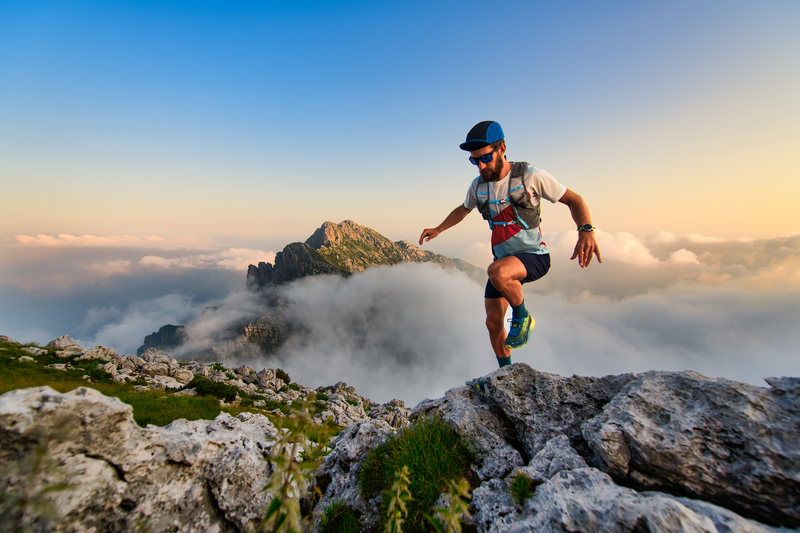
94% of researchers rate our articles as excellent or good
Learn more about the work of our research integrity team to safeguard the quality of each article we publish.
Find out more
EDITORIAL article
Front. Earth Sci. , 09 March 2023
Sec. Environmental Informatics and Remote Sensing
Volume 11 - 2023 | https://doi.org/10.3389/feart.2023.1163593
This article is part of the Research Topic Applications of Artificial Intelligence in the Oil and Gas Industry View all 9 articles
Editorial on the Research Topic
Applications of artificial intelligence in the oil and gas industry
This editorial summarizes the contributions to the Research Topic “Applications of Artificial Intelligence in the Oil and Gas Industry” established under the Environmental Informatics and Remote Sensing section of the Frontiers in Earth Science journal.
In recent years, the emergence of deep learning and big data has revolutionized many fields, including the oil and gas industry where the application of artificial intelligence (AI) has become increasingly popular. AI-based techniques have successfully addressed various challenges, making it essential to maximize their potential in the oil and gas sector. This Research Topic aims to explore the latest advances in this field and identify potential opportunities for further development.
Liu et al. proposed a novel approach for describing and simplifying flow fields using principal component analysis (PCA) downscaling and clustering algorithms. The PCA technique was used to reduce the dimensionality of flow line features, which enabled an accurate representation of flow lines without sacrificing many features. Clustering methods were then employed to simplify the flow field by dividing different flow lines and selecting the main flow lines in the injection-production units. This significantly improved the visualization effect of the flow line field and alleviated the interference of complex flow lines.
Liu and Liu further explored the identification of permeability configurations between injectors and producers in low-permeability reservoirs using machine learning methods. They trained neural networks, support vector machines, and decision trees on the dynamic data that affect non-linear permeability configurations. Their results indicated that support vector machines exhibited the best validation accuracy.
Tan et al. conducted another study focusing on a deep convolutional neural network (CNN) diagnosis model that combined multiscale normalization methods with the dynamometer card of a pumping rod pumping well. They proposed a novel dynamometer data preprocessing method that utilized multiple normalized dynamometer data from the original ones as model inputs, introducing more characteristic information and hence effectively enhancing the data’s class discrepancy. The CNN was found to outperform the support vector machine in various working condition diagnosis tasks.
Kangxing et al. conducted a study on balance and correlation analysis of oilfield injection systems based on data mining. This study identified the optimal injection well through a dynamic comparison of oil-water wells, analyzed the impact of injection volume on oil production efficiency, conducted injection prediction research, and proposed an injection prediction model. This provided a foundation for the design of corrective measures within the block to reduce the increase of water content, maintain production stability, and enhance the efficiency of mechanical production systems.
Wang et al. proposed a reservoir parameter prediction method based on a neural random forest model. In this study, a novel hybrid model combining neural networks and random forests was proposed for predicting porosity and saturation based on shale gas reservoir logging data. The ability of the proposed model to predict porosity and saturation was discussed, and the model was compared with baseline methods such as neural networks and random forests.
Liu et al. analyzed XGBoost stratigraphic thickness recognition based on logging data. They found that 12 response features selected from field logging data can effectively identify active rock layers and reservoirs according to the underground location in stratigraphic thickness and reservoir recognition. Through a comprehensive comparison of five discrimination methods, the study showed that the XGBoost algorithm was most effective for rock and reservoir recognition, with an average accuracy of more than 95%.
Zhang et al. used a gated recurrent neural network to simultaneously predict multiple parameters, including porosity, shale content, and water saturation. The Pearson product-moment correlation coefficient showed a weak correlation between elastic parameters and physical parameters. Nevertheless, the model was still able to effectively capture their non-linear high-dimensional relationship as well as accurately forecast and regenerate the missing parts in the log data.
Mahmoud et al. used different machine learning algorithms to evaluate the dynamic Young’s modulus of composite strata in real time according to drilling parameters. Three machine learning models, namely, random forest, subtractive clustering adaptive neural fuzzy inference system, and support vector regression, were optimized to estimate the dynamic Young’s modulus in real time according to the measurable drilling parameters on the ground. The results showed that the optimized machine learning models had high accuracy in estimating the dynamic Young’s modulus according to the measurable drilling parameters on the ground.
All authors have made a significant, direct, and intellectual contribution to the work and have given their approval for its publication.
QL would like to thank the support of the Science Foundation of China University of Petroleum, Beijing (Grant No. 2462022QNXZ002). DL would like to thank the support of the National Natural Science Foundation of China (Grant No. 12172115). KZ would like to thank the support of the National Natural Science Foundation of China under Grant 52274057, 52074340, and 51874335, 111 Project under Grant B08028.
We thank all the authors and reviewers who have participated in this Research Topic.
The authors declare that the research was conducted in the absence of any commercial or financial relationships that could be construed as a potential conflict of interest.
All claims expressed in this article are solely those of the authors and do not necessarily represent those of their affiliated organizations, or those of the publisher, the editors and the reviewers. Any product that may be evaluated in this article, or claim that may be made by its manufacturer, is not guaranteed or endorsed by the publisher.
Keywords: artificial intelligence, data mining, deep learning, oil and gas, reservoir property estimation, production optimization
Citation: Liao Q, Li D, Zhang K and Chang H (2023) Editorial: Applications of artificial intelligence in the oil and gas industry. Front. Earth Sci. 11:1163593. doi: 10.3389/feart.2023.1163593
Received: 11 February 2023; Accepted: 28 February 2023;
Published: 09 March 2023.
Edited and reviewed by:
Alexander Kokhanovsky, German Research Centre for Geosciences, GermanyCopyright © 2023 Liao, Li, Zhang and Chang. This is an open-access article distributed under the terms of the Creative Commons Attribution License (CC BY). The use, distribution or reproduction in other forums is permitted, provided the original author(s) and the copyright owner(s) are credited and that the original publication in this journal is cited, in accordance with accepted academic practice. No use, distribution or reproduction is permitted which does not comply with these terms.
*Correspondence: Daolun Li, bGRhb2xAdXN0Yy5lZHUuY24=
Disclaimer: All claims expressed in this article are solely those of the authors and do not necessarily represent those of their affiliated organizations, or those of the publisher, the editors and the reviewers. Any product that may be evaluated in this article or claim that may be made by its manufacturer is not guaranteed or endorsed by the publisher.
Research integrity at Frontiers
Learn more about the work of our research integrity team to safeguard the quality of each article we publish.