- 1Fujian Meteorological Observatory, Fuzhou, China
- 2Fujian Provincial Key Laboratory of Disaster Weather, Fuzhou, China
Objective temperature forecast products can achieve better forecast quality by using one-dimensional regression correction directly based on the present model temperature forecast product, and the forecast accuracy can be further improved by adding appropriate auxiliary factors. In this paper, ECMWF forecast products and ground observation data from Fujian are used to revise the surface temperature at 2 m by introducing a cloud cover forecast factor based on the model temperature forecast correction method. Analysis shows that the forecast deviation of daily maximum and minimum temperature after the revision of a single-factor forecast is obviously correlated with cloud cover. A variety of prediction schemes are designed, and the final scheme is determined through comparative testing. The following conclusions are drawn: all schemes based on cloud cover grouping can improve forecast performance, and the total cloud cover scheme is generally better than the low cloud cover scheme. There is a good positive correlation between the forecast deviation of maximum temperature and the mean total cloud cover; that is, the more cloud cover, the bigger the deviation. The minimum temperature is negatively correlated with cloud cover when the cloud cover is less than 40% and positively correlated for the rest. The absolute forecast deviations of the maximum and minimum temperatures are larger when the total cloud cover is less. Whether for Tmax or Tmin forecast, the binary regression scheme after grouping consistently showed the best performance, with the lowest MAE. The final scheme was used to forecast the maximum and minimum temperature in 2021, and most verification indicators showed improvement in most forecast periods. The forecast accuracy for the 36-h daily maximum and minimum temperature is 81.312% and 91.480%, respectively, which is 2.4%–2.6% higher than the single-factor regression scheme. The forecast skill scores (FSS) reach 0.065 and 0.086, indicating that the method can effectively improve forecast quality in a stable manner and can be used for practical forecasting.
1 Introduction
In recent years, with the advancement of numerical forecast and the continuous improvement of statistical methods such as model output statistics (MOS), perfect prognosis (PP), artificial neural networks (ANN), Kalman filter (KF), and the support vector machine (SVM) (Huang and Xie, 1993; Zhang and Sha, 2001; Wang et al., 2004; Chen et al., 2005; Wu et al., 2007; Qian et al., 2010; Chen et al., 2011; Li et al., 2011), the accuracy of temperature forecasts has been greatly improved, but it is still unable to meet people’s growing demand for accurate and refined temperature forecasts. Therefore, methods of improving the accuracy of forecasts is an urgent issue. Temperature is sensitive to local weather and geographical characteristics. The MOS is the most commonly method used in daily temperature forecasting. It can introduce many forecast factors that are difficult to introduce by other methods, match local weather and climate characteristics, and make appropriate corrections to the systematic deviations of numerical models (Liu et al., 2004).
The MOS forecast method usually requires a certain length of historical data samples to achieve better forecast results. The samples should preferably have the same climate background characteristics, and the consistency factors of the samples should be as large as possible. Che et al. (2011) used the K-mean clustering method to make seasonal divisions in North China for temperature forecasts; the forecast error is generally smaller than the traditional seasonal division. Zhi et al. (2010, 2014) compared the different training periods of super-ensemble temperature forecasts and found that a sliding training period is better than a fixed training period. Wu et al. (2016) further optimized the division method of the training period. They used the quasi-symmetric sliding training period method to revise the model temperature forecast by selecting the sample data 1 month before and after the forecast date and also considered the model consistency and the sample’s climate characteristics, which significantly improved the forecast quality. However, methods that highlight the training period do not consider the influencing factor of temperature. Many scholars in China have introduced multiple factors. Liu et al. (2004) selected multiple factors for MOS forecasts, and the forecast verification results showed that the short-term temperature forecast was improved in most cases. Zhang et al. (2011) used the MOS method to select 11 factors on the basis of T213 to forecast the daily maximum and minimum temperatures of 124 stations in Yunnan Province, and the forecast results were improved, especially in summer. Zhu and Mu (2013) established a MOS forecast equation based on the WRF model, using temperature, wind, sea level pressure, relative humidity, and precipitation at Urumqi Airport as forecast factors. The accuracy of the hourly temperature forecast was significantly improved compared with the forecast results directly output by the model. The introduction of multiple factors to establish equations can improve temperature prediction, but the factors should be selected to optimize the role of the main factors.
The local variation of temperature depends on temperature advection, pressure change, atmospheric stability, and diabatic processes (Zhu et al., 2000). Liang and Huang (2006) pointed out that in the absence of large-scale system transit, the diabatic processes are the main factors that affect the temperature change in the near-surface layer, while the diabatic processes are affected by many factors, such as the sky condition, the topography, underlying surface, and vegetation type. Therefore, it is necessary to fully consider the role of cloud cover in temperature forecast. Qin et al. (2007) analyzed the relationship between cloud cover and temperature in Nanning City and found that total cloud cover has a significant negative correlation with mean temperature and maximum temperature, while low cloud cover has a significant negative correlation with maximum temperature and a significant positive correlation with minimum temperature. Zheng et al. (2013) adopted the optimized cloud scheme in GRAPES, and the surface temperature simulated by the model was closer to the observed value. Luo et al. (2014) classified the sky conditions and established the classic MOS forecast model. They selected the numerical forecast product factors corresponding to the general occurrence time of maximum and minimum temperature, which positively and significantly improved the quality of local temperature forecast. Forecasters also make adjustments to temperature forecasts by evaluating the cloud cover in practice, but the specific adjustment extent varies from person to person and cannot be uniformly regulated.
Currently, most MOS temperature forecast methods directly perform one-dimensional regression correction on the model temperature forecast product, which can achieve good correction effects. The forecast quality is not worse than that of multi-factor modeling correction and has been widely used in many meteorological departments. Different amounts of cloud cover will cause differences in the deviation between the model temperature forecast and the actual observation. Therefore, it is meaningful to introduce cloud cover forecast as an auxiliary factor to further optimize the MOS temperature forecast, but few people have studied and applied it in practice. The Fujian Provincial Meteorological Observatory divided temperature samples according to different total cloud cover, established independent models for each subset, and achieved good correction effects. It ranked first in the comprehensive skill of temperature in the 2021 National Meteorological System Intelligent Forecast Technology Method Exchange Competition. In this paper, the optimal scheme of daily maximum and daily minimum temperature forecasts based on cloud cover is selected by further studying the cloud cover groupings and comparing several schemes.
2 Data and pre-processing
2.1 Data
In this paper, the maximum and minimum temperature at 2 m, total cloud cover, and low cloud cover of ECMWF from 2018 to 2021 issued by the China Meteorological Administration were adopted. The ECMWF data are obtained twice a day at 08:00 and 20:00 (Beijing time, same below), and the forecast time period is 0–240 h, the horizontal resolution is 0.125° × 0.125°, and the time resolution is 3 h for 0–72 h and 6 h for 78–240 h. To ensure calculation efficiency and reliability of the observation data, the testing stations are 70 national meteorological stations in Fujian Province.
2.2 Data pre-processing
The inverse distance weighting interpolation method is used to interpolate the ECMWF fine grid point surface elements to the station, and the Cressman objective interpolation method is used as a reference for the weighting coefficients. This is carried out for station-based modeling and forecast. The interpolation method is as follows:
In Formula 1, Pk is the forecast value of the element at the kth station obtained by interpolation, Pij is the forecast value of the element at the grid point (i, j), Wkij is the weight factor, and m and n are the numbers of grid points in the latitudinal and longitudinal directions, respectively. The weighting factor used in this paper can be expressed as follows:
In Formula 2, R is the effective influence radius and dkij is the distance from grid point (i, j) to station k. In operational work, for the convenience of calculation, the difference between longitude and latitude is used to represent the distance, and the effective influence radius is taken as 1°.
The daily maximum temperature (Tmax) and the daily minimum temperature (Tmin) are calculated as the maximum and minimum temperatures over a 24-h period, respectively. Cloud cover (total cloud cover or low cloud cover) is calculated as the 12-h mean cloud cover, and the mean cloud cover at a given point is the mean of all available cloud cover forecasts at that point within a given forecast time period. Because the latest ECMWF data are usually obtained later than the forecast start time, the forecast in this paper is the correction of the model lag of 12 h; that is, the first day of operational forecast corresponds to the 12–36-h period of the model forecast and so on for the other forecast periods.
3 Methods
3.1 Correction method
The one-dimensional linear regression equations for Tmax and Tmin at a certain forecast period for each station are established using the least square method:
In Formula 3,
During the day, direct solar radiation can reach the earth’s surface and warm it. If there is cloud cover, the cloud layer will reflect some of the solar radiation, reducing the energy input to the surface and hindering warming. At night, the heat released from the surface dissipates upwards, causing the surface temperature to decrease. If there is cloud cover, the cloud layer can reflect the heat from the surface, thereby weakening heat dissipation and hindering cooling. Therefore, the effect of cloud cover on surface temperature is opposite during the day and night, and the reverse is true under clear skies. Because cloud cover at night and during the day has opposite effects on temperature, daytime cloud cover is used as the auxiliary factor for the correction of Tmax, and nighttime cloud cover is used as the auxiliary factor for correction of Tmin.
3.2 Training period
The quasi-symmetric mixed sliding training period method can significantly improve the quality of a temperature forecast by the MOS method and has great application value in operational work (Wu et al., 2016). This paper continues to use this method, and the total samples during the training period mixed samples from 35 days before the forecast date and samples from 35 days after the forecast day of the previous year and used sliding sampling with the forecast date.
3.3 Inspection method
To evaluate the operational performance of the MOS forecast, the mean absolute error of temperature forecast (MAE, Zhou et al., 2006), the temperature forecast accuracy (FA), and the temperature forecast skill scores (FSS) were used in this paper:
In Formula 4, FA is the percentage of the absolute deviation between the temperature forecast whose observed value does not exceed 2°C, Nr is the number of stations (times) where the value of the difference between the forecast temperature and the observed value does not exceed 2°C, and Nf is the total number of stations (times) that have been forecasted.
In Formula 5,
4 Scheme comparison and improvement
4.1 Initial scheme design
Based on the observed temperature data from 2019 to 2020 and temperature, total cloud cover, and low cloud cover forecast data from ECMWF, three schemes are designed and compared to discuss the feasibility and the improvement direction of introducing cloud cover.
Scheme 1: No grouping, using the quasi-symmetric mixed sliding training period method (one-dimensional regression) to model and revise all temperature forecast samples (Wu et al., 2016).
Scheme 2: Grouping by total cloud cover, the temperature forecast samples with total cloud cover less than the specified threshold value are grouped for separate modeling correction, and the remaining samples are grouped as another group. The exhaustive method is used for the cloud cover threshold, starting from 0% total cloud cover as the threshold, increasing to 100% at 5% intervals; 21 cloud cover values were selected as grouping thresholds for correction.
Scheme 3: Grouping by low cloud cover, the temperature forecast samples with cloud cover less than the specified threshold are grouped for separate modeling correction, and the remaining samples are grouped as another group. Groupings of the cloud thresholds are the same as in Scheme 2.
According to the forecast results of Scheme 2 (Figure 1A), the absolute forecast deviation of Tmax for the first day is less than that of no grouping (the threshold of 0% cloud cover can be approximated to no grouping). When about 30%–40% cloud cover is used as the grouping threshold, the forecast result is better, the MAE is small, and the MAE of the optimal threshold can be reduced by about 0.05°C. For the Tmin forecast (Figure 1B), the MAE of grouping is also less than that of no grouping. The improvement is obvious when grouping by the less and more cloud cover threshold intervals, and grouping by the less cloud cover threshold is slightly better than grouping by the more cloud cover threshold. Because the potential for improvement of the Tmin forecast is smaller than that of the Tmax forecast, there is not much difference between the different thresholds. The performance of Scheme 3 is similar to that of Scheme 2 and will not be presented separately.
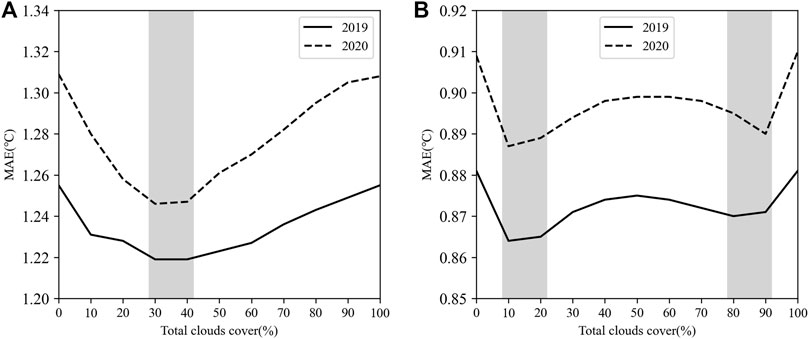
FIGURE 1. Comparison of the MAE of different total cloud cover threshold forecasts on the first day. (A) Tmax; (B) Tmin.
The optimal threshold scheme of Tmax and Tmin among the 21 grouping methods of Scheme 2 and Scheme 3 was selected to compare with Scheme 1 and the original uncorrected results. The results are shown in Figure 2. Seen from FA, the forecast results of Tmax and Tmin in all three revised schemes were significantly improved compared with the original uncorrected ones, and the improvement rate in the first 5 days was 100% or above. For Tmax, the scheme grouping by total cloud cover performed better than the other two schemes in all forecast periods in terms of FA and MAE (Figures 2A, C). The FA of the scheme grouping by low cloud cover improved compared with no grouping in the first 4 days, but it was not better on the fifth day, while the MAE improved in all time periods. For Tmin, the performance of Scheme 2 was also the best compared with the other schemes in all forecast periods (Figures 2B, D), followed by Scheme 3, and both schemes were better than Scheme 1 in terms of FA and MAE. In general, the introduction of cloud cover improved the forecast performance, and the introduction of total cloud cover was better than low cloud cover.
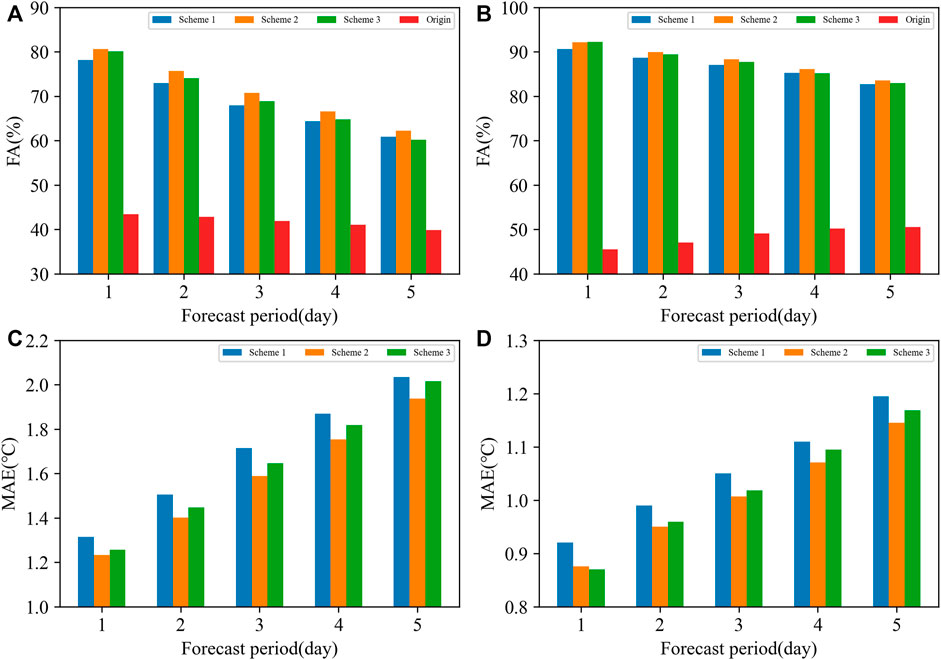
FIGURE 2. Comparison of the forecast results of the three schemes from 2019 to 2020. (A) FA of Tmax (unit: %), (B) FA of Tmin (unit: %), (C) MAE of Tmax (unit: °C), and (D) MAE of Tmin (unit: °C).
4.2 Relationship between cloud cover and temperature correction forecast deviation
In general, the least squares method is used to directly model and correct based on the modeled temperature forecast products. The ideal result of the mean deviation of the objective temperature forecast is unbiased; however, there is a significant correlation between forecast deviation of temperature and cloud cover. Figure 3 shows the relationship between the mean forecast deviation of Tmax and Tmin based on Scheme 1 and the total cloud cover with a 12–36 h forecast period in 2019 and 2020 for 70 national meteorological stations. The relationship between low cloud cover and temperature forecast is similar to the total cloud cover, which is not given in the paper. It can be seen that for Tmax (Figure 3A), the 2-year mean forecast deviation of temperature has a good positive linear correlation with cloud cover; the correlation coefficient is 0.85 in 2019 and 0.95 in 2020. The forecast value is often less than the actual when the cloud cover is below 30%–40% and has a large linear slope. The forecast tends to be greater than the actual when the cloud cover is above 40% and has a smaller slope. For Tmin (Figure 3B), a negative correlation exists below the threshold of 40% cloud cover, with a steep linear slope; the correlation coefficients were −0.96 in 2019 and −0.89 in 2020. Conversely, a positive correlation with a smaller linear slope was found above the threshold of 40% cloud cover; the correlation coefficients were 0.95 in 2019 and 0.96 in 2020. The common point of Tmax and Tmin is that the absolute deviation will be larger when the cloud cover is low. Although the influence of cloud cover has been considered in the ECMWF temperature forecast, there is still a strong mean correlation between the mean forecast deviation and cloud cover. Meanwhile, schemes of grouping by cloud cover demonstrate an improvement in forecast accuracy. Therefore, the multiple regression scheme introduces a cloud cover factor based on cloud cover grouping that can be used in professional work. In the following, schemes will be designed and compared to select the best.
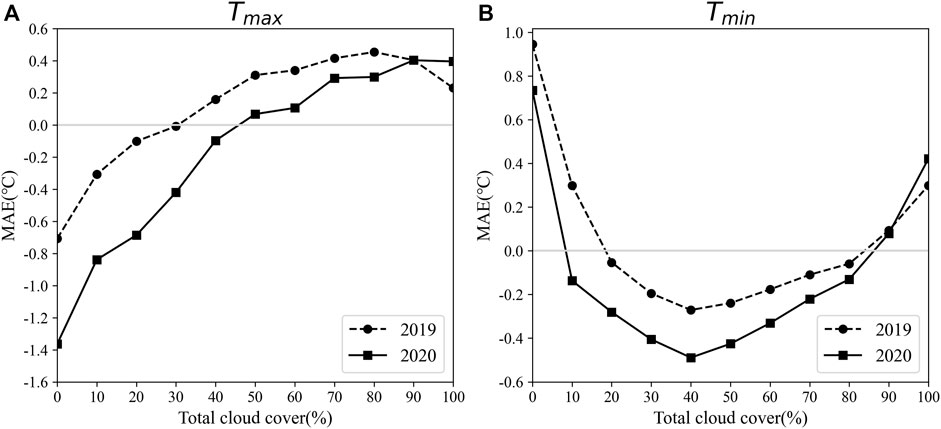
FIGURE 3. Relationship between the mean deviations of 12–36 h forecasts of temperature and the total cloud cover in 2019 and 2020. (A): Tmax and (B): Tmin.
4.3 Improving scheme design
Based on the aforementioned discussion, improvement plans were designed to maximize forecast performance by considering the roles of total cloud cover, grouping methods, and binary regression methods in the forecast.
Scheme 4: Binary regression, using the quasi-symmetric mixed sliding training period method (Wu et al., 2016), taking temperature and total cloud cover forecast as two forecast factors to establish the forecast equation.
Scheme 5: After grouping by total cloud cover, correction is performed using Scheme 4. From Figure 1A, it can be seen that the optimal threshold for the high-temperature grouping is at 30%–40% cloud cover, and Figure 3 shows that the 40% cloud cover is a special turning point in both Tmax and Tmin forecasts. In practical applications, less cloud cover is conducive to the increase of daytime Tmax and the decrease of nighttime Tmin. Therefore, the 40% cloud cover is used as the grouping threshold. After grouping, the cloud cover is introduced for binary regression to establish the forecast equation.
Using the aforementioned two plans, a verification experiment was conducted for Tmax and Tmin from 2019 to 2020, and the results were compared with Scheme 2 whose grouping threshold is set at 40% cloud cover (Figure 4). In terms of forecast verification results, for Tmax (Figures 4A, C), the FA in Scheme 5 is generally larger than that in Scheme 2. With increased forecast time, the improvement is more obvious, but it is slightly less than that of Scheme 4 on the third to fifth days, while Scheme 4 is slightly worse than Scheme 2 on the 1st day. In terms of MAE, Scheme 5 has a slight decrease, which is better than the other two schemes. For Tmin (Figures 4B, D), the overall improvement of Scheme 5 is more significant than that of Tmax, and all forecast indicators at all forecast periods are improved compared with other schemes. Scheme 3 is better than Scheme 4. In general, Scheme 5 is superior to other schemes. For Tmax, there is a linear relationship between the mean forecast deviation and cloud cover, with different slopes between low cloud cover and high cloud cover. Each of the three schemes has advantages, but the performance of the binary regression scheme after grouping performs more stably in terms of MAE. For Tmin, the mean forecast deviation has an opposite relationship between less and more cloud cover. Therefore, the binary regression scheme after grouping can better improve the forecast quality.
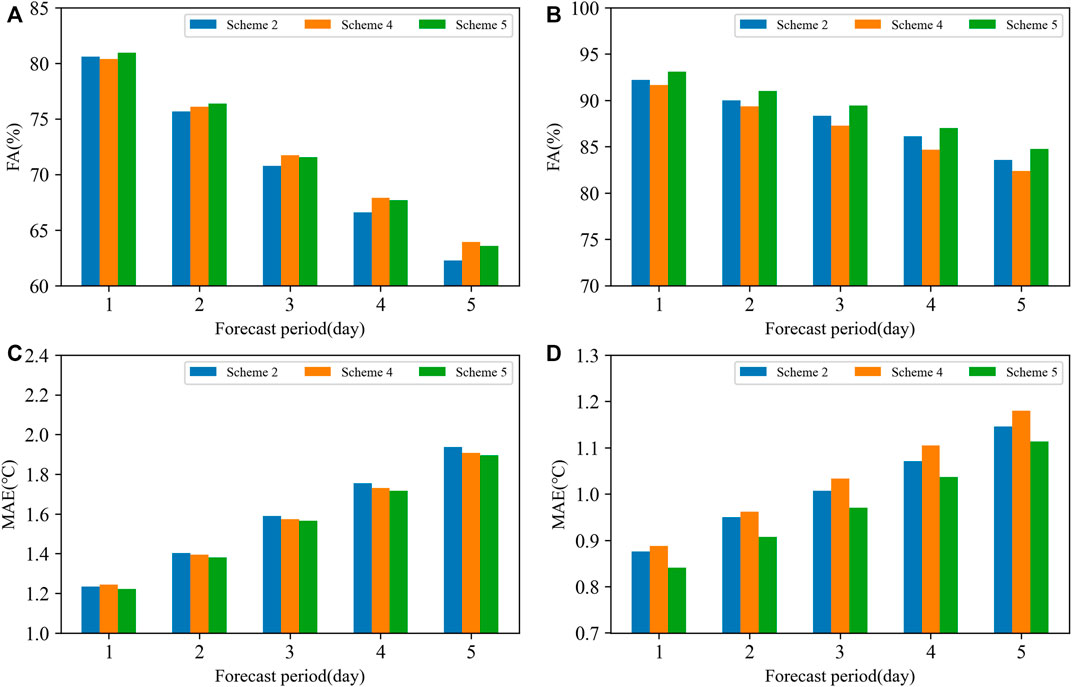
FIGURE 4. Comparison of the forecast results of the three schemes from 2019 to 2020. (A) FA of Tmax (unit: %), (B) FA of Tmin (unit: %), (C) MAE of Tmax (unit: °C), and (D) MAE of Tmin (unit: °C).
4.4 Availability and stability of improvement schemes
Scheme 5 was used for temperature forecast in 2021 to test the usability and stability of the method, and the verification results are shown in Table 1. According to the results of the Tmax forecast, all verification indicators in Scheme 5 show significant improvements compared to Scheme 1, with an overall increase in FA of 1.2–2.6%. The highest increase is 2.6% on the 1st day; after the improvement, the mean accuracy rate reached 81%. The accuracy continued to increase by about 2% in the fifth to sixth days. MAE decreased by 0.04–0.08°C on all forecast periods, except for slightly less on the 7th day. The first 3 days decreased by about 0.08°C, and the FSS can reach 0.05–0.06. In terms of the Tmin forecast, all indicators of all forecast periods also showed improvement, with an increase of 1.0%–2.4% in FA, and the highest increase was 2.36% on the first day. MAE decreased by approximately 0.05°C–0.08°C. The FSS in the first 3 days reached 0.06 to 0.08, and the FA on the first day reached 91.48%.
In recent years, the application of the quasi-symmetric sliding training period MOS forecast method has greatly improved Tmax and Tmin forecast results in Fujian Province. The method ranks among the top in national forecast quality inspections; especially, the FA of Tmin is basically more than 90%. Under the condition that the forecast accuracy of the original model has not been improved, there is some room for improvement of the forecast results, but the forecast accuracy of Scheme 5 increased by about 2% compared with Scheme 2 in Tmax and Tmin forecast in 2021. These findings show that this method can further improve the forecast quality, and it has a certain stability. At present, it has achieved good results in the operational application of actual temperature forecasts in Fujian. Although Scheme 5 showed some improvement in Tmax forecasts compared to Scheme 4, the difference was not significant, and the best plan should be selected based on local conditions and corresponding evaluations in practical applications.
5 Conclusion
This paper designs a MOS forecast method that uses total cloud cover as a predictor for the 2 m temperature forecast. Different schemes are designed and optimized using multiple verification indicators. The results show that:
1. All grouping schemes based on cloud cover show improvement in forecast performance, and the introduction of total cloud cover shows advantages over low cloud cover.
2. There is a good positive correlation between the annual mean forecast deviation of the Tmax and the mean total cloud cover. For Tmin, there is a negative correlation below 40% cloud cover and a positive correlation above it. Both Tmax and Tmin forecasts have larger absolute deviations when total cloud cover is less than 40%.
3. Whether for Tmax or Tmin forecast, the binary regression scheme after grouping consistently showed the best performance, with the lowest MAE.
4. Based on the optimization of the scheme in the last 2 years, the improved scheme is used to forecast the Tmax and Tmin in 2021. The verification indicators show certain improvements in most forecast periods, with FA for Tmax and Tmin being 81.312% and 91.480%, respectively, which is an improvement of 2.4%–2.6% relative to single-factor regression plans. The FSS of 0.065 for Tmax and 0.086 for Tmin indicate that this method effectively improves forecast quality and stability, making it suitable for practical forecasting. The introduction of total cloud cover to the MOS forecast can significantly improve the forecast quality, but the correction effect may be poor when the model’s cloud cover forecast has large biases from observations. Further research is needed to determine the reliability of cloud cover forecasts from multiple models and ensemble prediction products.
Data availability statement
The raw data supporting the conclusion of this article will be made available by the authors, without undue reservation.
Author contributions
HM: methodology and writing. WQ: data curation and software. LH: visualization. YS: editing and translation. WG: validation.
Funding
This work was supported by the Natural Science and Technology Program of Fujian Province (2021J01457), the National Key R&D Program (2018YFC1506905), and the Innovation Project of the China Meteorological Administration (CXFZ2021Z009). The Chinese Meteorological Administration’s retrospective summary project (FPZJ2023-061).
Conflict of interest
The authors declare that the research was conducted in the absence of any commercial or financial relationships that could be construed as a potential conflict of interest.
Publisher’s note
All claims expressed in this article are solely those of the authors and do not necessarily represent those of their affiliated organizations, or those of the publisher, the editors, and the reviewers. Any product that may be evaluated in this article, or claim that may be made by its manufacturer, is not guaranteed or endorsed by the publisher.
References
Che, Q., Zhao, S., and Fan, G. (2011). Seasonal partition problem of MOS forecast for extreme temperature in North China[J]. J. Of Appl. Meteorological Sci. 22 (4), 429–436. doi:10.11898/1001-7313.20110405
Chen, F., Jiao, M., and Chen, J. (2011). A new scheme calibration of ensemble forecast products based on bayesian processor of output and its study results for temperature prediction [J]. Meteorol. Mon. 37 (1), 14–20. doi:10.7519/j.issn.1000-0526.2011.1.002
Chen, Y., Chen, X., Ma, J., and Wen, L. P. (2005). Nano neodymium oxide induces massive vacuolization and autophagic cell death in non-small cell lung cancer NCI-H460 cells. J. Arid Meteorology 23 (4), 52–60. doi:10.1016/j.bbrc.2005.09.018
Huang, J., and Xie, Z. (1993). The application of kalman filter technique to weather forecast[J]. Meteorol. Mon. 21 (2), 3–7. doi:10.7519/j.issn.1000-0526.1994.9.009
Li, Q., Hu, B., and Wang, X. (2011). Analysis of hail disasters in the central region of gansu Province[J]. J. Arid Meteorology 29 (2), 231–235. doi:10.3969/j.issn.1006-7639.2011.02.017
Liang, L., and Huang, G. (2006). Research on forecast method of maximum and minimum temperature at single station[J]. J. Of Guangxi Meteorology 27 (3), 4–6.
Liu, H., Zhao, S., and Lu, Z. (2004). Objective element forecast at nmc—MOS system[J]. Xin Jiang Meteorol. 27 (3), 4–7.
Luo, J., Zhou, J., and Yan, Y. (2014). Local temperature MOS forecast method based on numerical forecast products and superior guidance[J]. Meteorological Sci. Technol. 42 (003), 443–450. doi:10.3969/j.issn.1671-6345.2014.03.015
Qian, L., Lan, X., and Yang, Y. (2010). The application of optimal subset neural network to temperature objective forecast in wuwei[J]. Meteorol. Mon. 36 (5), 102–107. doi:10.7519/j.issn.1000-0526.2010.5.015
Qin, W., Huang, D., and Liao, X. (2007). Analysis of the variation characteristics of cloudiness in nanning and relationship with temperature and precipitation[J]. J. Of Meteorlogical Res. Appl. 28 (4), 14–19.
Wang, X., Yang, X., and Shi, D. (2004). Application of numerical forecast products to summer high temperature prediction[J]. Meteorological Sci. Technol. 32 (S1), 47–49. doi:10.3969/j.issn.1671-6345.2004.z1.012
Wu, J., Pei, H., and Shi, Y. (2007). The forecast of surface air temperature using BP-MOS method based on the numerical forecast results [J]. Sci. Meteorol. Sin. 27 (4), 430–435. doi:10.3969/j.issn.1009-0827.2007.04.012
Wu, Q., Han, M., and Guo, H. (2016). The optimal training period scheme of MOS temperature forecast[J]. J. Appl. Meteorological Sci. 27 (4), 426–434. doi:10.11898/1001-7313.20160405
Zhang, W., and Sha, W. C. (2001). An interpolation and similarity method of the study on temperature prediction[J]. Sci. Meteorol. Sin. 21 (2), 241–245. doi:10.3969/j.issn.1009-0827.2001.02.017
Zhang, X., Cao, J., and Yang, S. (2011). Multi-model compositive MOS method application of fine temperature forecast[J]. J. Yunnan Univ. 33 (1), 67–70.
Zheng, X., Xu, G., and Rongqing, W. (2013). Introducting and influence testing of the new cloud fractiong scheme in the GRAPES[J]. Meteorol. Mon. 39 (1), 57–66. doi:10.7519/j.issn.1000-0526.2013.01.007
Zhi, X., Li, G., and Peng, T. (2014). On the probabilistic forecast of 2 meter temperature of a single station based on bayesian theory[J]. Trans. Atmos. Sci. 37 (6), 740–748. doi:10.13878/j.cnki.dqkxxb.20130613006
Zhi, X., Wu, Q., Bai, Y., and Qi, H. (2010). The multimodel superensemble prediction of the surface temperature using the IPCC AR4 scenario runs. J. Meteorological Sci. (5), 708–714.
Zhou, B., Zhao, C., and Zhao, S. (2006). Multi-model ensemble forecast technology with analysis and verification of the results[J]. J. Appl. Meteorological Sci. 17, 104–109. doi:10.3969/j.issn.1001-7313.2006.z1.015
Zhu, G., and Mu, H. (2013). Application of model outout Statistics into objective element forecast at Airport[J]. Desert Oasis Meteorology 7 (3), 13–16. doi:10.3969/j.issn.1002-0799.2013.03.004
Keywords: the maximum temperature, the minimum temperature, cloud cover, MOS method, forecast accuracy, mean absolute deviation
Citation: Mei H, Qishu W, Huijun L, Siyu Y and Guofei W (2023) Correction method by introducing cloud cover forecast factor in model temperature forecast. Front. Earth Sci. 11:1099344. doi: 10.3389/feart.2023.1099344
Received: 15 November 2022; Accepted: 31 March 2023;
Published: 24 April 2023.
Edited by:
Jianjun Xu, Guangdong Ocean University, ChinaReviewed by:
Husi Letu, Aerospace Information Research Institute (CAS), ChinaChao Wang, Nanjing University of Information Science and Technology, China
Copyright © 2023 Mei, Qishu, Huijun, Siyu and Guofei. This is an open-access article distributed under the terms of the Creative Commons Attribution License (CC BY). The use, distribution or reproduction in other forums is permitted, provided the original author(s) and the copyright owner(s) are credited and that the original publication in this journal is cited, in accordance with accepted academic practice. No use, distribution or reproduction is permitted which does not comply with these terms.
*Correspondence: Wu Qishu, 172475076@qq.com