metropolitan area, and to establish a season-al mathematical relationship model affecting the LST of Chengdu metropolitan area by correlation analysis, principal component analysis and multiple regression analysis, and finally summarize the analysis results for discussion.
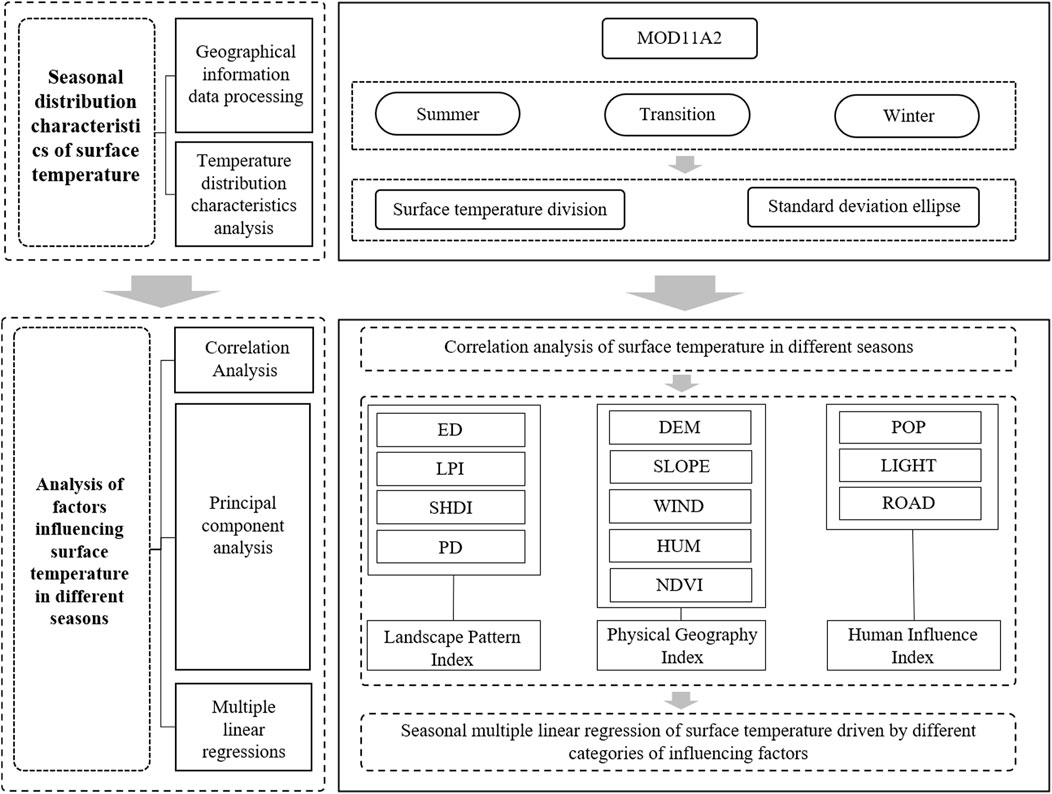
FIGURE 4. The framework for exploring the spatial and temporal distribution patterns of LST and the driving factors.
3.2.1 LST division
To investigate the seasonal fluctuations of Land Surface Temperature (LST) throughout the Chengdu metropolitan area, ArcGIS 10.8 was utilized to reclassify the LST data using the mean−standard deviation technique (Table 2). Among them, Ts represents the unit raster value of LST in Chengdu metropolitan area, μ represents the mean value of LST in Chengdu metropolitan area in different seasons, and std represents the standard deviation of LST in Chengdu metropolitan area in different seasons. Combining with related studies (Hu et al., 2022a; Hu and Li, 2022), we define the mid-temperature zone, second high-temperature zone and high-temperature zone as UHI regions with high heat sources.
3.2.2 Landscape pattern indices
Landscape pattern index is commonly employed to quantify landscape characteristics and effectively depict the shape and distribution features of various patches within a landscape. Based on previous studies (McGarigal et al., 2012; Zhang et al., 2020), landscape edge density (ED), maximum patch index (LPI), Shannon diversity index (SHDI), and patch density (PD) were selected in this study. And the optimal window size was selected to calculate the landscape pattern index using Fragstats 4.2 software, and the specific formula is shown in the table below (Table 3).
From Table 3, it can be observed that this study selects four different landscape pattern factors to represent distinct meanings. Among them, Edge Density (ED) is the ratio of the total perimeter of all patches to the total landscape area, often used to indicate the degree of landscape fragmentation by boundaries, reflecting the degree of landscape element fragmentation. The Largest Patch Index (LPI) is the ratio of the largest patch area to the total landscape area. It is used to calculate the proportion of the largest patch within the spatial unit, helping identify dominant patch types within the landscape and assessing human disturbance to the landscape. The Shannon Diversity Index (SHDI) is commonly used to measure landscape diversity, reflecting the uneven distribution of patches within the landscape. It can also detect changes in diversity and heterogeneity of the same landscape at different periods. In a landscape system, the more diverse the land use types, the richer the patch types, and correspondingly, the higher the SHDI value. Patch Density (PD) is the ratio of the total number of patches to the total landscape area. It characterizes the degree of landscape fragmentation caused by segmentation and reflects the degree of human disturbance to the landscape. A higher Patch Density index indicates a greater degree of landscape fragmentation within the landscape unit (Zheng et al., 2010; Li et al., 2012).
3.2.3 Standard deviation ellipse
The standard deviation ellipse is commonly used to study the spatial distribution characteristics of geographical elements, displaying their distribution patterns in space and identifying variations in the center of the elements. The long semi-axis of the ellipse represents the main direction of the element distribution, while the size of the short semi-axis indicates the degree of spatial aggregation. The larger the difference between the lengths of the long and short axes, the more pronounced the directional distribution of the element. The azimuth represents the angle in a clockwise direction from the north to the direction of the long axis of the ellipse, indicating the main direction of the element’s distribution (Zhao et al., 2022). Therefore, in this study, the standard deviation ellipse is used to quantitatively describe the spatial distribution and evolutionary characteristics of surface temperature in the Chengdu metropolitan area. The calculations are as follows:
In the equation, (
3.2.4 Correlation analysis
Correlation analysis is a statistical method used to assess the strength and direction of the relationship between two variables. In this study, this method is used to determine the correlation among various potential factors influencing surface temperature in the Chengdu metropolitan area, with a focus on determining the degree of correlation between surface temperature and factors such as elevation, slope, and humidity. Specifically, a random sampling tool in ArcGIS 10.8 was used to select 20,000 points and obtain their attribute values. Pearson correlation analysis was then conducted using SPSS software to calculate the Pearson correlation coefficients between each factor and surface temperature. Correlation analysis enables the identification of the main factors influencing surface temperature in the Chengdu metropolitan area and the assessment of the interrelationship among potential influencing factors. The correlation coefficient (R) can be expressed using the following formula:
In the formula, n represents the number of variables; xi represents the independent variable (in this case, the potential influencing factor); yi represents the dependent variable (in this case, the LST value). The range of the correlation coefficient R is |R|≤1. When the absolute value of R approaches 1, it indicates a stronger correlation between the variables; otherwise, it indicates a weaker correlation.
3.2.5 Principal component analysis
Principal component analysis (PCA) is a statistical method used for dimensionality reduction and data compression. Its main objective is to transform the original variables into a set of uncorrelated principal components to explain the variability in the data. In this study, PCA is employed to identify the main components or factors that explain the variations in surface temperature in the Chengdu metropolitan area. By reducing the number of variables, PCA helps simplify the model and better understand the underlying driving forces. Specifically, the study conducts PCA using SPSS software to analyze the potential influencing factors of surface temperature. The 16 potential influencing factors are subjected to dimensionality reduction based on their contribution rates, with values greater than 0.5 used as grouping criteria to classify them into different categories. This process eliminates the correlation among the factors and categorizes the 16 potential influencing factors into different groups of latent influencing factors.The calculation proceeded as follows:
Assuming that the original variables have m samples, with each sample having n observations, the matrix of the original variables is:
In the formula, X represents the potential driving force, n represents the number of potential driving force, and m represents the number of samples.
After obtaining each principal component, the extraction of principal components was performed according to Eq. 7:
Where,
Expressions for different categories of principal components:
Where, F1, F2 … Fn are the 1st principal component, 2nd principal component … nth principal component; a11, a21 … anm are the principal component coefficients.
3.2.6 Multiple linear regression
Multiple regression analysis is a statistical method used to establish the relationship between a dependent variable and multiple independent variables. In order to further investigate whether different categories of potential influencing factors have an impact on surface temperature, this study employs multiple linear regression to determine the effects of different categories of potential influencing factors on surface temperature. It identifies the important role played by each category of factors in the seasonal variation of surface temperature and their relative weights. This helps determine how surface temperature is influenced by different categories of potential influencing factors, and allows for targeted solutions to be proposed.
Assuming that there are independent variables x1, x2, x3 … xn and y is the dependent variable, then:
And in this study y denotes the LST magnitude and x1, x2, x3...xn denotes the potential impact factor.
The multiple regression coefficients obtained from the multiple linear regression model need to undergo collinearity diagnosis to determine the severity of collinearity in the multiple linear regression model. The commonly used VIF values are used for this purpose. The calculation formula is as follows:
R2 is the coefficient of determination in linear regression, which reflects the percentage of variation in the dependent variable explained by the regression equation. It can be obtained by squaring the multiple correlation coefficient between the dependent variable and the independent variables. If all VIF values are less than 10, it indicates that there is no multicollinearity problem in the model and the model construction is good.
In summary, this study identified 12 potential factors that affect the spatial distribution pattern of the urban heat island in the Chengdu metropolitan area. Through correlation analysis, principal component analysis (PCA), and multiple regression analysis, a seasonal mathematical model was established to explain the influences on surface temperature in the Chengdu metropolitan area. Specifically, correlation analysis was used to determine the relationships between factors, PCA was employed to reduce dimensionality and identify the main components, and multiple regression analysis was utilized to establish a mathematical model explaining the relationship between the dependent and independent variables. Therefore, these three research methods differ in analyzing the influencing factors of the urban heat island’s spatial distribution pattern in the Chengdu metropolitan area, and they complement each other. The comprehensive use of these methods provides a comprehensive understanding and modeling of the influencing factors of seasonal variations in surface temperature in the Chengdu metropolitan area.
4 Results
4.1 Temporal-spatial characteristics of LST in Chengdu metropolitan area
Figure 5 presents the annual LST patterns of Chengdu metropolitan area, which were extracted from MOD11A2 data and categorized into three phases: summer, transition season, and winter. During summer, the monthly mean LST was 35.3°C, with a maximum value of 46.5°C, a minimum of 19.4°C, and a standard deviation of 3.66. In the transition season, the monthly average LST reached 37.1°C, with a maximum value of 47.5°C, a minimum of 10.4°C, and a standard deviation of 5.09. As for winter, the monthly average LST was 9.3°C, with a maximum value of 17.4°C, a minimum of −8.1°C, and a standard deviation of 3.54.
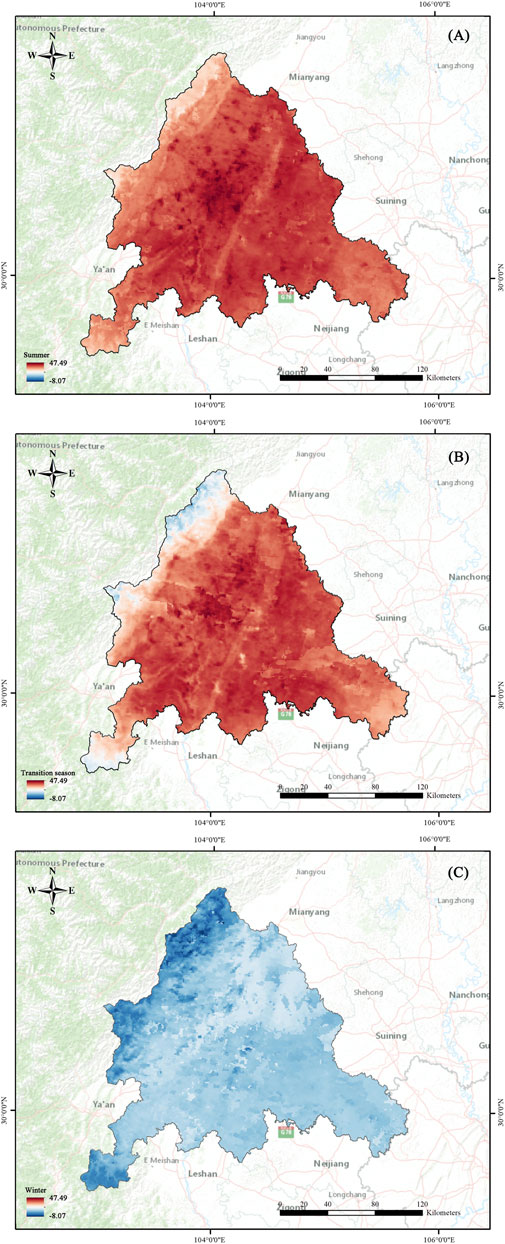
FIGURE 5. Spatial pattern of LST in Chengdu metropolitan area in different seasons: (A) summer (B) transition season (C) winter.
In order to obtain different characteristics of temporal and spatial changes of the UHI in the Chengdu metropolitan area, the study used the mean−standard deviation method to reclassify the LST into five zones: “high temperature zone, medium-high temperature zone, medium temperature zone, medium-low temperature zone, and low temperature zone” (Figure 6), in order to investigate the changes in area and space of the LST in different seasons.
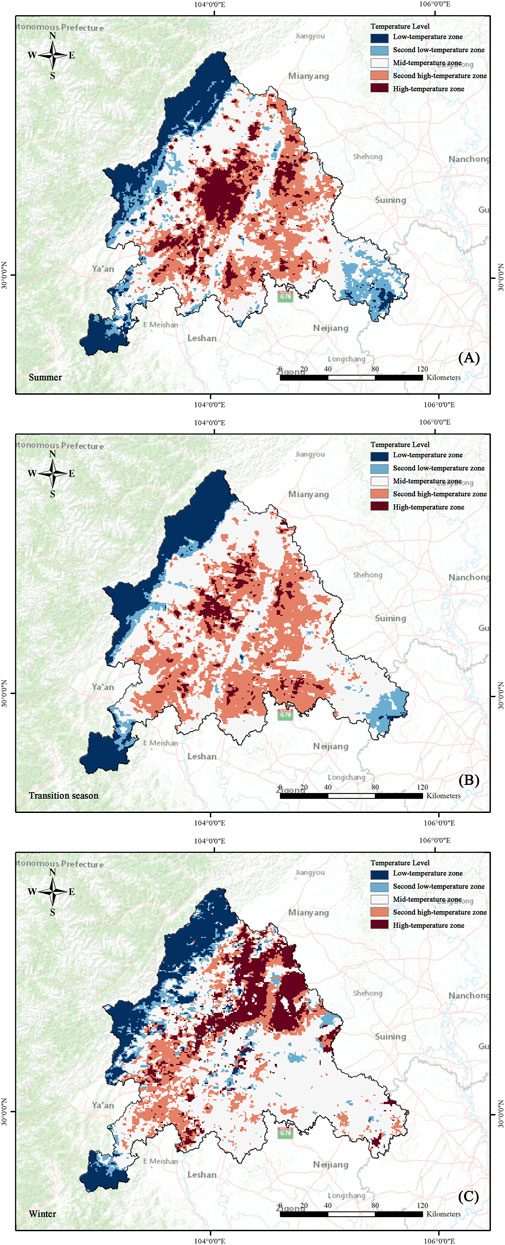
FIGURE 6. Spatial distribution of heat islands in Chengdu metropolitan area in different seasons: (A) summer (B) transition season (C) winter.
Based on the level values of LST in Chengdu metropolitan area after division, the area values of different temperature zones in Chengdu metropolitan area during the transition season, summer, and winter could be obtained. Based on the classification criteria in Table 3, as shown in Table 4: it could be found that the high-temperature zone and the second high-temperature zone of the Chengdu metropolitan area in summer have an area of 3328.25 km2 and 7778.57 km2 respectively, accounting for 10.06% and 23.50% of the total area of the Chengdu metropolitan area. Compared with the transition season, the area of high-temperature zones in summer is significantly higher, while compared with winter, the total area of high-temperature zones and the second high-temperature zones is significantly higher than in winter. From a seasonal perspective, the temperature in the Chengdu metropolitan area is generally high throughout the year, with relatively large areas of high-temperature zones and the second high-temperature zones in summer. In the transition season, the second high-temperature zone reaches its peak, accounting for the highest proportion (31.18%) among the three seasons; in winter, the high-temperature zone reaches its peak, accounting for the highest proportion (12.98%) among the three seasons (Figure 7).
In order to explore the spatial characteristics of surface temperature variations in the Chengdu metropolitan area, a standard deviation ellipse analysis was conducted. The analysis results (see Figure 8) show significant differences in the length of the major and minor axes of the ellipses for the three seasons. It can be observed that the temperature variations in the Chengdu metropolitan area exhibit a strong directional pattern in different seasons. From the figure, it can be seen that the temperature variations in the Chengdu metropolitan area follow a northeast-southwest direction. In the summer season, the length of the minor axis of the ellipse is significantly smaller than that in the transitional and winter seasons. The transitional season also shows a relatively smaller value compared to the winter season. Therefore, it can be inferred that the temperature variations in the summer season exhibit the most significant clustering characteristics, followed by the transitional and winter seasons. From winter to transition season, the heat island center moved southeastward by 49.3 km at a deviation angle of 7.5. From transition season to summer, the heat island center shifted northwest by 22.4 km with a deviation angle of 6.3°. Overall, the LST in Chengdu metropolitan area changed significantly with the seasons. In summer, the high-temperature zone was concentrated in the central area. In the transition season, the dispersion of the high-temperature zone caused the center of the heat island to move towards the plain area. In winter, most areas were normal, with low-temperature zones and second low temperature zones located in the northern mountainous areas, causing the center of the heat island to move towards the northern mountainous areas.
4.2 Potential drivers of LST in the Chengdu metropolitan area
To identify the factors influencing LST, the study considered various potential drivers, including POP, LIGHT, DEM, SLOPE, HUM, WIND, ROAD, ED, LPI, PD, SHDI, and NDVI. These factors were analyzed using SPSS and ArcGIS 10.8 to determine their correlation with LST in different seasons. The Spearman correlation analysis method was employed to calculate correlation coefficients between the potential factors and LST (Figure 9), and the results passed the significance test. Specifically, LPI, NDVI, DEM, and SLOPE were negatively correlated with LST in different seasons, indicating that they can significantly reduce LST. On the other hand, ED, PD, SHDI, WIND, HUM, ROAD, POP, and LIGHT were positively correlated with LST in different seasons, indicating that they can significantly increase LST. Meanwhile, the degree of influence on LST varies in different seasons, with slight differences observed in different seasons.
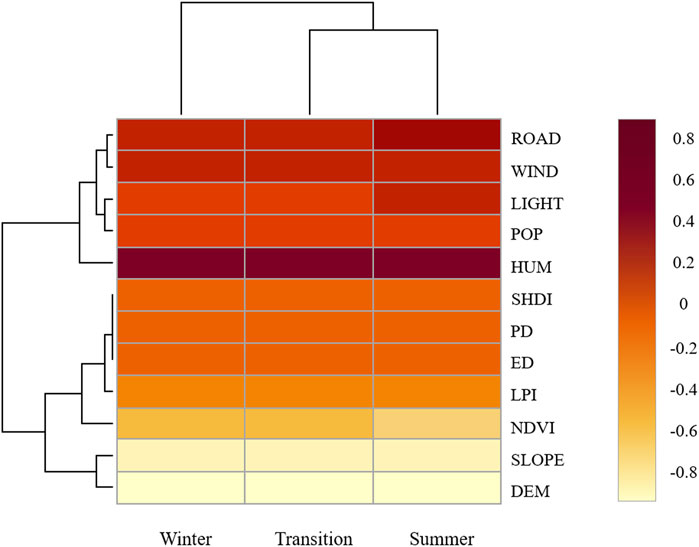
FIGURE 9. The Spearman’s rank correlation coefficient was utilized to analyze the influence of various potential factors on LST across diverse seasons.
In order to further explore the differences in the driving factors of land surface temperature in different seasons, this study used principal component analysis to further investigate them. Based on the findings presented in Tables 5, 6, principal component analysis (PCA) was employed to reduce the dimensionality of the twelve potential factors. The PCA results revealed that the first three principal components possessed eigenvalues greater than 1, suggesting that they accurately captured the variations in LST. Based on the component matrix after four iterations of rotation, we identified the three main factors affecting LST changes in Chengdu metropolitan area: landscape pattern, natural geography, and human influence factors.
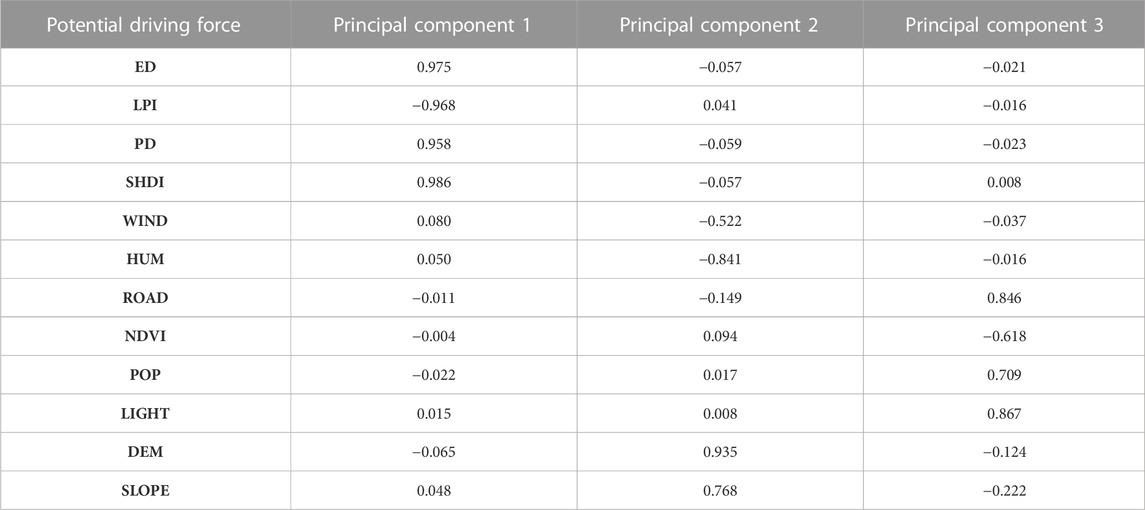
TABLE 6. Contribution of potential driving force affecting the magnitude of LST in Chengdu metropolitan area.
4.3 Seasonal relationship between LST and potential drivers in Chengdu metropolitan area
The study further explored the potential factors affecting LST in Chengdu metropolitan area by using the three principal components identified through principal component analysis, and established regression equations based on seasonal LST. From Tables 6, 7, it can be observed that the linear regression coefficients (R2) between the independent variables and dependent variables have different values in different seasons. When analyzing the temperature data for summer, the R2 is 0.621, indicating that the regression equation can explain 62.1% of the sample data. When analyzing the temperature data for the transitional season, the R2 is 0.746, indicating that the regression equation can explain 74.6% of the sample data. When analyzing the temperature data for winter, the R2 is 0.497, indicating that the regression equation can explain 49.7% of the sample data. Furthermore, in the collinearity analysis, the VIF (variance inflation factor) and TLR (tolerance limit ratio) values of Principal Component 1 are 9.7408 and 0.1027, respectively. For Principal Component 2, the VIF and TLR values are 3.6464 and 0.2742, respectively. For Principal Component 3, the VIF and TLR values are 7.2896 and 0.1372, respectively. It can be seen that the VIF and TLR values of Principal Component 2 (natural geography) are relatively good, indicating that the regression equation does not have a serious collinearity problem. However, the VIF and TLR values of Principal Component 1 (landscape pattern) and Principal Component 3 (socio-economics) are relatively poor. Based on the VIF and Tolerance values, it can be concluded that the regression equation does not have a serious collinearity problem.
Specifically, from Table 8, it can be seen that ED, PD, SHDI, NDVI, DEM, and SLOPE are negatively correlated with land surface temperature, while WIND, HUM, ROAD, POP, and LIGHT are positively correlated with land surface temperature. Overall, natural geographical factors such as WIND, HUM, DEM, and SLOPE have a greater impact on land surface temperature, followed by social and economic factors such as POP, LIGHT, NDVI, and ROAD. Landscape pattern factors such as ED, LPI, PD, and SHDI have the weakest impact on land surface temperature. In terms of seasonality, POP (0.125) and LIGHT (0.16) reach their maximum values in summer, while DEM (−0.857), SLOPE (−0.739), NDVI (−0.203), HUM (0.747), WIND (0.453), and ROAD (0.298) reach their maximum values in the transition season. ED (−0.08), LPI (0.089), PD (−0.076), and SHDI (−0.08) reach their maximum values in winter.
5 Discussion
5.1 Temporal-spatial characteristics of seasonal LST in Chengdu metropolitan area
The study area is situated in a basin typical of its kind, bounded by the Chengdu Plain to the east, the Minshan Mountains to the north, and the Emei Mountains to the southwest. Results from the analysis of LST reveal conspicuous spatial disparities in the UHI effect across the Chengdu metropolitan area. Specifically, areas with relatively low temperatures have formed in proximity to the Minshan Mountains and the Emei Mountains, situated in the north and southwest regions of the study area, respectively, while regions with high temperatures have emerged in the central plain. Collectively, these observations indicate that the thermal environment of the Chengdu metropolitan area exhibits a distinctive feature characterized by high temperatures in the southeast and low temperatures in the northwest.
By considering the spatiotemporal characteristics, it is observed that during summer, the regions with high and second high temperatures are predominantly located in the Chengdu, Deyang, Meishan, and Ziyang areas, with few other cities and counties also exhibiting localized hotspots. Meanwhile, the areas with low and second low temperatures are distributed mainly in the northern mountainous regions of Dujiangyan and the southwestern areas of the study region. In the transitional season, high and second high temperature regions exhibit more dispersed patterns with reduced regional coherence, whereas, low and second low temperature areas have a more concentrated distribution in the northwestern and southwestern sectors of the Chengdu metropolitan area. In winter, the high-temperature zone and the second high-temperature zone are mainly distributed in the northeast of the Chengdu metropolitan area, and are divided by the Longquan Mountains in a northeast-southwest direction. The low-temperature zone and the second low-temperature zone are distributed in the mountains in the northwest of the Chengdu metropolitan area and the Longquan Mountains in the southeast of Chengdu city. These results are consistent with previous research (Zhou et al., 2014).
Considering the distribution of cities, high-temperature areas are mainly concentrated in cities such as Chengdu, Deyang, Ziyang, Guanghan, and Meishan. These cities are located in the plain area, with dense population, developed economy, high level of urban construction, and compact distribution of buildings, which cause the LST to rise (Zhao et al., 2018; Hu et al., 2022b; Ren et al., 2022). And the study area is located inside the Sichuan Basin, surrounded by high-altitude mountains, which cannot disperse the heat airflow, resulting in the accumulation of heat island in the basin area between the mountains. This exacerbates the urban heat island effect (Wang Z. et al., 2022). Concentrated regions of low temperature are found primarily in the mountainous zones located in the northwestern and southwestern sectors. These areas are characterized by high-altitude mountainous terrain with abundant water systems and lush vegetation. Water systems and vegetation cover density can significantly contribute to mitigating the UHI effect. Water systems can directly cool the surface, while vegetation can reduce LST through transpiration (Jin, 2012; Hu et al., 2022c).
5.2 Exploring the driving relationship of seasonal LST in Chengdu metropolitan area
Based on the principal component analysis and judgment of the content of potential influencing factors, the factors affecting surface temperature in the Chengdu metropolitan area are categorized into three groups: landscape pattern factors, natural geographic factors, and socioeconomic factors. Current research indicates that socioeconomic factors play a dominant role in the urban heat island effect in plain areas (Zhou et al., 2016; Sun et al., 2019). However, through the correlation coefficient analysis of multiple linear regression, it is revealed that natural geographic factors have a significant advantage in the formation process of the urban heat island in the Chengdu metropolitan area. It is worth noting that the current research findings may not be quantitatively refutable due to differences in study design, data sources, and methods, which lead to certain limitations. On the one hand, the selection of influencing factors has a significant impact on research results. In this study, typical driving factors were selected as independent variables in the regression analysis, covering different aspects of variables as much as possible. However, it is still challenging to fully consider all possible factors. On the other hand, spatial and temporal scales also influence research outcomes. The urban heat island effect exhibits different patterns at different spatial scales (such as within cities and surrounding areas) and temporal scales (such as different seasons and time periods).
The research findings of this study may be related to the geographical environment of the study area, in which the Chengdu plain in the Sichuan Basin is surrounded by large mountains to the north and west. As a result, hot air is inhibited from rising, leading to sinking and resulting in warmer temperatures in the plain area. Moreover, in areas with higher elevations in the study area, a large number of vegetation trees are planted, and the increase in vegetation can effectively reduce the LST (Wang and Akbari, 2016). Among the landscape pattern factors, ED, PD, and SHDI are negatively correlated with LST, while only LPI has a positive correlation with LST, which indicates that most landscape pattern factors can reduce LST. Wind speed and humidity have a positive correlation with LST, indicating that wind speed and humidity can increase LST (Zhou et al., 2016). The location of the Chengdu metropolitan area, situated within a basin characterized by low wind speed and high humidity, is a determining factor. Corroborating the positive correlation between wind speed and LST, higher LST values are observed when wind speed is low. In the plain areas within the basin, the prevailing winds often come from inland regions. According to previous research (Al-Obaidi et al., 2021), when the winds originate from inland areas, they tend to generate a strong urban heat island effect. The presence of water in the atmosphere is known to exert a warming effect during periods of falling temperatures and a cooling effect during periods of rising temperatures, which are referred to as the “constant temperature effect,” ultimately leading to the maintenance of the surrounding temperature. However, the perpetual low wind speed in the Chengdu area impedes the cooling effect, thereby causing the land surface temperature (LST) to remain persistently high (Zong et al., 2019; Wu et al., 2021). Furthermore, the positive correlation identified between human-influenced factors and LST indicates that anthropogenic activities have a notable enhancing impact on the urban heat island phenomenon. Human construction activities will significantly affect the changes in urban climate (Ren et al., 2022), and the continuous expansion of cities and human energy consumption will generate a large amount of heat, resulting in an increase in LST.
In addition to the overall impact of indicators related to human activities leading to an increase in surface temperature, our further research has revealed that human activities are influenced by seasonal variations, showing varying degrees of impact on surface temperature. The effects of potential influencing factors on surface temperature also vary across different seasons. Specifically, during the summer season, social-economic factors such as population (POP) and nighttime light (LIGHT) exert a strong influence on surface temperature, which is more significant than during the transitional and winter seasons. On the other hand, road density (ROAD) shows a lower impact during the transitional season compared to the winter season. Additionally, there is a positive correlation between road density, nighttime light, population, and surface temperature, indicating that human activities have a more pronounced effect on surface temperature during the summer, leading to an increase in surface temperature. In the transitional season, natural geographical factors (WIND, HUM, DEM, SLOPE) have a greater impact on surface temperature compared to the summer and winter seasons, suggesting that natural geographical factors have a more significant influence on surface temperature during the transitional season. Moreover, the normalized difference vegetation index (NDVI) exhibits a more pronounced impact on surface temperature during the transitional season.
At the same time, besides human activities and natural factors, this study also considers other potential factors that may influence surface temperature, including landscape pattern indices. On one hand, changes in surface temperature can be influenced by the combined effects of multiple potential driving factors. On the other hand, the study of the aforementioned factors reveals that landscape pattern factors have a greater impact on surface temperature during the winter season compared to the transitional and summer seasons, indicating that landscape pattern factors have a more pronounced effect on surface temperature during the winter season. In summary, different categories of potential influencing factors have significant differences in their effects on surface temperature. Social-economic factors have the most significant impact during the summer season, natural geographical factors have the most significant impact during the transitional season, and landscape pattern factors have the most significant impact during the winter season.
5.3 Seasonal guidance strategies to mitigate urban heat island phenomenon in Chengdu metropolitan area
By examining the determinants of UHI, it becomes evident that both human activities and natural geographical characteristics exhibit seasonally-specific influences on UHI intensity in built environments. In light of these findings, several potential strategies may be recommended to mitigate the adverse consequences of UHI phenomena: 1) During the summer season, a key focus is placed on the significant impact of socio-economic factors, particularly in the urban development process. Adjusting urban land-use patterns is emphasized to alleviate population concentration. This entails optimizing and enhancing Chengdu’s role as the central urban area, leading to the development of surrounding small towns. It also involves extending the reach of public service facilities to the periphery, and rational control of development intensity and population density within the metropolitan area. These measures aim to mitigate the rise in surface temperature caused by population agglomeration. Furthermore, aligning with the direction of urban wind corridors, adjustments are made to the arrangement of buildings and road networks. This aims to prevent blockages in the urban wind corridors due to issues with urban road and building layouts. The goal is to ensure the ventilation pathways in the city are unobstructed, effectively mitigating the urban heat island effect during the summer season (Gedzelman et al., 2003; Ngarambe et al., 2021). 2) During the transitional season, a focus is placed on the critical influence of natural geographical factors. This involves establishing an urban ecological engineering network and an urban park green space system to enhance the city’s green environment. The primary framework of this ecological network is centered around the Chengdu Tianfu Greenway, creating an ecological network system. Additionally, the Chengdu Urban Ecological Zone is developed, accompanied by the optimization of the city’s landscape spatial structure. This optimization aims to interconnect the city’s lake water systems and vegetation green spaces, thereby enhancing the overall continuity of the urban landscape. Emphasis is placed on the ecological protection and restoration of rivers, lakes, and water systems, as well as mountainous vegetation within the metropolitan area. This effort extends to promoting ecological conservation and restoration in river basins like the Min River Basin, as well as strengthening ecological development in regions such as the Min Mountains and Qionglai Mountains. Through these initiatives, adjustments are made to the internal ecological and climatic features of the metropolitan area, aiming to optimize the Humidity (HUM) and Wind (WIND) patterns within the metropolitan area. This strategy contributes to alleviating the rise in urban surface temperature (Das et al., 2020; Hu et al., 2022a; Ren et al., 2022). 3) During the winter season, a key emphasis should be placed on the influence of landscape pattern factors. On one hand, it involves establishing comprehensive landscape elements to ensure the integrity of the urban ecological spaces. This includes advancing the ecological protection and restoration of Longquan Mountain, located at the central position of the metropolitan area, and increasing the edge density of landscapes within the urban spatial scope. This is to prevent human-induced disruptions to urban landscape spaces. Simultaneously, attention should be given to the arrangement of various types of landscape elements, enhancing the preservation of ecological diversity within the metropolitan area, diversifying landscape patches, and increasing the Spatial Heterogeneity Diversity Index (SHDI) value. These efforts aim to alleviate urban surface temperatures (Yu et al., 2019; Han et al., 2022). On the other hand, it involves constructing a network of urban ecological corridors, with mountain formations like Longquan Mountain, Longmen Mountain, and Qionglai Mountain serving as ecological barriers, and river systems such as the Minjiang River and Tuojiang River serving as green ecological corridors. This aims to establish an interconnected ecological system pattern. Through ecosystem restoration and optimization of various branch nodes, the goal is to link landscape nodes into a network, integrate fragmented landscape spaces, and form extensive ecological landscape patches. This approach also aims to reduce the Maximum Patch Area Index of landscapes within the urban spatial scope. Furthermore, it involves proposing corresponding network structure optimization strategies based on evaluating the potential ecological benefits of nodes and corridors within the network structure (Hu et al., 2022a; 2022c).
It is worth noting that the diverse guiding strategies mentioned above are based on an analysis of seasonal driving relationships. They inherently allow for the simultaneous implementation of various measures. However, emphasizing phased measures can accurately and effectively reduce urban surface temperatures, thus establishing a scientific and feasible theoretical basis for the formulation of relevant phased policies.
5.4 The limitations of the study
The limitations of this study are as follows: Firstly, the division of seasons in this study was based on monthly average temperature and precipitation, which may not accurately reflect the seasonal variations. Using alternative data sources for season delineation could improve the accuracy of seasonal changes. Secondly, the study employed multiple linear regression analysis to identify the impact of different factors on surface temperature, but incorporating other methods such as geographic detectors, random forests, and geographically weighted regression models could enhance the model construction and analysis process. Additionally, the study only collected data on 12 influencing factors on surface temperature. Collecting data on additional factors such as floor area ratio, building density, building height, haze pollution, and coastal wind circulation would contribute to a more comprehensive and scientifically grounded study. Lastly, the research primarily focused on the seasonal and spatial variations of surface temperature in the study area in 2020 due to the feasibility of obtaining corresponding data on influencing factors for that specific year. However, for studying the cross-year seasonal and spatial variations of surface temperature, acquiring data on influencing factors for different years poses challenges. Therefore, future research should explore the characteristics of cross-year variations in surface temperature and differences in driving factors, taking into consideration the feasibility of data acquisition.
6 Conclusion
Since the Industrial Revolution, human society has undergone rapid development, and the rate of urbanization has also increased significantly. However, as a result, there has been a significant increase in global LST. This rise in temperature has already had a huge impact on human production and life. Therefore, how to mitigate the increase in LST has become an important topic that urgently needs to be explored across various disciplines. The investigation of the Chengdu metropolitan area revealed a distinct spatial pattern of LST, characterized by a concentration of high temperatures in the central regions and lower temperatures in the western regions. Both natural geographical factors and human activities factors play a greater role in the size of LST. Among these variables, DEM and SLOPE exhibit an inverse relationship with LST, whereas WIND and HUM demonstrate a positive association with LST. In terms of human activities factors, POP, LIGHT, and ROAD are positively correlated with LST, while the impact of landscape pattern factors on LST is relatively small. Simultaneously, the influence of potential driving force on LST shows marked seasonal variation. In summer, the impact of POP, ROAD, and LIGHT on LST is significantly higher than in other seasons, while in the transitional season, the impact of WIND, HUM, DEM, SLOPE, and NDVI on LST is significantly higher than in other seasons. In winter, the impact of landscape pattern factors on LST is relatively large. Therefore, seasonal guidance strategies need to be adopted to effectively alleviate the rise in urban LST, including the rational use of terrain and topography, the increase of green vegetation within the city, and the regulation of human activities. It is hoped that this study can provide valuable reference and guidance for the future urban planning, design, and operational management of the research area, and lay a research basis for guiding other regions to develop phased measures to alleviate UHI.
Data availability statement
The original contributions presented in the study are included in the article/Supplementary material, further inquiries can be directed to the corresponding author.
Author contributions
CH; writing–revision of articles, software, supervision. GH; writing–original draft, software, data curation. ZW; data curation, software, supervision. All authors contributed to the article and approved the submitted version.
Funding
Key Project of National Social Science Foundation of China (21AZD048), National Natural Science Foundation of China (41901390, 51408248), Natural Science Foundation of Hubei Province (2021CFB012).
Conflict of interest
The authors declare that the research was conducted in the absence of any commercial or financial relationships that could be construed as a potential conflict of interest.
Publisher’s note
All claims expressed in this article are solely those of the authors and do not necessarily represent those of their affiliated organizations, or those of the publisher, the editors and the reviewers. Any product that may be evaluated in this article, or claim that may be made by its manufacturer, is not guaranteed or endorsed by the publisher.
References
Al-Obaidi, I., Rayburg, S., Półrolniczak, M., and Neave, M. (2021). Assessing the impact of wind conditions on urban heat islands in large Australian cities. J. Ecol. Eng. 22, 1–15. doi:10.12911/22998993/142967
Correa, E., Ruiz, M. A., Canton, A., and Lesino, G. (2012). Thermal comfort in forested urban canyons of low building density. An assessment for the city of Mendoza, Argentina. Build. Environ. 58, 219–230. doi:10.1016/j.buildenv.2012.06.007
Das, D. N., Chakraborti, S., Saha, G., Banerjee, A., and Singh, D. (2020). Analysing the dynamic relationship of land surface temperature and landuse pattern: A city level analysis of two climatic regions in India. City Environ. Interact. 8, 100046. doi:10.1016/j.cacint.2020.100046
Fang, C., Yin, H., Han, L., Ma, S., He, X., and Huang, G. (2021). Effects of semi-permeable membrane covering coupled with intermittent aeration on gas emissions during aerobic composting from the solid fraction of dairy manure at industrial scale. Econ. Geogr. 41, 1–9. doi:10.1016/j.wasman.2021.05.030
Gao, Y., Zhao, J., and Han, L. (2022). Exploring the spatial heterogeneity of urban heat island effect and its relationship to block morphology with the geographically weighted regression model. Sustain. Cities Soc. 76, 103431. doi:10.1016/j.scs.2021.103431
Gedzelman, S. D., Austin, S., Cermak, R., Stefano, N., Partridge, S., Quesenberry, S., et al. (2003). Mesoscale aspects of the urban heat island around New York city. Theor. Appl. Climatol. 75, 29–42. doi:10.1007/s00704-002-0724-2
Geng, X., Zhang, D., Li, C., Yuan, Y., Yu, Z., and Wang, X. (2023). Impacts of climatic zones on urban heat island: spatiotemporal variations, trends, and drivers in China from 2001–2020. Sustain. Cities Soc. 89, 104303. doi:10.1016/j.scs.2022.104303
Grimm, N. B., Faeth, S. H., Golubiewski, N. E., Redman, C. L., Wu, J., Bai, X., et al. (2008). Global change and the ecology of cities. Science 319, 756–760. doi:10.1126/science.1150195
Guo, A., Yang, J., Xiao, X., Xia (Cecilia), J., Jin, C., and Li, X. (2020). Influences of urban spatial form on urban heat island effects at the community level in China. Sustain. Cities Soc. 53, 101972. doi:10.1016/j.scs.2019.101972
Guo, G., Wu, Z., and Chen, Y. (2020). Evaluation of spatially heterogeneous driving forces of the urban heat environment based on a regression tree model. Sustain. Cities Soc. 54, 101960. doi:10.1016/j.scs.2019.101960
Guo, J., Han, G., Xie, Y., Cai, Z., and Zhao, Y. (2020). Exploring the relationships between urban spatial form factors and land surface temperature in mountainous area: a case study in chongqing city, China. Sustain. Cities Soc. 61, 102286. doi:10.1016/j.scs.2020.102286
Han, S., Li, W., Kwan, M.-P., Miao, C., and Sun, B. (2022). Do polycentric structures reduce surface urban heat island intensity? Appl. Geogr. 146, 102766. doi:10.1016/j.apgeog.2022.102766
Hu, C., and Li, H. (2022). Reverse thinking: the logical system research method of urban thermal safety pattern construction, evaluation, and optimization. Remote Sens. 14, 6036. doi:10.3390/rs14236036
Hu, C., Wang, Z., Huang, G., and Ding, Y. (2022a). Construction, evaluation, and optimization of a regional ecological security pattern based on MSPA–circuit theory approach. Int. J. Environ. Res. Public Health 19, 16184. doi:10.3390/ijerph192316184
Hu, C., Wang, Z., Li, J., Liu, H., and Sun, D. (2022b). Quantifying the temporal and spatial patterns of ecosystem services and exploring the spatial differentiation of driving factors: A case study of Sichuan Basin, China. Front. Environ. Sci. 10, 927818. doi:10.3389/fenvs.2022.927818
Hu, C., Wang, Z., Wang, Y., Sun, D., and Zhang, J. (2022c). Combining MSPA-MCR model to evaluate the ecological network in wuhan, China. Land 11, 213. doi:10.3390/land11020213
Imhoff, M. L., Zhang, P., Wolfe, R. E., and Bounoua, L. (2010). Remote sensing of the urban heat island effect across biomes in the continental USA. Remote Sens. Environ. 114, 504–513. doi:10.1016/j.rse.2009.10.008
Jin, M. S. (2012). Developing an index to measure urban heat island effect using satellite land skin temperature and land cover observations. J. Clim. 25, 6193–6201. doi:10.1175/JCLI-D-11-00509.1
Lai, J., Zhan, W., Huang, F., Quan, J., Hu, L., Gao, L., et al. (2018). Does quality control matter? Surface urban heat island intensity variations estimated by satellite-derived land surface temperature products. ISPRS J. Photogrammetry Remote Sens. 139, 212–227. doi:10.1016/j.isprsjprs.2018.03.012
Li, X., Zhou, W., Ouyang, Z., Xu, W., and Zheng, H. (2012). Spatial pattern of greenspace affects land surface temperature: evidence from the heavily urbanized Beijing metropolitan area, China. Landsc. Ecol. 27, 887–898. doi:10.1007/s10980-012-9731-6
Li, X., Zhou, W., and Ouyang, Z. (2013). Relationship between land surface temperature and spatial pattern of greenspace: what are the effects of spatial resolution? Landsc. Urban Plan. 114, 1–8. doi:10.1016/j.landurbplan.2013.02.005
Liao, Y., Shen, X., Zhou, J., Ma, J., Zhang, X., Tang, W., et al. (2022). Surface urban heat island detected by all-weather satellite land surface temperature. Sci. Total Environ. 811, 151405. doi:10.1016/j.scitotenv.2021.151405
Liu, H., Huang, B., Zhan, Q., Gao, S., Li, R., and Fan, Z. (2021a). The influence of urban form on surface urban heat island and its planning implications: evidence from 1288 urban clusters in China. Sustain. Cities Soc. 71, 102987. doi:10.1016/j.scs.2021.102987
Liu, H., Zheng, L., and Liao, M. (2021b). Dynamics of vegetation change and its relationship with nature and human activities — a case study of poyang lake basin, China. J. Sustain. For. 40, 47–67. doi:10.1080/10549811.2020.1738947
Luo, P., Yu, B., Li, P., Liang, P., Liang, Y., and Yang, L. (2023). How 2D and 3D built environments impact urban surface temperature under extreme heat: A study in Chengdu, China. Build. Environ. 231, 110035. doi:10.1016/j.buildenv.2023.110035
McGarigal, K., Cushman, S. A., and Ene, E. (2012). “FRAGSTATS v4: spatial pattern analysis program for categorical and continuous maps,” in Computer software program produced by the authors at the University of Massachusetts (Amherst), 15.
Ngarambe, J., Oh, J. W., Su, M. A., Santamouris, M., and Yun, G. Y. (2021). Influences of wind speed, sky conditions, land use and land cover characteristics on the magnitude of the urban heat island in seoul: an exploratory analysis. Sustain. Cities Soc. 71, 102953. doi:10.1016/j.scs.2021.102953
Peng, J., Xie, P., Liu, Y., and Ma, J. (2016). Urban thermal environment dynamics and associated landscape pattern factors: A case study in the Beijing metropolitan region. Remote Sens. Environ. 173, 145–155. doi:10.1016/j.rse.2015.11.027
Peng, J., Jia, J., Liu, Y., Li, H., and Wu, J. (2018). Seasonal contrast of the dominant factors for spatial distribution of land surface temperature in urban areas. Remote Sens. Environ. 215, 255–267. doi:10.1016/j.rse.2018.06.010
Ren, Y., Deng, L.-Y., Zuo, S.-D., Song, X.-D., Liao, Y.-L., Xu, C.-D., et al. (2016). Quantifying the influences of various ecological factors on land surface temperature of urban forests. Environ. Pollut. 216, 519–529. doi:10.1016/j.envpol.2016.06.004
Ren, T., Zhou, W., and Wang, J. (2021). Beyond intensity of urban heat island effect: a continental scale analysis on land surface temperature in major Chinese cities. Sci. Total Environ. 791, 148334. doi:10.1016/j.scitotenv.2021.148334
Ren, J., Yang, J., Zhang, Y., Xiao, X., Xia, J. C., Li, X., et al. (2022). Exploring thermal comfort of urban buildings based on local climate zones. J. Clean. Prod. 340, 130744. doi:10.1016/j.jclepro.2022.130744
Sun, R., Lü, Y., Yang, X., and Chen, L. (2019). Understanding the variability of urban heat islands from local background climate and urbanization. J. Clean. Prod. 208, 743–752. doi:10.1016/j.jclepro.2018.10.178
Sun, Z., Li, Z., and Zhong, J. (2022). Analysis of the impact of landscape patterns on urban heat islands: A case study of Chengdu, China. Int. J. Environ. Res. Public Health 19, 13297. doi:10.3390/ijerph192013297
Thompson, R. D., and Perry, A. H. (1997). Applied climatology: principles and practice. Psychology Press.
Wan, Z. (2008). New refinements and validation of the MODIS Land-Surface Temperature/Emissivity products. Remote Sens. Environ. 112, 59–74. doi:10.1016/j.rse.2006.06.026
Wang, Y., and Akbari, H. (2016). The effects of street tree planting on Urban Heat Island mitigation in Montreal. Sustain. Cities Soc. 27, 122–128. doi:10.1016/j.scs.2016.04.013
Wang, Y., Du, H., Xu, Y., Lu, D., Wang, X., and Guo, Z. (2018). Temporal and spatial variation relationship and influence factors on surface urban heat island and ozone pollution in the Yangtze River Delta, China. Sci. Total Environ. 631–632, 921–933. doi:10.1016/j.scitotenv.2018.03.050
Wang, W., Samat, A., Abuduwaili, J., and Ge, Y. (2021). Quantifying the influences of land surface parameters on LST variations based on GeoDetector model in Syr Darya Basin, Central Asia. J. Arid Environ. 186, 104415. doi:10.1016/j.jaridenv.2020.104415
Wang, Z., Meng, Q., Allam, M., Hu, D., Zhang, L., and Menenti, M. (2021). Environmental and anthropogenic drivers of surface urban heat island intensity: A case-study in the yangtze river delta, China. Ecol. Indic. 128, 107845. doi:10.1016/j.ecolind.2021.107845
Wang, Q., Wang, H., Chang, R., Zeng, H., and Bai, X. (2022). Dynamic simulation patterns and spatiotemporal analysis of land-use/land-cover changes in the Wuhan metropolitan area, China. Ecol. Model. 464, 109850. doi:10.1016/j.ecolmodel.2021.109850
Wang, Z., Sun, D., Hu, C., Wang, Y., and Zhang, J. (2022). Seasonal contrast and interactive effects of potential drivers on land surface temperature in the Sichuan Basin, China. Remote Sens. 14, 1292. doi:10.3390/rs14051292
Ward, K., Lauf, S., Kleinschmit, B., and Endlicher, W. (2016). Heat waves and urban heat islands in europe: a review of relevant drivers. Sci. Total Environ. 569, 527–539. doi:10.1016/j.scitotenv.2016.06.119
Wu, S., Yang, H., Luo, P., Luo, C., Li, H., Liu, M., et al. (2021). The effects of the cooling efficiency of urban wetlands in an inland megacity: a case study of Chengdu, southwest China. Build. Environ. 204, 108128. doi:10.1016/j.buildenv.2021.108128
Yang, J., Dong, J., Xiao, X., Dai, J., Wu, C., Xia, J., et al. (2019). Divergent shifts in peak photosynthesis timing of temperate and alpine grasslands in China. Remote Sens. Environ. 233, 111395. doi:10.1016/j.rse.2019.111395
Yu, Z., Yao, Y., Yang, G., Wang, X., and Vejre, H. (2019). Spatiotemporal patterns and characteristics of remotely sensed region heat islands during the rapid urbanization (1995–2015) of Southern China. Sci. Total Environ. 674, 242–254. doi:10.1016/j.scitotenv.2019.04.088
Yu, W., Shi, J., Fang, Y., Xiang, A., Li, X., Hu, C., et al. (2022). Exploration of urbanization characteristics and their effect on the urban thermal environment in Chengdu, China. Build. Environ. 219, 109150. doi:10.1016/j.buildenv.2022.109150
Zakšek, K., and Oštir, K. (2012). Downscaling land surface temperature for urban heat island diurnal cycle analysis. Remote Sens. Environ. 117, 114–124. doi:10.1016/j.rse.2011.05.027
Zhang, L., Hou, G., and Li, F. (2020). Dynamics of landscape pattern and connectivity of wetlands in western Jilin Province, China. Environ. Dev. Sustain 22, 2517–2528. doi:10.1007/s10668-018-00306-z
Zhao, C., Jensen, J., Weng, Q., and Weaver, R. (2018). A geographically weighted regression analysis of the underlying factors related to the surface urban heat island phenomenon. Remote Sens. 10, 1428. doi:10.3390/rs10091428
Zhao, Z., Shen, L., Li, L., Wang, H., and He, B.-J. (2020). Local climate zone classification scheme can also indicate local-scale urban ventilation performance: an evidence-based study. Atmosphere 11, 776. doi:10.3390/atmos11080776
Zhao, Y., Wu, Q., Wei, P., Zhao, H., Zhang, X., and Pang, C. (2022). Explore the mitigation mechanism of urban thermal environment by integrating geographic detector and standard deviation ellipse (SDE). Remote Sens. 14, 3411. doi:10.3390/rs14143411
Zhe, L. I., Shengbin, C., and Zhiyang, C. (2022). Spatial scale dependence between land surface temperature and land use types: A case study of Chengdu city. Ecol. Environ. 31, 999. doi:10.16258/j.cnki.1674-5906.2022.05.015
Zheng, X., Fu, M. C., and Yao, C. (2010). Landscape pattern spatial analysis technology and its application. Beijing, China: Science press.
Zhigang, C., Xinyue, Y., Chen, S., and Yajin, X. (2016). The trend of summer urban heat island effect and its relationship with urban development in Chengdu. Adv. Clim. Change Res. 12, 322. doi:10.12006/j.issn.1673-1719.2015.176
Zhou, D., Zhao, S., Liu, S., Zhang, L., and Zhu, C. (2014). Surface urban heat island in China’s 32 major cities: spatial patterns and drivers. Remote Sens. Environ. 152, 51–61. doi:10.1016/j.rse.2014.05.017
Zhou, D., Zhang, L., Li, D., Huang, D., and Zhu, C. (2016). Climate–vegetation control on the diurnal and seasonal variations of surface urban heat islands in China. Environ. Res. Lett. 11, 074009. doi:10.1088/1748-9326/11/7/074009
Keywords: Chengdu metropolitan area, land surface temperature (LST), urban heat island (UHI), correlation, multiple linear regression
Citation: Hu C, Huang G and Wang Z (2023) Exploring the seasonal relationship between spatial and temporal features of land surface temperature and its potential drivers: the case of Chengdu metropolitan area, China. Front. Earth Sci. 11:1226795. doi: 10.3389/feart.2023.1226795
Received: 22 May 2023; Accepted: 21 August 2023;
Published: 31 August 2023.
Edited by:
Yonghao Xu, Linköping University, SwedenReviewed by:
Zhichao Wang, Beijing Forestry University, ChinaKlemen Zakšek, University of Hamburg, Germany
Copyright © 2023 Hu, Huang and Wang. This is an open-access article distributed under the terms of the Creative Commons Attribution License (CC BY). The use, distribution or reproduction in other forums is permitted, provided the original author(s) and the copyright owner(s) are credited and that the original publication in this journal is cited, in accordance with accepted academic practice. No use, distribution or reproduction is permitted which does not comply with these terms.
*Correspondence: Zhiyong Wang, wangzhiyong@hust.edu.cn
†These authors have contributed equally to this work