- 1Joint Innovation Center for Modern Forestry Studies, College of Forestry, Nanjing Forestry University, Nanjing, China
- 2State Key Laboratory of Hydrology-Water Resources and Hydraulics Engineering, Hohai University, Nanjing, China
Introduction: The middle and lower reaches of the Yangtze River basin (hereafter MLYRB) are one of the most important wintering grounds for waterbirds along the East Asian-Australasian Flyway (EAAF). Climate change and human activities have greatly altered the habitats of waterbirds in this region.
Methods: The potential distribution of suitable habitats for the Siberian crane (Grus leucogeranus) in the MLYRB was projected using Maximum Entropy Models (MaxEnt) and three Global Climate Models (GCMs). Additionally, estimations for temperature and precipitation before 2060 were made using CMIP6 multi-model and multi-scenario data.
Results: 1) the MaxEnt model was highly applicable (AUC = 0.939) for analyzing the suitable habitat distribution and climatic suitability of Siberian cranes in the study area; 2) Precipitation of the driest quarter and altitude were the main factors affecting the potential suitable habitat distribution of Siberian cranes, accounting for 40% of the total contribution rate each; 3) The climatically suitable areas for the distribution of suitable habitats of Siberian cranes in the study area was mainly concentrated in parts of Poyang Lake, Dongting Lake, Taihu Lake, and the mainstream of the Yangtze River; 4) In the BCC-CSM2-MR, CanESM5, and CNRM-CM6-1 models, the suitable habitat area for Siberian cranes is expected to decrease under different SSP scenarios from 2021 to 2060 compared to 1970–2000.
Discussion: The BCC-CSM2-MR model’s SSP2-4.5 scenario shows the most significant decline in the suitable habitat area for Siberian cranes, with a maximum decrease of approximately 35.7%, followed by a maximum decrease of about 26.2% and 16.4% under the CNRM-CM6-1 and CanESM5 models’ SSP2-4.5 scenario, respectively. In general, the suitable habitat areas for Siberian cranes are projected to decline, indicating the need for comprehensive evaluation and uncertainty research using more models.
1 Introduction
Wintering waterbirds are critical indicators of the ecological health and functioning of wetland ecosystems (Yao et al., 2020). However, the loss of wetlands is now the most significant global threat to many bird species. In response, China took a major step towards its protection with the implementation of the Wetland Protection Law of the People’s Republic of China on 1 June 2022, which is the first legislation in China to specifically protect wetlands. This law helps to reinforce the importance of preserving migratory waterfowl populations and their habitats and underlines the need for ongoing efforts to safeguard our fragile ecosystems.
The Siberian crane (Grus leucogeranus) is a majestic wading bird that is endemic to wetlands and relies on these habitats for its survival and reproduction. Listed as “critically endangered” on the IUCN Red List since 2000 (Matson et al., 2011), the Siberian crane is also a Class I protected species in China. With an estimated global population of 3,800–4,000 individuals (Fengshan and Harris, 2012), separated into three distinct migration populations, the Eastern flyway population is the largest, accounting for 99% of the total (Chen et al., 2016). However, the Western and Central populations have declined significantly in recent decades and are close to extinction. Every autumn, almost all cranes migrate over a distance of 5,300 km, traveling from the northeastern part of Siberia, crossing through the eastern regions of China, and heading southwards to the middle and lower reaches of the Yangtze River and beyond for the winter (Kanai et al., 2002). A substantial decrease in waterbird populations has been observed along the majority of migratory routes globally. The East Asian-Australasian Flyway (EAAF) is the most species-rich and threatened among the nine migratory routes for waterfowl. It is estimated that the decline rate of this ecosystem ranges from 5% to 9% per year, which is among the highest rates of any ecological system on the planet (Mackinnon et al., 2012). The EAAF encompasses 22 countries and extends over a distance of 13,000 km, from the Arctic Circle to Australia and New Zealand (Bamford et al., 2008).
The presence and abundance of wetlands and water bodies, as well as the size and connectivity of these habitats, have a positive impact on waterfowl species’ diversity and abundance (Zhang et al., 2018). Unfortunately, human activities have caused a 50% reduction in the worldwide area of wetlands and a decline in the quality of waterfowl habitats (Sun et al., 2020). The combined effects of these changes and climate change have led to an unprecedented loss of biodiversity. The lakes in the floodplains of the MLYRB are essential wintering grounds for waterbirds on the East Asia-Australasian Flyway (Xia et al., 2017). However, in recent decades, wetland areas in Eastern China have continued to shrink, particularly in the lower reaches of the Yangtze River, leading to a significant loss of habitat. The effects of climate change, including increasingly unpredictable weather patterns, pose a threat to the survival of nearly a quarter of the world’s species. The hydrological situation of the MLYRB shows that the dry season is advanced and its duration is prolonged, most notably seen this year with an unusual autumn drought. This new normal state could have severe impacts on migratory birds. Additionally, the construction of the Three Gorges Dam has disrupted the water balance in the Yangtze River Basin and altered the wetland habitats and habitat suitability (Van and Maavara, 2016), which could negatively impact the feeding, nesting, and resting habits of waterbirds during their migration. The habitat quality is an important factor in protecting the overwintering habitat of Siberian cranes (Kellner et al., 1992). In China, human disturbances such as agricultural land conversion, urban expansion, road construction, drainage, diking and damming, nutrient enrichment, and pollution are the primary drivers of wetland loss and degradation, posing a serious threat to waterfowl populations and their survival (Li et al., 2021). Owing to climate change and human disturbances, the future of Siberian cranes is uncertain (Wang, 2020). Therefore, it is urgent to investigate and accurately project the potential distribution of species and their changing trends under different climate scenarios in the future.
In recent years, Species Distribution Models (SDMs) have become an important tool for predicting species distribution and habitat suitability. They use climate, topography, vegetation, and other environmental factors to forecast the potential range of species distribution (Shen et al., 2022), and establish the relationship between species occurrence sites and selected environmental variables (Almeida et al., 2022). The MaxEnt entropy model is widely recognized as one of the most popular and effective models in recent years (Lin et al., 2022). This model offers several advantages, such as ease of use, a low requirement for sample size, and a self-detection function. The model provides a self-detection function that can automatically generate ROC curves to simulate and test the model’s predictions. Additionally, when evaluating and predicting animal habitats, only “occurrence point” data is required (Yu et al., 2019). Compared with other models, MaxEnt’s predictive performance is consistently competitive with the highest performance methods (Elith et al., 2006), it combines machine learning and maximum entropy principles which can accurately predict the potential distribution of species (Su et al., 2021). Therefore, it has been widely used for many purposes in biogeography, conservation biology, ecology, and other fields (Li et al., 2020).
In this study, the MaxEnt algorithm model and the latest shared socioeconomic pathways (SSPs) from the Coupled Model Intercomparison Project in its sixth phase (CMIP6) were employed to project the habitat suitability distribution of Siberian cranes. The main objective of this study was to address the following questions: 1) Has the habitat quality of Siberian cranes in the MLYRB changed significantly during the past few decades? 2) What factors will have great impacts on the habitat quality of Siberian cranes under the changing environment? 3) How will the habitat quality of Siberian cranes evolve under different scenarios in the future? These questions are of great significance for the protection of waterfowl habitat quality in the MLYRB under the background of global warming.
2 Materials and methods
2.1 Study area
The MLYRB (106°7′-121°47′E, 24°30′-33°54′N) region is situated in Eastern China, encompassing areas in Hunan, Jiangxi, Hubei, southern Anhui, southern Jiangsu provinces, and the Shanghai municipality, with total coverage of 8.00 × 105 km2 (Figure 1). This region experiences a humid subtropical monsoon climate with four distinct seasons and abundant rainfall, with average annual temperatures ranging from 14°C to 18°C and average annual precipitation of around 1,300 mm. China’s terrain is characterized by higher elevations in the west and lower elevations in the east, with a roughly three-tiered, step-like distribution. Located in the third ladder of China, the MLYRB is characterized by its flat terrain, with a mixture of low mountains, hills, and plains (Deng et al., 2020). Some parts of the region are low-lying, with elevations of fewer than 50 m (Cui et al., 2013). This region boasts the largest concentration of freshwater lakes in China, including the five famous freshwater lakes: Poyang, Dongting, Taihu, Chaohu, and Hongze. There are as many as 100 lakes (area ≥10 km2) covering a total area of 10,593.4 km2. Additionally, the wetlands within the region span over 7.70 × 104 km2, accounting for 14.42% of the total wetland area in China (Geng, 2014).
The wetland in this study area is a unique hybrid of artificial and natural ecosystems and is located on the East Asia-Australasia Flyway, a critical migratory route for overwintering birds. The population of wintering waterfowl in the Poyang Lake wetland is a key indicator of biodiversity richness in the region. This ecosystem provides crucial water resources and habitats for millions of wintering waterbird species from around the world and offers a remarkable service through its diverse and abundant habitats (Huang et al., 2018). It is estimated to host between 30% and 50% of the birds in the Yangtze River wetlands and is the primary wintering ground for the eastern population of Siberian cranes, accounting for approximately 98% of the world’s population (Wang et al., 2017; Zhang et al., 2019).
2.2 Species occurrence data
For this study, the location data (including longitude and latitude) of Siberian cranes in the MLYRB was obtained from various sources, including the Global Biodiversity Information Facility (GBIF, http://www.gbif.org), Center for Agricultural and Biosciences International (CABI, http://www.cabi.org), and National Animal Collection Resource Center (http://museum.ioz.ac.cn/specimens.aspx). A total of 722 distribution records were gathered through these sources and published literature. After eliminating duplicate and invalid points with insufficient geolocation data, 193 sample points were obtained by querying the specific coordinates on the Geonames website (http://www.geonames.org). In addition, the study used three synchronized surveys of wintering waterbirds in the MLYRB organized by the State Forestry Administration of China and the World Wide Fund for Nature (WWF) Beijing office, covering the winters of 2003–2004, 2005–2006, and 2011. The research group also conducted field surveys on the populations of Siberian cranes in the Poyang Lake region, Changjiang Estuary of Chongming Island East Beach, and Jiangyin section of the Yangtze River in Jiangsu Province from 2019 to 2021. For records lacking precise geographic coordinates, the team used Omap software and Online Baidu Maps (https://lbsyun.baidu.com/) to obtain approximate location information and collected 64 distribution points in total. In the end, a total of 257 species occurrence records of Siberian cranes were used to establish the MaxEnt model. The sample point data was saved in a CSV file with three columns: species name, longitude, and latitude coordinate values (Zhang et al., 2022).
2.3 Environmental variables data
Environmental variables are the basis of a suitable habitat distribution model, which plays a key role in influencing the distribution of Siberian cranes (Li and Fan et al., 2020). Nineteen bioclimatic variables have been identified as the most critical factors in determining the potential distribution of the species (Nurhussen et al., 2021). Additionally, altitude plays a crucial role in species distribution and has been considered in this study. Therefore, data for 19 bioclimatic variables (for the period 1970–2000 and the future from 2021 to 2060) was obtained from the Global Climate Database with a spatial resolution of 30 arc seconds (approximately 1 km). Similarly, the elevation data was obtained from WorldClim v2.1 with a spatial resolution of 30 m, and terrain variables (slope and aspect) were calculated using the elevation layer in ArcGIS 10.2. To define the distribution niche of the Siberian crane, these variables were combined with the Normalized difference vegetation index (NDVI) factors and used to build the model. This study employed the current NDVI variables selected from the GIMMS NDVIl3g dataset, which covered the period 1982 to 2000 with a spatial resolution of 0.08333°. The future NDVI dataset was generated by simulating the 2021–2060 NPP dataset using the LPJ global vegetation dynamics model. The NPP data was then converted to the required NDVI data (Tian et al., 2021; Kong et al., 2022). The data were stored in NetCDF format along with related files. Lastly, the NC format was transformed into raster data, and the mean value of the vegetation index was obtained through the maximum synthesis method.
Before constructing the model, the data of the 23 variables were standardized. To maintain consistency in the spatial resolution across all variables, the raster size of 23 environmental variable layers was uniformly resampled to 0.08333° (Dai et al., 2017). And the coordinate system was projected uniformly to WGS-1984-UTM-Zone-49N, along with setting a uniform layer boundary, all accomplished within the ArcGIS software. The environmental variable layers were then converted to ASCII format for use in MaxEnt modeling. To minimize the influence of multicollinearity and overfitting in MaxEnt (Fotheringham and Oshan, 2016), a Pearson correlation analysis of the 23 variables was performed in SPSS 20 to analyze their relationship with the distribution habitats of the Siberian crane. As some of the variables were highly correlated, dimensionality reduction was necessary to avoid spurious results and optimize the final fitted model. If the correlation coefficient of the two variables was greater than 0.80 (Dormann et al., 2013), the variable with lower ecological significance was removed. Ultimately, only 12 environmental variables were retained for MaxEnt modeling (Table 1).
2.4 Selection of environmental variables
Global Climate Models (GCMs) are widely considered the most robust tools for simulating climate change and projecting future trends. Based on these models, numerous studies have made predictions of changes in global and regional climates, as well as the associated extremes, under different concentration pathways represented by the Shared Socioeconomic Pathways (SSPs) (Wang et al., 2009). The current stage of the Coupled Model Intercomparison Project (CMIP) under the World Climate Research Program (WRCP) is the sixth, and it is the most comprehensive so far with a large number of participating models, well-designed experiments, and a vast amount of data. CMIP6 provides a valuable reference for understanding the patterns of past climate change, forecasting future climate changes, and evaluating the impacts of climate change (Zhou et al., 2019). Our study concentrates on the current climatological period (1970–2000), and the future for two periods, including the 2030s (2021–2040), and 2050s (2041–2060). The future climate scenario data are derived from the CMIP6 downscaled projections, and we have selected four SSP scenarios (SSP1-2.6, SSP2-4.5, SSP3-7.0, and SSP5-8.5) from CMIP6 for our analysis (Zhou et al., 2021). The numbers after the dashes indicate the radiative forcing levels. Radiative forcing level refers to the quantification of the impact of an external forcing, such as changes in solar radiation or anthropogenic greenhouse gas emissions, on the energy balance of the Earth’s atmosphere system (Tebaldi et al., 2021). It represents the net radiative effect of this impact on the global climate system and is a crucial indicator for measuring the contribution of various factors to climate change. Radiative forcing levels are typically measured in units of positive or negative forcing per unit area. In our analysis, we have selected three CMIP6 models from the World Climate Center, including BCC-CSM2-MR, CanESM5, and CNRM-CM6-1, to compare the most appropriate climate change simulations for China (Table 2). This study also utilized Matlab programming software to plot the annual average temperature and precipitation changes in the wintering areas of Siberian cranes. The plots were generated under different SSPs scenario (SSP1-2.6, SSP2-4.5, SSP3-7.0, and SSP5-8.5) by employing CMIP6 model data from two time periods: 1970 to 2014 and 2015 to 2060. The aim was to infer the rationality of the distribution of the suitable habitat for Siberian cranes.
In our analysis of the impact of climate change on the distribution pattern of Siberian cranes, we used climate data from three different periods, corresponding to the four Shared Socioeconomic Pathways (SSPs) (Table 3). The climate data were acquired with a spatial resolution of 2.5 arc minutes (about 5 km × 5 km) and were restricted to the geographical area of the (MLYRB). All the data processing and analysis were conducted using ArcGIS 10.2 software.
2.5 Model establishment and evaluation methods
MaxEnt is a complicated machine learning approach based on the principle of maximum entropy, which can be run through the data of species occurrence and environmental variables. MaxEnt software (version 3.4.1), which is freely available on the website (https://biodiversityinformatics.amnh.org/open_source/maxent/) was selected for this study. There are extensive opinions on the setting of the parameter “random test percentage” in the model (Yuan et al., 2020). Not all model parameters are set uniformly, and some articles suggest that they should be set according to the situation of each specific study. In this study, the following parameters were set before the model runs the maximum background points = 10,000; to ensure the stability of the model prediction, the replicates = 10; the remaining parameters were set to the default values of the MaxEnt model. This setup has been considered reasonable and effective for a wide range of niche studies (Phillips and Dudik, 2008). In the process of modeling, 75% of the 257 distribution data samples of Siberian cranes were randomly selected as the training data to calibrate the model, and 25% of the samples were used as the testing data for accuracy checking.
In this study, we simulated the suitable habitat for the Siberian crane across the entire global range, including both wintering and breeding areas (Figure 2). We performed geographic segmentation on the simulated global region and ultimately obtained the suitable habitat distribution of the Siberian crane’s wintering area in the MLYRB. The jackknife test and the creation of response curves were selected to evaluate environmental variable contributions and thresholds to potentially suitable areas for Siberian cranes in the MLYRB. The receiver operating characteristic (ROC) threshold values were used to cut the modeled suitability matrices (Naeem et al., 2018). This approach determines the balance between omission and commission errors while determining the spatial distributional ranges of a species (Silva et al., 2014), to evaluate the prediction accuracy of this model. The area enclosed by the curve and the abscissa is the area under the curve (AUC) (Nurhussen et al., 2021). The average training AUC value is automatically calculated when the MaxEnt model is repeatedly run 10 times. The AUC ranged from 0 to 1, with higher values corresponding to a better prediction effect of the model (Fielding, 1997). An AUC value of 0.5–0.6 meant that the simulation effect of the model was invalid; 0.6–0.7 meant that it was poor; 0.7–0.8 meant that it was average; 0.8–0.9 meant that it was good; 0.9–1 meant that it was excellent (Swets, 1988).
The model leverages non-random connections between factors such as climate, altitude, vegetation, and other environmental variables to predict the distribution of suitable habitats for species. By analyzing species distribution points and study areas, the model makes accurate inferences and predictions. Under certain restrictions, the model determines the probability with the highest entropy, which optimizes its prediction of species habitats (Jimenez-Valverde, 2012).
2.6 Classification of current and future potential habitats
After modeling the potentially suitable areas, we used maps and spatial analysis tools available in ArcGIS 10.2 software to superimpose the simulation results of the MaxEnt model and classify the results of the probability of existence. The maps of current and future suitable areas that were generated by the model had values of existence: highly suitable (0.5 ≤ probability of presence ≤1), medially suitable (0.3 ≤ probability of presence <0.5), slightly suitable (0.1 ≤ probability of presence <0.3), and unsuitable (probability of presence <0.1), based on the predicted probability of presence (Gao et al., 2018). Finally, the ecological suitability distribution of waterbirds in the MLYRB based on the main ecological factors was mapped.
3 Results
3.1 Critical contributing environmental variables
The resulting model of the current potential distribution area of the Siberian crane in the MLYRB had a mean AUC of 0.939, indicating a high-quality model (Figure 3). The resulting model was based on twelve environmental variables. The mean AUC values for the MaxEnt models of Siberian cranes were significantly higher than the random prediction value (0.5), with a standard deviation of 0.001. This demonstrates the prediction results to be highly accurate and the potential distribution area generated by MaxEnt to be trustworthy.
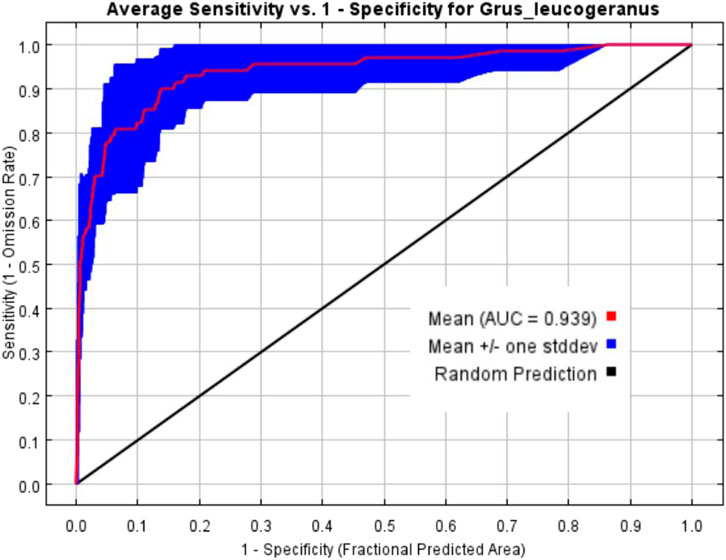
FIGURE 3. Average area under the curve (AUC) for 10 replicates MaxEnt run. The red line is the average value and the blue bar represents plus and minus one standard deviation.
The Jackknife analysis and contribution rate results (Figures 4, 5) revealed the contribution rate of environmental variables. Precipitation of the driest quarter (Bio17) and altitude contributed significantly to the model in contrast with other variables, accounting for 42.5%, and 40.2%, respectively. These two impact factors alone account for more than 80% of the contribution. Precipitation of the warmest quarter (Bio18), Precipitation seasonality (Bio15), temperature annual range (Bio7), mean temperature of the warmest quarter (bio10), aspect and mean temperature of the coldest quarter (Bio11) were the least important factors. The contribution of these factors combined is less than 5%. The other four parameters: mean temperature of the wettest quarter (Bio8), slope, NDVI (Normalized Difference Vegetation Index), and precipitation of the wettest quarter (Bio16) were also substantial contributors to the model, and the cumulative contribution rate reached 13.5%. In summary, precipitation and altitude were the main factors that limited the selection of suitable habitat sites for Siberian cranes.
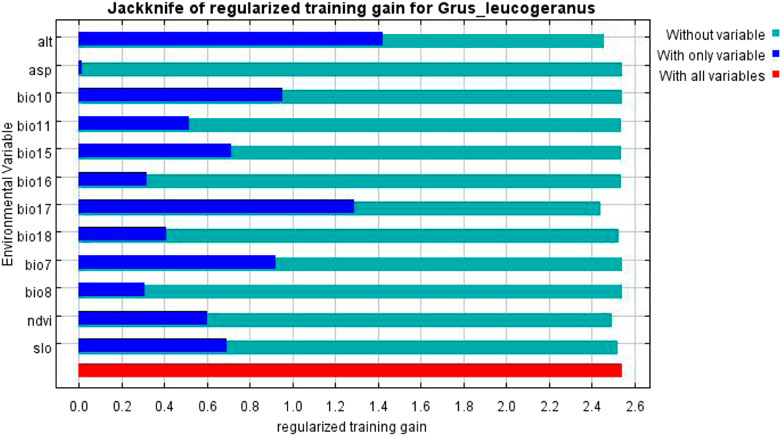
FIGURE 4. Jacknife test of variable importance for Siberian cranes. The blue, aqua, and red bars represent the results of the model created with each individual, all remaining variables, and all the variables respectively.
The probability of the appearance of Siberian cranes is consistent with the suitability index of their habitat. According to the response curve of precipitation of the driest quarter (Bio17), altitude (Alt), and mean temperature of the wettest quarter (Bio8) (Figure 6), the most suitable driest month precipitation for the wintering habitat of Siberian cranes is 160–170 mm, with suitability approaching 0 when it is less than 50 mm, and reaching the peak suitability at 170 mm. The altitude of 0–50 m is the most suitable for Siberian cranes, and the optimum suitability index for the average temperature of the wettest season is around 22°C.
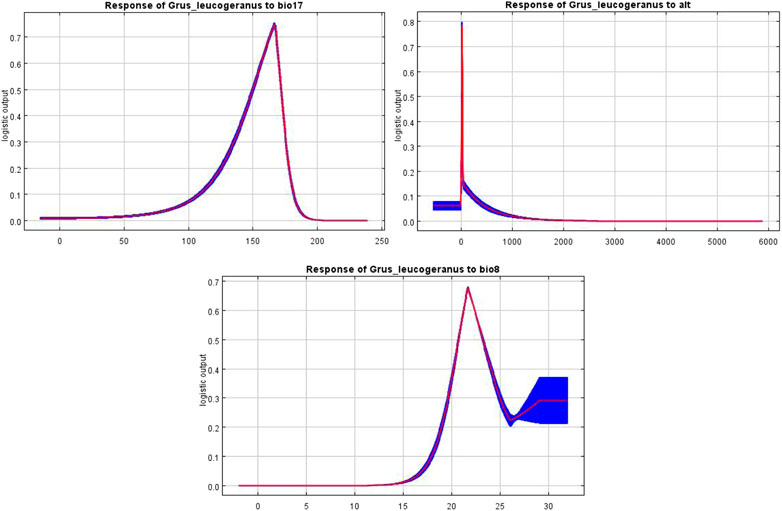
FIGURE 6. Response curves of three major environmental variables in the niche model of Siberian cranes.
3.2 Current potential distribution
The current potential distribution area of the Siberian crane has displayed in Figure 7. The simulation results showed that under current climatic conditions, the total suitable habitat area for the Siberian crane covers 302,170 km2, with the highly suitable area of 54,749 km2, the medially suitable area of 81,484 km2, and the slightly suitable area of 165,937 km2. The wintering sites of the Siberian crane are primarily located in Jiangxi’s Poyang Lake and Hunan’s Dongting Lake, with scattered individuals found in Yancheng and Dongtai in Jiangsu Province, Yuyao in Zhejiang Province, and coastal wetlands in Zhejiang, Shanghai, and Jiangsu. The main high-suitability area is in the Poyang Lake Basin, with the medially and slightly suitable areas primarily located around the outer Poyang Lake Basin. Areas far from the Yangtze River and lake wetlands show clear evidence of human activity, making them unsuitable for the Siberian crane’s feeding, reproduction, migration, and overwintering. The 257 Siberian crane data points were collected throughout China, with 25% of the occurrence points concentrated in highly suitable areas. There was also a small amount of distribution in the slightly and medially suitable areas, with no distribution in the unsuitable areas.
3.3 Suitable areas of Siberian cranes under future climate change scenarios
Compared to the period of 1970–2000, the suitable habitat area for cranes is expected to decrease in both future periods (2021–2040 and 2041–2060). Due to the large number of legends, only the legend for the period of 2021–2040 will be shown here. Figure 8 displays the projected distribution of suitable sites for the Siberian crane from 2021 to 2040 under various global climate change scenarios (SSP1-2.6, SSP2-4.5, SSP3-7.0, and SSP5-8.5). Suitability values (0–1) were classified into five classes. According to the prediction results of the ecological niche model. Climate warming has largely suppressed the expansion of potentially suitable habitats for the Siberian crane in the MLYRB region. The future environmental conditions will be even less conducive to the growth of this species than currently observed; therefore, the geographical expansion of the Siberian crane will be difficult to achieve under the influence of future climate conditions.
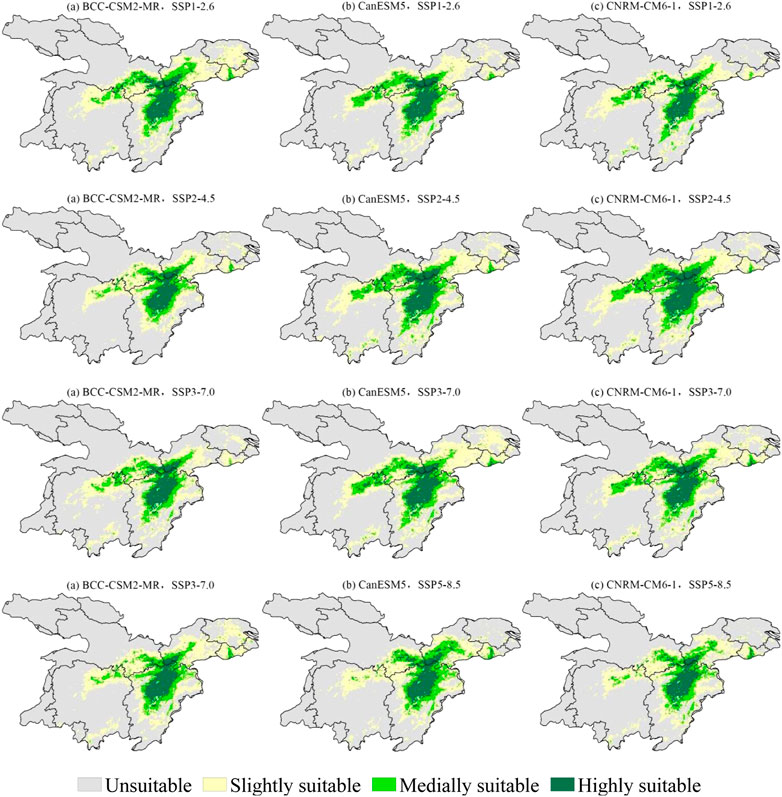
FIGURE 8. Prediction maps for the potential distribution of Siberian crane under future 2030s climate conditions using selected exemplar global climate models BCC-CSM2-MR, CanESM5, and CNRM-CM6-1, under four scenarios of Shared Socioeconomic Pathways (SSP1-2.6, SSP2-4.5, SSP3-7.0, and SSP5-8.5).
This study found that the geographical distribution of different types of suitable habitats under four climate change scenarios changes with climate warming. Except for unsuitable regions, the distribution range of the other three types of suitable habitats generally shows a decreasing trend. Compared with the currently suitable areas, they all show a trend of shifting toward the Poyang Lake wetland, high-latitude areas, and eastern high-altitude areas. The prediction map shows that the suitable areas in Hunan and Jiangxi have increased. In Jiangsu, Zhejiang, and other regions, the distribution of scattered moderately suitable areas has significantly decreased, and the medially suitable areas distributed in eastern Jiangsu, Shanghai, and Anhui have almost completely disappeared. At the same time, we found that compared with the current potential suitable habitat distribution, the distribution of suitable habitats is also more concentrated.
3.4 Future changes in suitable habitats
Comparing the four climatic scenarios, we found that under the SSP1-2.6 low-emission scenario, the area of the Yangtze River Estuary at high latitudes will be increasingly lost, while under the SSP5-8.5 scenario, the suitable range area along the Dongting Lake basin would increase (Figure 9). From 2021 to 2040, the prediction results under different scenarios of the BCC-CSM2-MR model showed that: under the SSP1-2.6 scenario, the suitable habitat area for Siberian cranes decreased by 3.45 × 104 km2, whereas the obtained marginal suitable area was 13.18 × 104 km2. Under the SSP2-4.5 scenario, the suitable habitat area of the Siberian crane lost 1.91 × 104 km2 and gained 19.72 × 104 km2. Under the SSP3-7.0 scenario, the suitable habitat area of the Siberian crane lost 3.50 × 104 km2 and gained 17.29 × 104 km2, whereas, under the SSP5-8.5 scenario, the suitable habitat area of the Siberian crane lost 3.39 × 104 km2 and gained 15.31 × 104 km2. Among them, SSP2-4.5 had the lowest loss area and highest gain area.
The prediction results of the CanESM5 model under the different scenarios showed that under the SSP1-2.6 scenario, the suitable habitat area for the Siberian crane was reduced by 3.05 × 104 km2, while the obtained marginal suitable area was 16.03 × 104 km2. Under the SSP2-4.5 scenario, the suitable habitat area for the Siberian crane decreased by 4.37 × 104 km2, and 11.36 × 104 km2 was gained. Under the SSP3-7.0 scenario, the suitable habitat area of the Siberian crane lost 3.63 × 104 km2 and gained 11.11 × 104 km2. Under the SSP5-8.5 scenario, the suitable habitat area of the Siberian crane lost 3.72 × 104 km2 and gained 5.92 × 104 km2. Among them, SSP1-2.6 had the lowest loss area and highest gain area.
The prediction results of the CNRM-CM6-1 model under different scenarios revealed that under the SSP1-2.6 scenario, the suitable habitat area for the Siberian crane decreased by 2.29 × 104 km2, and the obtained marginal suitable area was 17.49 × 104 km2. Under the SSP2-4.5 scenario, the suitable habitat area for the Siberian crane was reduced by 4.52 × 104 km2, albeit 10.40 × 104 km2 was gained. Under the SSP3-7.0 scenario, the suitable habitat area of the Siberian crane lost 3.22 × 104 km2 and gained 12.84 × 104 km2. Further, under the SSP5-8.5 scenario, the suitable habitat area of the Siberian crane decreased by 2.87 × 104 km2 but increased by 17.88 × 104 km2. Among them, SSP1-2.6 had the lowest loss area, while SSP5-8.5 had the highest gain area.
The results of the predictions for the Siberian crane’s suitable habitat under four Shared Socioeconomic Pathways (SSPs) and three models (BCC-CSM2-MR, CanESM5, and CNRM) were analyzed. Overall, the increase in unsuitable habitat distribution under moderate radiative forcing scenarios (SSP2-4.5) was relatively low. However, under low radiative forcing scenarios (SSP1-2.6) and high radiative forcing scenarios (SSP5-8.5), the distribution of unsuitable habitats for Siberian cranes showed a sharp increase, with large fluctuations.
It is estimated that in the next 40 years, the suitable habitat area for Siberian cranes will significantly decrease in all scenarios, and the expansion of slightly suitable habitat areas in very few scenarios can be almost ignored (Figure 10). In the multi-model scenarios SSP1-2.6, SSP2-4.5, SSP3-7.0, and SSP5-8.5 between 2021 and 2040, the total suitable habitat area decreases by around 10.00 × 104 km2. Especially in the SSP2-4.5 scenario of the BCC-CSM2-MR model, the maximum decrease in total suitable habitat area is 10.79 × 104 km2. Between 2041 and 2060, the maximum decrease in total suitable habitat area occurs in the SSP1-2.6 scenario of the BCC-CSM2-MR model, which is 10.50 × 104 km2. The second-largest decrease occurs in the SSP5-8.5 scenario of the CanESM5 model, which is 10.34 × 104 km2.
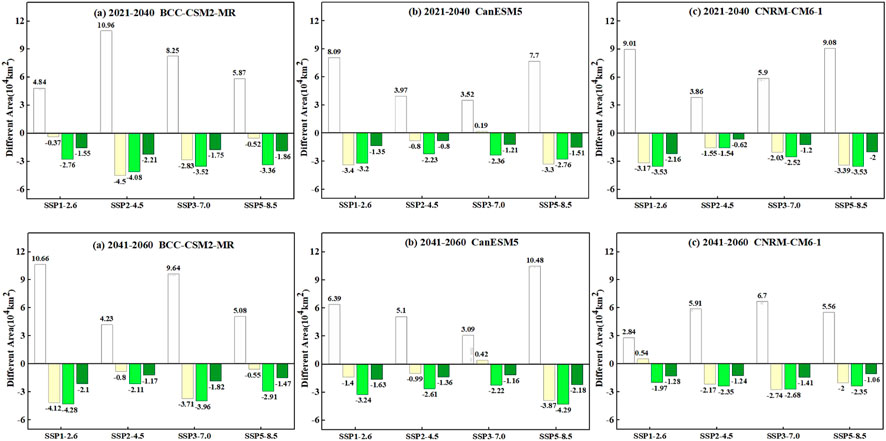
FIGURE 10. Percentage of change in future suitable areas compared with currently suitable areas under the four shared socioeconomic pathways: SSP1-2.6; SSP2-4.5; SSP3-7.0; SSP5-8.5.
Table 4 shows the highest percentage of total suitable area decline in various scenarios of multiple models from 2021 to 2060 compared to 1970–2000. The total suitable area is the sum of the slightly, medially, and highly suitable areas, whereas the total suitable area of the Siberian crane in 1970–2000 was 302,170 km2. Compared with 1970–2000, in the future (2021–2060) under different models and scenarios, the maximum decrease in the suitable area of Siberian cranes is as follows:
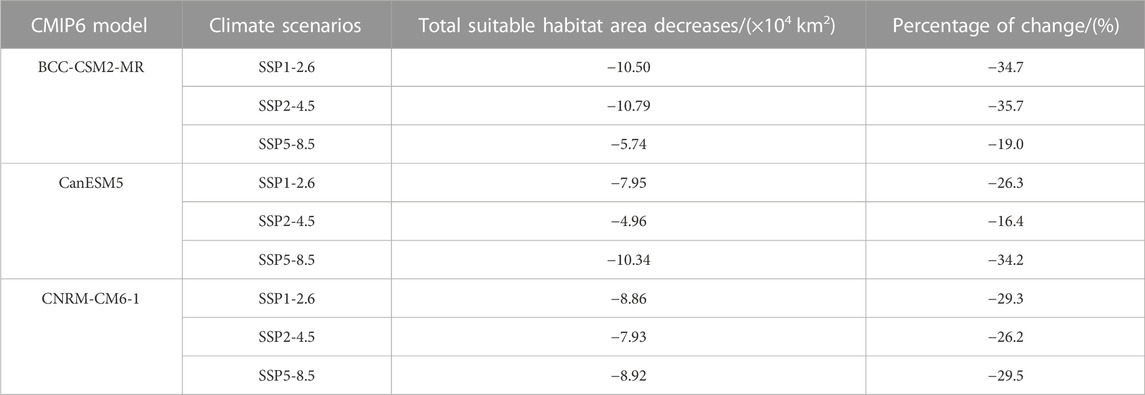
TABLE 4. The maximum decrease in the suitable habitat area for Siberian cranes between 2021 and 2060 under multiple models and scenarios, compared to the period of 1970–2000.
In the BCC-CSM2-MR model SSP1-2.6 scenario, the highest decline in the total suitable habitat area of Siberian cranes is approximately 10.50 × 104 km2, accounting for 34.7% of the total. In the CanESM5 model SSP1-2.6 scenario, the highest decline is approximately 7.95 × 104 km2, accounting for 26.3% of the total. In the CNRM-CM6-1 model SSP1-2.6 scenario, the highest decline is approximately 8.86 × 104 km2, accounting for 29.3% of the total; In the BCC-CSM2-MR model SSP2-4.5 scenario, the highest decline in total suitable habitat area of Siberian cranes is approximately 10.79 × 104 km2, accounting for 35.7%, which is the largest decline percentage among all scenarios of different models. In the CanESM5 model SSP2-4.5 scenario, the highest decline is approximately 4.96 × 104 km2, accounting for 16.4%, which is the smallest decline percentage among all scenarios of different models. In the CNRM-CM6-1 model SSP2-4.5 scenario, the highest decline is approximately 7.93 × 104 km2, accounting for 26.2% of the total; In the BCC-CSM2-MR model SSP5-8.5 scenario, the highest decline in total suitable habitat area of Siberian cranes is approximately 5.74 × 104 km2, accounting for 19.0%. In the CanESM5 model SSP5-8.5 scenario, the highest decline is approximately 10.34 × 104 km2, accounting for 34.2% of the total. In the CNRM-CM6-1 model SSP5-8.5 scenario, the highest decline is approximately 8.92 × 104 km2, accounting for 29.5% of the total.
3.5 Climate change and future projections
The trend of annual average temperature and precipitation changes in the wintering area of the Siberian cranes in the MLYRB is shown in Figure 11. The SSP1-2.6 and SSP3-7.0 scenario data for the CNRM-CM6-1 model were missing and not displayed in the legend. From 2015 to 2060, the temperature rise in the MLYRB in all model scenarios ranges from 2.4°C to 4.6°C, which is 0.38°C–2.42°C higher than the temperature rise range from 1970 to 2014. The increase in temperature is within 30%, and the increase in precipitation is within 35%.
In the BCC-CSM2-MR model, the upward trend of temperature is more pronounced than in the other two models, especially since the increase in future temperature is significantly higher. In all models, both the historical and future periods show that the temperature increase is smallest under the SSP1-2.6 scenario, and the change in precipitation is relatively insignificant, while under the SSP5-8.5 scenario, the temperature increase is most significant, and the fluctuation trend of precipitation is the largest, slightly higher than under the SSP2-4.5 scenario, and the warming amplitude in the future period is significantly higher than that in the historical period. In addition to changes in annual average temperature and precipitation, significant differences were also observed in extreme temperature and precipitation.
4 Discussion
4.1 Siberian cranes’ habitat quality declined along the MLYRB
Recent studies have focused on the impact of large-scale environmental changes on the migration patterns of wintering waterbirds (Van Eerden et al., 2005). The global average temperature has risen by approximately 0.8°C–1.3°C over the past 100 years, highlighting a clear trend of climate warming (Li et al., 2022). The increase in minimum temperatures has affected the wintering ranges and migration routes of migratory waterbirds (Lehikoinen et al., 2013). In this study, we compared the changes in the suitable habitat area for Siberian cranes between 1970 and 2000 and 2030s and found that almost all scenarios showed a significant expansion of unsuitable areas and a decrease in suitable areas. Except for the SSP3-7.0 scenario, all other scenarios showed a significant decline in the slightly, medially, and highly suitable areas for Siberian cranes, with the largest decline occurring in the BCC-CSM2-MR model SSP2-4.5 scenario, where the suitable habitat area for Siberian cranes decreased to a maximum of 10.79 × 104 km2. In the other two models’ SSP2-4.5 scenarios, the trend of decreasing suitable habitat areas for Siberian cranes was more moderate. Among them, the CanESM5 model SSP3-7.0 scenario had the lowest degree of decline in suitable habitat area for Siberian cranes, at 3.83 × 104 km2, with even a small increase in the slightly suitable habitat area. When comparing the changes in suitable habitat area for Siberian cranes between 1970 and 2000 and 2050s, except for the SSP2-4.5 scenario, all other scenarios showed a trend of sharp changes in the suitable habitat area. Especially in the BCC-CSM2-MR model SSP1-2.6 and SSP3-7.0 scenarios, as well as the CanESM5 model SSP5-8.5 scenario, the suitable habitat area decreased by around 10.00 × 104 km2. In the two future periods, the total suitable habitat area for Siberian cranes sharply decreased, and a trend of migrating to higher latitudes or elevations was observed. Stattersfield et al. (2008) believe that waterbird species richness increases at lower latitudes due to the increase in latitudinal diversity gradients, reflecting increases in temperature, water availability, ecosystem productivity, and habitat heterogeneity.
The results show that the temperature increase in the MLYRB will be within 30%, and the increase in precipitation will be within 35% in the future. Due to climate change, the significant interannual variations in the annual average temperature and precipitation will lead to more frequent floods and droughts, which will further exacerbate habitat vulnerability. These birds are particularly susceptible to changes in the climate, as their migration patterns can be closely tied to these changes (Dunn et al., 2009). As global warming impacts the entire East Asia-Australasian migratory flyway, the wintering waterbirds, being highly sensitive to weather fluctuations, may alter their migration routes and distribution patterns (Rainio et al., 2006). The fluctuation and changes in future temperature and precipitation may have a significant impact on the distribution of suitable habitats for waterbirds in the research area, and it is worth further in-depth research. Other environmental changes, such as the degradation of migratory bird habitats were greatly exacerbated by changes in land use under the influence of human activities and the discharge of wastewater in the watershed. The unnatural conversion, reduction, and destruction of natural wetlands have resulted in a decline in wetland ecosystem services, causing their functions as a food source, migratory stopover, and overwintering breeding grounds for species to weaken or even disappear. This includes the conversion of natural wetlands to artificial wetlands, then to cropland, and eventually to land for construction. Since 1950, there has been a large-scale lake enclosure to create fields in the MLYRB, resulting in the disappearance of over 1,000 lakes (with a total area of 13,000 km2) (Yang et al., 2022). In the past century, over 60% of the wetlands in the MLYRB have been replaced by urbanization and agricultural reclamation (Tian et al., 2014). Additionally, with the rapid population and economic development in recent decades, the lakes in the basin are facing increasing water pollution and eutrophication, which is also a great threat to the decrease of habitat quantity (Yang et al., 2015).
4.2 Simulation of habitat quality for Siberian cranes and differences in results
The results of the study indicate that the potentially suitable habitat for Siberian cranes in the MLYRB will decrease and become more concentrated under the four scenarios examined, as compared to the current climate. This is due to the impacts of global warming, which is causing a shift in the distribution of suitable habitats for this species. The study utilized all available data on species occurrence and environmental factors that influence their distribution to reach these conclusion.
The main factors that influenced the potential distribution of the Siberian crane in the study area were precipitation of the driest quarter (42.5%), altitude (40.2%), and mean temperature of the wettest quarter (4%), with a total contribution rate of 86.7%. The study showed that precipitation of the driest quarter and altitude had the highest contribution rate, accounting for approximately 80% of the total proportion. Among the bioclimatic variables, precipitation was the main environmental factor affecting the distribution of the Siberian crane, in addition to altitude. This is consistent with the findings of Luo et al. (2021), who showed that seasonal rainfall variation and annual minimum temperature were the most important climatic factors determining the distribution of waterbirds. The temperature was also an important influence, particularly in the distribution of migratory birds (Liang et al., 2021). Studies have shown that a combination of temperature and terrain variability at the best resolution is the most important predictor of global bird species richness (Davies et al., 2007). Further research is needed to determine if the temperature can delay or prevent the continuous migration of the Siberian crane to higher altitudes.
4.3 Potential reasons for changes in the habitat quality of wintering waterbirds
The current crisis for wintering waterbirds along the East Asia-Australasian migration route stems from multiple causes, among which shrinking wetland areas were one of the main drivers behind the loss of wintering waterbird biodiversity (Smart and Gill, 2003). The distribution of Siberian cranes was also impacted by a variety of practical factors, including interactions between species, food abundance, geological and geomorphological features, intensification of biological invasion, changes in hydrology and water ecology, anthropogenic activities, including land-use change, Critically Endangered species directly threatened by land-use change, and other variables. Comprehensive data on these impact factors were not available at the time of this study, which was a limitation.
We should be focused on changes in hydrology and water ecology, as well as the impacts of anthropogenic activities on habitat selection by Siberian cranes. For example, the hydrological conditions of foraging habitats (e.g., hydrological connectivity between different habitats, water depth, etc.) can serve as important predictors of foraging habitat selection for wading birds. The leg lengths of wading birds can determine the maximum depth to which they can forage, which limits the viability of foraging habitats (Lantz, 2008). Jiang et al. (2014) used water levels and a habitat map of the Xingzi measuring station to investigate the impacts of water levels on the spatial patterns of potentially suitable habitats for Siberian cranes, which revealed that increased water levels led to a reduction in their habitat area. Water levels higher than 13 m had certain negative impacts on the Siberian crane; however, arid environments are not necessarily beneficial for this species (Zou et al., 2021). To maintain a certain number of mudflats and shallow water, the optimal water level of the Poyang Lake wetland should be ∼12 m, which is conducive to the wintering of the Siberian crane. Furthermore, receding water levels may induce habitat selection by Siberian cranes to change from wetlands to highlands (Zhang et al., 2013).
In this study, the focus was on exploring the impact of abiotic factors, such as climate, topography, and vegetation index (NDVI), on the distribution of Siberian cranes in the MLYRB. Currently, the acquisition of NDVI data is mainly based on satellite data. If it is possible to use LIDAR data, more detailed data can be obtained, which may lead to better results (Yang and Wen et al., 2022a; Yang and Wen et al., 2022b). It was noted that although the Siberian crane is a migratory bird that chooses to winter in the MLYRB, its distribution is not limited to the wetlands around Poyang and Dongting Lakes, but also extends to surrounding farmland and coastal areas. The complexity of the distribution pattern of the Siberian crane, which is influenced by various factors such as environment, food sources, and human activities, made it challenging to choose relevant variables for the model. Only the abiotic factors were considered in this study, and the model did not include information on biological factors such as human influences, since the Siberian crane has specialized habitat requirements and a strong connection to shallow wetlands. However, due to the lack of accurate and high-resolution data, the study did not simulate the effects of hydrological regimes. To have a more comprehensive understanding of the impact of climate change on migratory birds, a database of temperature-humidity-hydrological indices under topographic conditions should be established. In conclusion, further research is necessary to examine the effects of climate change on other endemic bird populations and threatened species, such as ducks and shorebirds.
The rising temperature and decreasing precipitation caused by increasing carbon emissions have a profound impact on bird distribution, with greater temperature increases observed in high latitudes compared to low latitudes and a larger impact under high emission scenarios (Wang et al., 2020). It is imperative to reduce carbon emissions to mitigate this impact. The study found that the key limiting factors for Siberian cranes in the MLYRB are precipitation and altitude, indicating that the loss of biodiversity under the context of global warming is a major global challenge, and all countries must come together to protect the diversity of waterbirds.
5 Conclusion
In this study, we used the MaxEnt algorithm to establish the ecological niche model of the Siberian crane in the MLYRB and found that Precipitation of the driest quarter and altitude, a topographic variable, was the most important environmental factors affecting the suitable habitat distribution of the Siberian crane. The results of the study indicate that the most favorable habitats for Siberian cranes in the MLYRB are situated in the Poyang Lake, Dongting Lake, and Taihu Lake regions. In the BCC-CSM2-MR, CanESM5, and CNRM-CM6-1 models, the suitable habitat area for Siberian cranes is expected to decrease under different SSP scenarios in the future. Compared to 1970–2000, suitable habitat area for Siberian cranes is projected to decrease by 34.7%, 26.3%, and 29.3% under the SSP1-2.6 scenario in BCC-CSM2-MR, CanESM5, and CNRM-CM6-1 models, respectively. Under SSP2-4.5, the highest decrease is projected to be 35.7%, 16.4%, and 26.2%, while under SSP5-8.5, the highest decrease is projected to be 19.0%, 34.2%, and 29.5%, respectively, from 2021 to 2060. To protect Siberian crane habitats from the impacts of climate change, it is crucial to understand how climate change affects ecological networks along the entire EAAF and link social protection groups.
Data availability statement
The raw data supporting the conclusion of this article will be made available by the authors, without undue reservation.
Author contributions
ZZ provided the datasets including the required supporting software required for analyses; JZ cooperated in adjusting the ideas and framework of the paper; BZ and JT conducted the data processing and analysis. All authors contributed to the article and approved the submitted version.
Funding
This work was supported by the Research Funding of China Three Gorges Corporation (Grant number: 202003251); The funder was not involved in the study design, collection, analysis, interpretation of data, the writing of this article, or the decision to submit it for publication. the National Natural Science Foundation of China (Grant number: 41971025); Open Research Foundation of Hubei Key Laboratory of Intelligent Yangtze River and Hydropower Science (Grant number: ZH2002000113); Xu Zhou Science and Technology Project (KC21148), and the Priority Academic Program Development of Jiangsu Higher Education Institutions (PAPD).
Acknowledgments
Thanks to the meteorological data provided by the China Meteorological Administration, and thanks to the valuable comments provided by the editors and three anonymous expert reviewers, which greatly helped to improve the quality of the paper.
Conflict of interest
The authors declare that the research was conducted in the absence of any commercial or financial relationships that could be construed as a potential conflict of interest.
Publisher’s note
All claims expressed in this article are solely those of the authors and do not necessarily represent those of their affiliated organizations, or those of the publisher, the editors and the reviewers. Any product that may be evaluated in this article, or claim that may be made by its manufacturer, is not guaranteed or endorsed by the publisher.
References
Almeida, A. M., Martins, M. J., Campagnolo, M. L., Fernandez, P., Albuquerque, T., Gerassis, S., et al. (2022). Prediction scenarios of past, present, and future environmental suitability for the Mediterranean species Arbutus unedo L. Sci. Rep. 12, 84. doi:10.1038/s41598-021-03996-0
Bamford, M., Watkins, D., Bancroft, W., Tischler, G., and Wahl, J. (2008). Migratory shorebirds of the East asian-australasian flyway: Population estimates and internationally important sites. Wetl. Int. 1, 1–240.
Chen, B., Cui, P., Xu, H., Lu, X., Lei, J., Wu, Y., et al. (2016). Assessing the suitability of habitat for wintering Siberian cranes (Leucogeranus leucogeranus) at different water levels in Poyang Lake area, China. Pol. J. Ecol. 64, 84–97. doi:10.3161/15052249PJE2016.64.1.008
Cui, L., Gao, C. J., Zhao, X. S., Ma, Q. F., Zhang, M. Y., Li, W., et al. (2013). Dynamics of the lakes in the middle and lower reaches of the Yangtze River basin, China, since late nineteenth century. Environ. Monit. Assess. 185, 4005–4018. doi:10.1007/s10661-012-2845-0
Dai, G. H., Yang, J., Huang, C. H., Sun, C. W., Jia, L. M., and Ma, L. Y. (2017). The effects of climate change on the development of tree plantations for biodiesel production in China. Forests 8, 207. doi:10.3390/f8060207
Davies, R. G., Orme, C. D., David, S., Olson, V. A., Thomas, G. H., Ross, S. G., et al. (2007). Topography, energy and the global distribution of bird species richness. Proc. Biol. Sci. 274, 89–97. doi:10.2307/25223911
Deng, Y. J., Yao, S. B., Hou, M. Y., and Zhang, T. Y. (2020). Temporal and spatial variation of vegetation NDVI and its topographic differentiation effect in the middle and upper reaches of the Yangtze River basin. Resour. Environ. Yangtze Basin 29, 66–78.
Dormann, C. F., Elith, J., Bacher, S., Buchmann, C., Carl, G., Carre, G., et al. (2013). Collinearity: A review of methods to deal with it and a simulation study evaluating their performance. Ecography 36, 27–46. doi:10.1111/j.1600-0587.2012.07348.x
Dunn, R. R., Harris, N. C., Colwell, R. K., Koh, L. P., and Sodhi, N. S. (2009). The sixth mass coextinction: Are most endangered species parasites and mutualists? Proc. Biol. Sci. 276, 3037–3045. doi:10.1098/rspb.2009.0413
Elith, J., Graham, C. H., Anderson, R. P., Dudik, M., Ferrier, S., Guisan, A., et al. (2006). Novel methods improve prediction of species' distributions from occurrence data. Ecography 29, 129–151. doi:10.1111/j.2006.0906-7590.04596.x
Fengshan, L. I., Harris, J., and Burnham, J. (2012). Number and distribution of cranes wintering at Poyang Lake, China during 2011–2012. Chin. Birds 3, 180–190. doi:10.5122/cbirds.2012.0027
Fielding, A., and Bell, J. F. (1997). A review of methods for the assessment of prediction errors in conservation presence/absence models. Environ. Conserv. 24, 38–49. doi:10.1017/s0376892997000088
Fotheringham, A. S., and Oshan, T. M. (2016). Geographically weighted regression and multicollinearity: Dispelling the myth. J. Geogr. Syst. 18, 303–329. doi:10.1007/s10109-016-0239-5
Gao, M., Ni, S. P., and Shen, L. (2018). Analysis of global potential ecological suitable producing area for salvia miltiorrhiza based on Maxent model. China Pharm. 29, 2243–2247.
Geng, G. B. (2014). The situation of wetland protection in China is not optimistic – outcomes of the second national wetland resources survey. Green China 3, 8–11. doi:10.3969/j.issn.1672-7789.2014.02.003
Huang, Z. L., Lu, L., Jiao, G. Y., Jiang, J. H., and Ye, Q. (2018). Analysis of the correlations between environmental factors and rare cranes in the Poyang Lake region of China. J. Gt. Lakes. Res. 44, 140–148. doi:10.1016/j.jglr.2017.11.003
Jiang, F., Qi, S. H., Liao, F. Q., Ding, M. J., and Wang, Y. Q. (2014). Vulnerability of Siberian crane habitat to water level in Poyang Lake wetland, China. GIScience Remote Sens. 51, 662–676. doi:10.1080/15481603.2014.978126
Liang, J., Peng, Y. H., Zhu, Z. Q., Li, X., Xing, W. L., Li, X., et al. (2021). Impacts of changing climate on the distribution of migratory birds in China: Habitat change and population centroid shift. Ecol. Indic. 127, 107729. doi:10.1016/J.ECOLIND.2021.107729
Jimenez-Valverde, A. (2012). Insights into the area under the receiver operating characteristic curve (AUC) as a discrimination measure in species distribution modelling. Glob. Ecol. Biogeogr. 21, 498–507. doi:10.1111/j.1466-8238.2011.00683.x
Kanai, Y., Ueta, M., Germogenov, N., Nagendran, M., Mita, N., and Higuchi, H. (2002). Migration routes and important resting areas of Siberian cranes (Grus leucogeranus) between northeastern Siberia and China as revealed by satellite tracking. Biol. Conserv. 106, 339–346. doi:10.1016/S0006-3207(01)00259-2
Kellner, C. J., Brawn, J. D., and Karr, J. R. (1992). What is habitat suitability and how should it be measured? Germany: Springer Netherlands, 476–488. doi:10.1007/978-94-011-2868-1_36
Kong, R., Zhang, Z., Huang, R., Tian, J., Feng, R., and Chen, X. (2022). Projected global warming-induced terrestrial ecosystem carbon across China under SSP scenarios. Ecol. Indic. 139, 108963. doi:10.1016/j.ecolind.2022.108963
Lantz, S. (2008). The effects of water depth and vegetation on wading bird foraging habitat selection and foraging success in the Everglades. Diss. Theses - Gradworks 74, 268–277. doi:10.1097/00007890-200207270-00019
Lehikoinen, A., Jaatinen, K., Vahatalo, A. V., Clausen, P., Crowe, O., Deceuninckk, B., et al. (2013). Rapid climate driven shifts in wintering distributions of three common waterbird species. Glob. Chang. Biol. 19, 2071–2081. doi:10.1111/gcb.12200
Li, J., Fan, G., and He, Y. (2020a). Predicting the current and future distribution of three Coptis herbs in China under climate change conditions, using the Maxent model and chemical analysis. Sci. Total Environ. 698, 134141. doi:10.1016/j.scitotenv.2019.134141
Li, L. H., Zhang, Y., Zhou, T. J., Wang, K. C., Wang, C., Wang, T., et al. (2022). Mitigation of China's carbon neutrality to global warming. Nat. Commun. 13, 5315. doi:10.1038/s41467-022-33047-9
Li, Y. D., Wieland, H., Yong, C. C., Pavel, K., Olga, K., Alexander, K., et al. (2021). The state of migratory landbirds in the East asian flyway: Distributions, threats, and conservation needs. Front. Ecol. Evol. 9, 613172. doi:10.3389/fevo.2021.613172
Li, Y. C., Li, M. Y., Li, C., and Liu, Z. Z. (2020b). Optimized maxent model predictions of climate change impacts on the suitable distribution of Cunninghamia lanceolata in China. Forests 11, 302. doi:10.3390/f11030302
Lin, S. Y., Zhao, L., and Feng, J. L. (2022). Predicted changes in the distribution of Antarctic krill in the Cosmonaut Sea under future climate change scenarios. Ecol. Indic. 142, 109234. doi:10.1016/j.ecolind.2022.109234
Luo, Y. Q., Hu, H. J., Xu, Z. C., Ding, Z. F., Yang, X. T., Chen, Q. J., et al. (2021). Hotspots of the waterbirds diversity in guangdong-Hong Kong-Macao greater bay area based on maxent model. Acta Ecol. Sin. 41, 7589–7598.
Mackinnon, J., Verkuil, Y. I., and Murray, N. J. (2012). Iucn situation analysis on east and southeast asian intertidal habitats, with particular reference to the yellow sea (including the bohai sea). Gland, Switzerland: International Union for Conservation of Nature.
Matson, J., Woodman, N., Castroarellano, I., Grammont, H. D., Barrera, G., Floresvillela, O., et al. (2011). Iucn red list of threatened species. United Kingdom: International Union for Conservation of Nature.
Naeem, M., Yuan, X., Huang, J., and An, J. (2018). Habitat suitability for the invasion of Bombus terrestris in EastEast Asian countries: A case study of spatial overlap with local Chinese bumblebees. Sci. Rep. 8, 11035. doi:10.1038/s41598-018-29414-6
Nurhussen, A., Clement, A., and Worku, Z. (2021). Species Distribution Modelling performance and its implication for Sentinel-2-based prediction of invasive Prosopis juliflora in lower Awash River basin, Ethiopia. Ecol. Process 10, 250–265. doi:10.1186/S13717-021-00285-6
Phillips, S. J., and Dudik, M. (2008). Modeling of species distributions with maxent: New extensions and a comprehensive evaluation. Ecography 31, 161–175. doi:10.1111/j.0906-7590.2008.5203.x
Rainio, K., Laaksonen, T., Ahola, M., Vähätalo, A. V., and Lehikoinen, E. (2006). Climatic responses in spring migration of boreal and arctic birds in relation to wintering area and taxonomy. J. Avian Biol. 37, 507–515. doi:10.1111/j.0908-8857.2006.03740.x
Shen, Y. F., Tu, Z. H., Zhang, Y. L., Zhong, W. P., Xia, H., Hao, Z. Y., et al. (2022). Predicting the impact of climate change on the distribution of two relict Liriodendron species by coupling the Maxent model and actual physiological indicators in relation to stress tolerance. J. Environ. Manage 322, 116024. doi:10.1016/J.JENVMAN.2022.116024
Silva, D. P., Gonzalez, V. H., Melo, G. A. R., Lucia, M., Alvarez, L. J., and De Marco, P. (2014). Seeking the flowers for the bees: Integrating biotic interactions into niche models to assess the distribution of the exotic bee species lithurgus huberi in south America. Ecol. Modell. 273, 200–209. doi:10.1016/j.ecolmodel.2013.11.016
Smart, J., and Gill, J. A. (2003). Non-intertidal habitat use by shorebirds: A reflection of inadequate intertidal resources? Biol. Conserv. 111, 359–369. doi:10.1016/S0006-3207(02)00304-X
Stattersfield, A., Bennun, L., and Jenkins, M. (2008). State of the world's birds: Indicators for our changing world. Cambridge, UK: BirdLife International.
Su, H. Y., Bista, M., and Li, M. S. (2021). Mapping habitat suitability for asiatic black bear and red panda in Makalu Barun National Park of Nepal from Maxent and GARP models. Sci. Rep. 11, 14135. doi:10.1038/s41598-021-93540-x
Sun, C. Z., Koenig, H. J., Uthes, S., Chen, C., Li, P., and Hemminger, K. (2020). Protection effect of overwintering water bird habitat and defining the conservation priority area in Poyang Lake wetland, China. Environ. Res. Lett. 15, 125013. doi:10.1088/1748-9326/abc6d0
Swets, J. A. (1988). Measuring the accuracy of diagnostic systems. Science 240, 1285–1293. doi:10.1126/science.3287615
Tebaldi, C., Debeire, K., Eyring, V., Fischer, E. M., Ziehn, T., Friedlingstein, P., et al. (2021). Climate model projections from the scenario model intercomparison project (ScenarioMIP) of CMIP6. Earth Syst. Dyn. 12, 253–293. doi:10.5194/ESD-12-253-2021
Tian, J. X., Zhang, Z. X., Kong, R., Zhu, B., Zhang, F. Y., Jiang, S. S., et al. (2021). Changes in water use efficiency and their relations to climate change and human activities in three forestry regions of China. Theor. Appl. Climatol. 144, 1297–1310. doi:10.1007/s00704-021-03600-5
Tian, R., Cao, C. X., Peng, L., Ma, G. R., Bao, D. M., Guo, J. H., et al. (2014). The use of HJ-1A/B satellite data to detect changes in the size of wetlands in response in to a sudden turn from drought to flood in the middle and lower reaches of the Yangtze River system in China. Geomatics, Nat. Hazards Risk 1, 287–307. doi:10.1080/19475705.2014.897654
Van, C. P., and Maavara, T. (2016). Rivers in the Anthropocene: Global scale modifications of riverine nutrient fluxes by damming. Ecohydrol. Hydrobiology 16, 106–111. doi:10.1016/j.ecohyd.2016.04.001
Van Eerden, M. R., Drent, R. H., Stahl, J., and Bakker, J. P. (2005). Connecting seas: Western palaearctic continental flyway for water birds in the perspective of changing land use and climate. Glob. Chang. Biol. 11, 894–908. doi:10.1111/j.1365-2486.2005.00940.x
Wang, H. J., Tang, G. L., Chen, H. S., Wu, S. H., and Xiao, C. D. (2020). The belt and road region climate change: Facts, impacts and possible risks. Trans. Atmos. Sci. 43, 1–9. doi:10.13878/j.cnki.dqkxxb.20191110003
Wang, H. J., Wang, T., Jiang, D. P., and Fu, Y. H. (2009). Will the climate change smaller than the projection? Quat. Sci. 29, 1011–1014.
Wang, W. J., Fraser, J. D., and Chen, J. K. (2017). Wintering waterbirds in the middle and lower Yangtze River floodplain: Changes in abundance and distribution. Bird. Conserv. Int. 27, 167–186. doi:10.1017/S0959270915000398
Wang, Y. (2020). Poyang Lake and wintering siberian cranes. Front. Ecol. Environ. 18, 100. doi:10.1002/fee.2171
Xia, S. X., Wang, Y. Y., Lei, G., Liu, Y., Lei, J. Y., Xiu, B. Y., et al. (2017). Restriction of herbivorous waterbird distributions in the middle and lower Yangtze River floodplain in view of hydrological isolation. Wetl. Wilmingt. 37, 79–88. doi:10.1007/s13157-016-0841-9
Yang, G. S., Xu, X. B., and Wang, W. (2022). Basic situation, challenges and coordinated governance of protection and development for Yangtze River in past 30 years. Resour. Environ. Yangtze Basin 31, 1653–1663.
Yang, J., Wen, X. R., Wang, Q. L., Ye, J. S., Zhang, Y. L., and Sun, Y. (2022a). A novel algorithm based on geometric characteristics for tree branch skeleton extraction from LiDAR point cloud. Forests 13, 1534. doi:10.3390/f13101534
Yang, J., Wen, X. R., Wang, Q. L., Ye, J. ZS., Zhang, Y. L., and Sun, Y. (2022b). A novel scheme about skeleton optimization designed for ISTTWN algorithm. Remote Sens. (Basel) 14, 6097. doi:10.3390/rs14236097
Yang, S. L., Xu, K. H., Milliman, J. D., Yang, H. F., and Wu, C. S. (2015). Decline of Yangtze River water and sediment discharge: Impact from natural and anthropogenic changes. Sci. Rep. 5, 12581. doi:10.1038/srep12581
Yao, S. Y., Li, X. Y., Liu, C. L., Zhang, J., Li, Y. K., Gan, T., et al. (2020). New assessment indicator of habitat suitability for migratory bird in wetland based on hydrodynamic model and vegetation growth threshold. Ecol. Indic. 117, 106556. doi:10.1016/j.ecolind.2020.106556
Yu, Y. Z., Wu, X. Y., Yin, D. D., Zhang, L. B., and Mu, W. J. (2019). Habitat suitability evaluation of moose in genbe region of inner Mongolia based on Maxent riche model. For. Sci. Technol. 44, 1–4. doi:10.19750/j.cnki.1001-9499.2019.02.001
Yuan, Y., Tang, X., Liu, S., and Zhang, J. (2020). The major factors influencing distribution of three species of dendrobium: Analysis of potential ecologically suitable distributions. J. Appl. Res. Med. Aromat. Plants 19, 100275. doi:10.1016/j.jarmap.2020.100275
Zhang, L., Ma, D. L., Li, C., Zhou, R. B., Wang, J., and Liu, Q. Y. (2022). Projecting the potential distribution areas of Ixodes scapularis (Acari: Ixodidae) driven by climate change. Biol. (Basel) 11, 107. doi:10.3390/biology11010107
Zhang, M., Ni, L., Xu, J., He, L., Fu, H., and Liu, Z. (2013). Annual dynamics of the wetland plants community in Poyang Lake in response to water-level variations. Res. Environ. Sci. 26, 1057–1063. doi:10.13198/j.issn.1001-6929.2013.10.010
Zhang, N., Li, Y. K., Shan, J. H., Tu, X. B., Zhang, Y. H., Li, Y., et al. (2019). Community structure, abundance and spatial distribution of water birds wintering in Poyang Lake wetland. J. Lake Sci. 31, 183–194. doi:10.18307/2019.0117
Zhang, W. Y., Li, X. H., Le, Y., and Si, Y. (2018). Multi-scale habitat selection by two declining East Asian waterfowl species at their core spring stopover area. Ecol. Indic. 87, 127–135. doi:10.1016/j.ecolind.2017.12.035
Zhou, T. J., Zou, L. W., and Chen, X. L. (2019). Commentary on the coupled model intercomparison project phase 6(CMIP6). Adv. Clim. Chang. Res. 15, 445–456.
Zhou, Y. C., Zhang, Z. X., Zhu, B., Cheng, X. F., Yang, L., Gao, M. K., et al. (2021). Maxent modeling based on cmip6 models to project potential suitable zones for Cunninghamia lanceolata in China. Forests 12, 752. doi:10.3390/f12060752
Keywords: Siberian crane, habitat suitability, MAXENT model, CMIP6, middle-lower Yangtze River
Citation: Liu X, Zhang Z, Zhang J, Zhu B and Tian J (2023) Projection of the potential distribution of suitable habitats for Siberian crane (Grus leucogeranus) in the middle and lower reaches of the Yangtze River basin. Front. Earth Sci. 11:1193677. doi: 10.3389/feart.2023.1193677
Received: 29 March 2023; Accepted: 21 June 2023;
Published: 30 June 2023.
Edited by:
Guojie Wang, Nanjing University of Information Science and Technology, ChinaReviewed by:
Dan Zhang, Chinese Academy of Sciences (CAS), ChinaZhenghua Hu, Nanjing University of Information Science and Technology, China
Yanhong Wu, Chinese Academy of Sciences (CAS), China
Copyright © 2023 Liu, Zhang, Zhang, Zhu and Tian. This is an open-access article distributed under the terms of the Creative Commons Attribution License (CC BY). The use, distribution or reproduction in other forums is permitted, provided the original author(s) and the copyright owner(s) are credited and that the original publication in this journal is cited, in accordance with accepted academic practice. No use, distribution or reproduction is permitted which does not comply with these terms.
*Correspondence: Zengxin Zhang, bmZ1emhhbmdAMTYzLmNvbQ==