- Department of Physics, University of Toronto, Toronto, ON, Canada
We have developed a physics ensemble of Weather Research and Forecasting (WRF) model simulations for the Middle East, Mediterranean and North Africa (MEMNA) regions. These simulations use different configurations for the cumulus, microphysics, surface layer, planetary boundary layer, and land surface schemes and are forced by the Community Earth System Model (CESM) General Circulation Model for the historical period 1979–1993. We have also created a complementary ensemble in which the WRF model is fully-coupled to the Regional Ocean Modelling System (ROMS) that simulates the dynamics of the entire Mediterranean Sea. Analysis of our ensembles reveals that the simulated precipitation and near surface temperature (T2) fields in WRF are largely influenced by the cumulus and the land surface schemes during the summer and winter seasons, respectively. The coupling of Weather Research and Forecasting to Regional Ocean Modelling System yields Mediterranean sea surface temperatures that are directly correlated with T2 and have higher spatial resolution than the global model. Meanwhile no significant difference is found between the atmospheric fields from the coupled and uncoupled runs because the Community Earth System Model sea surface temperatures over the Mediterranean, that are used for surface forcing in the uncoupled runs, are already in close agreement with both Regional Ocean Modelling System and observations. We conclude that our high-resolution coupled atmosphere-ocean modelling system is capable of producing climate data of good quality, and we identify those combinations of physics schemes that result in an acceptable level of bias that facilitates their use in future studies.
1 Introduction
The Middle East and the regions surrounding the Mediterranean Sea (displayed in Figure 1 for its location and in Supplementary Figure S1 for its topography) are one of the most densely populated regions on Earth, and contain several settlements that have beencontinuously inhabited since their establishment thousands of years ago. The large spatial extent of the region spans several climate zones and the way in which the characteristics of regional climates have evolved over the modern instrumental period is reasonably well understood from lengthy and detailed observational records (Malanotte-Rizzoli and Hecht, 1988; Vilibić et al., 2019). In order to study the climate over this region for future or past time periods, regional and global climate models are used.
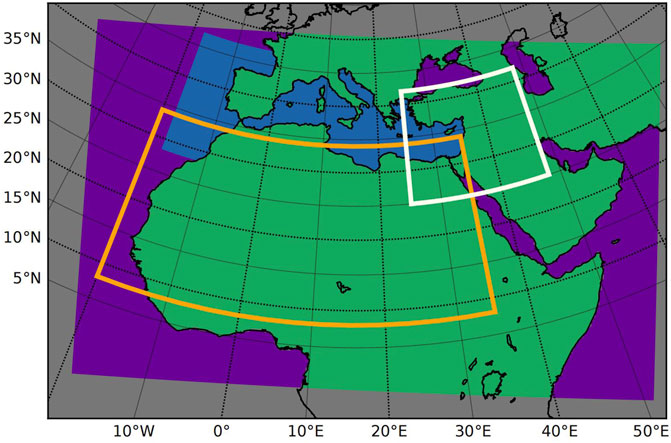
FIGURE 1. The WRF and ROMS regional domains employed in this study. The colored region shows the WRF domain and the blue region shows the ROMS domain that is used when WRF and ROMS are run in coupled mode. Oceanic regions within WRF domain where CESM SST forcing is applied is shown in purple. The white and orange boxes show the Middle East and North Africa regions respectively.
The current generation of state-of-the-art Global Climate Models (GCMs) are very capable of simulating the Earth’s climate as a whole for past, present and future periods and thereby contribute to our understanding of the large-scale dynamics of the climate system and how they have, or are expected to evolve (Taylor et al., 2012; IPCC, 2013; Eyring et al., 2016; Otto-Bliesner et al., 2017; IPCC, 2021). However, there is growing interest in studying regional climate features that are heavily influenced by local geographical features such as mountains, lakes, and coasts. Since these features have small spatial scales, they are not captured by the coarse resolution global climate models and require one to instead use high-resolution variants, known as Regional Climate Models (RCMs), that are configured to simulate a small region of the Earth. An important initiative in the regional climate modelling community is CORDEX (COordinated Regional Downscaling EXperiment, Giorgi et al. (2009)) which describes common experiment protocols for selected regions of interest. In particular our region of interest is covered by the EURO-CORDEX (Jacob et al., 2020) over Europe1, Med-CORDEX (Ruti et al., 2016) over Mediterranean Sea2 and MENA-CORDEX over Middle-East-North-Africa region3. A great number of RCM studies have been performed, both within and outside the context of CORDEX, that have contributed to our understanding of climate over the region and to our understanding of the capabilities and limits of regional climate models in effectively reproducing the observed climate. For example, over Europe the EURO-CORDEX RCM performance is examined by (Kotlarski et al., 2014; García-Díez et al., 2015), and internal variability of RCM over Europe is discussed by (Ho-Hagemann et al., 2020; Lavin-Gullon et al., 2021; 2022). For the MENA region Dell’Aquila et al. (2018) examined the capability of RCMs in general to reproduce the decadal variations in precipitation and surface air temperature over the Mediterranean by comparing results from 16 different RCMs. Zittis and Hadjinicolaou (2017) examined the surface air temperature anomalies over the Middle East and North Africa regions with respect to the choice of radiation scheme employed within a customized version of the Weather Research and Forecasting (WRF) model, and the same version of WRF model has its land surface scheme performance examined in Constantinidou et al. (2020) over the same MENA region. Romera et al. (2015) performed a comparison of the capabilities of 4 RCMs at reconstructing the observed precipitation over North Africa. Results from these and other studies demonstrated that climate over the Mediterranean and Middle East regions can be simulated by regional climate models with high accuracy.
An important regional feature that cannot be ignored while simulating the climate for the Mediterranean region is the Mediterranean Sea. It contains a huge volume of water that regulates the surrounding surface air temperature, and the thermal regulation effect is further enhanced via heat transport through its various circulations (Pinardi et al., 2019). Fully resolving the impact of the Mediterranean sea on the local climate requires a dynamical regional ocean model that is coupled to the regional atmospheric model. Several studies have taken this coupled regional modelling approach over the Mediterranean to study either short term weather events (Berthou et al., 2015; Ricchi et al., 2017), or long term climatologies (Sevault et al., 2014; Katsafados et al., 2016; Turuncoglu and Sannino, 2017; Akhtar et al., 2018). All of these studies concluded that employing active ocean coupling improved overall model performance, with the exception of Sevault et al. (2014) who did not explicitly note any improvements from ocean coupling, but such improvements were nevertheless evident from their results.
While climate modelling studies for the regions surrounding the Mediterranean have looked at the present and the future periods (studies that examined future climate change signal over the Mediterranean includes Constantinidou et al. (2019); Zittis et al. (2019); Parras-Berrocal et al. (2020) and many others), the Mid-Holocene (about 9,000 ∼6,000 years before present) is also a time period of interest for this region. The reason for such interest is that some of the earliest human permanent settlements on the planet have been discovered in the Levant and the Mesopotamia. These settlements have been dated to the Mid-Holocene period, around 6,000 years before present day (BP). The discovery of these settlements have given the Middle East region the appellation ’cradle of civilization’, and the founding of these settlements were undoubtedly influenced by the local climate during the Mid-Holocene period (Robinson et al., 2006). Reconstructions of Mid-Holocene climate from various geological proxies show that there was more precipitation than today over North Africa and Middle East during the Mid-Holocene period (Finné et al., 2011; Mauri et al., 2015; Peyron et al., 2017; Andrews et al., 2020). However, the availability of proxies is limited and they provide a very sparse picture of the climate of the time, and therefore climate models are necessary to develop a most comprehensive understanding of the Mid-Holocene climate (Timm et al., 2010; Brayshaw et al., 2011; Finné et al., 2011; Roberts et al., 2011; Peyron et al., 2017). One of the more recent efforts to model the climate of this time period was by Chandan and Peltier (2020) in which a global climate model configured with appropriate solar insolation, atmospheric green house gas concentrations and highly-accurate land surface conditions is used to simulate a climate that matched the available geological proxies quite closely. It has been recently shown, for the case of Southeast Asia, that further improvement on the agreement between climate proxies for the Mid-Holocene and GCM simulations can be achieved by downscaling the results of the GCM using an appropriate setup high-resolution RCM for the region of interest (Huo et al., 2021).
Before applying RCM model to simulate Mid-Holocene climate for the Middle East, Mediterranean and North Africa (MEMNA) region, one first needs to determine the configurations of the RCM that are suitable for this region by comparing the results of each configuration with the observed climatology over the recent instrumental period. The objectives of this study are therefore two-fold: firstly, we wish to validate our dynamical downscaling pipeline, that has been previously applied successfully to several regions of the globe (Gula and Peltier, 2012; Erler et al., 2015; Huo and Peltier, 2019), and now includes an online ocean coupling component for the MEMNA region, and secondly, we want to optimize the selection of physics schemes for this region by evaluating the performance of the model in simulating the present-day climate, and gain insights on the impacts of selected physics schemes on the RCM results.
The structure of this paper is as follows: Section 2 describes the experimental setup, climate models and the observational datasets used in this study. Results from our RCM simulations are presented in Section 3 where we first discuss results from the atmosphere only simulations (Section 3.1.1) so as to isolate the effects of the choice of physics schemes in the atmospheric model and then discuss coupled atmosphere-ocean simulations in Section 3.1.2 to verify the coupling process and its effect. Section 4 concludes the paper with a general discussion of our findings.
2 Experiment design, model, and validation datasets
2.1 Experimental design
The results presented here are based on an analysis of four high-resolution physics ensembles for the MEMNA region, each containing 5 ensemble members. The first two ensembles consist of atmosphere-only simulations that were obtained with the Weather Research and Forecasting (WRF) model, version 4.1.2, with the Advanced Research WRF (ARW) dynamical core (Skamarock et al., 2019). These two ensembles differ only with regards to the chosen land surface scheme; the ensemble set ‘N’ uses the Unified Noah land surface scheme (Chen and Dudhia, 2001) while the ensemble set ‘L’ uses the Community Land Model (CLM) Version 4 (Lawrence et al., 2011). Each ensemble is comprised of five members with different combinations of microphysics scheme {New Thompson (Thompson et al., 2008) or WSM-6 (Hong and Lim, 2006)}, cumulus scheme {Grell-Freitas (GF) (Grell and Freitas, 2014) or Tiedtke (Tiedtke, 1989)}, planetary boundary layer scheme {Mellor-Yamada-Nakanishi-Niino (MYNN) Level 2.5 (Nakanishi and Niino, 2006; 2009) or Yonsei University Scheme (YSU) (Hong et al., 2006)} and surface layer scheme {MYNN or Revised MM5 (Jiménez et al., 2012)}. The names of the ensemble members and the physics schemes they are configured with are documented in Table 1. All simulations use the RRTMG shortwave and longwave radiation scheme (Iacono et al., 2008) with aerosol input based on Tegen et al. (1997). The selection of schemes that are investigated in this study is motivated by the findings of other studies with the WRF-ARW model over our region of interest (Zittis et al., 2014; Li et al., 2015; Zittis and Hadjinicolaou, 2017; Constantinidou et al., 2020; Glotfelty et al., 2021), and studies over other regions of the Earth (Erler et al., 2015; Huo and Peltier, 2019; Xie et al., 2021).
The other two ensembles are coupled atmosphere-ocean ensembles for which the WRF model, while using the same series of physics schemes as the uncoupled ensembles, has been coupled to the Regional Ocean Modelling System (ROMS; Shchepetkin and McWilliams (2005)). ROMS is a high-resolution, free-surface, regional ocean model that incorporates a terrain-following coordinate. The version of ROMS used is Roms_Agrif v3.1.1 (Debreu et al., 2012). The coupler used to exchange data between WRF and ROMS during model runtime is OASIS3-MCT version 3.0 (Craig et al., 2017). Our implementation of the atmosphere-ocean coupling is based on the setup used in the Coastal and Regional Ocean COmmunity model (CROCO, Auclair et al. (2018)), with modifications made to both the dynamical downscaling pipeline and the CROCO software to facilitate our unique experimental setup. Since WRF and ROMS domains are not identical, the OASIS coupler interpolates data between WRF and ROMS grids during data exchange using a 1st degree conservative mapping method. The time frequency for data exchange between the coupled models is set to 1 h. In comparison, timesteps for integration in WRF are 120s, and in rare case where a model instability is encountered, they are reduced to 60s for short periods. Similarly, integration steps in ROMS are either 240s or 120s.
All simulations are performed for the historical period 1979–1993. The first 5 years are used as the spinup period for both uncoupled and coupled simulations. The suitability of this spinup duration has been examined via a test run in which the ocean initial condition were taken from the ROMS final state at the end of a 15 years coupled simulation. SST and atmospheric fields from this test run differ very little from those obtained from the standard coupled simulation, indicating that the 5 years spinup in the standard coupled simulation is sufficient.
We use the following definitions to discuss seasonal results: spring (March, April, May), summer (June, July, August) fall (September, October, November) and winter (December, January, February). Year to year variations in the WRF regional model with the same dynamical downscaling pipeline has been discussed in Erler et al. (2015). Meanwhile, interannual variations within RCMs is discussed in (Zittis et al., 2014) over MENA-CORDEX domain that is very similar to the domain in this study, and in (Ho-Hagemann et al., 2020; Lavin-Gullon et al., 2021; 2022) over an European domain that partially overlaps with our domain. (also see (Deser et al., 2014; Peings et al., 2017) for a general discussion on the internal variability of climate models).
2.2 RCM model configuration and forcing data
The atmospheric dynamical downscaling experiments presented in this paper are based on the atmosphere-only dynamical downscaling pipeline described in Erler (2015), and used previously to perform downscaling experiments for the regions surrounding the Great Lakes of North America (Gula and Peltier, 2012; d’Orgeville et al., 2014; Xie et al., 2021), Western Canada region (Erler et al., 2015; Erler and Peltier, 2016; 2017), and the Indian sub-continent and South-east Asia regions (Huo and Peltier, 2019; 2020; 2021; Huo et al., 2021; 2022). This dynamical downscaling pipeline has also been extended here for the MEMNA region by coupling a regional oceanic model to the regional atmospheric model. Technical details of this coupling, as well as various model and dataset used to conduct the experiment and analyze its results, are described in the sections below.
The regional domain of the WRF model is shown in Figure 1. WRF has been configured with a single domain with Lambert conformal projection at 30 km resolution covering North Africa, the Middle East, and the Mediterranean Sea with its surrounding land masses that includes the southern parts of Europe. The resulting domain is quite close to the Middle East North Africa domain of the CORDEX project (Giorgi et al., 2009), with the exact boundaries adjusted to best utilize the parallelization capability of WRF model. Terrain topography of the WRF domain is displayed in Supplementary Figure S1. Two regions within this domain are of particular interest in this paper: the Middle East within 25 ∼ 48°E, 25 ∼ 42°N, and North Africa within 20°W ∼ 35°E, 8 ∼ 34°N. Both regions are indicated on Figure 1 and have ocean grids masked out. The Middle East region is important because the earliest human permanent settlements anywhere on the planet have been discovered in this region and dated to the mid-Holocene. There is considerable interest in the anthropological and archaeological communities to understand the factors that would have facilitated the transition of neolithic humans from a hunter-gatherer lifestyle to a sedentary lifestyle, with the local climate being one of the factors. The North Africa region is important because of the existence of Green Sahara which resulted in a northward migration of flora and fauna into regions that are today parched and uninhabited (some example of archaeological evidence are discussed in Dunne et al. (2012); Manning and Timpson (2014), and discussion on the dynamics of Mid-Holocene climate with are Green Sahara can be seen in Robinson et al. (2006); Larrasoaña et al. (2013) and others). While this study is focused on validating the performance of the model for the present day climate, we will put a particular emphasis on how the models perform in these two regions because those ensemble members that perform well in these regions will be selected for modeling the mid-Holocene in a future study.
The ROMS model is configured as a single domain using latitude-longitude square grid with 0.1° resolution that covers all of the Mediterranean Sea and a small part of the Atlantic Ocean west of the Strait of Gibraltar (Figure 1). We note that the Black Sea, the Turkish Straits (the straits of Bosporus and Dardanelles), and the Sea of Marmara, are not included in the ROMS domain. The primary reason for this is that the Black Sea is not connected to the Mediterranean Sea in the CESM GCM run from which the GCM forcing is derived, and the regional domain needs to maintain consistency with the GCM setup.
Both the WRF atmospheric model and the ROMS oceanic model require boundary forcings and initial conditions that are, in this study, derived from the Community Earth System Model version 1 (Gent et al., 2011), which has been developed by the National Center for Atmospheric Research (NCAR) and contains submodels for all major components of the climate system. The version of GCM that provides data is the University of Toronto version of CCSM4 (Peltier and Vettoretti, 2014). CESM data used to drive the WRF model includes 6-hourly atmospheric temperature, wind, humidity and pressure fields, provided as atmospheric boundary forcings and monthly sea surface temperature (SST) field provided as the lower boundary condition over oceanic regions, as-well-as land surface temperature and assorted land variables that are used for the initialization of the WRF land component. In addition to boundary forcing, spectral nudging is also applied to the pressure, potential temperature and humidity fields in all ensemble members in order to preserve the large-scale circulation features of CESM in WRF. The effects of spectral nudging on regional climate modelling is discussed in Separovic et al. (2012); Alexandru et al. (2009), and in particular Omrani et al. (2015) discusses spectral nudging effects on simulating regional climate over Europe and Mediterranean. For ROMS, CESM monthly 4D ocean temperature and salinity fields are used as initial and boundary conditions. Preprocessing of CESM data for use by ROMS is done using modified community software packages that are available for ROMS (Penven et al., 2008) and CROCO. In coupled configuration, CESM SST is used as the lower boundary condition for WRF over oceanic regions that are not covered by our ROMS domain including Black Sea, Red Sea, and small parts of the Atlantic and Indian Oceans within the WRF domain. In uncoupled simulations, WRF takes prescribed CESM SST for all ocean surfaces. All input CESM data is available at 1° resolution.
In addition to CESM forced simulations, several uncoupled test runs of WRF were conducted using the ERA-I reanalysis product (Dee et al., 2011) as RCM forcing to verify the robustness of the model and our domain setup. These reanalysis forced test runs are not extensively discussed in this study because they do not enable us to make an effective comparison between coupled and uncoupled simulations, which is a focus of this study. This limitation arises because ERA-I does not have 4D ocean data with which to force our ocean model, while combining it with ocean forcing from other dataset would possibly introduce uncertainty from the fact that the atmospheric and oceanic datasets are not at equilibrium at the ocean surface. Such combined atmosphere-ocean reanalysis forcings were used in Sevault et al. (2014) and Akhtar et al. (2018), but these studies also employed long spinup periods to ensure equilibrium between the atmosphere and ocean is reached at the start of their simulation. Therefore, combined reanalysis forcing is not ideal for this study as the uncertainty from the disequilibrium at the ocean surface could interfere with effects from ocean model coupling. Our reanalysis runs are also use to support some conclusion drawn from CESM forced atmosphere only simulations.
2.3 Validation datasets
In order to validate the atmospheric fields from our simulations, the CRU dataset (Harris et al., 2013) is used as an observational reference dataset, and the ERA-Interim reanalysis product (Dee et al., 2011) is used as a reanalysis reference dataset. These two datsets have been widely used by other regional climate modeling studies (e.g., CRU is used in Zittis et al. (2014); Zittis and Hadjinicolaou (2017); Turuncoglu and Sannino (2017); Zittis et al. (2019); Constantinidou et al. (2020), and ERA-Interim is used either as a forcing dataset or as reference dataset in (Diaz et al., 2015; Katragkou et al., 2015; Klein et al., 2015; Omrani et al., 2015; Alaka Jr and Maloney, 2017; Constantinidou et al., 2019; Achugbu et al., 2020; Lavin-Gullon et al., 2021).) In this study we will primarily use CRU as reference for atmospheric fields, namely, precipitation and near surface temperature (T2), while ERA-I reanalysis is employed to understand possible uncertainties in these reference data. For oceanic fields, the World Ocean Atlas (WOA) observational dataset (Boyer et al., 2019) is used as reference to provide sea surface temperature (SST) and sea surface salinity (SSS) fields.
3 Results
In this section, the simulated regional climates for the Mediterranean, North Africa and Middle East are evaluated by comparison with our validation datasets. Results from the uncoupled atmospheric only runs are presented first, followed by results from atmosphere-ocean coupled runs.
3.1 Atmosphere-only downscaling
3.1.1 Regional climate overview
The summer average precipitation biases (throughout this paper, bias is defined as model minus observation) with respect to the CRU dataset over the land surface in the WRF domain are presented for the WRF ensemble members and the CESM GCM in Figure 2. It is clear that our WRF ensemble members spans a wide range of precipitation biases, from very dry to very wet, especially over the tropical convection region that is close to 10°N latitude during Northern hemisphere summer. The physics scheme that appears to be most relevant to the simulation of summer precipitation is the cumulus scheme: members that use the GF cumulus scheme (N2, N3, N5, L2, L3, L5) are wetter than those that uses the Tiedtke scheme (N1, N4, L1, L4). The strong influence of the cumulus scheme on the simulated precipitation field in WRF is consistent with results from other downscaling experiments for the MEMNA (Zittis et al., 2014), the EURO-CORDEX (Katragkou et al., 2015), Western Canada (Erler et al., 2015), North American Great Lakes (Xie et al., 2021) and South East Asia (Huo and Peltier, 2019) regions.
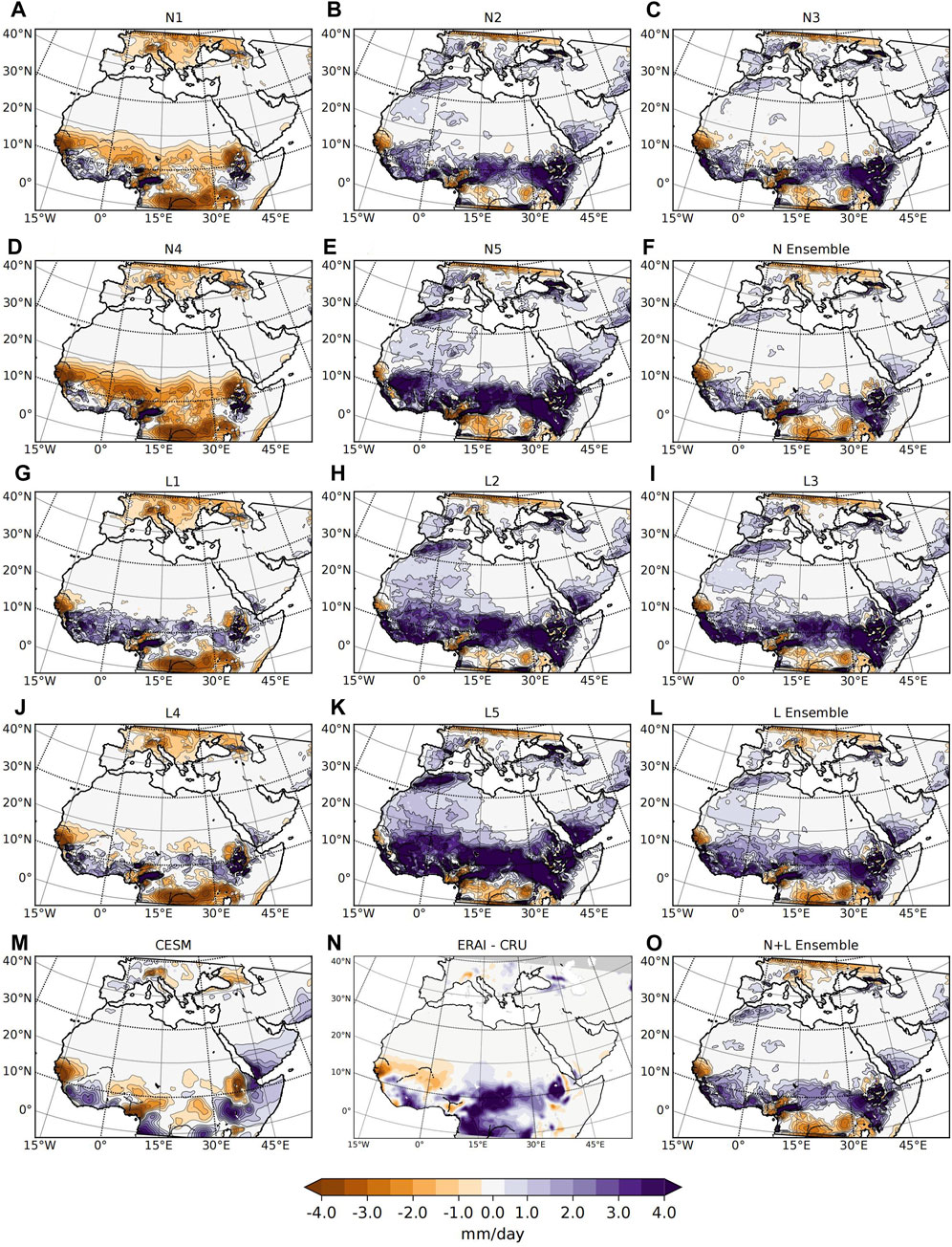
FIGURE 2. Summer precipitation anomalies compared to the CRU dataset for the N ensemble members (A–E), N ensemble average (F), L ensemble members (G–K), L ensemble average (L), full ensemble average (O) and CESM (M). Panel (N) shows the difference between the ERAI and CRU datasets.
Precipitation bias is also found to be influenced by the choice of the microphysics scheme and the land surface scheme: members using the New Thompson scheme (N1, N2, N5, L1, L2, L5) are slightly wetter than those using the WSM-6 scheme (N3, N4, A3, L4), and members using Noah-LSM (N ensemble) are dryer than those using CLM (L ensemble). While changes to either of these schemes does not influence precipitation as strongly as a change to the cumulus scheme, the impact is nonetheless clearly observable during the summer season. This finding is in contrast with Constantinidou et al. (2020), which did not find any significant changes to the annual precipitation field owing to changes to the land surface scheme. The reason we are able to observe a difference is partly because the physics ensemble employed in this study has been designed to highlight the difference from land surface schemes, and partly because analyzing seasonal data amplifies the difference signal since precipitation is concentrated during the summer season. The effect of the microphysics scheme on simulated precipitation is similar to that reported in Klein et al. (2015).
When comparing with CESM GCM results, it is clear that the CESM precipitation pattern is completely overwritten by WRF over the tropics. This is not surprising because the main source of precipitation over this region is from local convection, which is dominated by cumulus scheme employed in the model as discussed above. One exception to this independence of WRF pattern is over the western tip of Africa (Cap-Vert in Senegal) where all WRF members and CESM share a dry bias. This might have its source in the CESM simulated SSTs over the Atlantic and west Africa monsoon system that WRF would inherit through prescribed SST and spectral nudging respectively. A rather peculiar observation is that ensemble members that are wet biased over the tropical convection zone also exhibit a wet bias over Western Sahara and the Maghreb region, though this bias attenuates greatly in ensemble means.
The average summer near surface temperature (T2) biases over land for the WRF ensemble members and for CESM are presented in Figure 3. Here, the Noah-LSM ensemble displays distinctly different patterns of spatial bias compared to the CLM ensemble in the sense that the Noah-LSM ensemble members are cooler over the Middle East and North Africa north of 20° N. Since all ensemble members employs the RRTMG radiation scheme, and the bias exists between all pairs of ensemble members that differ only in the land surface scheme, this difference in T2 is likely caused not by variation in incoming radiation but by the differences in land-radiation interaction between the different land surface schemes. In particular, differences in the representation of bare soil and sandy surfaces between the two land surface schemes should be the primary cause as most of the region has little-to-no vegetation cover. In the equatorial and sub-Saharan regions south of 20° N, summer season tropical convection is an important driver of precipitation and therefore ensemble members that exhibit wet bias in Figure 2 are also associated with cold T2 bias whereas dry members have a warm T2 bias.
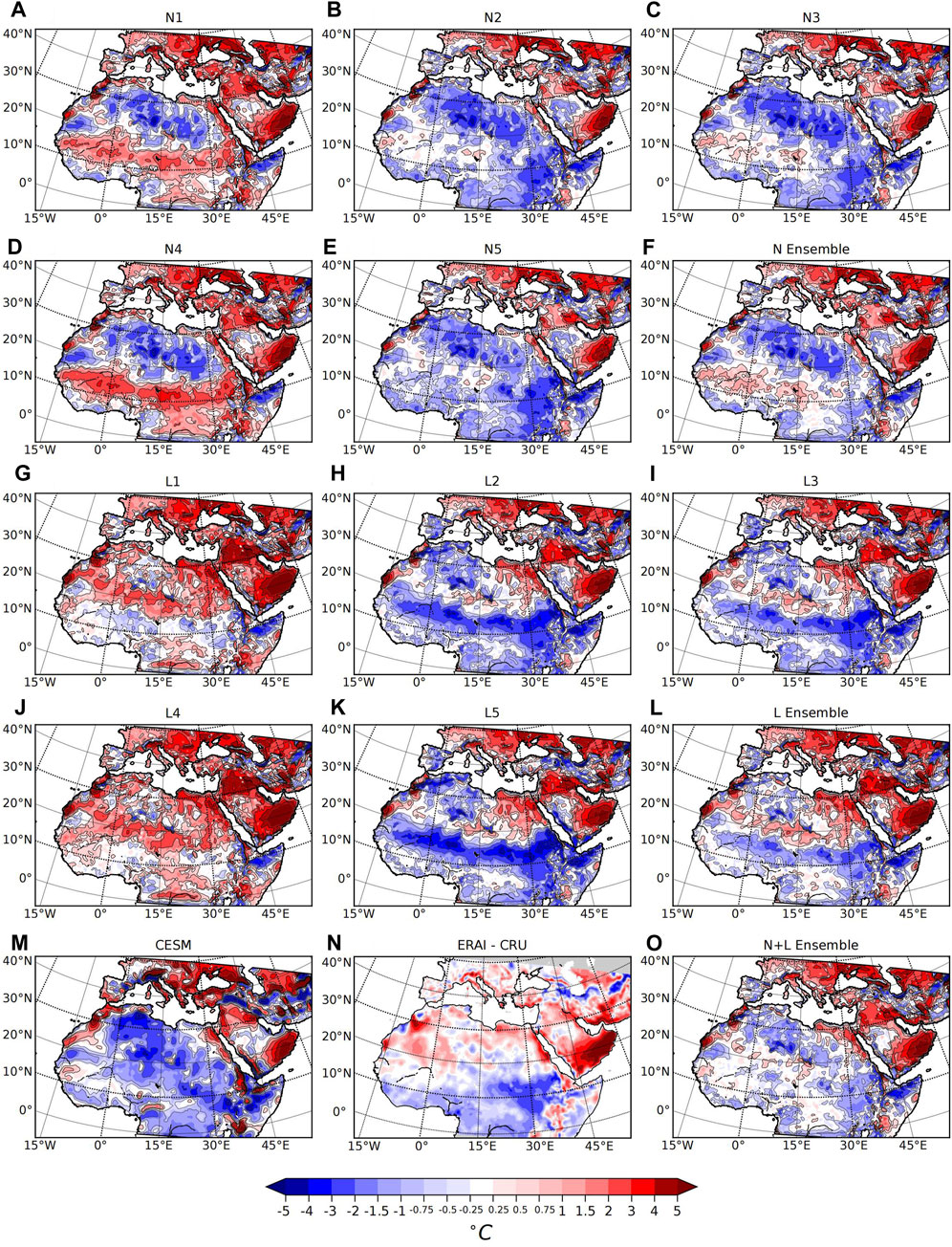
FIGURE 3. Similar to Figure 2 but for the summer temperature biases.
T2 differences between our two ensembles become more striking during winter (Figure 4). All simulations with Noah-LSM show a strong cold bias over the entirety of North Africa and the Arabian peninsula while the T2 fields in simulations with CLM are in much better agreement with the reference dataset. In contrast, differences in cumulus and microphysics schemes have much smaller impacts on the simulated winter temperatures. This is not very surprising because winter is the dry season in North Africa and therefore T2 is not strongly influenced by physics schemes involved in the parameterization of convection and cloud cover. Meanwhile, over the historical region of Mesopotamia the CLM ensemble is slightly warmer than reference whereas the Noah-LSM ensemble is closer to reference. These features of the simulated winter T2 biases are also evident in the means of the two ensembles (Figure 4F,l).
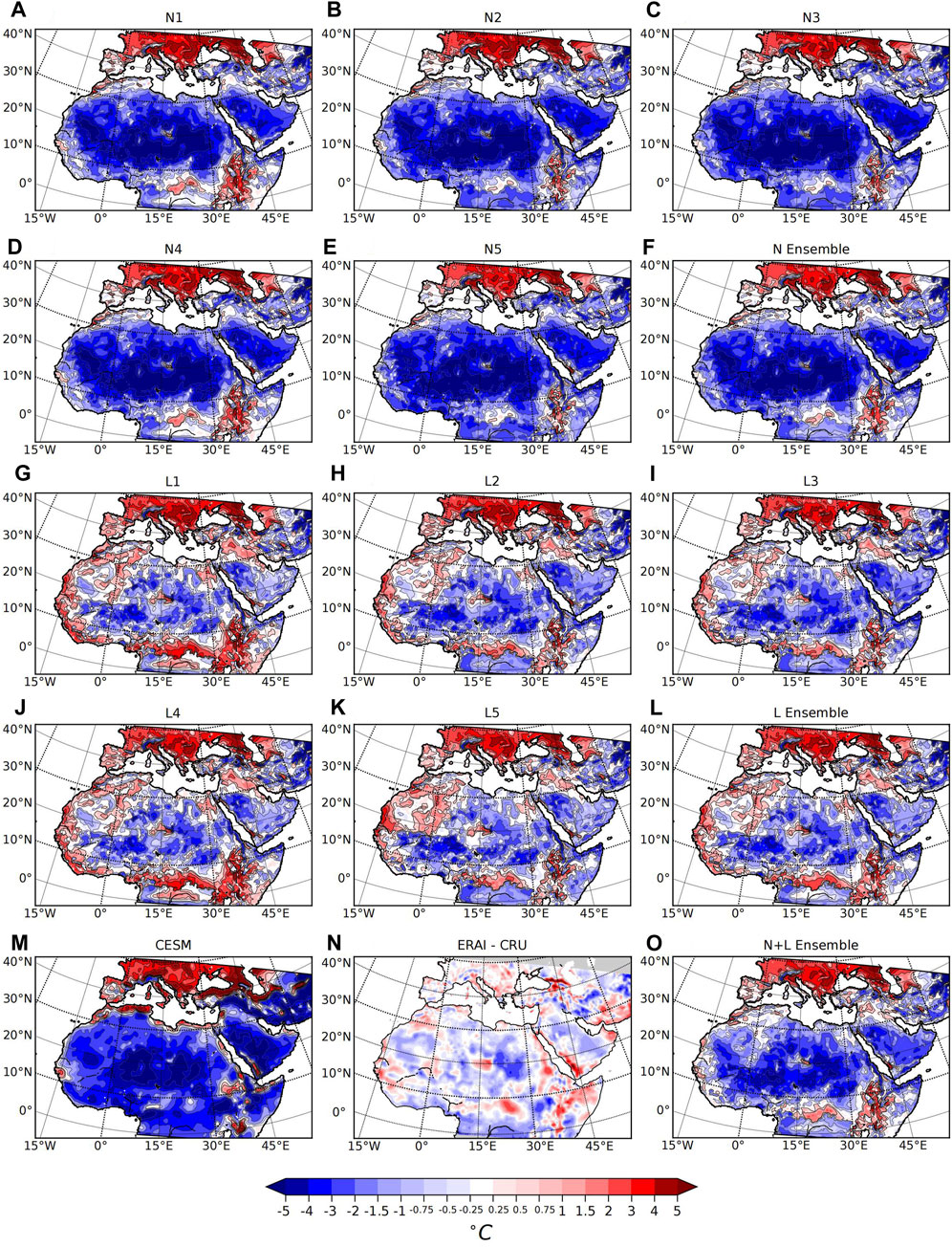
FIGURE 4. Similar to Figure 2 but for the winter temperature biases.
One may speculate that the WRF model’s performance with respect to the winter season T2 could be improved with a different radiation scheme. Zittis and Hadjinicolaou (2017) have shown that radiation schemes indeed strongly influence final temperature results. However, the authors also found that the RRTMG scheme is one of the better performing radiation scheme. Therefore, the real cause for the winter cold bias found in this study likely has to do with how other schemes collectively influence the net energy fluxes, rather than with the amount of radiative energy produced by the scheme. Glotfelty et al. (2021) demonstrated that for the land surface schemes included in WRF, changing land usage profile and modifying surface albedo variable both have noticeable impact on the simulated climate over parts of Africa that are south of the Sahara. The Sahara itself would not benefit from land usage profile changes as it is mostly barren desert in our simulations, but changes to the surface albedo, owing to different land surface schemes, might potentially improve winter season T2 performance.
Another point worth noting is that CESM displays a strong warm bias over Europe in winter that is largely inherited by all ensemble members. This is likely because the part of Europe in the regional domain is right next to the boundary, and westerly winds from the boundary might carry too much warm air from North Atlantic across continental Europe. This is in contrast to the case for North Africa where CESM simulates a strong cold bias, yet WRF either mitigates that to a large extent in the CLM ensemble or makes it worse in the Noah-LSM ensemble, demonstrating that WRF dynamics and parameterization schemes dominate away from the boundaries of the domain. It is worth mentioning here that the extension of region that inherits warm bias over Europe is likely aided by spectral nudging, as such bias is driven by westerly winds. (see Alexandru et al. (2009); Separovic et al. (2012) for a discussion on the effect of spectral nudging on regional climate modelling.).
While winter is a dry season over tropical and sub-tropical Africa in the northern hemisphere, it is the main rainy season along the coastal regions of the Mediterranean and in the regions surrounding the Black Sea and the Caspian Sea. It is found that in the winter season, WRF regional simulations largely exhibit wet biases over Anatolia and the coastal regions of the Black Sea and a dry bias over the eastern and southern coasts of the Mediterranean (Figure 5). Since this spatial pattern also exists in CESM, it is likely that CESM SSTs that are directly employed in these uncoupled atmosphere only runs contribute to these downscaled precipitation biases. Ensemble members that are relatively wet over the tropical convection region in summer also produce more precipitation in the winter season (N5, L2, L3 and L5 in particular). There is also significant terrain induced precipitation over the Alps, Carpathian, Caucasus, and Zagros Mountains in WRF results that are not in CESM, which is from the combined effects of increased spatial resolution in the regional model and increased moisture in the warm biased air.
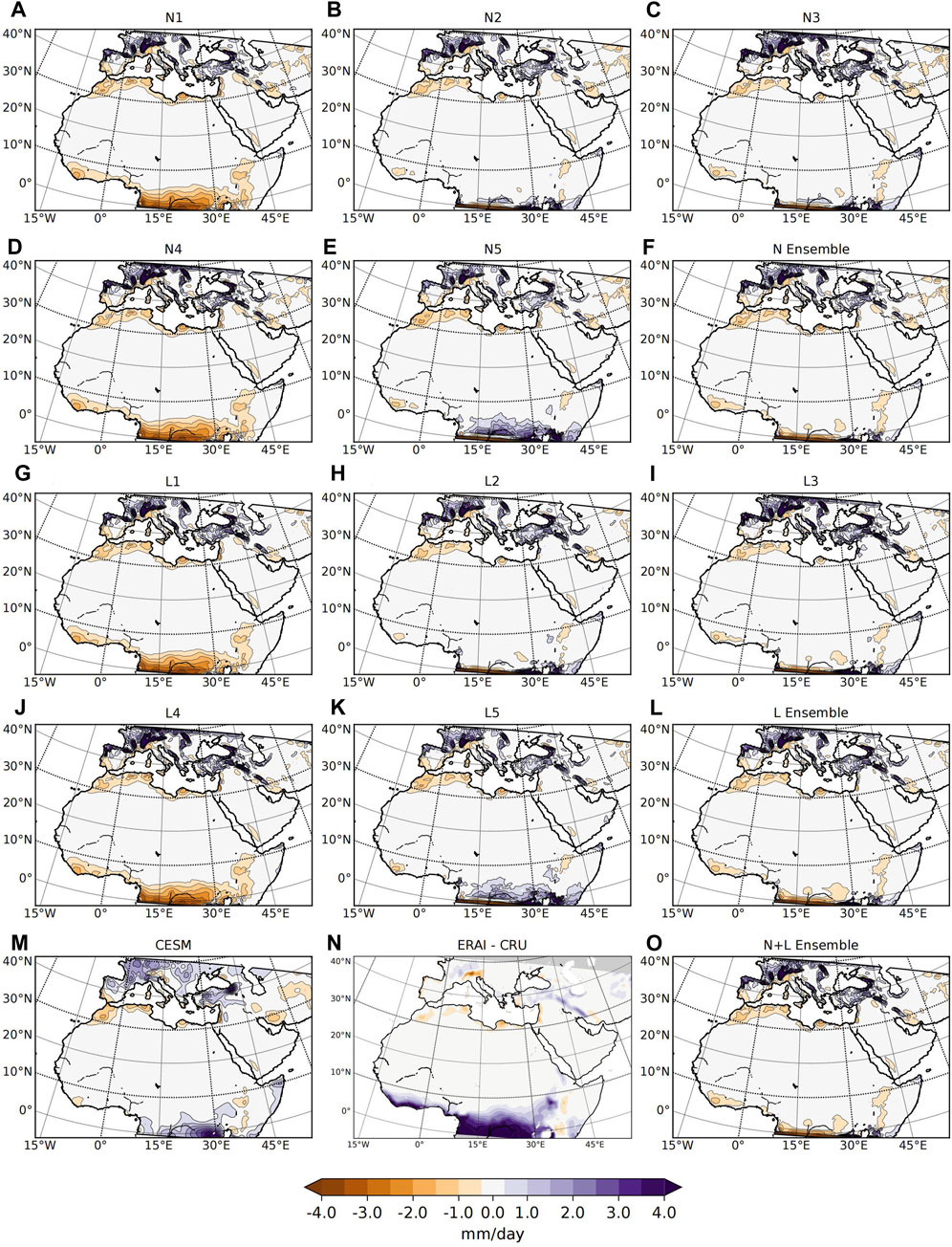
FIGURE 5. Similar to Figure 2 but for the winter precipitation biases.
Statistical means and standard deviations for the seasonal results presented above, as well as annually averages, are provided in Supplementary Table S1. Regional features discussed above such as variation in summer precipitation bias and the cold winter T2 in N ensemble are captured by these variables. Moreover, the annual statistical means calculated from ensemble averages are quite small; precipitation bias of the N ensemble average is 0.02 mm/day and T2 bias of the L ensemble average is 0.02°C. When cross comparing with results from other studies, a typical good simulations from a study would achieve an averaged precipitation bias
So far, we have assessed the performance of our simulations by comparing them to the CRU dataset. Meanwhile, since observational dataset is limited by the spatial and temporal coverage of data stations, using reanalysis dataset as an additional reference dataset would provide more insight to the results we obtained. A commonly used alternative is the ERA-Interim reanalysis. Here, we provide a brief comparison between ERA-Interim and CRU, which provides useful insights into the differences between the reference datasets themselves and what it means for the simulated biases discussed above. The comparison leads to three noteworthy observations; firstly, summer season precipitation in ERA-I shares the dry bias on the tip of West Africa and is notably wetter than CRU over the rest of the tropics (Figure 2), which means the ensemble mean might not be as wet biased as comparison with CRU data suggests and dry bias over west Africa might relate to lack of precise observational record over that region. Secondly, in the summer season the difference in T2 between ERA-I and CRU is quite similar to the mean T2 bias of the CLM ensemble (Figure 3), especially with regards to the cold region northwest of Ethiopia and the warm region over the southern part of the Arabian peninsula. As both CRU and ERA-I are approximations of the true climate state, these two observations suggest that the simulation of the summer climate by ensembles may be even better than that inferred from the preceding discussion. The third observation to note is that in contrast to summer, during winter the CRU and ERA-I T2 fields are quite close to each other. This means that winter season WRF model performance in our simulations over North Africa is similar regardless of the reference dataset used and that it needs improvement, which is partially achieved by switching the land surface scheme from Noah-LSM to CLM.
From the results presented in this study, it is possible to suggest some hypotheses on causality between WRF biases and WRF physics schemes that observed to have dominant effects. One example is that influence of cumulus scheme over summer precipitation bias can be attributed to difference between the stochastic method used in GF scheme and the mass-flux with convective available potential energy closure method used in Tiedtke scheme, as well as parameter settings within these schemes. Another example is that the main reason behind winter season T2 bias that explicitly differs between N and L ensemble is highly likely from surface albedo field that gets resolved differently under Noah-LSM and CLM schemes, with the former uses prescribed input and the latter computes surface albedo within the scheme. However, biases in the simulated precipitation and T2 fields will include compensation across processes. Some processes like microphysics scheme and planetary boundary layer scheme are observed in this study, while some other processes like radiation scheme (which is discussed in Zittis and Hadjinicolaou (2017)), and effects from clouds (discussed in Diaz et al. (2015), and also in Kotlarski et al. (2014); Chen et al. (2019; 2022) but over several different region of interest) are not fully explored by our physics ensemble. Therefore it is preferred to not exaggerate our findings on the influence of particular schemes, since the objective of this study is to optimize WRF’s performance from a general perspective instead of focusing on any particular scheme selection, in contrary to studies that are designed to focus on a scheme of interest such as Glotfelty et al. (2021) that focuses on land surface scheme’s impact on simulation results.
It is also worth noting that there are other sources of uncertainty in simulated regional climate results that are not fully addressed in this study. Two of these are biases inherited from GCM forcing and internal variability of RCM. Acquiring an in depth knowledge regarding these uncertainties would require additional dedicated experiments that cross-compare results from multi-RCM model under multi-GCM forcing (one example study that uses such a design is Zittis et al. (2019)). This has not been done here because that would move the focus of the study away from ocean coupling validation to RCM uncertainty (the latter has already been explored by many studies that have been referenced earlier in this paper). For the particular GCM-RCM combination employed in this study, RCM internal variability in large scale climate features is likely mitigated via averaging over a 10 year period and suppressed by the usage of spectral nudging method. The influence of spectral nudging is particularly strong for winter season T2 bias over continental Europe as discussed above. For regional, small scale features, influence of physics schemes would dominate over internal variability and boundary forcing uncertainty. This is particularly the case for North Africa region (except the western tip of Africa, as discussed earlier), over which cumulus schemes exert dominant influence over summer season tropical convection regions via resolving local convective activities, and land surface schemes dominate desert regions in the winter season through their influence on the interaction of the land surface with incoming radiation. Results from our ERA-I simulations (not shown here) also support the conclusion that the biases are mostly due to RCM and not boundary forcing. Therefore, analysis of the averaged climate results presented in this section lead us to conclude that selection of physics scheme is the primary cause of bias variation between ensemble members, with other sources of bias having visible but secondary influence on simulation results.
3.1.2 Climate over regions of interest
After presenting WRF physics ensemble results over all regional domain, we now focus on two key regions within our downscaling domain for further discussion. Firstly, for the North Africa region, Figure 6 shows the distributions of precipitation and T2 biases in the uncoupled WRF physics ensembles. It is readily evident from this plot that during the summer season, precipitation and T2 biases are related over the region such that ensemble members with higher simulated precipitation also have lower T2. This is to be expected, since higher precipitation leads to more surface moisture whose subsequent evaporation extracts energy from the land surface. The outlier data points in the precipitation box plot are located around the tropical convection zone, where precipitation rate depends largely on how local convection is resolved by the regional model. The winter temperature bias over North Africa, as described earlier, is highly dependent on the choice of land surface schemes: CESM and Noah-LSM ensemble members have cold biases of magnitudes
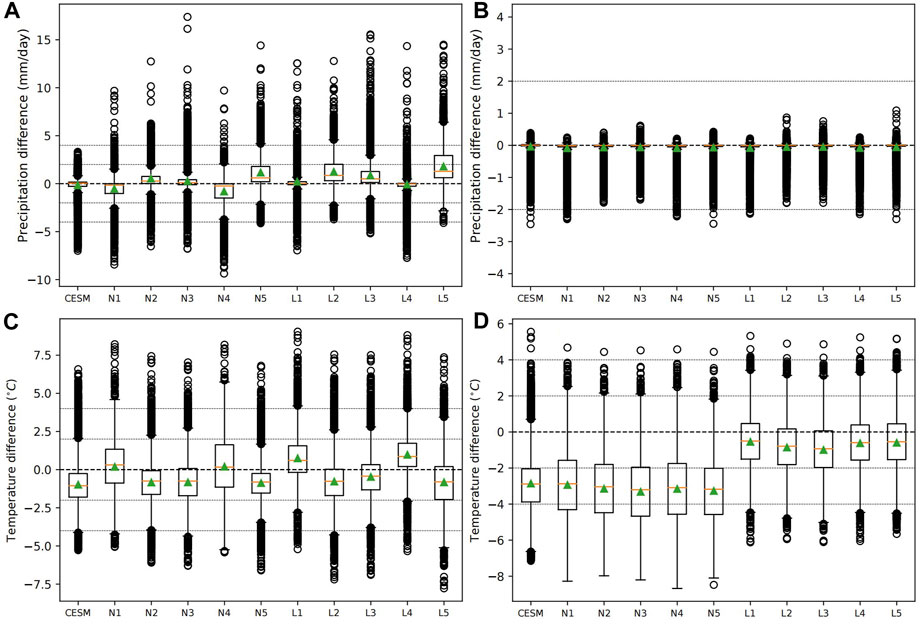
FIGURE 6. Box and whiskers plots for the distribution of precipitation and temperature anomalies, with respect to CRU, in the North Africa region for summer (A,C) and winter (B,D) seasons. Here Green triangles and orange solid lines represent mean and median values respectively. The North Africa region is shown in Figure 1.
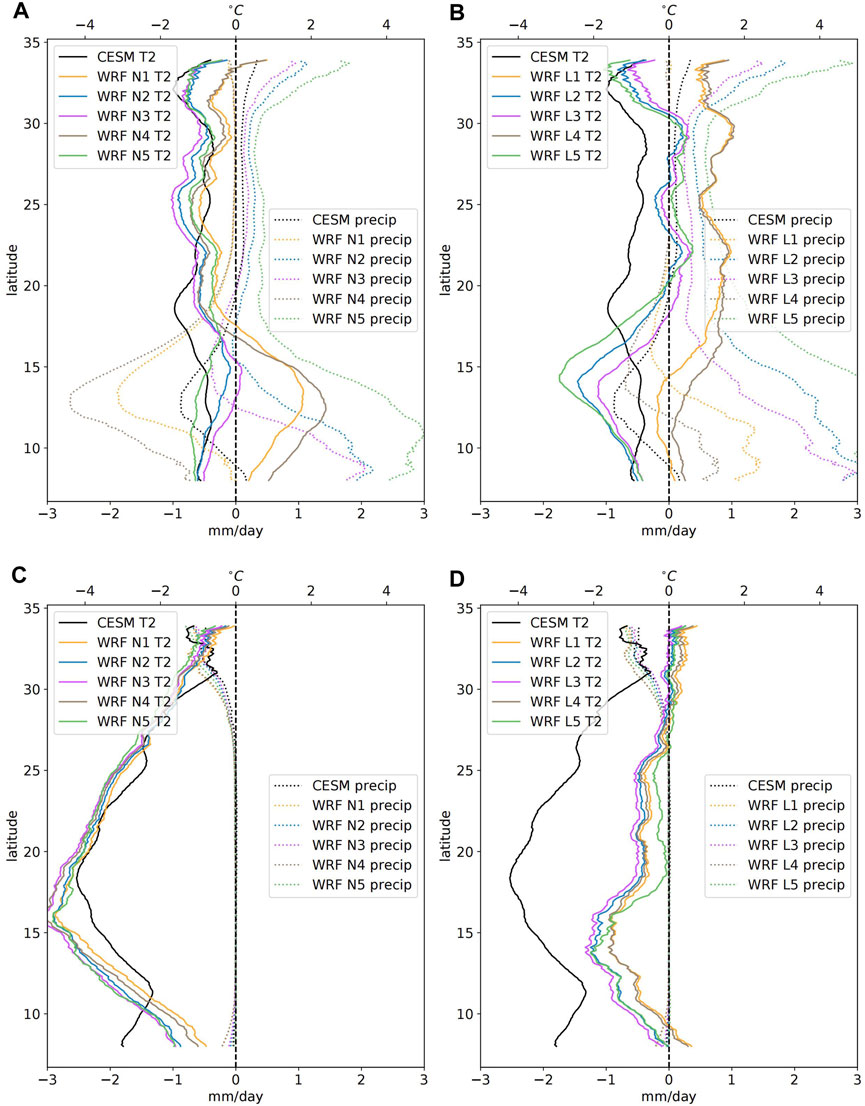
FIGURE 7. Zonally averaged precipitation and temperature fields over the North Africa region for summer season (A,B) and winter season (C,D). Subfigure (A,C) are for the N ensemble while (B,D) are for the L ensemble. Dashed lines are precipitation and solid lines are temperature.
In the Middle East region, during both winter and summer seasons, precipitation and T2 biases (Figure 8) are smaller in magnitude compared to those over North Africa. Ensemble members that exhibit wet biases over North Africa are also wetter over the Middle East, but with a smaller mean bias. In the winter season, mean precipitation bias is overall positive among WRF members, which is most likely due to orographically induced precipitation over elevated terrain that is not fully captured by the low resolution reference data. Summer temperature bias is again influenced by precipitation, but the magnitudes of the mean T2 biases in WRF simulations are less than 2°C. For the winter season, while the mean biases are also less than 2°C for the Noah MP ensemble, they are very close to zero for the CLM ensemble. During summer, the models have a warm bias near 32°N that gradually decreases towards lower and higher latitude (Figure 9), till 40°N where the effects of Black Sea and Caspian Sea become evident. During winter, T2 bias has the coldest region at the southernmost location that gradually warms up and stays near zero northward till about 40°N, where the warm bias over continental Europe becomes visible. Note that the warm T2 bias in CESM during winter introduces a warm bias in the surface water temperatures over the Black Sea and the Caspian Sea, which then act as a prescribed source of heat during winter in WRF simulations and heating the air mass above. The warm air mass then helps spread the T2 bias to coastal region on the south and east of the Black sea that are part of our Middle East region of interest. Influence of the Black Sea also appears in precipitation fields over the surrounding regions during summer season, and in winter season the region of influence extends to the Zagros mountains. These precipitation fields are also modified by the physics schemes in WRF, as members that have high precipitation bias over North Africa also have regions around Black Sea to be more wet than other members. The statistical variables computed over Middle East region are presented in Supplementary Table S3.
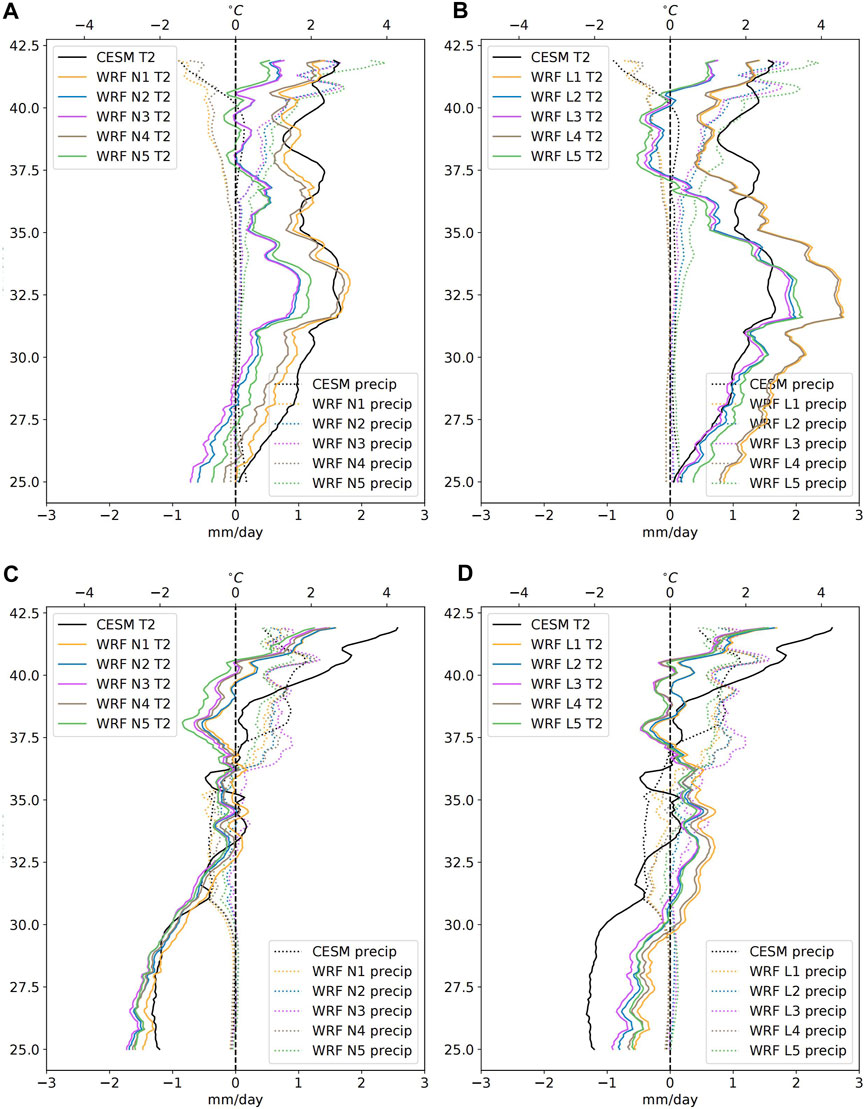
FIGURE 9. Zonally averaged precipitation and temperature anomalies over the Middle East region for the summer (A,B) and winter (C,D) seasons. (A,C) are for the N ensemble while (B,D) are for the L ensemble. Dashed lines are precipitation and solid lines are temperature.
3.1.3 Summary
Overall, the uncoupled WRF physics ensembles demonstrate performance that is acceptable for various investigations of the climate over North Africa and the Mediterranean, although there is scope for more improvement, and ensemble members with better performance able to match low level of bias from other studies. All ensemble members exhibit near surface temperature biases that are lower or similar in magnitude to biases in the global model that is used to force these simulations. For the winter season, the CLM ensemble is able to considerably improve the T2 biases over North Africa compared to the global model and clearly outperforms the Noah-LSM ensemble. Summer season T2 bias for each ensemble member is strongly connected to the precipitation bias in the same member, with the latter showing dependency on the choice of all physics schemes in WRF, and particularly on the choice of the cumulus scheme. The precipitation fields vary from wet to dry among WRF members, most obviously over the tropical convection zone during summer season with smaller variations in other parts of the domain over all seasons. Here it is worth noting that a close to zero net bias in the results might came from biases from different model components that acts to cancel out each other, instead of accurately reproducing every climatological aspect. For more discussion on compensating biases from different physics schemes over our region of interest, see (Zittis and Hadjinicolaou, 2017; Achugbu et al., 2020) for discussion on precipitation and T2 fields and (Kotlarski et al., 2014; Diaz et al., 2015) that discuss cloud cover in addition.
3.2 Coupled downscaling results
Next, we present results from the atmosphere-ocean coupled ensembles. In this section the oceanic variables will be presented in comparison with oceanic observational dataset, while atmospheric variables will be presented in difference with respect to the uncoupled ensemble, so the effect from ocean model coupling becomes directly visible.
The sea surface temperature (SST) anomalies from the coupled ROMS ocean model and from CESM are presented in Figures 10, 11 for the summer and winter seasons, respectively. It is readily visible that the higher resolution of the regional ocean model allows for the simulation of greater detail in the SST field and the ability to capture the complex coastlines with superior fidelity. During summer, the ROMS simulated Mediterranean SST is similar to that simulated by CESM, but during winter it is slightly warmer. This warm winter SST bias likely originates from the warm atmospheric T2 bias over continental Europe (Figure 4) which would explain why those biases are closer to the northern regions of the Mediterranean Sea. Conversely, since the simulated T2 biases over Africa are colder in comparison to those over Europe, the SST biases in the southern Mediterranean are cooler. These connections between the T2 biases in the uncoupled simulations and the SST biases in the coupled simulations suggests that online coupling by OASIS is working as intended.
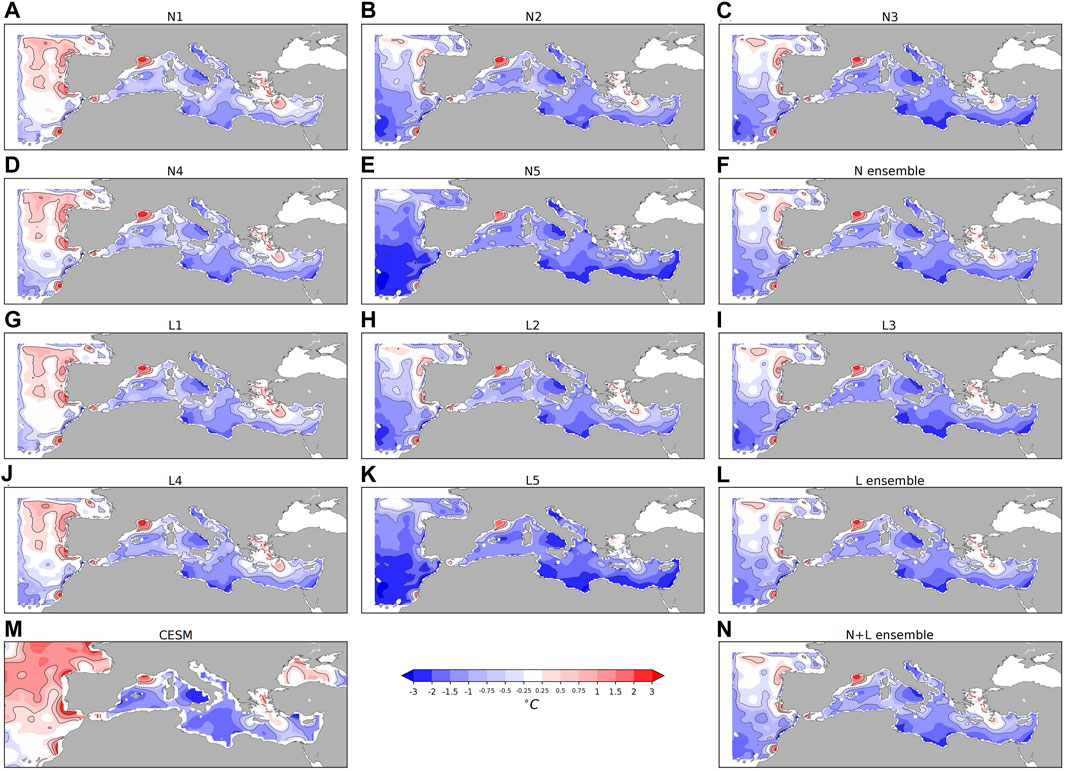
FIGURE 10. ROMS simulated summer season SST anomalies compared to the WOA dataset in the N coupled ensemble (A–E) and the L coupled ensemble (G–K). Anomalies of the ensemble means are shown in sub-figures f, (L) and (N). The CESM model anomaly is shown in (M). Note that the colorbar employs a non-linear color scheme. Contour lines have been plotted at the following values: −3, −2, −1, −0.5, 0.5, 1, 2, 3.
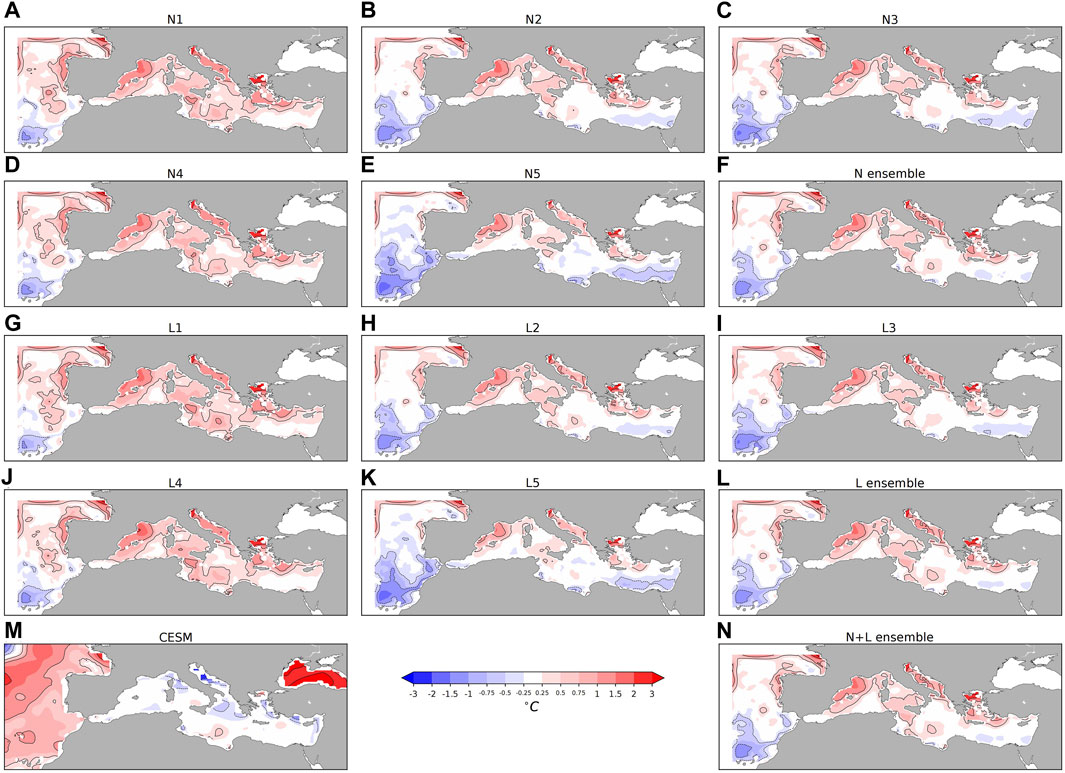
FIGURE 11. Similar to Figure 10 but for the winter SST anomalies.
The SST differences between CESM and ROMS over the Mediterranean are rather small, and are on the order of 1°C in magnitude. This is similar to differences in SSTs between coupled and uncoupled simulations reported by Akhtar et al. (2018) who used reanalysis data to force the atmospheric component. Furthermore, compared to the reference data, the ROMS SST (and the CESM SST) bias is ∼1°C in magnitude, which is also similar to the bias reported by Akhtar et al. (2018), and smaller than the bias obtained by Parras-Berrocal et al. (2020) using a different coupled regional model. Given that the CESM SSTs are quite good in both seasons, ROMS is not able to improve upon that meaningfully. However, CESM does suffers from a very high salinity bias in the Mediterranean which is significantly remediated by ROMS in our coupled configuration (Supplementary Figure S2).
The difference in T2 between coupled and uncoupled simulations (coupled results minus uncoupled results) that uses the same physics scheme set is presented in Figure 12 and Supplementary Figure S3 for summer and winter season respectively. Spatially the main region of T2 difference is unsurprisingly concentrated above water surfaces where ROMS operates, with opposite signed T2 differences over the Atlantic and the Mediterranean regions of the ROMS domain. Over the North Atlantic the T2 fields from the coupled simulations are colder in all seasons, with a clear cutoff at ROMS domain boundary towards the Atlantic. Over the Mediterranean the WRF T2 is slightly warmer in most of the coupled simulations, with higher temperature towards the north and cooler temperature near the coast of Egypt. This spatial distribution of temperature differences matches the SST feature from ROMS that we discussed above and reaffirms the atmosphere-ocean coupling framework is working correctly.
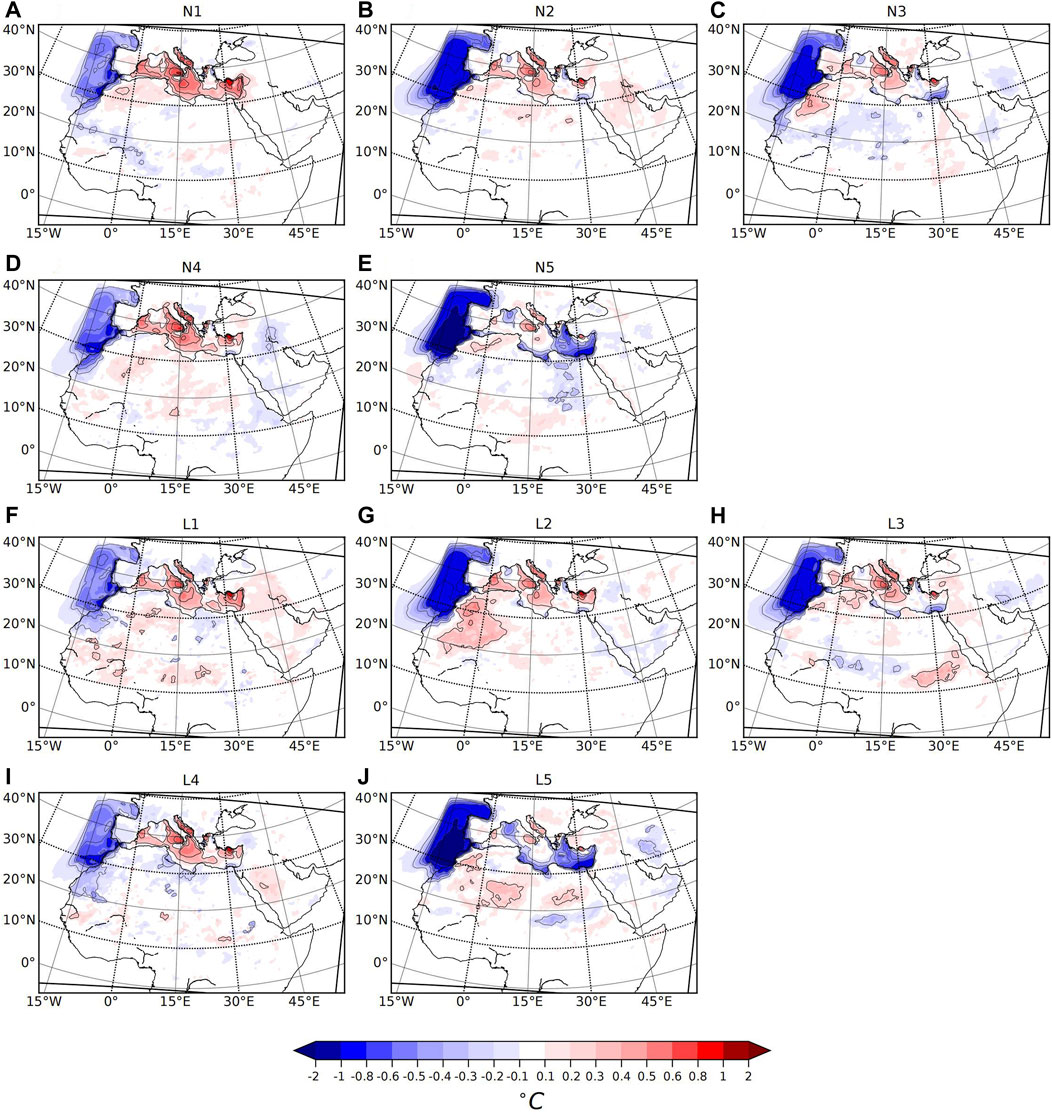
FIGURE 12. Summer temperature changes in coupled simulations with respect to uncoupled simulations for the N ensemble members (A–E) and L ensemble members (F–J). Note that the colorbar range in this figure is smaller than the one used in Figure 3 to highlight the small differences.
There are also T2 differences over land among coupled ensemble members. Although most of the influence of a coupled Mediterranean Sea is confined to within a few-hundred kilometers of the coastal regions, some ensemble members (namely, N1, N4, N5, L1 and L4) do show T2 differences over regions, in the northern most sections of North Africa, that are far removed from the sea. Meanwhile, the T2 difference over tropical convection zone near the equator are more likely due to the internal variability of the WRF model instead of ROMS coupling. The magnitudes of these inland T2 differences are overall small, making it hard to clearly distinguish effects from ROMS coupling and WRF internal variability. Lavin-Gullon et al. (2022) show that the magnitude of internal variations might not be small for slow responding model components. However, simulations in this study all use spectral nudging that would act to limit internal variability of the regional model (Alexandru et al., 2009). Thus, differences between coupled and uncoupled results are more likely from ocean coupling than internal variability.
Precipitation differences between coupled and uncoupled simulations are presented in Figure 13 for the summer season and Supplementary Figure S4 for the winter season. During summer the difference field is largely located over the tropical convection region below 20°N and is highly noisy. This is likely due to internal variability of the model rather than a result of coupling with ROMS. Meanwhile the decrease of precipitation over the ROMS resolved portion of the Atlantic and the continental Europe is most likely due to ocean coupling: reduced SST over the Atlantic leads to lower evaporation, which reduces the moisture load in the downwind region thus reducing precipitation. Only members that are colder over the Atlantic show this decrease in downwind precipitation, while warmer members will have precipitation drop only above Atlantic surface, or close to no drop at all. There is not a lot of summer precipitation difference around the coastal regions of Mediterranean, partly because it is the dry season, and partly because ROMS SST does not differ much from CESM SST during this season. During winter season, however, ROMS SST over northern Mediterranean is notably warmer than CESM, and therefore, the coupled simulations simulate an increase of winter season local precipitation events along the coastlines of the Adriatic and Ionian Seas and over the coastal regions of Turkey. This SST sensitivity of coastal region precipitation hints on the importance of regional ocean model in resolving such precipitation, which is discussed in Berthou et al. (2015) and also mentioned in Parras-Berrocal et al. (2020).
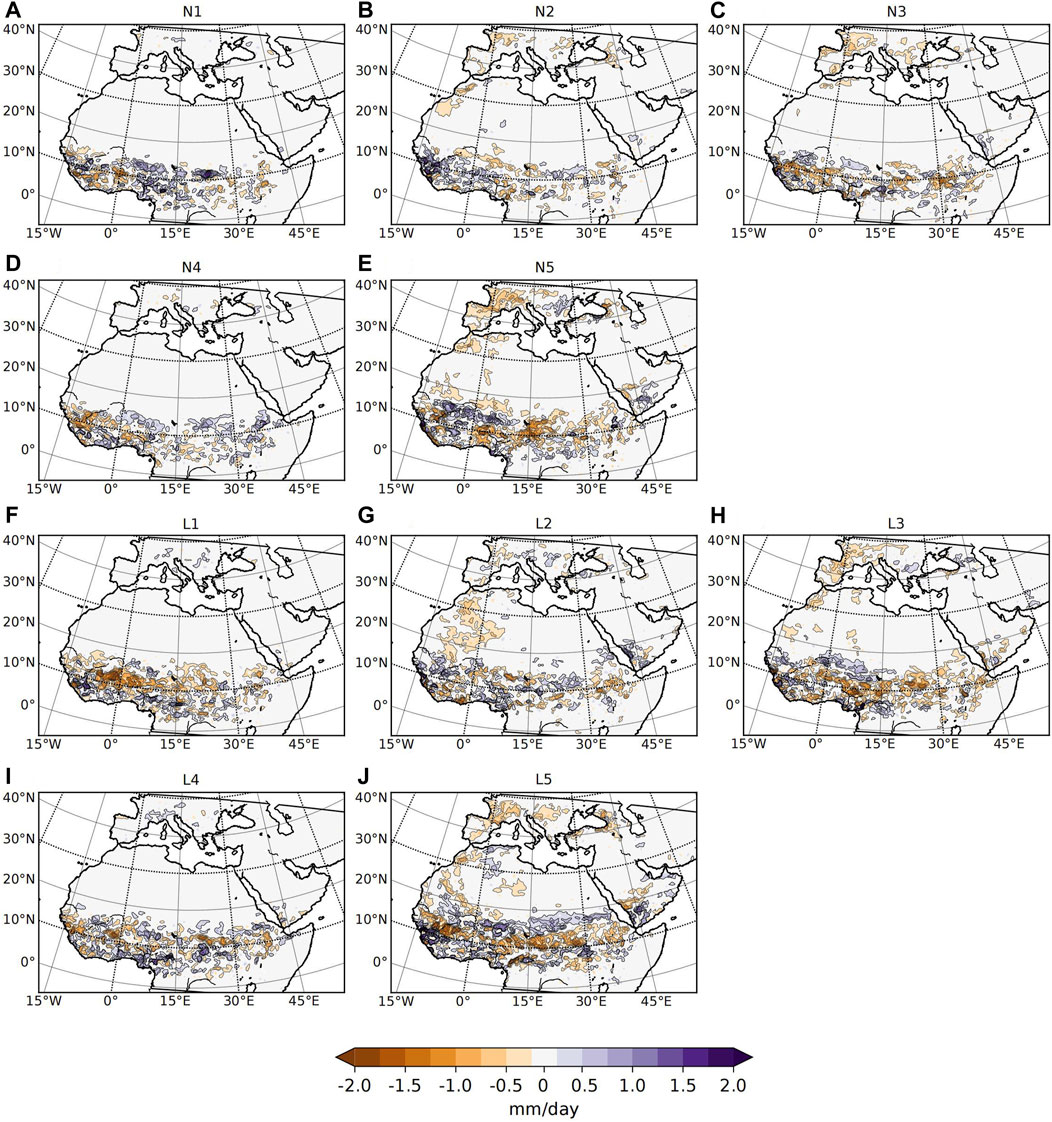
FIGURE 13. Similar to Figure 12 but for the summer precipitation anomalies. Color scale is again smaller than Figure 2 to highlight the small differences.
The difference between atmosphere-ocean coupled simulations and atmosphere only simulations is overall small, with magnitude of difference over land surface not exceeding 0.6°C for T2 and 0.75 mm/day for precipitation in any ensemble member. The main reason for this is that the CESM SST over Mediterranean which provides ocean surface data is already quite close to reference observations, leaving little room for further improvement when ocean coupling is employed. However, we do observe feedbacks between atmosphere and ocean fields that prove the atmosphere-ocean coupling is working as designed. Analysis of climate results over North Africa region and Middle East region will not be performed again for the coupled simulation results, because according to the differences discussed above there is no major change in coupled results over these regions.
4 Summary and discussion
In this study, we assess the ability of the Weather Research and Forecasting (WRF) model to yield realistic dynamically downscaled climate over north Africa, Middle East and the Mediterranean regions for the 1979–1993 historical period. Results from the two uncoupled WRF ensembles show that the biases are largely related to the choices of the various physics schemes. For instance, during the summer season, the precipitation bias is mostly influenced by the choice of the cumulus scheme. This is especially important over the tropical convection zone where the cumulus scheme is responsible for resolving local convection. Summer season T2 biases are also tied to precipitation biases and consequently to the choice of the cumulus scheme through energy transport via evaporation and convection. The influence on either of these biases from other physics schemes is much smaller. Meanwhile in the winter season, when precipitation drops the cumulus scheme no longer exerts a dominant control on the T2 bias, which now comes to be dominated by land surface scheme. Ensemble members that use Noah-LSM have large cold biases over North Africa but very little bias over the Mesopotamia and Levant regions of the Middle East, whereas members that use CLM have much smaller cold bias over North Africa but are slightly warm over Middle East. Given these two regions are both important, it would be wise to keep using both land surface schemes in future studies. It should be noted that the CESM GCM forcing data employed in this study is also not bias free, as previous studies had showed that GCM forcing data could strongly influence biases in regional climate (Xie et al., 2021).
Results from the two additional ROMS coupled ensemble shows that when WRF is coupled to the ROMS ocean model over the Mediterranean and a small part of the North Atlantic, ROMS SSTs interact with the WRF simulated atmosphere and are free to evolve dynamically. This results in SSTs that are different from those simulated by CESM and which were employed in the uncoupled WRF simulations. ROMS SSTs do not vary a lot among the ensemble members and they are very close to the CESM SSTs, however, ROMS SSTs do tend to be slightly warmer than CESM over the northern part of Mediterranean during the winter season. Furthermore, comparing ROMS and CESM with the World Ocean Atlas underscores the fact that they both do a very good job of reproducing the observed SSTs. Because biases in CESM SSTs are mostly within 1°C of observational record, there is little improvement that ROMS can provide on this front, and therefore, the atmospheric fields of the coupled runs are very similar to those from the uncoupled runs. However, CESM has a very serious sea surface salinity bias which is remediated to a large extent by ROMS. Although this does not significantly impact the simulated climate in our study, this improvement in the salinity profile is expected to be helpful for the maintenance of realistic circulations in the Mediterranean.
While the agreement between CESM and observed SSTs for the Mediterranean is impressive, it is not entirely surprising given that modern climate models are configured to reproduce the recent observational record as closely as possible (see Hourdin et al. (2017) for a general discussion; Gent et al. (2011) for details pertaining to our version of CESM. However, how well CESM (or any other modern GCM) simulates Mid-Holocene Mediterranean SSTs remains an open question and in this regard our WRF-ROMS coupled pipeline offers the desirable ability to simulate dynamically and physically consistent oceanic and atmospheric fields for the Mediterranean and surrounding regions for our forthcoming analysis focused on the Mid-Holocene.
We identify a subset of physics configurations that can be employed in future studies for the MEMNA region. Firstly, an examination of the near surface temperature field shows that the L ensemble set performs better over North Africa while the N ensemble set performs better over the Middle East. Since both regions are important for the Mid-Holocene, we are obliged to retain members from both sets for any future study. Examination of the precipitation fields, however, shows that we can exclude member N4/L4, because they are too dry, and N5/L5, because they are too wet. This leaves us with a reduced set of six simulations, namely, N1, N2, N3 from the Noah-LSM ensemble and L1, L2, L3 from the CLM ensemble. Simulated temperature and precipitation anomalies averaged over the selected members are shown in Figure 14. For our following study that will focus on dynamically downscaling the climate of the Mid-Holocene, these six configurations would constitute a mini physics ensemble that provides a good representation of the climate of the MEMNA region while preserving some diversity of the physics schemes.
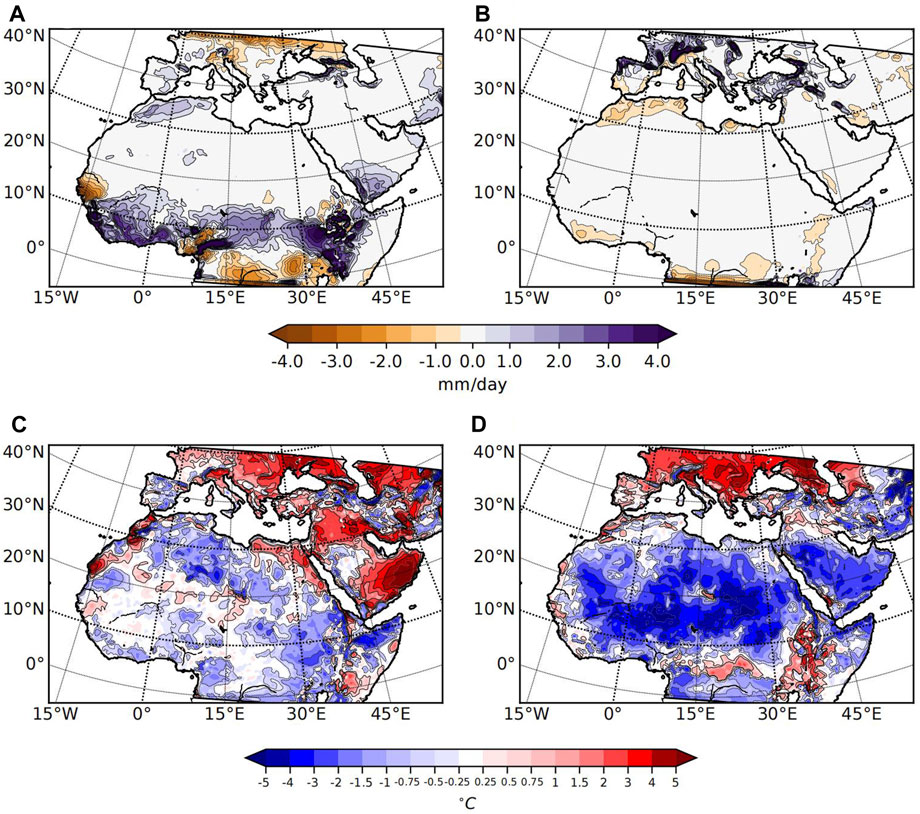
FIGURE 14. Average climate anomalies over the selected better performing members for precipitation over summer (A) and winter (B) seasons, as well as temperature over summer (C) and winter (D) seasons.
Data availability statement
The raw data supporting the conclusion of this article will be made available by the authors, without undue reservation.
Author contributions
FX, DC, and WRP contributed to the conception and design of this study. FX and DC contributed to the software, data analysis and visualization of this study. All authors contributed to the article and approved the submitted version.
Funding
The research of WRP and his students is funded by NSERC Discovery Grant A9627.
Acknowledgments
The simulations presented in this paper were performed on the SciNet High Performance Computing facility at the University of Toronto, which is a component of the Compute Canada HPC platform. The authors would like to thank Jonathan Gula and Valentine Bernu for providing assistance on implementing the CROCO software.
Conflict of interest
The authors declare that the research was conducted in the absence of any commercial or financial relationships that could be construed as a potential conflict of interest.
Publisher’s note
All claims expressed in this article are solely those of the authors and do not necessarily represent those of their affiliated organizations, or those of the publisher, the editors and the reviewers. Any product that may be evaluated in this article, or claim that may be made by its manufacturer, is not guaranteed or endorsed by the publisher.
Supplementary material
The Supplementary Material for this article can be found online at: https://www.frontiersin.org/articles/10.3389/feart.2023.1200004/full#supplementary-material
Footnotes
3https://mena-cordex.cyi.ac.cy/
References
Achugbu, I. C., Dudhia, J., Olufayo, A. A., Balogun, I. A., Adefisan, E. A., and Gbode, I. E. (2020). Assessment of wrf land surface model performance over west africa. Adv. Meteorology 2020, 1–30. doi:10.1155/2020/6205308
Akhtar, N., Brauch, J., and Ahrens, B. (2018). Climate modeling over the mediterranean sea: impact of resolution and ocean coupling. Clim. Dyn. 51, 933–948. doi:10.1007/s00382-017-3570-8
Alaka, G. J., and Maloney, E. D. (2017). Internal intraseasonal variability of the west african monsoon in wrf. J. Clim. 30, 5815–5833. doi:10.1175/jcli-d-16-0750.1
Alexandru, A., De Elia, R., Laprise, R., Separovic, L., and Biner, S. (2009). Sensitivity study of regional climate model simulations to large-scale nudging parameters. Mon. Weather Rev. 137, 1666–1686. doi:10.1175/2008mwr2620.1
Andrews, J., Carolin, S., Peckover, E., Marca, A., Al-Omari, S., and Rowe, P. (2020). Holocene stable isotope record of insolation and rapid climate change in a stalagmite from the zagros of Iran. Quat. Sci. Rev. 241, 106433. doi:10.1016/j.quascirev.2020.106433
Auclair, F., Bordois, L., Dossmann, Y., Duhaut, T., Paci, A., Ulses, C., et al. (2018). A non-hydrostatic non-boussinesq algorithm for free-surface ocean modelling. Ocean. Model. 132, 12–29. doi:10.1016/j.ocemod.2018.07.011
Berthou, S., Mailler, S., Drobinski, P., Arsouze, T., Bastin, S., Béranger, K., et al. (2015). Sensitivity of an intense rain event between atmosphere-only and atmosphere–ocean regional coupled models: 19 september 1996. Q. J. R. Meteorological Soc. 141, 258–271. doi:10.1002/qj.2355
Boyer, T., Baranova, O., Locarnini, R., Mishonov, A., Grodsky, A., Paver, C., et al. (2019). World ocean atlas 2018 product documentation ocean climate laboratory ncei. NESDIS/NOAA NOAA National Centers for Environmental Information. doi:10.13140/RG.2.2.34758.01602
Brayshaw, D. J., Rambeau, C. M., and Smith, S. J. (2011). Changes in mediterranean climate during the holocene: insights from global and regional climate modelling. Holocene 21, 15–31. doi:10.1177/0959683610377528
Chandan, D., and Peltier, W. R. (2020). African humid period precipitation sustained by robust vegetation, soil, and lake feedbacks. Geophys. Res. Lett. 47, e2020GL088728. doi:10.1029/2020gl088728
Chen, F., and Dudhia, J. (2001). Coupling an advanced land surface-hydrology model with the penn state-ncar mm5 modeling system. part i: model implementation and sensitivity. Mon. Weather Rev. 129, 569–585. doi:10.1175/1520-0493(2001)129⟨0569:CAALSH⟩2.0.CO;2
Chen, J., Wu, X., Lu, C., and Yin, Y. (2022). Seasonal and diurnal variations of cloud systems over the eastern Tibetan plateau and east China: a cloud-resolving model study. Adv. Atmos. Sci. 39, 1034–1049. doi:10.1007/s00376-021-0391-9
Chen, J., Wu, X., Yin, Y., Lu, C., Xiao, H., Huang, Q., et al. (2019). Thermal effects of the surface heat flux on cloud systems over the Tibetan plateau in boreal summer. J. Clim. 32, 4699–4714. doi:10.1175/JCLI-D-18-0604.1
Constantinidou, K., Hadjinicolaou, P., Zittis, G., and Lelieveld, J. (2020). Performance of land surface schemes in the wrf model for climate simulations over the mena-cordex domain. Earth Syst. Environ. 4, 647–665. doi:10.1007/s41748-020-00187-1
Constantinidou, K., Zittis, G., and Hadjinicolaou, P. (2019). Variations in the simulation of climate change impact indices due to different land surface schemes over the mediterranean, middle east and northern africa. Atmosphere 10, 26. doi:10.3390/atmos10010026
Craig, A., Valcke, S., and Coquart, L. (2017). Development and performance of a new version of the oasis coupler, oasis3-mct_3.0. Geosci. Model Dev. 10, 3297–3308. doi:10.5194/gmd-10-3297-2017
Debreu, L., Marchesiello, P., Penven, P., and Cambon, G. (2012). Two-way nesting in split-explicit ocean models: algorithms, implementation and validation. Ocean. Model. 49-50, 1–21. doi:10.1016/j.ocemod.2012.03.003
Dee, D. P., Uppala, S. M., Simmons, A. J., Berrisford, P., Poli, P., Kobayashi, S., et al. (2011). The era-interim reanalysis: configuration and performance of the data assimilation system. Q. J. R. Meteorological Soc. 137, 553–597. doi:10.1002/qj.828
Dell’Aquila, A., Mariotti, A., Bastin, S., Calmanti, S., Cavicchia, L., Deque, M., et al. (2018). Evaluation of simulated decadal variations over the euro-mediterranean region from ensembles to med-cordex. Clim. Dyn. 51, 857–876. doi:10.1007/s00382-016-3143-2
Deser, C., Phillips, A. S., Alexander, M. A., and Smoliak, B. V. (2014). Projecting north american climate over the next 50 years: uncertainty due to internal variability. J. Clim. 27, 2271–2296. doi:10.1175/JCLI-D-13-00451.1
Diaz, J., González, A., Expósito, F., Pérez, J., Fernández, J., García-Díez, M., et al. (2015). Wrf multi-physics simulation of clouds in the african region. Q. J. R. Meteorological Soc. 141, 2737–2749. doi:10.1002/qj.2560
d’Orgeville, M., Peltier, W. R., Erler, A. R., and Gula, J. (2014). Climate change impacts on great lakes basin precipitation extremes. J. Geophys. Res. Atmos. 119 (10), 10,799–10,812. doi:10.1002/2014JD021855
Dunne, J., Evershed, R. P., Salque, M., Cramp, L., Bruni, S., Ryan, K., et al. (2012). First dairying in green saharan africa in the fifth millennium bc. Nature 486, 390–394. doi:10.1038/nature11186
Erler, A. R. (2015). High resolution hydro-climatological projections for western Canada. Ph.D. thesis. University of Toronto.
Erler, A. R., and Peltier, W. R. (2016). Projected changes in precipitation extremes for western Canada based on high-resolution regional climate simulations. J. Clim. 29, 8841–8863. doi:10.1175/JCLI-D-15-0530.1
Erler, A. R., and Peltier, W. R. (2017). Projected hydroclimatic changes in two major river basins at the canadian west coast based on high-resolution regional climate simulations. J. Clim. 30, 8081–8105. doi:10.1175/JCLI-D-16-0870.1
Erler, A. R., Peltier, W. R., and D’Orgeville, M. (2015). Dynamically downscaled high-resolution hydroclimate projections for western Canada. J. Clim. 28, 423–450. doi:10.1175/JCLI-D-14-00174.1
Eyring, V., Bony, S., Meehl, G. A., Senior, C. A., Stevens, B., Stouffer, R. J., et al. (2016). Overview of the coupled model intercomparison project phase 6 (cmip6) experimental design and organization. Geosci. Model Dev. 9, 1937–1958. doi:10.5194/gmd-9-1937-2016
Finné, M., Holmgren, K., Sundqvist, H. S., Weiberg, E., and Lindblom, M. (2011). Climate in the eastern mediterranean, and adjacent regions, during the past 6000 years – a review. J. Archaeol. Sci. 38, 3153–3173. doi:10.1016/j.jas.2011.05.007
García-Díez, M., Fernández, J., and Vautard, R. (2015). An rcm multi-physics ensemble over europe: multi-variable evaluation to avoid error compensation. Clim. Dyn. 45, 3141–3156. doi:10.1007/s00382-015-2529-x
Gent, P. R., Danabasoglu, G., Donner, L. J., Holland, M. M., Hunke, E. C., Jayne, S. R., et al. (2011). The community climate system model version 4. J. Clim. 24, 4973–4991. doi:10.1175/2011JCLI4083.1
Giorgi, F., Jones, C., Asrar, G. R., et al. (2009). Addressing climate information needs at the regional level: the cordex framework. World Meteorol. Organ. (WMO) Bull. 58, 175.
Glotfelty, T., Ramírez-Mejía, D., Bowden, J., Ghilardi, A., and West, J. J. (2021). Limitations of wrf land surface models for simulating land use and land cover change in sub-saharan africa and development of an improved model (clm-af v. 1.0). Geosci. Model Dev. 14, 3215–3249. doi:10.5194/gmd-14-3215-2021
Grell, G. A., and Freitas, S. R. (2014). A scale and aerosol aware stochastic convective parameterization for weather and air quality modeling. Atmos. Chem. Phys. 14, 5233–5250. doi:10.5194/acp-14-5233-2014
Gula, J., and Peltier, W. R. (2012). Dynamical downscaling over the great lakes basin of NorthNorth America using the WRF regional climate model: the impact of the great lakes system on regional greenhouse warming. J. Clim. 25, 7723–7742. doi:10.1175/jcli-d-11-00388.1
Harris, I., Jones, P. D., Osborn, T. J., and Lister, D. H. (2013). Updated high-resolution grids of monthly climatic observations–the CRU TS3. 10 Dataset. Int. J. Climatol. 34, 623–642. doi:10.1002/joc.3711
Ho-Hagemann, H. T. M., Hagemann, S., Grayek, S., Petrik, R., Rockel, B., Staneva, J., et al. (2020). Internal model variability of the regional coupled system model gcoast-ahoi. Atmosphere 11, 227. doi:10.3390/atmos11030227
Hong, S.-Y., and Lim, J.-O. J. (2006). The wrf single-moment 6-class microphysics scheme (wsm6). Asia-Pacific J. Atmos. Sci. 42, 129–151.
Hong, S.-Y., Noh, Y., and Dudhia, J. (2006). A new vertical diffusion package with an explicit treatment of entrainment processes. Mon. Weather Rev. 134, 2318–2341. doi:10.1175/MWR3199.1
Hourdin, F., Mauritsen, T., Gettelman, A., Golaz, J.-C., Balaji, V., Duan, Q., et al. (2017). The art and science of climate model tuning. Bull. Am. Meteorological Soc. 98, 589–602. doi:10.1175/bams-d-15-00135.1
Huo, Y., and Peltier, W. R. (2019). Dynamically downscaled climate simulations of the indian monsoon in the instrumental era: physics parameterization impacts and precipitation extremes. J. Appl. Meteorology Climatol. 58, 831–852. doi:10.1175/JAMC-D-18-0226.1
Huo, Y., and Peltier, W. R. (2020). Dynamically downscaled climate change projections for the south asian monsoon: mean and extreme precipitation changes and physics parameterization impacts. J. Clim. 33, 2311–2331. doi:10.1175/jcli-d-19-0268.1
Huo, Y., and Peltier, W. R. (2021). The southeast asian monsoon: dynamically downscaled climate change projections and high resolution regional ocean modelling on the effects of the Tibetan plateau. Clim. Dyn. 56, 2597–2616. doi:10.1007/s00382-020-05604-9
Huo, Y., Peltier, W. R., and Chandan, D. (2021). Mid-holocene monsoons in south and southeast asia: dynamically downscaled simulations and the influence of the green sahara. Clim. Past 17, 1645–1664. doi:10.5194/cp-17-1645-2021
Huo, Y., Peltier, W. R., and Chandan, D. (2022). Mid-holocene climate of the Tibetan plateau and hydroclimate in three major river basins based on high-resolution regional climate simulations. Clim. Past 18, 2401–2420. doi:10.5194/cp-18-2401-2022
Iacono, M. J., Delamere, J. S., Mlawer, E. J., Shephard, M. W., Clough, S. A., and Collins, W. D. (2008). Radiative forcing by long-lived greenhouse gases: calculations with the aer radiative transfer models. J. Geophys. Res. Atmos. 113. doi:10.1029/2008JD009944
IPCC (2013). The fifth assessment report (AR5) of the united nations intergovernmental panel on climate change (IPCC), climate change 2013: the physical science basis, IPCC WGI AR5. Climate phenomena and their relevance for future regional climate change. Cambridge University Press. chap. 14.
IPCC (2021). Climate change 2021: the physical science basis. Contribution of working group I to the sixth assessment report of the intergovernmental panel on climate change. Cambridge, United Kingdom and New York, NY, USA: Cambridge University Press. vol. In Press. doi:10.1017/9781009157896
Jacob, D., Teichmann, C., Sobolowski, S., Katragkou, E., Anders, I., Belda, M., et al. (2020). Regional climate downscaling over europe: perspectives from the euro-cordex community. Reg. Environ. change 20, 51–20. doi:10.1007/s10113-020-01606-9
Jiménez, P. A., Dudhia, J., González-Rouco, J. F., Navarro, J., Montávez, J. P., and García-Bustamante, E. (2012). A revised scheme for the wrf surface layer formulation. Mon. Weather Rev. 140, 898–918. doi:10.1175/MWR-D-11-00056.1
Katragkou, E., García-Díez, M., Vautard, R., Sobolowski, S., Zanis, P., Alexandri, G., et al. (2015). Regional climate hindcast simulations within euro-cordex: evaluation of a wrf multi-physics ensemble. Geosci. Model Dev. 8, 603–618. doi:10.5194/gmd-8-603-2015
Katsafados, P., Papadopoulos, A., Korres, G., and Varlas, G. (2016). A fully coupled atmosphere–ocean wave modeling system for the mediterranean sea: interactions and sensitivity to the resolved scales and mechanisms. Geosci. Model Dev. 9, 161–173. doi:10.5194/gmd-9-161-2016
Klein, C., Heinzeller, D., Bliefernicht, J., and Kunstmann, H. (2015). Variability of west african monsoon patterns generated by a wrf multi-physics ensemble. Clim. Dyn. 45, 2733–2755. doi:10.1007/s00382-015-2505-5
Kotlarski, S., Keuler, K., Christensen, O. B., Colette, A., Déqué, M., Gobiet, A., et al. (2014). Regional climate modeling on european scales: a joint standard evaluation of the euro-cordex rcm ensemble. Geosci. Model Dev. 7, 1297–1333. doi:10.5194/gmd-7-1297-2014
Larrasoaña, J. C., Roberts, A. P., and Rohling, E. J. (2013). Dynamics of green sahara periods and their role in hominin evolution. PloS one 8, e76514. doi:10.1371/journal.pone.0076514
Lavin-Gullon, A., Fernandez, J., Bastin, S., Cardoso, R. M., Fita, L., Giannaros, T. M., et al. (2021). Internal variability versus multi-physics uncertainty in a regional climate model. Int. J. Climatol. 41, E656–E671. doi:10.1002/joc.6717
Lavin-Gullon, A., Milovac, J., García-Díez, M., and Fernández, J. (2022). Spin-up time and internal variability analysis for overlapping time slices in a regional climate model. Clim. Dyn. 61, 47–64. doi:10.1007/s00382-022-06560-2
Lawrence, D. M., Oleson, K. W., Flanner, M. G., Thornton, P. E., Swenson, S. C., Lawrence, P. J., et al. (2011). Parameterization improvements and functional and structural advances in version 4 of the community land model. J. Adv. Model. Earth Syst. 3. doi:10.1029/2011MS00045
Li, R., Jin, J., Wang, S.-Y., and Gillies, R. R. (2015). Significant impacts of radiation physics in the weather research and forecasting model on the precipitation and dynamics of the west african monsoon. Clim. Dyn. 44, 1583–1594. doi:10.1007/s00382-014-2294-2
Malanotte-Rizzoli, P., and Hecht, A. (1988). Large-scale properties of the eastern mediterranean-a review. Oceanol. Acta 11, 323–335. doi:10.1016/S0967-0645(99)00020-X
Manning, K., and Timpson, A. (2014). The demographic response to holocene climate change in the sahara. Quat. Sci. Rev. 101, 28–35. doi:10.1016/j.quascirev.2014.07.003
Mauri, A., Davis, B., Collins, P., and Kaplan, J. (2015). The climate of europe during the holocene: a gridded pollen-based reconstruction and its multi-proxy evaluation. Quat. Sci. Rev. 112, 109–127. doi:10.1016/j.quascirev.2015.01.013
Nakanishi, M., and Niino, H. (2006). An improved mellor–yamada level-3 model: its numerical stability and application to a regional prediction of advection fog. Boundary-Layer Meteorol. 119, 397–407. doi:10.1007/s10546-005-9030-8
Nakanishi, M., and Niino, H. (2009). Development of an improved turbulence closure model for the atmospheric boundary layer. J. Meteor. Soc. Jpn. 87, 895–912. doi:10.2151/jmsj.87.895
Nikiema, P. M., Sylla, M. B., Ogunjobi, K., Kebe, I., Gibba, P., and Giorgi, F. (2017). Multi-model cmip5 and cordex simulations of historical summer temperature and precipitation variabilities over west africa. Int. J. Climatol. 37, 2438–2450. doi:10.1002/joc.4856
Omrani, H., Drobinski, P., and Dubos, T. (2015). Using nudging to improve global-regional dynamic consistency in limited-area climate modeling: what should we nudge? Clim. Dyn. 44, 1627–1644. doi:10.1007/s00382-014-2453-5
Otto-Bliesner, B. L., Braconnot, P., Harrison, S. P., Lunt, D. J., Abe-Ouchi, A., Albani, S., et al. (2017). The pmip4 contribution to cmip6–part 2: two interglacials, scientific objective and experimental design for holocene and last interglacial simulations. Geosci. Model Dev. 10, 3979–4003. doi:10.5194/gmd-10-3979-2017
Parras-Berrocal, I. M., Vazquez, R., Cabos, W., Sein, D., Mañanes, R., Perez-Sanz, J., et al. (2020). The climate change signal in the mediterranean sea in a regionally coupled atmosphere–ocean model. Ocean Sci. 16, 743–765. doi:10.5194/os-16-743-2020
Peings, Y., Cattiaux, J., Vavrus, S., and Magnusdottir, G. (2017). Late twenty-first-century changes in the midlatitude atmospheric circulation in the cesm large ensemble. J. Clim. 30, 5943–5960. doi:10.1175/JCLI-D-16-0340.1
Peltier, W. R., and Vettoretti, G. (2014). Dansgaard-oeschger oscillations predicted in a comprehensive model of glacial climate: a “kicked” salt oscillator in the atlantic. Geophys. Res. Lett. 41, 7306–7313. doi:10.1002/2014GL061413
Penven, P., Marchesiello, P., Debreu, L., and Lefèvre, J. (2008). Software tools for pre- and post-processing of oceanic regional simulations. Environ. Model. Softw. 23, 660–662. doi:10.1016/j.envsoft.2007.07.004
Peyron, O., Combourieu-Nebout, N., Brayshaw, D., Goring, S., Andrieu-Ponel, V., Desprat, S., et al. (2017). Precipitation changes in the mediterranean basin during the holocene from terrestrial and marine pollen records: a model–data comparison. Clim. Past 13, 249–265. doi:10.5194/cp-13-249-2017
Pinardi, N., Cessi, P., Borile, F., and Wolfe, C. L. P. (2019). The mediterranean sea overturning circulation. J. Phys. Oceanogr. 49, 1699–1721. doi:10.1175/JPO-D-18-0254.1
Ricchi, A., Miglietta, M. M., Barbariol, F., Benetazzo, A., Bergamasco, A., Bonaldo, D., et al. (2017). Sensitivity of a mediterranean tropical-like cyclone to different model configurations and coupling strategies. Atmosphere 8, 92. doi:10.3390/atmos8050092
Roberts, N., Brayshaw, D., Kuzucuoğlu, C., Perez, R., and Sadori, L. (2011). The mid-holocene climatic transition in the mediterranean: causes and consequences. Holocene 21, 3–13. doi:10.1177/0959683610388058
Robinson, S. A., Black, S., Sellwood, B. W., and Valdes, P. J. (2006). A review of palaeoclimates and palaeoenvironments in the levant and eastern mediterranean from 25,000 to 5000 years bp: setting the environmental background for the evolution of human civilisation. Quat. Sci. Rev. 25, 1517–1541. doi:10.1016/j.quascirev.2006.02.006
Romera, R., Sánchez, E., Domínguez, M., Gaertner, M. Á., and Gallardo, C. (2015). Evaluation of present-climate precipitation in 25 km resolution regional climate model simulations over northwest africa. Clim. Res. 66, 125–139. doi:10.3354/cr01330
Ruti, P. M., Somot, S., Giorgi, F., Dubois, C., Flaounas, E., Obermann, A., et al. (2016). Med-cordex initiative for mediterranean climate studies. Bull. Am. Meteorological Soc. 97, 1187–1208. doi:10.1175/BAMS-D-14-00176.1
Separovic, L., de Elía, R., and Laprise, R. (2012). Impact of spectral nudging and domain size in studies of rcm response to parameter modification. Clim. Dyn. 38, 1325–1343. doi:10.1007/s00382-011-1072-7
Sevault, F., Somot, S., Alias, A., Dubois, C., Lebeaupin-Brossier, C., Nabat, P., et al. (2014). A fully coupled mediterranean regional climate system model: design and evaluation of the ocean component for the 1980–2012 period. Tellus A Dyn. Meteorology Oceanogr. 66, 23967. doi:10.3402/tellusa.v66.23967
Shchepetkin, A. F., and McWilliams, J. C. (2005). The regional oceanic modeling system (roms): a split-explicit, free-surface, topography-following-coordinate oceanic model. Ocean. Model. 9, 347–404. doi:10.1016/j.ocemod.2004.08.002
Skamarock, W. C., Klemp, J. B., Dudhia, J., Gill, D. O., Liu, Z., Berner, J., et al. (2019). A description of the advanced research WRF model version 4. Tech. Rep. 145.
Taylor, K. E., Stouffer, R. J., and Meehl, G. A. (2012). An overview of cmip5 and the experiment design. Bull. Am. meteorological Soc. 93, 485–498. doi:10.1175/bams-d-11-00094.1
Tegen, I., Hollrig, P., Chin, M., Fung, I., Jacob, D., and Penner, J. (1997). Contribution of different aerosol species to the global aerosol extinction optical thickness: estimates from model results. J. Geophys. Res. Atmos. 102, 23895–23915. doi:10.1029/97jd01864
Thompson, G., Field, P. R., Rasmussen, R. M., and Hall, W. D. (2008). Explicit forecasts of winter precipitation using an improved bulk microphysics scheme. part ii: implementation of a new snow parameterization. Mon. Weather Rev. 136, 5095–5115. doi:10.1175/2008MWR2387.1
Tiedtke, M. (1989). A comprehensive mass flux scheme for cumulus parameterization in large-scale models. Mon. Weather Rev. 117, 1779–1800. doi:10.1175/1520-0493(1989)117⟨1779:ACMFSF⟩2.0.CO;2
Timm, O., Köhler, P., Timmermann, A., and Menviel, L. (2010). Mechanisms for the onset of the african humid period and sahara greening 14.5–11 ka bp. J. Clim. 23, 2612–2633. doi:10.1175/2010JCLI3217.1
Turuncoglu, U. U., and Sannino, G. (2017). Validation of newly designed regional earth system model (regesm) for mediterranean basin. Clim. Dyn. 48, 2919–2947. doi:10.1007/s00382-016-3241-1
Vilibić, I., Horvath, K., and Palau, J. L. (2019). Meteorology and climatology of the mediterranean and Black seas. Springer.
Xie, F., Erler, A. R., Chandan, D., and Peltier, W. R. (2021). Great lakes basin heat waves: an analysis of their increasing probability of occurrence under global warming. Front. Water 157. doi:10.3389/frwa.2021.782265
Zebaze, S., Jain, S., Salunke, P., Shafiq, S., and Mishra, S. K. (2019). Assessment of cmip5 multimodel mean for the historical climate of africa. Atmos. Sci. Lett. 20, e926. doi:10.1002/asl.926
Zittis, G., and Hadjinicolaou, P. (2017). The effect of radiation parameterization schemes on surface temperature in regional climate simulations over the mena-cordex domain. Int. J. Climatol. 37, 3847–3862. doi:10.1002/joc.4959
Zittis, G., Hadjinicolaou, P., Klangidou, M., Proestos, Y., and Lelieveld, J. (2019). A multi-model, multi-scenario, and multi-domain analysis of regional climate projections for the mediterranean. Reg. Environ. Change 19, 2621–2635. doi:10.1007/s10113-019-01565-w
Keywords: regional climate modelling, dynamical downscaling, Mediterranean climate, Levant climate, North Africa climate
Citation: Xie F, Chandan D and Peltier WR (2023) A climate modeling study over Northern Africa and the Mediterranean basin with multi-physics ensemble and coupling to a regional ocean modeling system. Front. Earth Sci. 11:1200004. doi: 10.3389/feart.2023.1200004
Received: 04 April 2023; Accepted: 20 October 2023;
Published: 28 November 2023.
Edited by:
Andreas Franz Prein, National Center for Atmospheric Research (UCAR), United StatesReviewed by:
Panos Hadjinicolaou,The Cyprus Institute, CyprusChunsong Lu, Nanjing University of Information Science and Technology, China
Copyright © 2023 Xie, Chandan and Peltier. This is an open-access article distributed under the terms of the Creative Commons Attribution License (CC BY). The use, distribution or reproduction in other forums is permitted, provided the original author(s) and the copyright owner(s) are credited and that the original publication in this journal is cited, in accordance with accepted academic practice. No use, distribution or reproduction is permitted which does not comply with these terms.
*Correspondence:W. Richard Peltier, cGVsdGllckBhdG1vc3AucGh5c2ljcy51dG9yb250by5jYQ==
†ORCID: Fengyi Xie, orcid.org/0009-0007-9464-231X; Deepak Chandan, orcid.org/0000-0002-0756-754X; W. Richard Peltier, orcid.org/0000-0002-5555-7661