- School of Chinese Materia Medica, Beijing University of Chinese Medicine, Beijing, China
Introduction: Environmental changes have led to changes in the suitable areas for growing certain medicinal plants, which might lead to changes in cultivation areas and quality of medicinal materials. Polygonatum kingianum is a commonly used medicinal plant and, with its growing demand, the sustainable development of P. kingianum is particularly important.
Methods: Therefore, this study comprehensively considered environmental variables, such as climate, soil, and topography, collected 181 valid distribution records, and used the MaxEnt to predict and evaluate the changes and potential distribution of P. kingianum.
Results: The results showed that the current high-suitability areas (31,534.60 km2) for this medicinal plant are mainly distributed in the Southern part of Yunnan Province, western part of Guangxi Province, and Southwestern part of southwestern Guizhou Province. Under a future climate change scenario, the area of suitable habitats showed a trend of first expansion and then contraction, but the overall trend still expanded. The medicinal plant’s migration trend to the northwest of Guizhou Province and northeast of Yunnan Province was more clear. At the Chinese scale, temperature and precipitation were the important factors affecting the medicinal plant’s distribution. At the national scale, Bio7, Bio12, Bio17, Bio11, S_USDA_TEX_CLASS, and Elevation were key variables affecting P. kingianum distribution, whose cumulative value of percent contribution was 93.6%. At a more precise scale, precipitation, soil variables, and altitude became the key factors affecting its distribution. Among them, S_USDA_TEX_CLASS has the highest contribution rate.
Discussion: Overall, the results of this study provided a reference for the determination of suitable planting areas of P. kingianum in China.
1 Introduction
Polygonatum kingianum Coll. et Hemsl is one of the original plants of the “Polygonati Rhizoma” medicinal materials. It has the effect of nourishing qi and nourishing yin and benefiting kidney (National Pharmacopoeia Committee, 2020). It is the main component of several common medicines, such as Ganluoxin pills, Huaqi capsules, Huangjingzanyu capsules, and Huoxuetongmai tablets. Modern medicine has shown that P. kingianum has an effect in treating diseases, such as diabetes, Alzheimer’s disease, and cancer (Liu et al., 2021). As the market demand for P. kingianum continues to grow, wild resources have difficulty meeting demand Jiao, 2018; Cui et al., 2021. Therefore, analyzing and predicting the changes in P. kingianum’s distribution in the future is a key problem to ensuring the sustainable use of their resources. This medicinal plant likes a cool and humid environment, which is mainly distributed in Yunnan, Guizhou, and Guangxi Provinces (Lu, 2022).
Global warming is having a major impact on all aspects of medicinal plants. For example, the average temperature difference between day and night and precipitation in the coldest season are key environmental factors that affect the distribution of Coptis chinensis, which can affect medicinal quality by regulating key genes in the alkaloid biosynthesis pathway of the medicinal plant (Li, 2021). The content of Saposhnikovia divaricata was negatively correlated with pH, conductivity, available phosphorus and available calcium in soil (Guo, 2020). To predict the changes in suitable habitats and help production, plants distribution models based on environmental variables has become a common method in many studies. Among them, the MaxEnt model is the most efficient (Qi et al., 2022).
The MaxEnt model is based on maximum entropy theory and predicts the potential distribution of P. kingianum (Philips et al., 2004). The model simplifies ecosystem complexity and its prediction results from a small sample size are also accurate and reliable. It has been recognized and used by many scholars (Lu et al., 2022). In this study, the MaxEnt model was used to analyze the changing trend of suitable areas of P. kingianum in different periods of climate change, to provide guidance for its production practice.
2 Materials and methods
2.1 Processing of the distribution data of P. kingianum
By searching the Global Biodiversity Information Facility (GBIF, https://www.gbif.org/), Chinese Virtual Herbarium (SVH, http://www.cvh.ac.cn/), National Specimen Information Infrastructure (NSII, http://www. nsii. org.cn), Plant Photo Bank of China (PPBC, http://ppbc.iplant.cn), and previous literature, a total of 726 occurrence records of P. kingianum in China were collected, mainly distributed in Southwest China, including Yunnan, Guangxi, Sichuan, Guizhou, and Hunan (Akaike, 1973; Fick and Hijmans, 2017; Yao et al., 2021). To increase accuracy, only one sampling point was selected for each raster (1 × 1 km). (Ye et al., 2020). Therefore, after removing latitude and longitude duplication and wrong distribution points, there were 181 occurrence records obtained by buffer analysis, which were saved in. csv format for the MaxEnt model (Figure 1).
2.2 Handling of environmental variables
The 19 bioclimatic variables were obtained from the WorldClim database (http://www.worldclim.org/) and the spatial resolution of the data at 2.5 m. Among them, climate data in the past, including LIG (last interglacial period), LGM (last glacial maximum), and MH (middle Holocene). Climate data for the current period was baseline data (1970–2000) (Fick and Hijmans, 2017). Climate data in the future: the 2050s, 2070s, and 2090s (average of forecasts for 2041–2060, 2061–2080, and 2081–2100, respectively). These climate data was selected from one global climate model (BCC-CSM2-MR, the Beijing Climate Center Climate System Model) of the Coupled Model Inter-comparison Project Phase 6 (CMIP6), and two shared socioeconomic pathways (SSP126 and SSP585) (Zhan et al., 2022). There are 4 scenarios in the BCC-CSM2-MR model: a future with sustainable and low radiative forcing (SSP126), a future with no significant changes in socioeconomic models compared to past models and moderate forcing (SSP242), a low level of economic growth, as the future of severe environmental degradation and moderate high radiative forcing (SSP370) and a future of highly industrialized, fossil fuel-based, and high radiative forcing (SSP585) (Guo et al., 2022). SSP126 and SSP585 were chosen because of a combination of local realities and their good significance (Yao et al., 2021).
The elevation variables were obtained from the WorldClim database (http://www.worldclim.org/). And the slope and aspect were obtained by ArcGIS software, with the data spatial resolution at 2.5 m. The 36 soil variables were obtained from the Harmonized World Soil Database (http://www.fao.org/soils-portal/soil-survey/soil-maps-and-databases/harmonized-world-soil-database-v12/en/) (FAO/IIASA/ISRIC/ISSCAS/JRC, 2012). Therefore, a total of 58 environmental variables (Supplementary Material S1; Supplementary Table S1) were considered in the current period model analysis. Past and future scenarios for terrain and soil variables were not available. ArcGIS software (Version 10.4.1) could extract and convert the raster data of environmental data and obtain the. asc format required for operation of the MaxEnt model.
Using the MaxEnt model for multiple operation analysis, the value of an environmental variable (percent contribution >0) corresponded to the distribution points extracted for correlation analysis. When |Pearson| was >0.85 between two variables, the smaller contribution rate was eliminated, with 10 environmental factors finally obtained to participate in model analysis.
2.3 Modeling parameter optimization process
This process is realized by R software. The complexity of the MaxEnt model is closely related to its regularization multiplier (RM) and feature combination (FC) (Zhu et al., 2016). In its default setting, the RM was 1. There were 5 features in MaxEnt, namely linear features (L), quadratic features (Q), hinge features (H), threshold features (T), and product features (P; collectively, LQHTP). An appropriate parameter optimization process was beneficial for avoiding errors caused by overfitting of the model. The ENMeval package evaluated the degree of model optimization by testing the Delta AIC value and omission rate (OR) corrected by the MaxEnt model under different parameters (Peterson et al., 2008; Warren et al., 2014; Zhu et al., 2021). Among them, the Akaike information criterion correction (AIC) is a standard for measuring the goodness of statistical model fitting, which can weigh the complexity of the estimated model and goodness of the model fitting data. This gives priority to the source with the smallest Delta AICc Model (Akaike, 1973). Therefore, when the omission rate was <5% and the Delta AICc the smallest, the model parameters were optimal (Cobos et al., 2019), with the input of optimized parameters entered into MaxEnt.
2.4 Species distribution modeling process and model validation
The 181 P. kingianum distribution point data and filtered environmental variable data were added to the software. 75% of the distribution points were randomly selected as the training set to establish the prediction model, and the rest were used to verify the model. The jackknife method was selected to determine the importance of each variable, chose to create response curves, and project them to past and future environmental scenarios. The rest of the parameters were set according to the results in Section 2.3 and the process repeated 10 times. The final output ASCII result file was the 10-time average and the raster values the survival probability (p-value) given as a logical value (Guo et al., 2022).
The credibility of the MaxEnt model was measured by the area under the ROC curve (AUC) (Cui et al., 2022). The AUC value was generally between 0 and 1. An AUC value from 0 to 0.5 indicated that the model prediction failed, 0.6–0.7 the prediction effect was poor, 0.7–0.8 the prediction effect general, from 0.8 to 0.9 the prediction good, and >0.9 the prediction excellent, with the closer it was to 1, the better the prediction effect (Liao et al., 2020).
2.5 Classification of suitable habitual levels and changes in core distribution
The ArcGIS format conversion tool could convert the output data of the MaxEnt model from ASCII format to Raster format and combined with the reclassify command. The suitable habitats were divided into 4 categories: highly suitable (0.7,1), moderately suitable (0.3,0.7), lowly suitable (0.1,0.3), and unsuitable habitats (0,0.1).
ArcGIS was used to reduce the range of suitable habitats in different periods to a single center point, calculated the position and change of the distribution center in different periods, revealed displacement of the centroid, and analyzed the migration trend of P. kingianum’s suitable habitats.
2.6 Analysis of suitable habitats of P. kingianum at a more precise scale
The distribution of P. kingianum in southwest China was more accurately described using environmental data with a spatial resolution of 1 km in the current period for cartographic analysis. The method in Section 2.1 was also used for distributed data processing and the method in Section 2.3 used to optimize parameters. The process was repeated 10 times using the MaxEnt software.
3 Results
3.1 Evaluation of credibility
In the model, FC and RM are initially default values. At this time, the OR of the model was 0.142857143 and Delta AICc at 54.50138876, but their values did not meet the requirements. The parameter optimization process produced a total of 1,160 candidate models, all of which were statistically significant. Of these, 860 models met the OR criteria and 2 models met the AICc criteria, ending up with 2 statistically significant models that met the criteria for both OR and AICc. The parameters of these candidate models were shown in Table 1 and Model A with Delta AICc at 0 was selected for formal modeling.
Model precision was tested based on the AUC values obtained after 10 repeated runs of the above method. The results showed that, under contemporary climate conditions, the average training AUC value of the distribution model of the suitable distribution of P. kingianum was 0.956 ±0.009 and the average testing AUC value 0.956 ± 0.0072. The testing and training AUC values of Future epochs (ssp126_2050s, ssp585_2050s, ssp126_2070s, ssp585_2070s, ssp126_2090s, and ssp585_2090s) and past epochs (LIG, LGM, and HM) were all above 0.9. The experimental results showed that simulation effects reached a very high level and that experimental results had high reliability.
3.2 Environmental variable analysis
After multiple operations using MaxEnt, the values of the environmental variables (percent contribution >0) corresponding to the distribution points were eliminated. The correlations of the 16 environmental variables obtained are shown in Figure 2 (and Supplementary Material S1, Supplementary Table S2). Among the 10 environmental variables screened for modeling according to the method in Section 2.2 were shown in Table. 2, Bio7, Bio12, Bio17, Bio11, S_USDA_TEX_CLASS, and Elev were key variables affecting P. kingianum distribution. Their cumulative value of percent contribution and permutation importance were 93.6% and 66.7%, respectively.
From jackknife test results, when only a single environmental variable was used, the environmental variables related to temperature and precipitation had the greatest impact on the regularization training gain, test gain, and AUC values (Figure 3). The gain values were all >1.3 and AUC values all >0.9, which indicated that these environmental variables might have had the most useful information and were important environmental variables affecting the medicinal plant’s geographical distribution. In general, temperature and precipitation were important factors affecting its distribution.
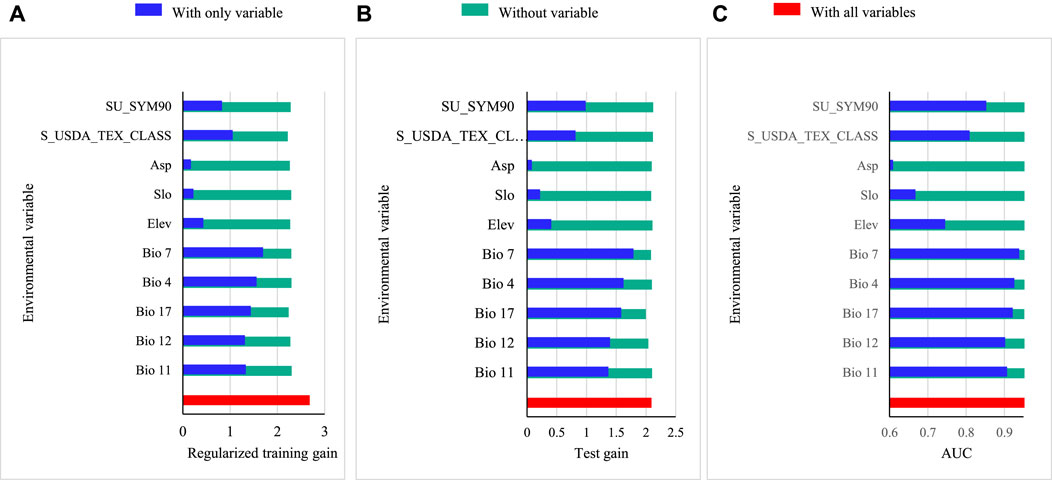
FIGURE 3. Jackknife test of the importance of environment variables in Maxent. (A) Jackknife of regularized training gain for P. kingianum (A); Jackknife of test gain for P. kingianum, (B); and Jackknife of AUC for P. kingianum, (C).
3.3 Changes in habitats in the past period
The distribution of P. kingianum is shown in Figures 4A–D. The areas of all periods are shown in Figure 5. During the LIG, the suitable habitats of P. kingianum were limited and there were lowly suitable habitats in most of southern Yunnan, Guangxi, Guangdong, Fujian, Hainan, and Taiwan Provinces (Figure 4A). Some areas had moderately suitable or highly suitable habitats. The coverage suitable habitats area was about 403,824.00 km2. Compared with the current, the lowly suitable habitats in LIG was reduced by 118,910.91 km2, moderately suitable habitats reduced by 551,864.70 km2, and highly suitable habitats reduced by 31,534.60 km2.
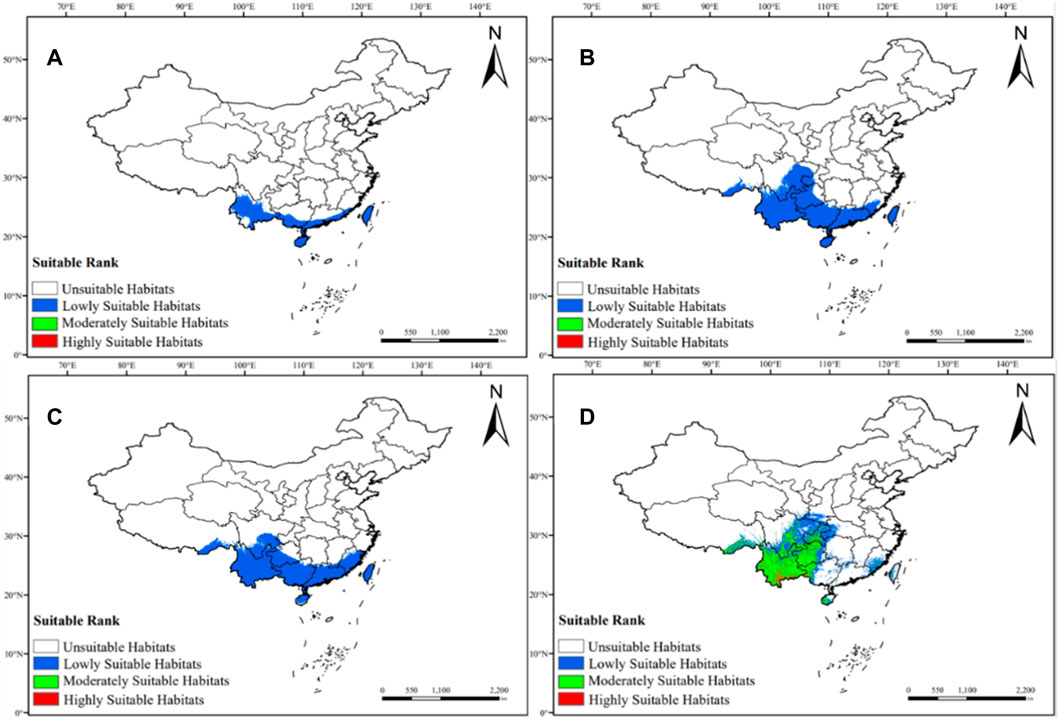
FIGURE 4. Distribution of P. kingianum in China under past and current climate scenarios. LIG; LGM; MH; and Current [(A–D), respectively].
During the LGM, the range of suitable habitats expanded significantly, mainly in southern China (Figure 4B). Compared with the LIG, the area covered by suitable habitats in the LGM expanded by 737,525.29 km2. Although the climate was cold, the area of suitable habitats for P. kingianum was the largest (Figure 5). This might have been since Yunnan Province et al. were close to the inland and Hainan and Taiwan Provinces close to tropical areas, where the relatively warm and humid climate was suitable for the medicinal plant’s growth (Zhu et al., 2021).
During the HM, suitable habitats began to decrease towards Yunnan Province et al., which might have been caused by high temperature during this period (Figure 4C). Compared with the HM, the areas of moderately and highly suitable habitats in the current period have expanded (Figure 4D), increased by 551,325.70 km2 and 31,534.60 km2, respectively, while the area of lowly suitable habitats decreased by 564,329.94 km2. The range of suitable habitats in Guangdong, Guangxi, and Fujian Provinces during the HM was greatly reduced in the current period, which might have been because the summer temperature in China during the HM was generally slightly higher than that in the lower reaches of the Yellow River in northern China and the southeast of the Northeast, which was slightly higher than that of modern times. However, in the current period, the Southeastern part of China was affected by the monsoon climate, causing this phenomenon (Zhu et al., 2021).
3.4 Changes in habitats in the current and optimal environmental conditions
In this period, the suitable habitat area for P. kingianum cultivation was 1,106,134.21 km2. The highly suitable area was 31,534.60 km2 (Figure 5), distributed in Honghe, Pu’er, and Dehong in southern Yunnan Province, Baise and Hechi in western Guangxi Province and Qianxinan in southwestern Guizhou Province (Figure 4D). The moderately suitable area was about 552,317.53 km2, including surrounding area distribution habitats (Yunnan Province, central and western Guizhou, western Guangxi, southern Sichuan, and western Hainan Provinces). The predicted results of this study were in good agreement with the actual distribution (Yin and Qian, 2020).
3.5 Changes in P. kingianum’s habitats in the future
3.5.1 Changes in suitable habitats
Figure 6 shows the conditions of suitable areas of P. kingianum under different future periods and climate scenarios. Changes in the area for each future period and climate scenario compared to the current (Figure 4D) are shown in Figure 7 and Table 3. In SSP126 and SSP585 scenarios, future changes in the total suitable areas showed a trend of first increasing and then decreasing. However, the total area of suitable areas in 2090S still increased compared to the current. This might have been caused by a relatively large reduction in the area of lowly suitable habitat area and a small increase in the area of highly suitable habitat area. Among them, the variation range of SSP585 scenario was larger than that of SSP126 scenario. Moreover, under SSP126 scenario, the maximum suitable habitat appeared in 2050S, at 1,599,152.55 km2; under the SSP585 scenario, the maximum suitable habitat appeared in 2070S, at 1,666,506.18 km2. Under the two scenarios, the area of suitable habitat in 2090S increased by 200,265.03 and 178,597.01 km2, respectively, compared with the current increase. This showed that it was suitable to scientifically expand the planting area of P. kingianum in the future.
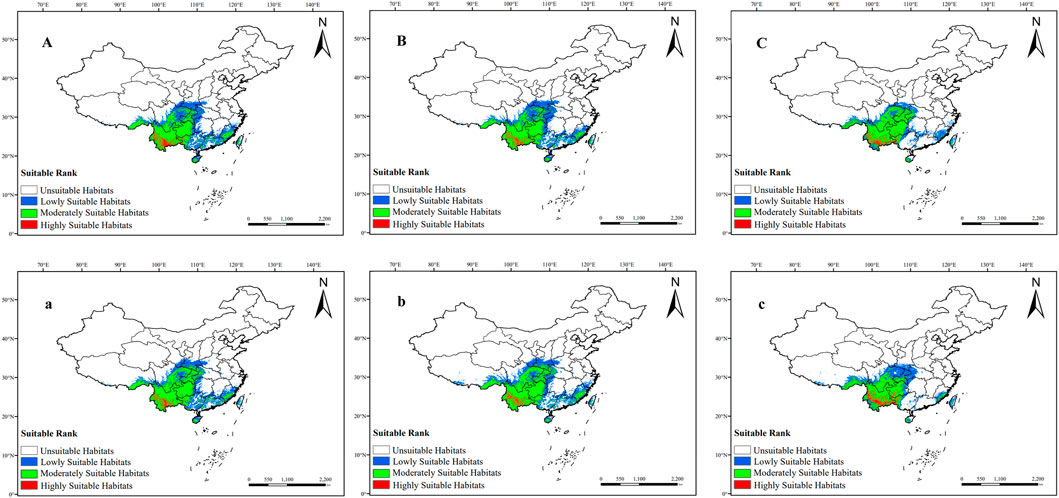
FIGURE 6. Suitable habitats for P. kingianum under different future climate scenarios. 2050S, SSP126; 2070S, SSP126; and 2090S SSP126 [(A–C), respectively] and 2050S, SSP585; 2070S, SSP585; and 2090S SSP585 [(a–c), respectively].
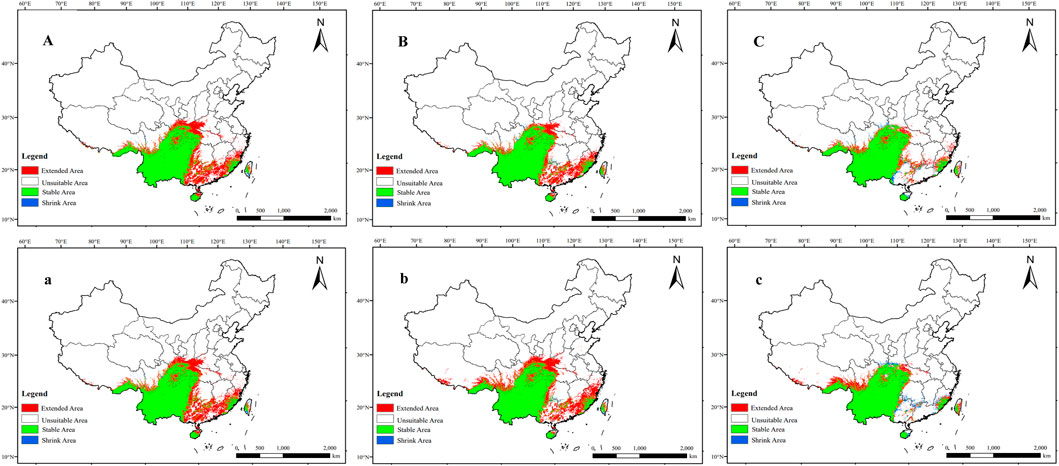
FIGURE 7. Unchanged, contracted and expanded suitable habitats for P. kingianum under different future climate scenarios. 2050S, SSP126; 2070S, SSP126; and 2090S SSP126 [(A–C), respectively]; and 2050S, SSP585; 2070S, SSP585; and 2090S SSP585 [(a–c), respectively].
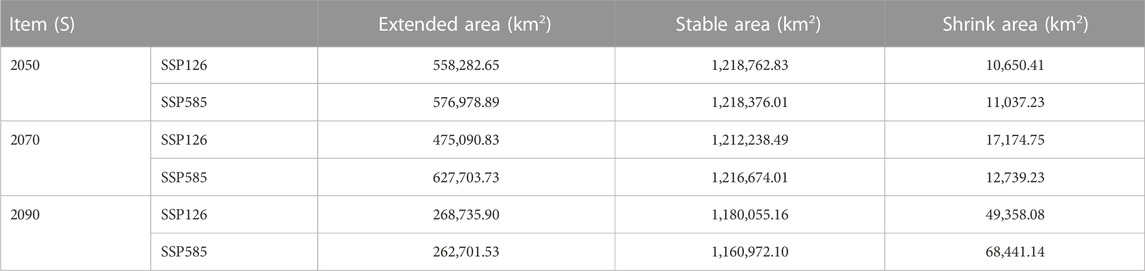
TABLE 3. Stable, shrink, and increased areas (km2) of suitable habitat for P. kingianum in the future.
3.5.2 Change of P. kingianum’s centroid displacement
The distribution cores of P. kingianum during the LIG, LGM, and HM periods were located in Debao county in Baise city, Guangxi Province (106.96°E, 23.36°N), Ziyun county in Anshun city, Guizhou Province (106.34°E, 25.46°N), and Tian’e county in Hechi city, Guangxi Province (106.85°E, 25.02°N; Figure 8). In the past period, the distribution core of P. kingianum showed a trend of moving northward as a whole. In the current, the distribution core was located in Hezhang county in Bijie city, Guizhou Province (104.75°E, 27.12°N). Compared with the HM, the distribution core shifted to the northwest by 313.50 km. Under the SSP126 environment, the distribution core moved southeast to Xifeng County in Guiyang City, Guizhou Province in 2050S (179.28 km migration distance), moved northwest to Qianxi County, Bijie City, Guizhou Province in 2070S (43.70 km migration distance), continued to move northwest to Qixingguan County in Bijie City, Guizhou Province in 2090S (82.71 km migration distance). Under the SSP585 environmental scenario, the distribution core moved northeast to Qianxi County in Bijie City, Guizhou Province in 2050S (157.53 km migration distance), moved northwest to Jinsha County in Bijie City, Guizhou Province in 2070S (44.79 km migration distance), moved southwest to Hezhang County in Bijie City, Guizhou Province in 2090S (163.19 km migration distance). On the whole, the distribution of P. kingianum in China generally showed a trend of migration from Southeast to northwest and the migration range of SSP585 larger than that of SSP126. This might have been due to the former fluctuating more in climate change than the latter.
3.6 Comparative analysis of the distribution of P. kingianum at the regional and national scales in Southwest China
Combined with the above distributions, to further reduce error, this part of the study used the 30 s scale to accurately predict the current distribution of P. kingianum in Yunnan, Guizhou, Guangxi, and Sichuan Provinces and Chongqing City (Figures 9, 10). Compared with the national scale results, the AUC value of this model decreased to 0.748 ± 0.030, which might have been due to the insufficient variety of environmental variables, but the accuracy could still be judged more accurately. According to the percent contribution of the modeling variables (Table 4), S_USDA_TEX_CLASS, bio17, bio6, Elev, and bio18 were the key variables affecting the medicinal plant’s distribution. The key factors for its distribution in southwest China were different from the results predicted on the national scale. Due to narrowing of the forecast range, there was little change in temperature within the region, but significant differences in precipitation, elevation, and soil. This reasonably explained that the fine-scale falling water variable had a greater effect than the temperature variable. This part of the experiment showed that the more precise the scale of environmental variables and the smaller the prediction range, the more consistent the results were with the actual situation.
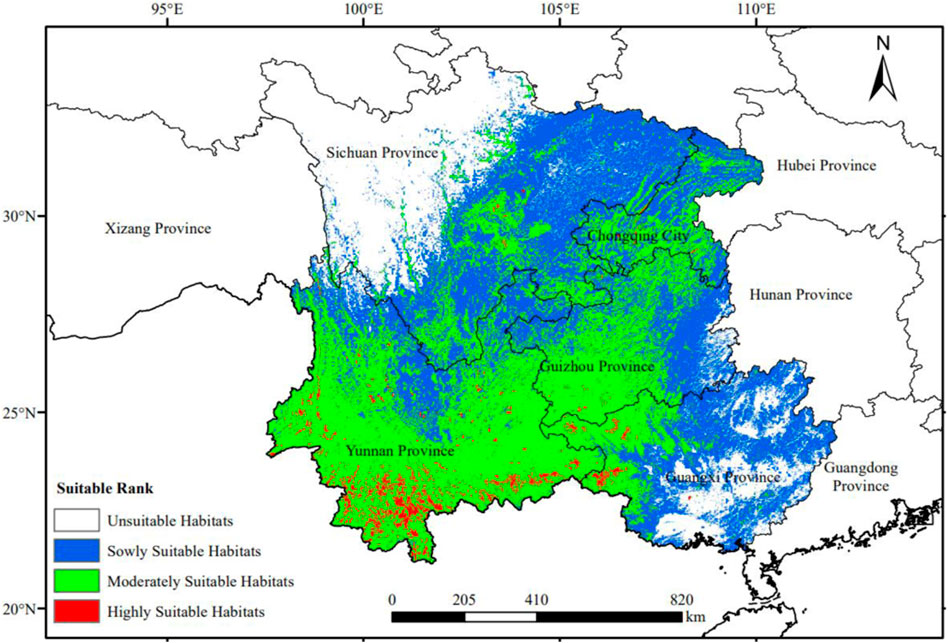
FIGURE 9. Distribution of the suitable habitats for P. kingianum in Southwest China (Yunnan, Guizhou, Guangxi, and Sichuan Provinces, and Chongqing City).
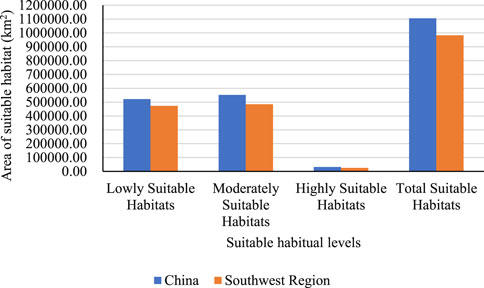
FIGURE 10. Comparison of the area of suitable habitats of P. kingianum in China and Southwest China (Yunnan, Guizhou, Guangxi, and Sichuan Provinces, and Chongqing City) in the current period.
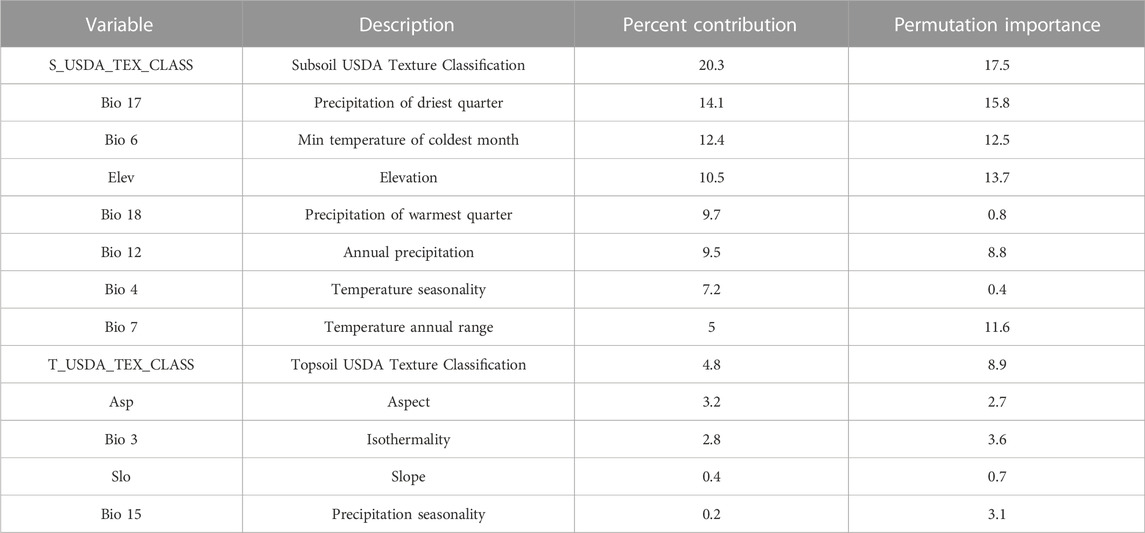
TABLE 4. Contribution and permutation importance of 13 environmental variables involved in modeling.
4 Discussion
As one of the “Three Immortal Grasses”, P. kingianum is known as “Immortal Surplus Grain,” and it is the most potential traditional advantageous industry inChina. The planting industry of P. kingianum has further promoted the leap-forward development of the under-forest economy, cultivated new kinetic energy for industrial development, and made great contributions to increasing the income of farmers in the mountains (Yao et al., 2018; Yin et al., 2020). This study predicts the current and future suitable areas of P. kingianum and provides a reference for expanding its planting area and improving planting patterns. In addition, it was found that southeastern Yunnan Province, southwestern Guizhou Province, western Guangxi Province and Chongqing City were suitable for planting P. kingianum. Among them, the highly suitability area is concentrated in southern Yunnan Province.
The present results might also have been affected by several uncertainties. First, parameters have a big impact on most modeling (Zhuo et al., 2020). At present, some toolkits provide related tools for parameter optimization, such as ENMTools and Rsoft. Even if the model parameters were adjusted, it was difficult to ensure that the state was completely optimal. (Qiao et al., 2015). Furthermore, thresholds might lead to biased results, for example, predicted plants distributions that are too large or too small. In this study, the average value of the thresholds at the same level in each period under the natural breakpoint method was used as the final threshold to reduce error. Second, the MaxEnt model does not consider the interaction between plants and the environment, which will lead to errors. It also does not take into account factors, for example, biological interactions in the habitat, which are an important part of ecological impact. In this study, these problems were ameleliorated to a certain extent through parameter optimization but, at the same time, showed that the MaxEnt model hs room for further development.
P. kingianum has been distributed in the southwest China since ancient times. The distribution area of LIG, LGM and HM period is generally lower than the current period. This might have been caused by an unstable climate, such as extreme cold events (Zhan et al., 2022). P. kingianum likes a mild climate (Nian et al., 2017), which led to the distribution result of LIG. According to previous researches and records, most P. kingianum is naturally distributed in Guizhou, Yunnan, Sichuan, Guangxi Provinces, and other places, which is similar to the results of this study Proven is up to the reader to conclude from this study, which demonstrated that the results are highly reliable (Ye et al., 2020).
By comparing the limiting factors of the distribution of suitable areas of P. kingianum in China and Southwest China, we found that one of the top five factors was different, and the order was also different. In the range of China, the influence of temperature and precipitation on the suitable area of P. kingianum was greater than that of soil factors, which was similar to the results of Yao et al. (2018) to a certain extent. However, the influence of soil factors on the suitable areas of P. kingianum was greater than that of temperature and precipitation in the Southwest. This phenomenon is also common in previous studies and our team’s predictions for other medicinal plants. Because of China’s vast territory and vast latitude and longitude, climate and precipitation vary significantly depending on location. The southwest area is small, and the changes in temperature and precipitation are the same, which highlights the influence of different soil factors on the growth of P. kingianum in different producing areas in this area. Therefore, S_USDA_TEX_CLASS becomes the limiting factor for the distribution of P. kingianum in the southwest area. In addition, elevation data with a precision of 30 s was used in this study, while only 36 of the many factors were used for soil factor. In future studies, if the accuracy and quantity of data are more optimized, it is believed that the predicted results will be further improved based on this study.
By analyzing the characteristics of P. kingianum of suitable areas in the past, present and future periods, it was found that the spatial distribution pattern of suitable areas shifted to the direction of “Northwest—Northeast—North”. The center of gravity of the suitable areas of P. kingianum moved northward and the future climate warming may be an important factor leading to the change of the suitable area of P. kingianum. Both the current and future centroid shifts occur in Guizhou Province, because the centroid is a collection of low, medium and high suitability areas, and the high suitability areas occupy a relatively low proportion of the area. Therefore, there is a certain deviation between the location of the centroid and the location of the genuine producing area, which is within the consideration range and does not adversely affect the result. These were more consistent with previous textual research on herbal medicine (Jiang et al., 2017).
Among the three medicinal plants derived from P. kingianum, the quality of P. kingianum is the best. On this basis, it is possible to predict its future distribution changes and the key factors affecting its growth, so as to be able to plant P. kingianum more scientifically and rationally and promote the development of traditional Chinese medicine.
5 Conclusion
In this study, the parameter-optimized MaxEnt model was used to evaluate and predict the distribution of suitable habitats for P. kingianum at all periods. Based on environmental variable analysis and the univariate response results, the most suitable environmental conditions for the growth of P. kingianum were: temperature annual range at 16°C–20°C, annual precipitation at 1,100–1,400 mm, precipitation of driest quarter at 50–80 mm, mean temperature of coldest quarter at 10°C–15 °C, and elevation at 1,300–2,000 m. The prediction effects at different scales were then compared and the results showed that the area of suitable habitats for this medicinal plant reached the highest level in the LGM period in the past. In the current period, the most suitable habitat was located in the southeastern part of Yunnan Province, and it was expected that the suitable habitat would shift to the northwest of Guizhou Province and northeast of Yunnan Province in the future. In addition, the prediction of the small-scale precision scale was more realistic, but the collection and selection of corresponding environmental variables were more demanding. In conclusion, the results of this study provide a useful reference for the determination of the suitable planting area of P. kingianum, predict the present and future high-suitable areas, and rationally arrange and grasp the cultivation technology in these areas to ensure high yield and high quality, which can meet the market demand, and provide a theoretical basis for the actual production.
Data availability statement
The original contributions presented in the study are included in the article/Supplementary Material, further inquiries can be directed to the corresponding author.
Author contributions
All authors listed have made a substantial, direct, and intellectual contribution to the work and approved it for publication.
Funding
National Natural Science Foundation of China (No.82274090), China Agriculture Research System of MOF and MARA (Grant No. CARS-21) supported this work.
Conflict of interest
The authors declare that the research was conducted in the absence of any commercial or financial relationships that could be construed as a potential conflict of interest.
Publisher’s note
All claims expressed in this article are solely those of the authors and do not necessarily represent those of their affiliated organizations, or those of the publisher, the editors and the reviewers. Any product that may be evaluated in this article, or claim that may be made by its manufacturer, is not guaranteed or endorsed by the publisher.
Supplementary material
The Supplementary Material for this article can be found online at: https://www.frontiersin.org/articles/10.3389/feart.2023.1111878/full#supplementary-material
References
Akaike, H. (1973). “Information theory and an extension of the maximum likelihood principle,” in 2nd international symposium on information theory. Editors B. N. Petrov, and F. Csáki (Budapest: Akadémiai Kiadó), 267–281.
Cobos, M. E., Peterson, A. T., Barve, N., au, , and Osorio-Olvera, L., 2019, kuenm: an R package for detailed development of ecological niche models using Maxent. PeerJ. 7: e6281. doi:10.7717/peerj.6281
Cui, H., Jing, Z. X., and Zhou, H. (2022). Research on the regionalization of Aconitum carmichaelii Debx. (Fuzi) ecological suitability distribution based on MaxEnt model. Southwest China Journal of Agricultural Sciences, Available at: http://kns.cnki.net/kcms/detail/51.1213.S.20220801.1825.002.html.
Cui, K. S., Xiao, T., Li, H. P., Khan, A., Bawa, G., Chen, G. P., et al. (2021). Research progress of China's Polygonatum germplasm resources. Jiangsu Agric. Sci. 49 (11), 35–39. doi:10.15889/j.issn.1002-1302.2021.11.006
FAO/IIASA/ISRIC/ISSCAS/JRC (2012). Harmonized world soil database. Rome, Italy and IIASA Laxenburg, Austria: FAO. version 1.2.
Fick, S. E., and Hijmans, R. J. (2017). WorldClim 2: New 1–km spatial resolution climate surfaces for global land areas. Int. J. Climatol. 37, 4302–4315. doi:10.1002/joc.5086
Guo, X. (2020). Relationship between quality and ecological environment and its suitable growth areas of Saposhnikovia divaricate. Changchun, China: Jilin Agricultural University. doi:10.27163/d.cnki.gjlnu.2020.000376
Guo, Y. B., Mo, K., Wang, G. R., Zhang, Y., Zhang, W., Zhou, J. G., et al. (2022). Analysis of prediction and spatial-temporal changes of suitable distribution of gastrodiae rhizoma under future climate conditions. Chin. J. Inf. Traditional Chin. Med. doi:10.19879/j.cnki.1005-5304.202111032
Jiang, W., Ye, C. S., Wu, Z. G., and Tao, Z. M. (2017). Textual research on the materia medica of polygonati rhizoma. J. Chin. Med. Mater. 40 (11), 2713–2716. doi:10.13863/j.issn1001-4454.2017.11.049
Jiao, J. (2018). Astudy on germplasm resources of polygonati rhizoma. Northwest A&F University. Xianyang, China .
Li, J. J. (2021). Prediction of potential distribution area of Coptis chinensis Franch. and analysis of key environmental factors affecting its quality formation. Chengdu Univ. Traditional Chin. Med. doi:10.26988/d.cnki.gcdzu.2021.000706
Liao, J. F., Yi, Z. L., Li, S. C., and Xiao, L. (2020). Maxent modeling for predicting the potentially geographical distribution of Miscanthus nudipes under different climate conditions. Acta Ecol. Sin. 40 (22), 8297–8305. doi:10.5846/stxb201911092361
Liu, S., Hu, S. T., Jia, Q. J., and Liang, Z. S. (2021). Advances in chemical constituents and pharmacological effects of Polygonati Rhizoma. Nat. Prod. Res. Dev. 33 (10), 1783–1796. doi:10.16333/j.1001-6880.2021.10.019
Lu, C. R. (2022). The main points of cultivation of Polygonati Rhizoma. Yunnan Agric. 2022 (02), 81–82.
Lu, L., Zhang, Z. W., Lin, M. Y., Zhao, Z. Y., Xiao, N. W., and Wang, Q. (2022). Prediction of the potential habitats of terrestrial firefly Pyrocoelia pectoralis in Beiiina based on MaxEnt model. J. Plant Prot. 49 (4), 1217–1224. doi:10.13802/j.cnki.zwbhxb.2022.2021177
National Pharmacopoeia Committee (2020). Pharmacopoeia of people's Republic of China. 11th Ed. Beijing, China: Medical Science and Technology Press, 319.
Nian, J. Y., Nian, G. F., Wang, T., Nian, Y. G., and He, W. R. (2017). Resource distribution and wild-like cultivation of Polygonatum kingianum Coll.et Hemsl. Rural Pract. Technol. J. 2017 (1), 22–24. doi:10.3969/j.issn.1673-310X.2017.01.006
Peterson, A. T., Papeş, M., and Soberón, J. (2008). Rethinking receiver operating characteristic analysis applications in ecological niche modeling. Ecol. Model. 213, 63–72. doi:10.1016/j.ecolmodel.2007.11.008
Philips, S. J., Dudik, M., and Schapire, R. E. (2004). “A maximum entropy approach to species distribution modeling,” in Proceedings of the twenty-first international conference on Machine learning, Alberta,Canada, July 4 - 8, 2004 (Banff), 83.//Association for Computing Machinery.
Qi, S., Huang, D. F., Yang, Z. Q., Lu, M. L., Xia, G. F., and Yin, D. M. (2022). Prediction of potentially suitable distribution area of houttuynia cordata Thunb.in China based on MaxEnt model. North. Hortic. 2022 (15), 148–155. doi:10.11937/bfyy.20220346
Qiao, H., Soberón, J., Peterson, A. T., and Kriticos, D. (2015). No silver bullets in correlative ecological niche modelling: Insights from testing among many potential algorithms for niche estimation. Methods Ecol. Evol. 6, 1126–1136. doi:10.1111/2041-210x.12397
Warren, D. L., Wright, A. N., Seifert, S. N., and Shaffer, H. B. (2014). Incorporating model complexity and spatial sampling bias into ecological niche models of climate change risks faced by 90 California vertebrate species of concern. Divers. Distributions 20, 334–343. doi:10.1111/ddi.12160
Yao, J. M., Liu, D., Duan, L. C., and Cai, Z. (2021). Prediction of potential distribution of diaphorina citri kuwayama in China under future climate change scenarios of CMIP6. Chin. J. Agrometeorology 42 (12), 1031–1041. doi:10.3969/j.issn.1000-6362.2021.12.005
Yao, X., Zhang, J. Y., Wan, Q. Q., Li, Y. R., and Shen, T. (2018). Potential geographical distribution of polygonatum kingianum and lts climatic suitability analysis. J. Trop. Subtropical Bot. 26 (5), 439–448. doi:10.11926/jtsb.3874
Ye, X., Zhao, G., Zhang, M., Cui, X., Fan, H., and Liu, B. (2020). Distribution pattern of endangered plant Semiliquidambar cathayensis (Hamamelidaceae) in response to climate change after the last interglacial period. Forests 11, 434. doi:10.3390/f11040434
Yin, J. H., Ynag, W. W., and Chang, X. Y. (2020). Research progress and development suggestions of Polygonati Rhizoma. Seed Sci. Technol. 38 (7), 6–9. doi:10.3969/j.issn.1005-2690.2020.07.004
Yin, S. Y., and Qian, L. H. (2020). Research progress in diversity of origin plants of Chinese medicinal polygonati rhizoma and lts utilization. Chin. Wild Plant Resour. 41 (2), 49–57. doi:10.3969/j.issn.1006-9690.2022.02.008
Zhan, P., Wang, F. Y., Xia, P. G., Zhao, G. H., Wei, M. T., Wei, F. G., et al. (2022). Assessment of suitable cultivation region for Panax notoginseng under different climatic conditions using MaxEnt model and high-performance liquid chromatography in China. Industrial Crops Prod. 2022, 114416. doi:10.1016/j.indcrop.2021.114416
Zhu, G. P., and Qiao, H. J. (2016). Effect of the Maxent model's complexity on the prediction of species potential distributions. Biodivers. Sci. 24 (10), 1189–1196. doi:10.17520/biods.2016265
Zhu, X. Y., Han, Y. C., and Wang, Z. Y. (2021). Dynamic downscaling simulation of millennial climate in chinasince the last glacial maximum——climate comparison of three typical periods. Quat. Sci. 41 (3), 842–855. doi:10.11928/jissn.1001-7410.2021.03.18
Keywords: P. kingianum, MAXENT model, climatic conditions, prediction, suitable distribution
Citation: Guo Y, Zhang S, Tang S, Pan J, Ren L, Tian X, Sun Z and Zhang Z (2023) Analysis of the prediction of the suitable distribution of Polygonatum kingianum under different climatic conditions based on the MaxEnt model. Front. Earth Sci. 11:1111878. doi: 10.3389/feart.2023.1111878
Received: 30 November 2022; Accepted: 13 January 2023;
Published: 30 January 2023.
Edited by:
Folco Giomi, Independent researcher, Padova, ItalyReviewed by:
Tao Shen, Yuxi Normal University, ChinaGbenga Akomolafe, University of Science Malaysia (USM), Malaysia
Copyright © 2023 Guo, Zhang, Tang, Pan, Ren, Tian, Sun and Zhang. This is an open-access article distributed under the terms of the Creative Commons Attribution License (CC BY). The use, distribution or reproduction in other forums is permitted, provided the original author(s) and the copyright owner(s) are credited and that the original publication in this journal is cited, in accordance with accepted academic practice. No use, distribution or reproduction is permitted which does not comply with these terms.
*Correspondence: Zilong Zhang, emhhbmd6aWxvbmc3NkAxNjMuY29t