- 1Beijing Earthquake Agency, Beijing, China
- 2School of Geodesy and Geomatics, Wuhan University, Wuhan, China
- 3Nordic Volcanological Center, Institute of Earth Sciences, University of Iceland, Reykjavík, Iceland
To quantitatively investigate the relationship between earthquakes and ionospheric anomalies, this paper presents a statistical study of pre-earthquake vertical total electron content (VTEC) variations. A total of 1522 shallow (≤60 km) strong (Mw≥6.0) earthquakes in the global area during 2000-2020 are selected, and classified according to different magnitudes, latitudes and focal depths. A quartile-based process with different lengths of sliding windows, equaling 10 days, 15 days and 27 days, respectively, has been utilized to detect VTEC anomalies. The abnormal level is first defined, and then VTEC anomalies occurrence probabilities (
1 Introduction
The seismic process is not only confined to the lithosphere, but also has impacts on the troposphere, ionosphere and even magnetosphere through the electromagnetic fields effect. The ionospheric anomalies within a few days before the earthquakes are relatively stable at short time scales, and have been studied widely in the field of earthquake prediction (Pulinets and Boyarchuk, 2004; Liperovsky et al., 2008; Pulinets and Ouzounov, 2011). A large number of studies have shown that ionospheric perturbations before many earthquakes could be identified (e.g., Liu et al., 2001; Le et al., 2011; Zhu et al., 2014; Tang et al., 2015; Sun et al., 2016; Parrot and Li, 2018; Pulinets et al., 2021). The possible earthquake-related NmF2 (F2 layer peak electron density) and TEC anomalies have been widely discussed in recent decades (e.g., Nishihashi et al., 2009; Ma et al., 2014). Especially, because of the development of the Global Navigation Satellite system (GNSS), GNSS VTEC have attracted more and more attention in the investigation of the ionospheric variations prior to large earthquakes (Liu et al., 2004; Shah and Jin, 2015). For the first time, Liu et al. (2001) used GNSS(GPS) VTEC to study the ionospheric disturbance before the Chi-Chi earthquake, and found that the VTEC over the epicenter decreased significantly 1, 3 and 4 days before the earthquake. Later on, more and more scientists began to focus on GNSS VTEC variations before earthquakes, aiming to detect the potential ionospheric anomalies related to the forthcoming earthquakes. For example, Yao et al. (2012) analyzed ionospheric variations prior to the 2011 Mw9.0 Japan earthquake, and indicated that ionospheric anomalies occurring on 8 March might be a precursor of the earthquake; Ho et al. (2013) showed that TEC increased 9–19 days before the 2010 M8.8 Chile earthquake and specifically over the epicenter; Su et al. (2013) investigated ionospheric TEC variations before the Hector Mine earthquake, and found that ionospheric disturbance appeared just above the epicenter 5 days before the earthquake. These studies show that the GNSS TEC anomalies appear a few days before the earthquake with different magnitude and focal depth.
Over about 50 years of research, no consensus in the scientific community has been formed on the existence of ionospheric earthquake precursors (Rishbeth, 2006; Dautermann et al., 2007; Masci, 2012; Ovalle et al., 2013; Masci and Thomas, 2014; Zolotov et al., 2019). Dautermann et al. (2007) indicated that there was no statistically significant correlation between TEC anomalies and earthquakes in Southern California during 2003–2004; Kon et al. (2011) selected M≥6.0 earthquakes in Japan during 1998–2010, and found that significant positive TEC anomalies within 1000 km above the epicenter appeared within 1–5 days before the earthquakes. According to Masci (2012), the analysis of Kon et al. (2011) was not reliable because of the influence of global geomagnetic events. Ovalle et al. (2013) concluded that it remained controversial whether the observed NmF2 and TEC anomalies were unambiguously related to the 2010 M8.8 Chile earthquake. Background geomagnetic events may impact revealing the relationship between TEC anomalies and earthquakes.
To validate the relationship between ionospheric anomalies and earthquakes in response to the controversy, many scientists have undertaken a multitude of studies on the physical mechanism of generating ionospheric anomalies. Firstly, the morphological characteristics of ionospheric anomalies before a large number of earthquakes are summarized. For instance, Liu et al. (2004) analyzed Ms5.0+ earthquakes in Taiwan from 1999 to 2002, and found that obvious negative TEC anomalies occurred 5 days before the earthquakes. Le et al. (2011) made a statistical study of global 736 M≥6.0 earthquakes during 2002–2010, and proposed that occurrence rates of abnormal days are larger for earthquakes with greater magnitude and lower depth. De Santis et al. (2019) analyzed the electron density and magnetic field data from 3 Swarm satellites to detect possible anomalies associated with 1312 M≥5.5 shallow earthquakes from January 2014 to August 2018, and the results showed that anomalies occurred between a few days and 80 days before the earthquakes with larger peaks at around 10, 20 and 80 days, and supported the Lithosphere-Atmosphere-Ionosphere Coupling (LAIC) with clear statistical significance. Shah et al. (2020) studied the ionospheric anomalies before the global Mw≥5.0 earthquakes from 1998 to 2019, and the results revealed that prominent ionospheric anomalies appeared within 5 days before and after the earthquakes. Based on the characteristics of ionospheric anomalies prior to a large number of earthquakes, the physical mechanisms of seismic LAIC have been extensively studied (Freund, 2011; Klimenko et al., 2012; Pulinets, 2012; Zolotov et al., 2012). For example, Freund (2011) proposed that positive holes released by stressed rocks are highly mobile and can reach the Earth’s surface, and then ionize the atmosphere and change the vertical electric field between the ground and the lower edge of the ionosphere. In addition, a large number of studies have shown that anomalous atmospheric electric field variations in the earthquake preparation zone are likely to be the main cause of ionospheric disturbance (Zhang et al., 2014; Jiang et al., 2017; Pulinets and Davidenko, 2018; Davidenko and Pulinets, 2019). For example, Namgaladze et al. (2009) proposed that vertical plasma motion in the ionospheric F2 region under the action of the zonal electric field is the main disturbance formation factor, and ionospheric anomalies before strong earthquakes at middle and low latitudes verified this mechanism. Liu et al. (2010) studied the crest of equatorial ionization anomaly (EIA) variations before 150 M≥5.0 earthquakes in Taiwan, and the results implied that the weak atmospheric electric field a few days before the earthquakes may cause the EIA crest anomalies.
Both the characteristics of ionospheric anomalies and the physical mechanisms of lithosphere-atmosphere-ionosphere coupling present diversity and complexity, and the influence factors may include magnitudes, focal depths, latitude and longitude of the epicenter, focal mechanisms, the weather, the season, solar and geomagnetic activity and so on. However, many studies focused on a single large earthquake, and there are relatively few statistical results based on a large number of earthquakes. The corresponding characteristics of the ionospheric anomalies are still not fully understood. In addition, a quartile-based process is the most common method for extracting ionospheric VTEC anomalies, but different authors use different numbers of days as the lengths of the sliding windows, such as, 10 days (e.g., Zhou et al., 2009; Zhu et al., 2014), 15 days (e.g., Liu et al., 2010; Ke et al., 2016; Liu and Xu, 2017), 27 days (e.g., Xu et al., 2011; Guo et al., 2015), etc. It should be noted that the effects of different sliding windows on the ionospheric anomalies are rarely studied (Zolotov et al., 2019). To solve the above problems, this study uses GIM VTEC to carry out a statistical analysis by studying the VTEC anomalies within 1–10 days before 1522 global shallow (≤60 km) Mw≥6.0 earthquakes during 2000–2020. The factors, including the magnitude, focal depth and the latitude of the epicenter are considered for all the earthquakes, and the effects of different sliding windows on ionospheric anomalies are investigated. Moreover, ionospheric anomalies during background days are also analyzed to compare with those prior to the earthquakes. This study aims at helping the research on the physical mechanisms of lithosphere-atmosphere-ionosphere coupling by summarizing the characteristics of ionospheric anomalies comprehensively, and determining whether different lengths of sliding windows affect ionospheric anomalies features.
2 Data and method
2.1 Data source
The worldwide Mw≥6.0 earthquakes during 2000–2020 are selected to analyze ionospheric anomalies in this study. The data are retrieved from the Global Centroid Moment Tensor (CMT) Project (http://www.globalcmt.org/). The earthquakes selected in this study are declustered from aftershocks following the method of Michael (2011), and the earthquakes occurring at the similar location but with the short interval (<10 days) from the previous ones are also excluded to avoid possible confounded effects from adjacent earthquakes. Finally, 1522 shallow (≤60 km) earthquakes are selected, and Figure 1 illustrates epicenter locations of these earthquakes.
The GIM VTEC is derived using the observations from hundreds of global GNSS stations (Hernández-Pajares et al., 2009). The GIM covers
The equatorial geomagnetic activity index (Dst) data provided by the World Data Center for Geomagnetism, Kyoto (https://wdc.kugi.kyoto-u.ac.jp/) are used to represent the geomagnetic activity.
2.2 Statistical method
In this study, a quartile-based process is performed to detect ionospheric VTEC anomalies within 1–10 days prior to each earthquake. As the length of sliding window is limited by the seasonal variability of the ionosphere at longer timescales, 10, 15, and 27 days are chosen as the candidate lengths of sliding windows based on the previous studies. At each time point on any day, the median
After the analysis of seismo-ionospheric anomalies for each event, 1522 earthquakes are divided into three different latitudinal zones (as shown in Figure 1), and VTEC anomalies occurrence probabilities (
The occurrence rates for the
3 Results and discussions
3.1 Seismo-ionospheric anomalies occurrence probabilities
According to the method described above, we calculated
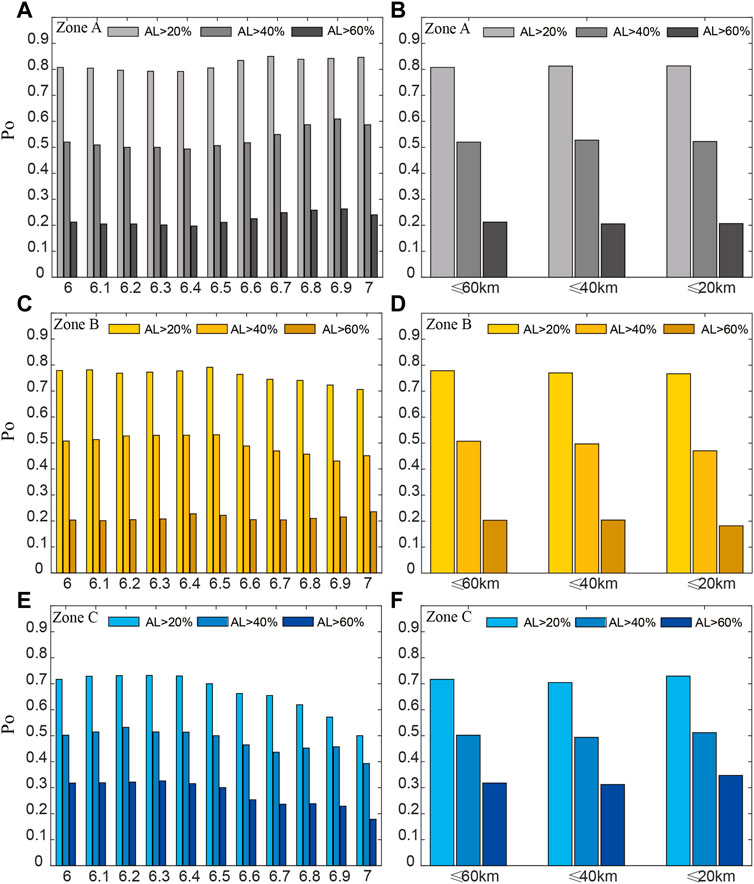
FIGURE 2. Seismo-ionospheric anomalies occurrence probabilities of AL>20%, 40%, and 60% with different magnitudes (A, C, E) and depths (B, D, F), respectively (15-day sliding window). (A, C) are the results in Zone A. (C, D) are the results in Zone B. (E, F) are the results in Zone C.
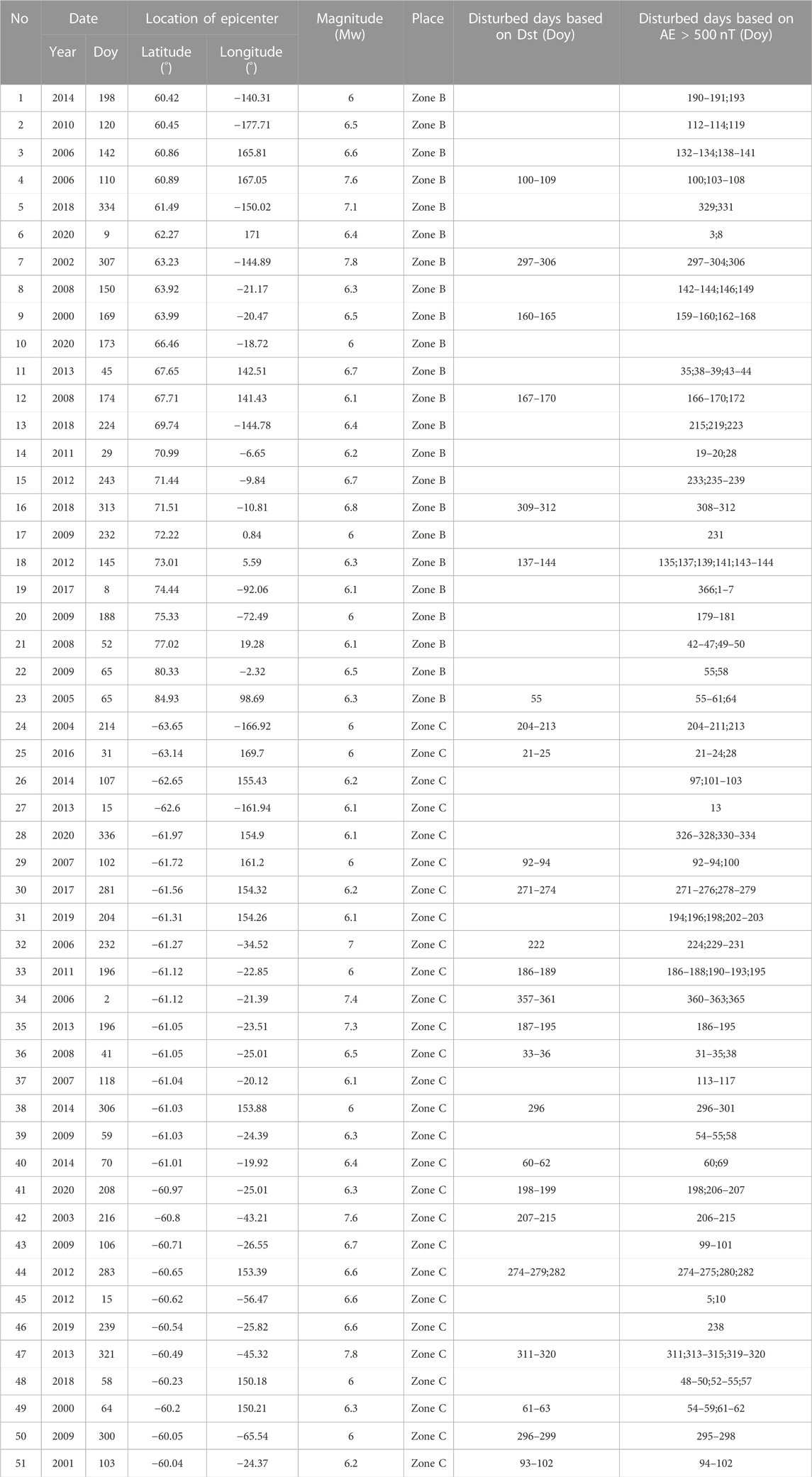
TABLE 1. The difference of disturbed days based on Dst and AE before 51 Earthquakes at high latitudes.
According to Figure 2 (right), one can find that the values of
To study the effects of different lengths of the sliding windows on
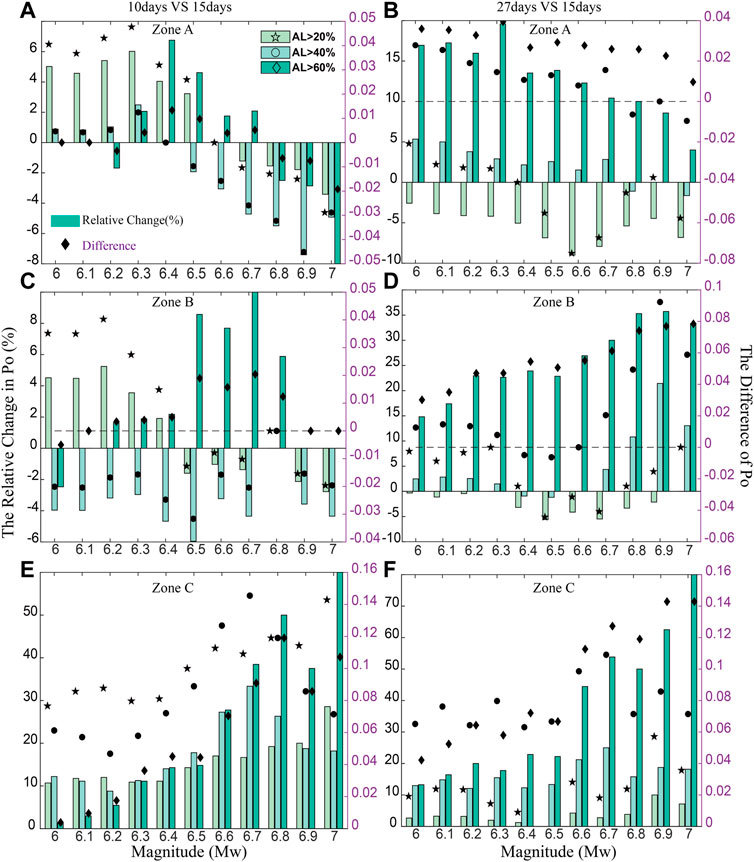
FIGURE 3. The differences and relative changes of seismo-ionospheric anomalies occurrence probabilities between the results of different sliding windows. (A, C, E) show the difference between the results of 10-day and 15-day sliding windows in Zone A, Zone B and Zone C, respectively. (B, D, F) show the difference between the results of 27-day and 15-day sliding windows in Zone A, Zone B and Zone C, respectively.
For the earthquakes in the northern mid- and high-latitude region (Zone B), compared with the results of 15-day sliding window: 1) most differences are random, and are also greater using 27-day sliding window; 2) For the cases of AL>20% or AL>40%, most relative changes are between −6% and 10%. For the cases of AL>60%, the 27-day results increase systematically, and the maximum difference and relative change can reach 0.092% and 35.71%, respectively.
For the earthquakes in the low-latitude and equatorial region (Zone A), compared with the results of 15-day sliding window: 1) the results vary systematically using the 27-day sliding window; 2) For the cases of AL> 20% or AL>40%, most relative changes are between −8% and 8%. For the cases of AL>60%, the 27-day results increase systematically and the differences decrease with the magnitude increasing, with the maximum difference and relative change reaching 0.039% and 19.59%, respectively.
When AL > 20% or AL > 40% is selected to extract seismo-ionospheric anomalies, most of the relative changes of different sliding window are under 20%. In this study, GIM produced by the Jet Propulsion Laboratory (JPL) is used, and the error of VTEC estimation during the creating process of global maps by JPL is about 10%–17% (Zakharenkova et al., 2008). Therefore, for the case of AL > 20% or AL > 40%, different lengths of sliding windows have a statistically insignificant influence on
Figure 4 shows the Index Number in percentage of the seismo-ionospheric anomalies within 1–10 days before the earthquakes. The Index Number is calculated as the ratio of the cumulative number of seismo-ionospheric anomalies in a single day and the total number of seismo-ionospheric anomalies (Shah et al., 2020). The Index Number enhances within 5 days before the earthquakes in all the three zones, especially in Zone A and B. Figures 5, 6 show the differences and relative changes between the results of different sliding windows. It can be seen that the differences between the results of different sliding windows are random, and are larger between the results of 15-day and 27-day sliding windows. The differences are smallest in Zone A, followed by Zone B. The differences raise with AL increasing. For the case of AL>20% or AL>40%, compared with the results of 15-day sliding window, the most of relative changes are between −20% and 25%. For the case of AL > 60%, the differences are more significant, as the relative changes can exceed 50% in all the three zones. So when AL>60% is selected, the impacts of different sliding windows on the Index Number can not be neglected.
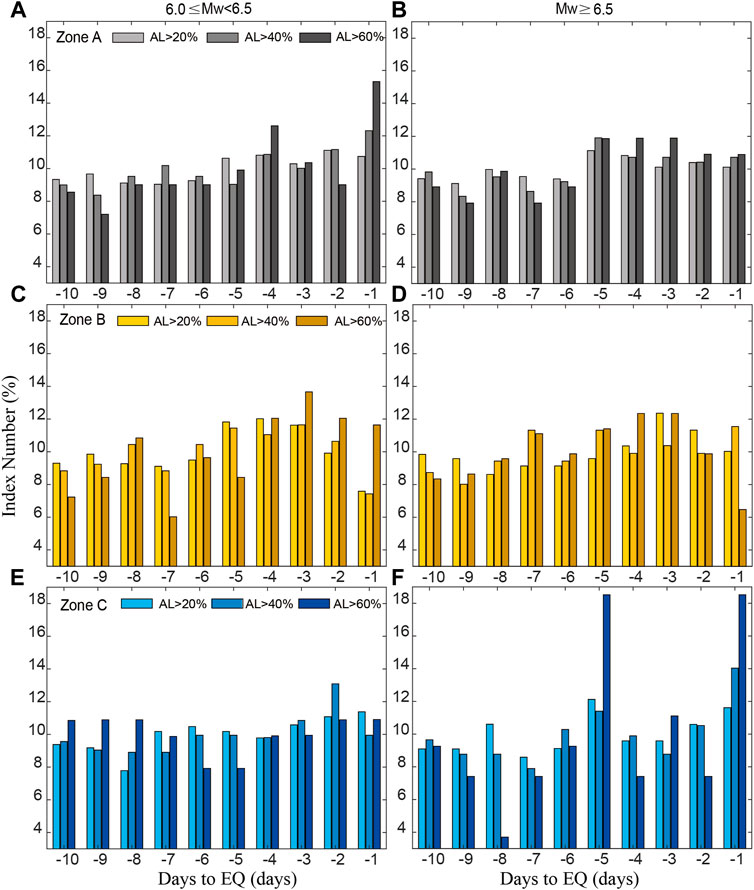
FIGURE 4. The Index Numbers of the seismo-ionospheric anomalies within 1-10 days before the global earthquakes during 2000-2020 (15-day sliding window). (A, C, E) are the results of 6.0≤Mw<6.5 in Zone A, Zone B and Zone C, respectively. (B, D, F) are the results of Mw≥6.5 in Zone A, Zone B and Zone C, respectively.
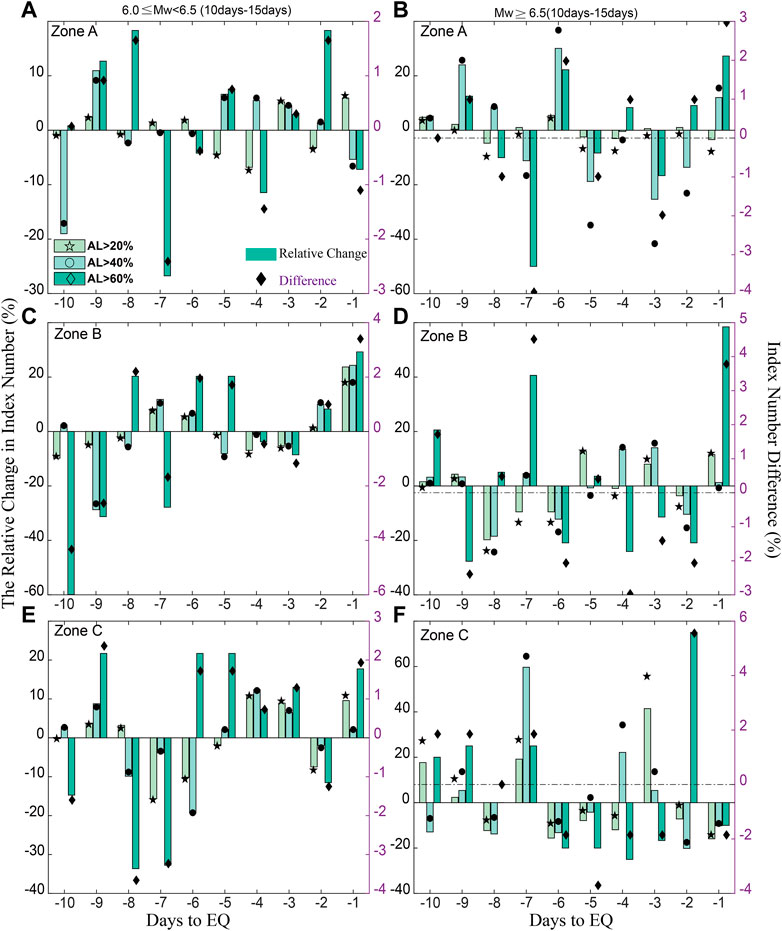
FIGURE 5. The differences and relative changes of the Index Numbers of the seismo-ionospheric anomalies between the results of 10-day and 15-day sliding windows. (A, C, E) represent the results of 6.0≤Mw<6.5 in Zone A, Zone B and Zone C, respectively. (B, D, F) represent the results of Mw≥6.5 in Zone A, Zone B and Zone C, respectively.
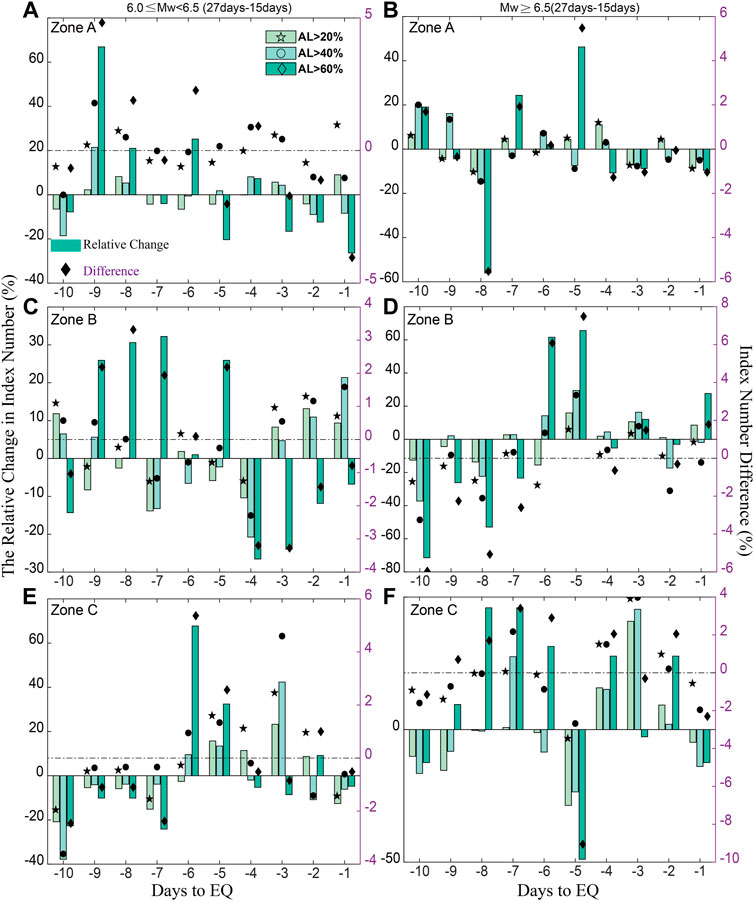
FIGURE 6. The differences and relative changes of the Index Numbers of the seismo-ionospheric anomalies between the results of 27-day and 15-day sliding windows. (A, C, E) represent the results of 6.0≤Mw<6.5 in Zone A, Zone B and Zone C, respectively. (B, D, F) represent the results of Mw≥6.5 in Zone A, Zone B and Zone C, respectively.
3.2 Seismo-ionospheric anomalies occurrence rates
According to Eq. 2, seismo-ionospheric anomalies occurrence rates
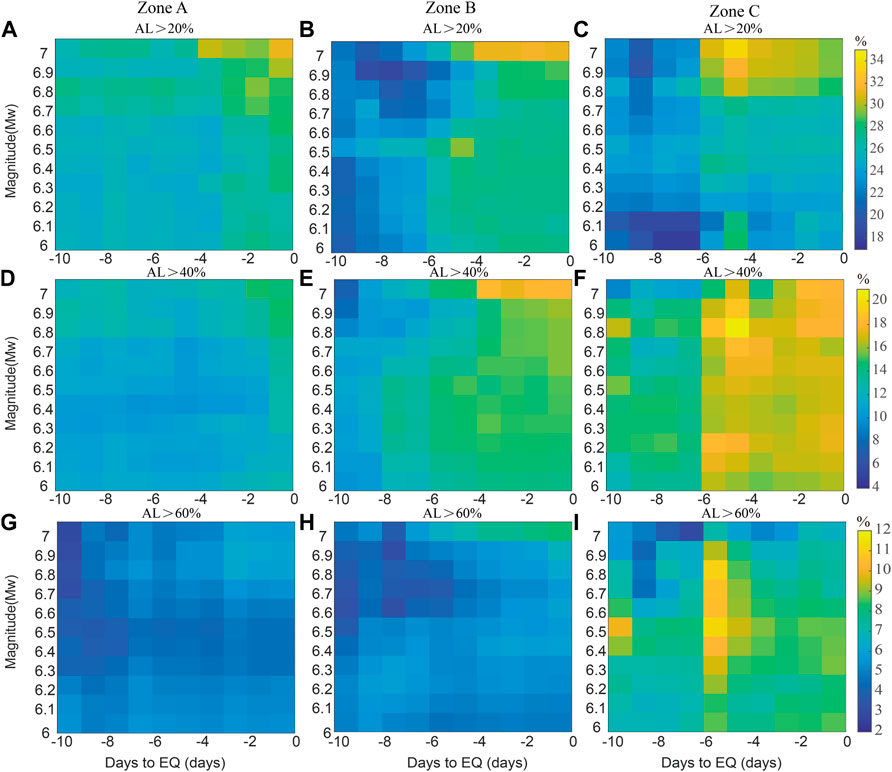
FIGURE 7. Seismo-ionospheric anomalies occurrence rates of AL>20%, 40%, and 60% within 1-10 days before earthquakes of Zone A (A, D, G), Zone B (B, E, H), and Zone C (C, F, I), respectively (15-day sliding window).
Figures 8, 9 represent the
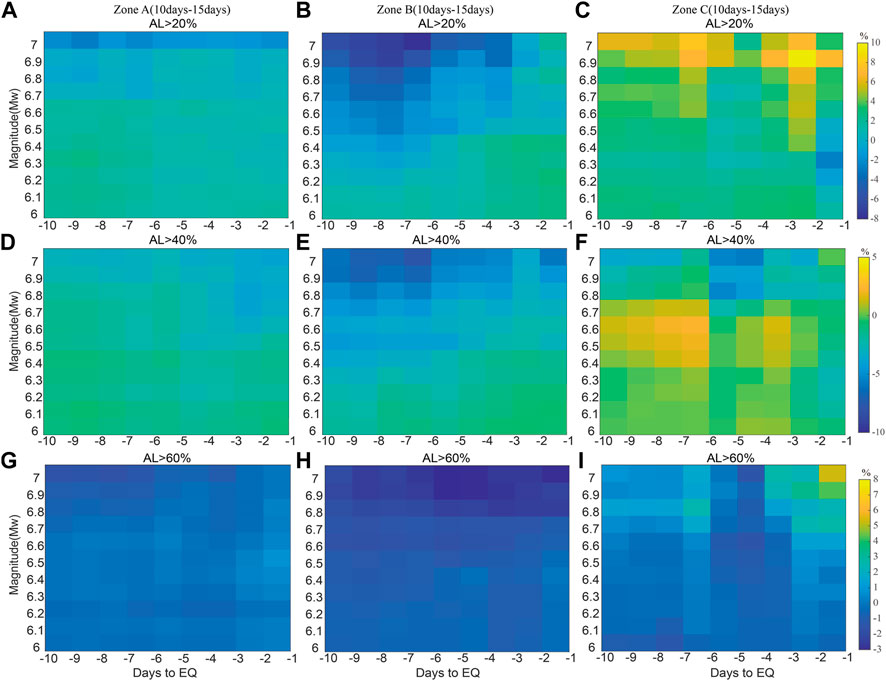
FIGURE 8. The differences of seismo-ionospheric anomalies occurrence rates between the results of 10-day and 15-day sliding windows. (A, D, G) represent the results in Zone A. (B, E, H) represent the results in Zone B. (C, F, I) represent the results in Zone C.
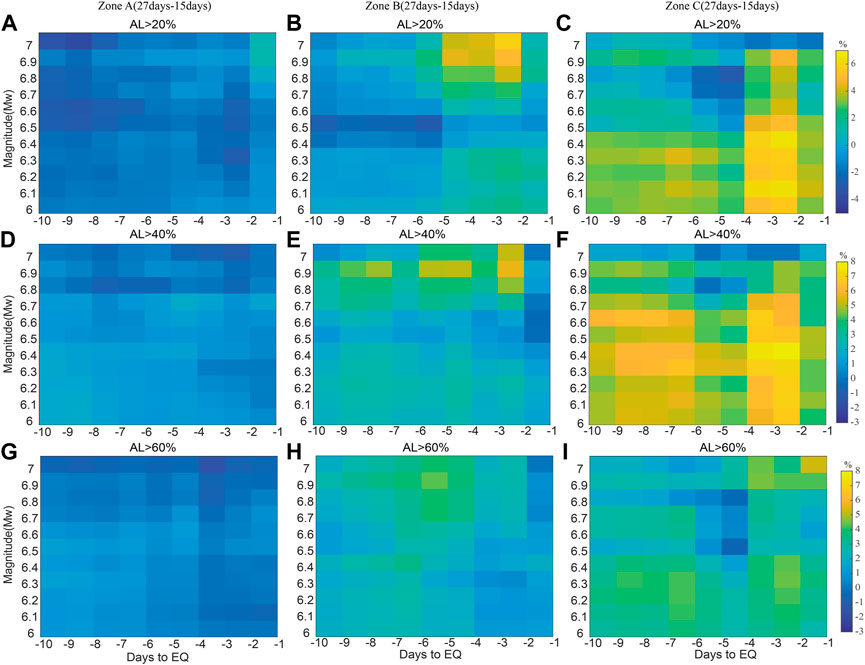
FIGURE 9. The differences of seismo-ionospheric anomalies occurrence rates between the results of 27-day and 15-day sliding windows. (A, D, G) represent the results in Zone A. (B, E, H) represent the results in Zone B. (C, F, I) represent the results in Zone C.
To compare the difference between the seimo-ionospheric anomalies a few days before the earthquakes and the day-to-day ionospheric variation, the ionospheric anomalies occurrence rates during background days
In this equation,
Figure 10A shows the values of
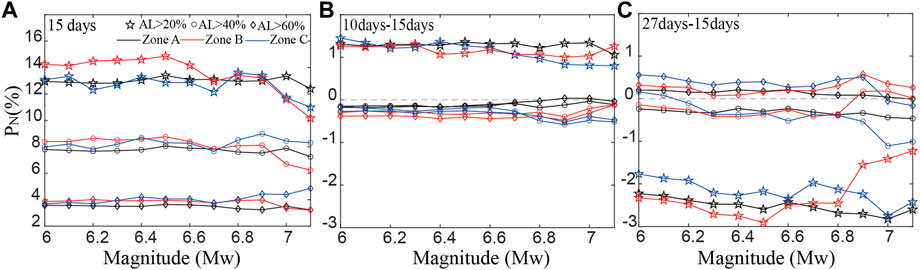
FIGURE 10. (A) Ionospheric anomalies occurrence rates of A >20%, 40%, and 6% during background days; (B) The differences of ionospheric anomalies occurrence rates between the results of 10-day and 15-day sliding windows; (C) The differences of ionospheric anomalies occurrence rates between the results of 27-day and 15-day sliding windows.
Figure 11 shows the ratio of
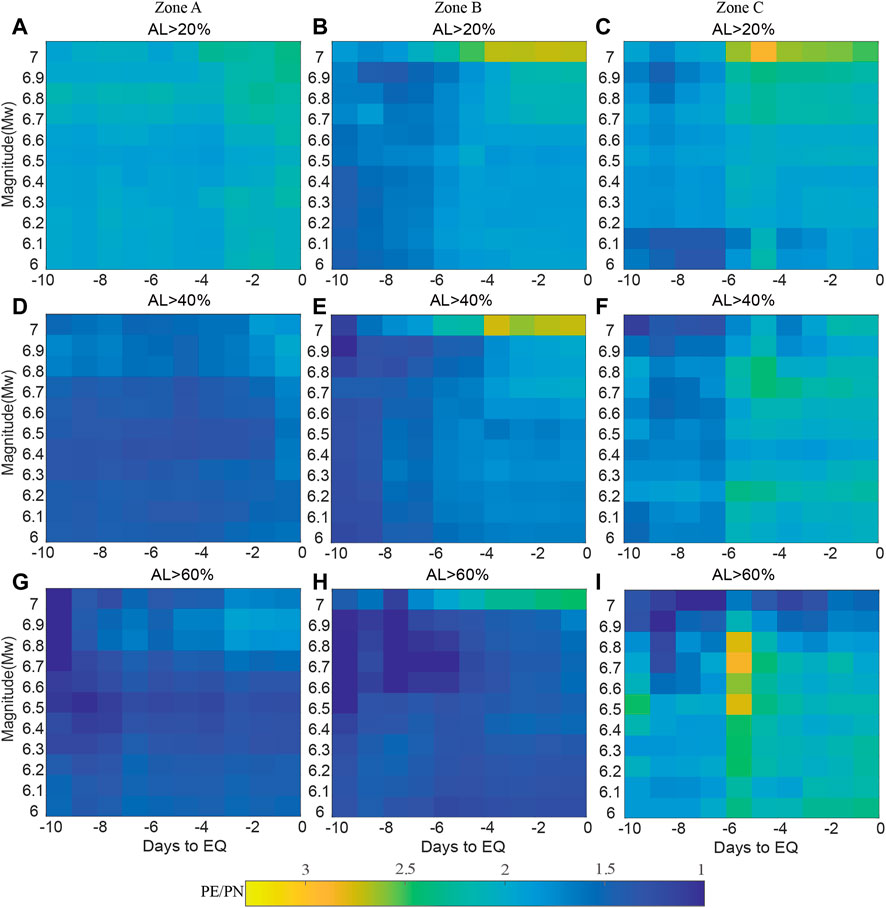
FIGURE 11. The ratio of the seimo-ionospheric anomalies occurrence rates within 1-10 days before the earthquakes to the ionospheric anomalies occurrence rates during background days (15-day sliding window). (A, D, G) represent the results in Zone A. (B, E, H) represent the results in Zone B. (C, F, I) represent the results in Zone C.
4 Conclusion
Both the latitude of the epicenter and the magnitude can affect the characteristics of seismo-ionospheric anomalies before shallow earthquakes. In terms of seismo-ionospheric anomalies occurrence probabilities
Both the number of days before earthquakes and the AL can affect the characteristics of seismo-ionospheric anomalies before shallow earthquakes. The number of seismo-ionospheric anomalies within 1–5 days before the earthquakes increases significantly in all the three zones, and the positive correlation between the values of
For the mid- and high-latitude region, the effects of different lengths of sliding windows on seismo-ionospheric anomalies can not be ignored. For the case of AL>60%, different sliding windows have a significant impact on the values of
Moreover, there are large differences between the seismo-ionospheric anomalies occurrence rates
Data availability statement
The raw data supporting the conclusion of this article will be made available by the authors, without undue reservation.
Author contributions
MY and ZX contributed to conception of the study. Data were collected by HL, DH, and YR. MY, ZX, and YY performed the experimental analysis. MY wrote the first draft of the manuscript. ZX revised the manuscript. All authors read and approved the final manuscript.
Funding
This work was supported by Beijing Natural Science Foundation Grant Numbers 8224094.
Acknowledgments
We are grateful to the World Data Center for Geomagnetism, Kyoto providing Dst index, the Global CMT Project providing parameters of earthquakes, and IGS providing GIM.
Conflict of interest
The authors declare that the research was conducted in the absence of any commercial or financial relationships that could be construed as a potential conflict of interest.
Publisher’s note
All claims expressed in this article are solely those of the authors and do not necessarily represent those of their affiliated organizations, or those of the publisher, the editors and the reviewers. Any product that may be evaluated in this article, or claim that may be made by its manufacturer, is not guaranteed or endorsed by the publisher.
References
Dautermann, T., Calais, E., Haase, J., and Garrison, J. (2007). Investigation of ionospheric electron content variations before earthquakes in southern California, 2003-2004. J. Geophys. Res. 112, 1074–1086. doi:10.1029/2006JB004447
Davidenko, D. V., and Pulinets, S. A. (2019). Deterministic variability of the ionosphere on the eve of strong (M≥6) earthquakes in the regions of Greece and Italy according to long-term measurements data. Geomagn. Aeron. 59 (4), 493–508. doi:10.1134/S001679321904008X
De Santis, A., Marchetti, D., Pavón-Carrasco, F. J., Cianchini, G., Perrone, L., Abbattista, C., et al. (2019). Precursory worldwide signatures of earthquake occurrences on Swarm satellite data. Sci. Rep. 9, 20287. doi:10.1038/s41598-019-56599-1
Dobrovolsky, I. P., Zubkov, S. I., and Miachkin, V. I. (1979). Estimation of the size of earthquake preparation zones. Pure Appl. Geophys. 117 (5), 1025–1044. doi:10.1007/BF00876083
Freund, F. (2011). Pre-earthquake signals: Underlying physical processes. J. Asian Earth Sci. 41 (4-5), 383–400. doi:10.1016/j.jseaes.2010.03.009
Fujiwara, H., Kamogawa, M., Ikeda, M., Liu, J. Y., Sakata, H., Chen, Y. I., et al. (2004). Atmospheric anomalies observed during earthquake occurrences. Geophys. Res. Lett. 31, L17110. doi:10.1029/2004GL019865
Guo, J., Li, W., Yu, H., Liu, Z., Zhao, C., and Kong, Q. (2015). Impending ionospheric anomaly preceding the Iquique Mw8.2 earthquake in Chile on 2014 April 1. Geophys. J. Int. 203 (3), 1461–1470. doi:10.1093/gji/ggv376
Hernández-Pajares, M., Juan, J., Sanz, J., Orus, R., Garcia-Rigo, A., Feltens, J., et al. (2009). The IGS VTEC maps: A reliable source of ionospheric information since 1998. J. Geod. 83 (3-4), 263–275. doi:10.1007/s00190-008-0266-1
Ho, Y. Y., Jhuang, H. K., Su, Y. C., and Liu, J. Y. (2013). Seismo-ionospheric anomalies in total electron content of the GIM and electron density of DEMETER before the 27 February 2010 M8.8 Chile earthquake. Adv. Space Res. 51 (12), 2309–2315. doi:10.1016/j.asr.2013.02.006
Jiang, W., Ma, Y., Zhou, X., Li, Z., An, X., and Wang, K. (2017). Analysis of ionospheric vertical total electron content before the 1 April 2014 Mw 8.2 Chile earthquake. J. Seismol. 21 (6), 1599–1612. doi:10.1007/s10950-017-9684-y
Ke, F., Wang, Y., Wang, X., Qian, H., and Shi, C. (2016). Statistical analysis of seismo-ionospheric anomalies related to Ms>5.0 earthquakes in China by GPS TEC. J. Seismol. 20 (1), 137–149. doi:10.1007/s10950-015-9516-x
Klimenko, M. V., Klimenko, V. V., Zakharenkova, I. E., and Pulinets, S. A. (2012). Variations of equatorial electrojet as possible seismo-ionospheric precursor at the occurrence of TEC anomalies before strong earthquake. Adv. Space Res. 49 (3), 509–517. doi:10.1016/j.asr.2011.10.017
Kon, S., Nishihashi, M., and Hattori, K. (2011). Ionospheric anomalies possibly associated with M ≥ 6.0 earthquakes in the Japan area during 1998–2010: Case studies and statistical study. J. Asian Earth Sci. 41 (4), 410–420. doi:10.1016/j.jseaes.2010.10.005
Le, H., Liu, J. Y., and Liu, L. (2011). A statistical analysis of ionospheric anomalies before 736 M6.0+ earthquakes during 2002-2010. J. Geophys. Res. 116 (A2), A02303. doi:10.1029/2010JA015781
Liperovsky, V. A., Pokhotelov, O. A., Meister, C. V., and Liperovskaya, E. V. (2008). Physical models of coupling in the lithosphere-atmosphere-ionosphere system before earthquakes. Geomagn. Aeron. 48, 795–806. doi:10.1134/S0016793208060133
Liu, J. Y., Chen, C. H., Chen, Y. I., Yang, W. H., Oyama, K. I., and Kuo, K. W. (2010). A statistical study of ionospheric earthquake precursors monitored by using equatorial ionization anomaly of GPS TEC in Taiwan during 2001–2007. J. Asian Earth Sci. 39 (1), 76–80. doi:10.1016/j.jseaes.2010.02.012
Liu, J. Y., Chen, Y. I., Chuo, Y. J., and Tsai, H. F. (2001). Variations of ionospheric total electron content during the Chi-Chi earthquake. Geophys. Res. Lett. 28 (7), 1383–1386. doi:10.1029/2000GL012511
Liu, J. Y., Chuo, Y. J., Shan, S. J., Tsai, Y. B., Chen, Y. I., Pulinets, S. A., et al. (2004). Pre-earthquake ionospheric anomalies registered by continuous GPS TEC measurements. Ann. Geophys. 22 (5), 1585–1593. doi:10.5194/angeo-22-1585-2004
Liu, W., and Xu, L. (2017). Statistical analysis of ionospheric TEC anomalies before global Mw≥7.0 earthquakes using data of CODE GIM. J. Seismol. 21 (4), 759–775. doi:10.1007/s10950-016-9634-0
Ma, X., Lin, Z., and Chen, H. (2014). Analysis on ionospheric perturbation of TEC and NmF2 based on GPS and COSMIC data before and after the Wenchuan earthquake. Chin. J. Geophys 57 (8), 2415–2422. doi:10.6038/cjg20140803
Masci, F. (2012). The study of ionospheric anomalies in Japan area during 1998–2010 by Kon et al.: An inaccurate claim of earthquake-related signatures? J. Asian Earth Sci. 57, 1–5. doi:10.1016/j.jseaes.2012.06.009
Masci, F., and Thomas, J. (2014). Comment on “Temporal and spatial precursors in ionospheric total electron content of the 16 October 1999 Mw 7.1 Hector Mine earthquake” by Su et al. (2013). J. Geophys. Res. 119, 6994–6997. doi:10.1002/2014JA019896
Michael, A. J. (2011). Random variability explains apparent global clustering of large earthquakes. Geophys. Res. Lett. 38, L21301. doi:10.1029/2011GL049443
Namgaladze, A. A., Klimenko, M. V., Klimenko, V. V., and Zakharenkova, I. E. (2009). Physical mechanism and mathematical modeling of earthquake ionospheric precursors registered in total electron content. Geomagn. Aeron. 49 (2), 252–262. doi:10.1134/S0016793209020169
Nishihashi, M., Hattori, K., Jhuang, H. K., and Liu, J. Y. (2009). Possible spatial extent of ionospheric GPS-TEC and NmF2 anomalies related to the 1999 Chi-Chi and Chia-Yi earthquakes in Taiwan. Terr. Atmos. Ocean. Sci. 20, 779–789. (T). doi:10.3319/tao.2009.01.22.01(t)
Ovalle, E., Villalobos, C., Bravo, M., and Foppiano, A. (2013). Maximum electron concentration and total electron content of the ionosphere over Concepción, Chile, prior to the 27 February 2010 earthquake. Adv. Space Res. 52, 1274–1288. doi:10.1016/j.asr.2013.07.005
Parrot, M., and Li, M. (2018). “Statistical analysis of the ionospheric density recorded by DEMETER during seismic activity,” In pre-earthquake processes: a multidisciplinary approach to earthquake prediction studies (geophysical monograph 234). Editors D. Ouzounov, S. Pulinets, K. Hattori, and P. Taylor (Washington, DC: American Geophysical Union John Wiley and Sons, Inc.).
Pulinets, S. A., and Boyarchuk, K. (2004). Ionospheric precursors of earthquakes. Berlin: Springer Press.
Pulinets, S. A., and Davidenko, D. V. (2018). The nocturnal positive ionospheric anomaly of electron density as a short-term earthquake precursor and the possible physical mechanism of its formation. Geomagn. Aeron. 58, 559–570. doi:10.1134/s0016793218040126
Pulinets, S. A., Krankowski, A., Hernandez-Pajares, M., Marra, S., Budnikov, P., Zakharenkova, I., et al. (2021). Ionosphere sounding for pre-seismic anomalies identification (INSPIRE): Results of the Project and perspectives for the short-term earthquake forecast. Front. Earth Sci. 9, 610193. doi:10.3389/feart.2021.610193
Pulinets, S. (2012). Low-latitude atmosphere-ionosphere effects initiated by strong earthquakes preparation process. Int. J. Geophys 2012, 1–14. doi:10.1155/2012/131842
Pulinets, S., and Ouzounov, D. (2011). Lithosphere-Atmosphere-Ionosphere Coupling (LAIC) model-An unified concept for earthquake precursors validation. J. Asian Earth Sci. 41 (4), 371–382. doi:10.1016/j.jseaes.2010.03.005
Rishbeth, H. (2006). Ionoquakes: Earthquake precursors in the ionosphere? Eos. Trans. Am. Geophys. Union 87, 316. doi:10.1029/2006EO320008
Shah, M., Ahmed, A., Ehsan, M., Khan, M., Tariq, M. A., Calabia, A., et al. (2020). Total electron content anomalies associated with earthquakes occurred during 1998-2019. Acta Astronaut. 175, 268–276. doi:10.1016/j.actaastro.2020.06.005
Shah, M., and Jin, S. (2015). Statistical characteristics of seismo-ionospheric GPS TEC disturbances prior to global Mw≥5.0 earthquakes (1998–2014). J. Geodyn. 92, 42–49. doi:10.1016/j.jog.2015.10.002
Su, Y. C., Liu, J. Y., Chen, S. P., Tsai, H. F., and Chen, M. Q. (2013). Temporal and spatial precursors in ionospheric total electron content of the 16 october 1999Mw7.1 hector mine earthquake: Ionoquack OFM7.1 hector mine earthquake. J. Geophys. Res. 118 (10), 6511–6517. doi:10.1002/jgra.50586
Sun, Y., Liu, J., Lin, C., Tsai, H. F., Chang, L. C., Chen, C. Y., et al. (2016). Ionospheric f2 region perturbed by the 25 april 2015 Nepal earthquake: Ionosphere perturbed by Nepal earthquake. J. Geophys. Res. 121, 5778–5784. doi:10.1002/2015JA022280
Tang, J., Yao, Y., and Zhang, L. (2015). Temporal and spatial ionospheric variations of 20 April 2013 earthquake in Yaan, China. IEEE Geosci. Remote Sens. Lett. 12, 2242–2246. doi:10.1109/LGRS.2015.2463081
Xu, T., Chen, Z., Li, C., Wu, J., Hu, Y., and Wu, Z. (2011). GPS total electron content and surface latent heat flux variations before the 11 March 2011 M9.0 Sendai earthquake. Adv. Space Res. 48 (8), 1311–1317. doi:10.1016/j.asr.2011.06.024
Yao, Y., Chen, P., Wu, H., Zhang, S., and Peng, W. (2012). Analysis of ionospheric anomalies before the 2011 Mw 9.0 Japan earthquake. Chin. Sci. Bull. 57, 500–510. doi:10.1007/s11434-011-4851-y
Zakharenkova, I. E., Shagimuratov, I. I., Tepenitzina, N. Y., and Krankowski, A. (2008). Anomalous modification of the ionospheric total electron content prior to the 26 September 2005 Peru earthquake. J. Atmos. Sol-terr. Phy. 70 (15), 1919–1928. doi:10.1016/j.jastp.2008.06.003
Zhang, X., Shen, X., Zhao, S., Yao, L., Ouyang, X., and Qian, J. (2014). The characteristics of quasistatic electric field perturbations observed by DEMETER satellite before large earthquakes. J. Asian Earth Sci. 79 (2), 42–52. doi:10.1016/j.jseaes.2013.08.026
Zhou, Y., Wu, Y., Qiao, X., and Zhang, X. (2009). Ionospheric anomalies detected by ground-based GPS before the Mw7.9 Wenchuan earthquake of May 12, 2008, China. J. Atmos. Sol-terr. Phy. 71, 959–966. doi:10.1016/j.jastp.2009.03.024
Zhu, F., Zhou, Y., Lin, J., and Su, F. (2014). A statistical study on the temporal distribution of ionospheric TEC anomalies prior to M7.0+ earthquakes during 2003-2012. Astrophys. Space Sci. 350, 449–457. doi:10.1007/s10509-014-1777-2
Zolotov, O. V., Knyazeva, M. A., and Romanovskaya, Y. V. (2019). Computer analysis of total electron content in the Earth’s ionosphere in problems of searching for and detection of earthquake precursors: Current problems and challenges. Russ. J. Phys. Chem. B 13 (4), 681–684. doi:10.1134/S1990793119040146
Keywords: shallow strong earthquakes, seismo-ionospheric anomaly, VTEC, statistical analysis, different lengths of sliding window
Citation: Ma Y, Zhou X, Yang Y, Hu L, Dong H and Yan R (2023) Statistical analysis of ionospheric vertical total electron content anomalies before global Mw≥6.0 shallow earthquakes during 2000–2020. Front. Earth Sci. 11:1137177. doi: 10.3389/feart.2023.1137177
Received: 04 January 2023; Accepted: 03 April 2023;
Published: 14 April 2023.
Edited by:
Giovanni Martinelli, National Institute of Geophysics and Volcanology, ItalyReviewed by:
Patricl Taylor, National Aeronautics and Space Administration (NASA), United StatesAngelo De Santis, Istituto Nazionale di Geofisica e Vulcanologia (INGV), Italy
Funchun Chen, Chinese Academy of Sciences (CAS), China
Copyright © 2023 Ma, Zhou, Yang, Hu, Dong and Yan. This is an open-access article distributed under the terms of the Creative Commons Attribution License (CC BY). The use, distribution or reproduction in other forums is permitted, provided the original author(s) and the copyright owner(s) are credited and that the original publication in this journal is cited, in accordance with accepted academic practice. No use, distribution or reproduction is permitted which does not comply with these terms.
*Correspondence: Xiaohui Zhou, xhzhou@sgg.whu.edu.cn