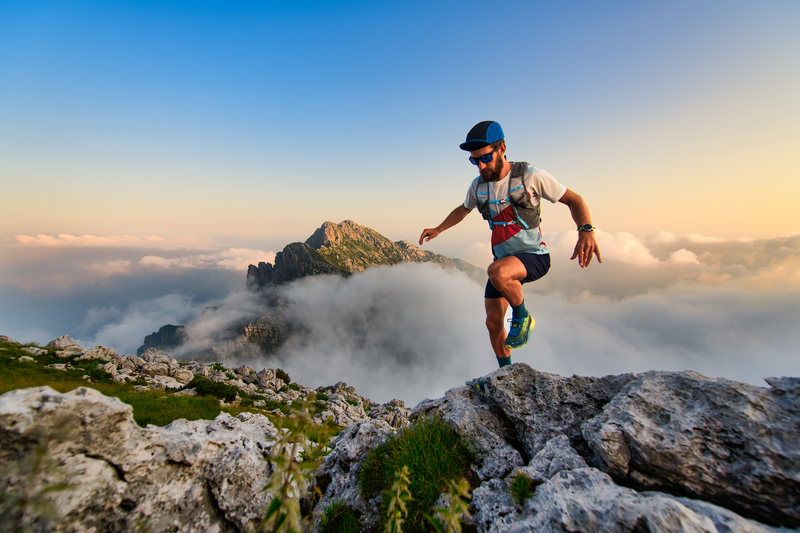
95% of researchers rate our articles as excellent or good
Learn more about the work of our research integrity team to safeguard the quality of each article we publish.
Find out more
EDITORIAL article
Front. Earth Sci. , 17 May 2023
Sec. Atmospheric Science
Volume 11 - 2023 | https://doi.org/10.3389/feart.2023.1212045
This article is part of the Research Topic Advanced Technologies in Remote Sensing of Aerosols and Trace Gases View all 8 articles
Editorial on the Research Topic
Advanced technologies in remote sensing of aerosols and trace gases
The seven papers in this Research Topic span a range of satellite remote-sensing applications, from characterizing near-surface aerosol pollution and SO2 concentrations (Qu et al.; Xu et al., respectively), to atmospheric column methane and cloud measurement (Karoff and Vara-Vela; Jiang et al., respectively), to the stratospheric aerosol distribution over the over Xinjiang region of China (Li and Tang). In these efforts to advance the field, common threads are the use of various machine learning methods to extract patterns from vast amounts of satellite data, and in many cases, the integration of multiple datasets, from different sources, sometimes including models, to provide the constraints required to obtain meaningful geophysical quantities.
The articles in this special issue address challenging problems, and the work presented here represents a sampling of a great many such efforts performed by the wider community of Earth remote-sensing scientists. For example, there is a vast literature documenting efforts to obtain surface-level information from space-based passive remote sensing, which generally entails many assumptions, and external constraints are usually required. Qu et al. aim to improve long-range (∼40-day) air quality forecasting by combining total-column aerosol optical depth (AOD) from NASA’s MODerate resolution Imaging Spectroradiometer (MODIS) instrument with local temperature, relative humidity, wind speed, and pressure data from a reanalysis model, plus results from a different model providing historical, sub-seasonal-to-seasonal, larger-scale meteorology. Similarly, to fill spatial and temporal gaps in ground-level SO2 concentration, Xu et al. integrate 1800 surface-station data records with meteorological data from a reanalysis model, and hourly radiance observations from the Himawari-8 geostationary satellite. To detect airborne dust over arid and semi-arid source regions in passive, multi-spectral remote-sensing observations from the MODIS instrument, with the possible confounding presence of cloud, Qu et al. (2023b) create a map of surface reflectance under dust-free conditions. create a map of surface reflectance under dust-free conditions. Radiance differences between the map and individual observations are then used to identify airborne dust. For pattern recognition and/or the integration of information from multiple sources in these data-rich studies, all three enlist machine learning techniques.
A fourth study (Han et al.) uses data from NASA’s Multi-angle Imaging SpectroRadiometer (MISR) instrument to simultaneously retrieve surface reflectance and AOD over Case II waters, which include inland lakes and coastal regions, of particular interest, as these areas tend to support high biological productivity.
One persistent issue for satellite remote sensing of aerosols as well as surfaces is the screening of clouds. Many multi-spectral techniques have been proposed over the years for cloud detection, and Jiang et al. offer an example. They calculate the three most significant principal components in the Chinese Orbita hyperspectral satellite’s (OHS) 32-spectral-channel imagery, and train an aggregate of machine-learning models to detect cloud in the scenes, using a transfer technique that applies a second model, previously validated for cloud detection.
Another, traditional application of satellite spectral remote sensing is the mapping of trace gases in the atmosphere. Karoff and Vara-Vela aim to detect methane sources from spectral data in the ultraviolet obtained by the Tropospheric Monitoring Instrument (TropOMI) aboard ESA’s Sentinel-5 satellite. Here again, ancillary data from other sources, in this case land-cover-type maps from MODIS and the Sentinel World Land Cover data, were needed to identify the nature of the underlying surfaces. Machine learning was applied for error-correcting the methane retrievals. The conclusions include the surprising result that although high methane amount was retrieved over croplands and lower values appeared over shrublands, unexpectedly low values were found over wetlands as well. Further work is indicated, to determine whether this result is robust, or whether there is an issue with the retrieval, e.g., due to low surface albedo over wetlands.
A final application in this Research Topic is the use of CALIPSO space-based lidar to map stratospheric aerosol over Xinjiang, China. This is an especially difficult application; based primarily on limb-sounding observations, AOD integrated over the stratosphere is extremely low, typically ∼0.01 at mid-visible wavelengths, except after major volcanic eruptions or pyro-cumulonimbus events. From nighttime CALIPSO soundings (when signal/noise is highest), Li and Tang conclude that stratospheric aerosol amounts are higher in spring and summer than in autumn and winter, and that the aerosol signal tends to diminish with elevation within the stratosphere. They aggregate data seasonally, over 11 years, to obtain statistical significance in these results.
As the applications of satellite aerosol remote sensing presented in this Research Topic are not yet very mature, each paper calls for additional work to further explore the strengths and limitations of the many available techniques.
The author confirms being the sole contributor of this work and has approved it for publication.
The author declares that the research was conducted in the absence of any commercial or financial relationships that could be construed as a potential conflict of interest.
All claims expressed in this article are solely those of the authors and do not necessarily represent those of their affiliated organizations, or those of the publisher, the editors and the reviewers. Any product that may be evaluated in this article, or claim that may be made by its manufacturer, is not guaranteed or endorsed by the publisher.
Keywords: aerosol remote sensing, satellite aerosol retrieval, advanced remote sensing techniques, machine learning for remote sensing, satellite aerosol remote-sensing applications
Citation: Kahn RA (2023) Editorial: Advanced technologies in remote sensing of aerosols and trace gases. Front. Earth Sci. 11:1212045. doi: 10.3389/feart.2023.1212045
Received: 25 April 2023; Accepted: 05 May 2023;
Published: 17 May 2023.
Edited and reviewed by:
Yuqing Wang, University of Hawaii at Manoa, United StatesCopyright © 2023 Kahn. This is an open-access article distributed under the terms of the Creative Commons Attribution License (CC BY). The use, distribution or reproduction in other forums is permitted, provided the original author(s) and the copyright owner(s) are credited and that the original publication in this journal is cited, in accordance with accepted academic practice. No use, distribution or reproduction is permitted which does not comply with these terms.
*Correspondence: Ralph A. Kahn, cmFscGgua2FobkBuYXNhLmdvdg==
Disclaimer: All claims expressed in this article are solely those of the authors and do not necessarily represent those of their affiliated organizations, or those of the publisher, the editors and the reviewers. Any product that may be evaluated in this article or claim that may be made by its manufacturer is not guaranteed or endorsed by the publisher.
Research integrity at Frontiers
Learn more about the work of our research integrity team to safeguard the quality of each article we publish.