- 1Key Laboratory of Regional Sustainable Development Modeling, Institute of Geographic Sciences and Natural Resources Research, Chinese Academy of Sciences, Beijing, China
- 2Research Center of Regional Development and Planning, Key Laboratory of Geospatial Technology for the Middle and Lower Yellow River Region, College of Geography and Environmental Science, Henan University, Kaifeng, China
The intensified competition for innovation among countries and the various risks that come with it have made innovation resilience a central concern of the international community in recent years. The concepts of resilience and innovation are inextricably intertwined; however, both theoretical discussions and case studies on innovation resilience are in the “embryonic” stage. This article attempts to integrate the system and nodes of network resilience, the potential and connectivity of city resilience, and the hierarchy and assortativity of urban network resilience to construct a theoretical analysis framework of intercity innovation network resilience and conduct a case study on the resilience of a patent cooperation network among 338 prefecture-level cities in China during 2017–2019. The results show that the systemic resilience of Chinese urban innovation networks exhibits relatively low hierarchical and yearly decreasing disassortative characteristics, and the node resilience shows a spatial pattern of being high in the southeast and low in the northwest, as well as higher innovation resilience in cities with higher administrative levels. The results provide insights into the overall systemic and internal structural characteristics of innovation resilience in Chinese urban networks and also expand the application of resilience concepts in the field of innovation research from the perspective of urban networks.
1 Introduction
Resilience and innovation are both regarded as central aspects of UN’s sustainable development goals (Berkes et al., 2003; Lv et al., 2018; Van Wellen, 2019). In the process of scientific and technological innovation and interaction, uncertainty and unknown risks such as technology blockade, talent monopoly, and the impact of major public health emergencies are rising constantly (Lee, 2018); many countries even find themselves squeezed out of their former technology marketplaces in recent years (Cooke et al., 2012). The US promulgated the Innovation and Competition Act of 2021 (Sun and Luo, 2021), which has triggered a new round of discussions on innovation competition among countries, and brought more risks and challenges to China’s innovation and development. Innovation collaboration is increasingly affected and restricted by national borders. Therefore, improving national innovation resilience has become an important issue. Resilience is connected with vulnerability which implies risk, meaning the capacity to tackle “shocks” and “stresses.” Shocks are inevitable and come in many forms whose impact depends on activity types and their traits in terms of uncertainty, riskiness, and resistance. The concept “resilience” originated from engineering mechanics, and was later applied to the field of ecology (Holling, 1973). Nowadays, it has been widely applied in many fields of economy and society (Liu et al., 2007) where policy discussions of regional development have recently shifted from a focus on growth and competitiveness to the analysis of the relative resilience of regional economies in response to rapid transitions in technologies, markets, and exogenous economic shocks (Clark et al., 2010). Resilience is a highly multidisciplinary concept, and there are different meanings and approaches within different disciplines (Vogus and Sutcliffe, 2003; Ponis and Koronis, 2012; Hosseini et al., 2016; Linnenluecke, 2017; Sanchez et al., 2017). It is possible to identify a sequence of resilience concepts: from systemic equilibrium toward a more open, non-static, ongoing, co-evolutionary, non-linear, complex, and socio-ecological-technological systems (Fastenrath et al., 2019). There have been multiple positive conceptualizations of resilience (Pike et al., 2010), along with some skepticism, questioning the value of the concept, especially by the followers of evolutionary economic geography (Hassink, 2010; Pike et al., 2010). This “evolutionary” perspective of resilience (Davoudi et al., 2013) is a criticism of the static understanding of resilience, which is an important turning point in conceptualizing and understanding resilience, inspiring researchers to explore more aspects of resilience.
Resilience is the basis for the continuity of innovative activities and for entering a new development path which is crucial in determining aptitude for sustained innovative output (Balland et al., 2015). Therefore, sustained evolutionary performance for regional innovation systems necessitates the capacity for those regions to reconfigure themselves effectively and rapidly to adapt to new or altered surroundings generated by these shocks (Lee, 2018). Innovation plays a key role in the renewal and reorientation of development (Athey et al., 2008), which is the driving force of long-term economic transformation and change (Schumpeter, 1939, 1942). However, innovation involves a high level of uncertainty, idiosyncrasy, risk, and sensitivity to turbulence; and the innovative outcomes are uncertain (Freeman and Soete, 1997; Fleming, 2007). By its nature, resilience of innovation considerably differs from other types of resilience due to the specifics, and the essence of innovation is knowledge (Pavit, 2006; Popadiuk and Choo, 2006; Salter and Alexy, 2014; Dost et al., 2019), which is a unique, cumulative, and peculiar economic good, whose resources, features, continuing cumulatively immuring to further turbulences is the key element of the resilience of innovation (Antonelli, 2019). Meanwhile, learning of emerging types of knowledge and the absorption of external knowledge is also based on the constant combination and complementarity with existing types of knowledge (Wang, 2007), creating unlimited opportunities and potentials for innovation. As for a country or region, the agglomeration and diffusion of knowledge will accelerate the cumulative effect of knowledge and thus enhance the overall innovation capacity; this ability of agglomeration and diffusion will affect the continuity of regional innovation and the ability to adapt to environmental changes, which involves the issue of innovation resilience.
The role of innovation and knowledge has also been highlighted in relation to the concept of regional resilience (Lawson, 1999; Amin and Cohendet, 2004; Simmie and Martin, 2010). Following the concept of resilience, innovation resilience was viewed as the capability to cope with uncertainties associated with innovation through effectively integrating stability and adaptability (Lv et al., 2018; Pinto et al., 2018). However, these concepts are too general and do not demonstrate the essential characteristics of knowledge and innovation. Innovation resilience is generated in the process of knowledge aggregation and diffusion, so innovation resilience should highlight the ability of innovation entities to create, reorganize, reconstruct, and apply various types of knowledge within the territory they belong to, as well as the ability to generate new synergies and complementarities in shocks, and thus show stronger adaptation (variations within predetermined paths) and adaptability (departing or altering from the present path) under external shocks.
Resilience theory (Gunderson and Holling, 2002) and the diffusion of innovations theory (Rogers, 2003) are two interdisciplinary routes of inquiry that explore the interaction between human decision-making and the process of change (R.C. Atwell et al., 2008; Euchner, 2019). Resilience theory is in its theoretical adolescence and is favored by scientists and practitioners in different fields (Liu et al., 2007); but, it is also criticized for its weak integration and application of social science theories and complex network approaches (Janssen et al., 2006). In contrast, innovation diffusion theory has evolved more maturely over half a century of research. However, over the past 2 decades, the field of innovation diffusion research has also changed to focus on the sort of multilevel and ecological system drivers, which are the core topics of concern for resilience theory (Rogers, 2003).
Innovation resilience has received international attention, but it is particularly important to explore the issue of innovation resilience at the national scale due to the growing competition for innovation between countries, which has made innovation diffusion increasingly influenced by national governments or national borders. In addition, as agglomerations of innovative resources and elements, cities are the basic nodes of national innovation development (Ma et al., 2015). The network pattern of knowledge flows within and between cities, becoming a reflection of national innovation capacity and profoundly affects the national innovation resilience (Clark et al., 2010). We explore the innovation resilience of national-scale urban networks from the perspective of knowledge flow and technological innovation cooperation, expecting to establish a method to evaluate national innovation resilience and aim to find a way to improve the national innovation resilience from the perspective of urban networks of co-invention.
The remainder of this article is arranged as follows. Section 2 discusses how innovation and knowledge networks are influential in the framework of the abounding literature on regional resilience. Section 3 introduces the research methodology and data, which is followed by five structural properties to measure the innovation network resilience. The research results related to the systemic structural and nodal characteristics of urban innovation network resilience using Chinese cities as an example are demonstrated in Section 4. The article concludes in Section 5 by making summaries and giving new insights for future studies.
2 Literature review and theoretical foundations
2.1 The concept of resilience in regional innovation
Research studies on resilience are nowadays indicate a growing interest as the macroeconomic context is characterized by chronic instability, as financial crisis, rapid technological cycles, environmental considerations, and new growing consumption paradigms challenge global but also regional policies (Crespo et al., 2013). In an increasingly interconnected world, challenges in one part may have a strong impact on other parts. The uncertainty associated with complex urban systems and networks calls for more attention to resilience. The development of the resilience theory has evolved through three stages: engineering resilience, ecological resilience, and evolutionary resilience (Modica and Reggiani, 2015). The first definition of resilience in the social and ecology domain dates back to 1973 (Holling, 1973) for understanding how some performing regions can decline at a given moment in time, while others are able to renew and sustain their growth in a disturbed economic environment.
There are two main perspectives of the meaning of resilience: as a double capacity, that of resisting a shock or limiting its effects but also that of anticipating and thus adapting to the shock or to a rapid evolution in the economic context by creating new systems (Gilly et al., 2014). The first one as a static property, namely, as engineering resilience, refers to stability and resistance capacity to pre-shock structure and function or stable equilibrium when subject to change (McDonald, 2006; Burnard and Bhamra, 2011; Lengnick-Hall et al., 2011). The second perspective has a dynamic character. It corresponds to the recovery ability of an equilibrium state to restore damaged components and redistribute flows, as well as create a new opportunity to change structure and function in response to a shock, which means forging a new pathway rather than continuing with an established path dependence (Reggiani et al., 2002; Allenby and Fink, 2005; Folke, 2006; Brede and de Vries, 2009; Schweitzer et al., 2009).
From the duality view, the concept of innovation resilience can be divided into two primary dimensions: stability and adaptability, which are integral parts of resilience and provide implications for constructing and measuring innovation resilience (Jackson, 1999; Eisenhardt et al., 2010; Farjoun, 2010; Sonenshein, 2016). Stability means reducing the vulnerabilities and absorbing shocks (Lengnick-Hall and Beck, 2005), which involves standardization, modularization, institutionalization, systematic planning, and focuses on the efficiency of innovation in a low-changing and predictable context. Adaptability is targeted at flexibility of taking advantage of opportunities and creating a fundamentally new system in high-variation and unpredictable environments. Nevertheless, behind this large consensus on regional resilience and its links with innovation and knowledge networks (Lawson, 1999; Amin and Cohendet, 2004; Simmie and Martin, 2010), we have found that few research studies have pursued the question of the elements of resilience in the innovation and the long-run evolution of regional innovative structures (Crespo et al., 2013). Overlooking the role that resilience plays in the long-term prosperity of a regional innovation system restricts a holistic understanding of the factors comprising such a dynamic structure.
Mainly dedicated to innovative cities and technological collaborations, this research is part of a more general wave of research on regional resilience. We emphasize the dynamic nature of resilience in an innovation network and consider it an ongoing process to create and use the accumulation of knowledge. Following the concept of regional resilience, we view innovation resilience as the capability to cope with the uncertainties associated with innovation, perform innovation continuously in turbulent environments, increase knowledge accumulation persistently, and create reinforcement mechanisms in innovation that translate into new capabilities. The differing spatial dynamics of innovation activities suggests that networks can be of either a local or regional nature (Clark et al., 2010), of which regional innovation resilience studies should rely on the structural and topological properties as key factors (Andergassen et al., 2014).
Using the structural properties of networks to evaluate regional resilience has been adopted by many scholars (Crespo et al., 2013; Suire and Vicente, 2014; Kurth et al., 2020), but research studies on the knowledge network were mainly conducted from a micro perspective based on a local firm or industrial level, and it has been challenging to investigate the spatial characteristics of regional resilience. Although the emergence of network properties is commonly founded on micro-economic behaviors, these innovation entities and their behaviors are constrained by their local space. The aggregation of knowledge flows between actors or organizations in the micro scale into spatial flows between cities in the macro scale, constituting an appropriate measure to reflect spatial knowledge diffusion and spillover (Ma et al., 2018). In other words, the resilience of an intercity network structure can essentially be seen as the spatial performance of regional resilience (Newman, 2003; Crespo et al., 2013; Boschma, 2015). In this study, an intercity co-invention network was built from a macro perspective for better analyzing spatial characteristics of regional innovation resilience. When we take a city as the study area, these networks include both internal parts established within the city and external connections existing outside the city.
2.2 Innovation network resilience on the system level
In most of the definitions found in the literature, resilience is identified as an inherent attribute of a system (Holling, 1973; Hollnagel et al., 2006; Erol et al., 2010), the resilience of which relates to the functioning and interaction rather than to the stability of their components or the ability to maintain or return to some equilibrium state. Regions that are capable of taking advantage of new opportunities logically achieve higher resilience to potential shocks (Dabson et al., 2012). These extra-regional competencies serve to diversify a region’s risk or decrease its vulnerability to internal or external perturbations (Asheim and Isaksen, 2002; Bathelt et al., 2004; Moodysson, 2008; Fitjar and Rodriguez-Pose, 2011). In the knowledge-based view, resources are the result of the creation and development of knowledge, whose accumulation plays a critical role in innovation resilience. Along the same line, Lundvall (Lundvall, 1992) formulated an understanding of regional innovation systems as the actors and exchanges that jointly participate in the development, diffusion, and subsequent use of new and useful knowledge. It is through these interactions underpinning the regional innovation system that cities access knowledge that cannot be generated internally, making regions inclined to exhibit less rigidity and more flexibility to outside influences.
As cities typically no longer innovate in isolation but through a complex set of intra or intercity interactions, networks have been recognized as a crucial ingredient in regional innovation resilience (Watts and Strogatz., 1998; Nooteboom and Gilsing, 2004; Christopherson et al., 2010; Pike et al., 2010). Structural properties of a network can provide insights about how the network is structured and the most important nodes and connections (Reggiani, 2013). The literature acknowledges two categories that shape social network structures: closure and bridging network strategies. Triadic closure implies that a node associates with two other nodes will increase the probability for these two nodes to have a tie between them. The higher the degree of a node, the more the node is attractive for receiving new ties, so that the network grows through an increasing core-component hierarchy (Albert and Barabasi, 2001). Since the low connectivity between the core nodes and the peripheral ones limits the reorganization of knowledge flows, closure favors network assortativity. Bridging ties is more entrepreneurial than the former, meaning that one node finds an opportunity to connect disconnected nodes, which has implications on the overall structure since they can enable better circulation of knowledge between the core and the periphery of nodes (Cattani and Ferriani, 2008).
The level of hierarchy of the node degree and the level of assortativity therefore appear as two simple statistical signatures of the ability of the regional innovation system to perform through their endogenous resilient capabilities. The hierarchy exemplifies the level of heterogeneity in terms of actual relational capacities and positions, which reveals a pattern that the network dynamics is being driven by a process of self-organization determining a hierarchical connectivity structure (Carrington et al., 2005; Reggiani and Nijkamp, 2006). A network with a rather flat hierarchy displays high resistance to external shock regardless of whatever node is removed, but the absence of a core group weakens the control of the collective behaviors (Crespo et al., 2013). On the other hand, a network with a rather sloped hierarchy remains sufficiently linked to fresh and new ideas coming from peripheral nodes for future collaborations. But when closure strategies of core groups exceed a certain threshold, then the redundancy of knowledge flows and conformity effects prevail and the possibilities for regional resilience fall unavoidably. That is to say, the hierarchy in the appropriate interval is helpful to improve the resilience of the regional innovation system.
Based on the extent of whether the node degree displays a positive or negative correlation, networks can be characterized as assortative or disassortative (Crespo et al., 2013). A network structure is assortative when high-degree nodes are connected disproportionately to other high-degree nodes, and low-degree nodes are preferentially connected to low-degree nodes, which gives a formal representation of the way knowledge flows between central and more peripheral nodes. The emergence of such structures is mainly due to the preferential attachment process (Newman, 2003; Dorogovtsev et al., 2008). Generally, highly connected nodes are tied predominantly with other highly connected nodes in the core, and peripheral nodes remain connected between themselves. Networks have to develop bridging strategies in order to open more disruptive relations between the core and peripheral nodes, so as to enable the system as a whole to facilitate a collective process of knowledge integration between complementary cities along the knowledge value chain than for the entirely assortative one.
2.3 Innovation network resilience on the node level
As cities are well-connected by knowledge networks, even a moderate shock in a city may cause unpredictable changes in the entire innovation network system. Cities’ resilience is based on both absorption of external knowledge through networking and creation of new knowledge. More diversified regions, especially those able to rely more heavily on outside influences are structured in a more resilient manner (Simmie and Martin, 2010; Balland et al., 2015). The generation of new knowledge builds upon previously learned information which extend knowledge stocks, reduces resource constraints, and increases the probability of subsequent innovation (Duguet and Monjon, 2002; Clausen et al., 2012). In this way, the transformation of accumulated knowledge conceivably enables the region to better prepare for unforeseen shocks and better recover from shocks by rapidly adapting to new conditions. This involves assessing the quality and strength of two key features of the innovation system at the node level: innovative potential and connectivity which together represent their degrees of resilience (Sunley, 2013). These two in combination allow for the capacity of a node/city to face destabilizing shocks, manage processes of creative destruction through innovation, and recovery.
First is its potential, measured in terms of the variety of innovation resources and achievements present from the interaction among diverse local entities. A region that is technologically capable must include local entities featuring an autonomous innovative capacity owing to learning, searching, and gathering of information ultimately leading to innovation. Innovative activities initially take place in the active interaction between innovative subjects in the city, as geographic proximity reduces uncertainty and resolves coordination problems in balancing risks and costs of innovation (Boschma, 2005; Ma et al., 2021). Regional innovation system theorists such as Cooke (Cooke, 2001) pointed out that the richer the local innovation resources and the closer the internal connections, the healthier the network innovation system and the stronger the competitiveness (Ma and Xu, 2022). The unintended spillover effects generated by the linkage of innovation agents within cities cannot be ignored on the overall regional resilience.
The second is connectedness, the degree to which it has interlocking systems assisting its governance. For physical networks, efficiency and resilience are incompatible, that is, the more efficient the network is in minimizing ties and maximizing reachability, the more sensitive it is to external shocks and the more it exhibits fragility properties (Brede and de Vries, 2009). But for innovation network as one type of socio-economic networks, because of the ability of nodes to build and maintain ties for overlapping explorative and exploitative ties, performing networks are not necessarily incompatible with regional resilience. Erhardt et al. (2007) studied the relationship between connectivity and social network resilience and showed that sharp transitions, hysteresis, and equilibrium multiplicity of connectivity are salient characteristics of social dynamics. More diversified regions, especially those able to rely more heavily on outside influences are structured in a more resilient manner (Simmie and Martin, 2010; Balland et al., 2015). A node with high transitivity means that it can quickly exchange knowledge and technology with others in the network, promote learning and innovation in the whole system, and enhance the resilience to withstand shocks (Dabson et al., 2012; Rose and Krausmann, 2013).
Equally important to the high transitivity of a node in a regional innovation system as the interaction of innovation-focused actors is the assurance that the external links of the nodes are of made up of many diverse paths. In an evolutionary framework, adaptability and adaptation are two critical concepts (Hassink, 2010). Adaptability means to develop a new path while regions with adaptation typically might be locked into historical path dependence. Resilient regions usually are able to overcome a trade-off between adaptation and adaptability (Pike et al., 2010). In other words, a resilient network should be redundant (Ouyang et al., 2012). It has been proven that cities with a knowledge base that has a high degree of relatedness to technologies that are not yet present in the city are better able to avoid technological crises. Therefore, diversified innovation systems, presenting openness to a variety of extra local links would be able to redirect their development paths to adapt to changing conditions, and would be less susceptible to lock-in effects (Dabson et al., 2012; Balland et al., 2015). Regardless, if the node is removed, the knowledge flow will still find paths to irrigate the whole network. Such a network displays a strong potential for knowledge flow reorganization and diffusion since the nodes are linked by many paths.
3 Materials and methods
3.1 Study area and data source
This study takes 338 cities in China as examples, including 289 prefecture-level cities, 26 autonomous prefectures, 4 municipalities, 6 prefectures, 3 leagues, and 10 counties administrated by the province (excluding Taiwan, Macao, and Hong Kong). Patent co-invention is a manifestation of knowledge spillover, which is an important achievement of scientific and technological achievement, and a major indicator of regional innovation capabilities. Using the information of China’s patent co-invention number as the original data from 2017 to 2019, we analyzed the resilience of China’s urban innovation network based on the structural and topological properties. Vector files of administrative boundaries and urban administrative centers at a scale of 1:1,000,000 were provided by the basic geographical database of the National Geomatics Center of China.
3.2 Conceptual framework
Network is a simple but useful method to analyze resilience from an evolutionary perspective (Boschma, 2015). Drawing on the conceptual framework of the transportation infrastructure proposed by Wang et al. (2020) and the previous theoretical analysis of innovation resilience and network structure; the analytical framework of urban innovation network resilience is shown in Figure 1.
The first step is defining and constructing urban innovation network resilience. As a complex system, an urban innovation network consists of nodes and links, where nodes represent different cities and links manifest the relational innovation connections and interactions among cities. Next, urban innovation network resilience is identified from the system level and node level. Systemic resilience represents the comprehensive ability of the urban innovation network against technological ricks, which can be quantitatively measured by two simple statistical signatures: hierarchy and assortativity. Resilience of innovation on the node level represents the capacity of a city to face destabilizing shocks, manage processes of creative destruction through innovation, and recover, which can be measured by potential and connectivity. The following is a detailed description of measurement methods and the final is calculations and a result analysis of the urban innovation network resilience.
3.3 Measuring methods
3.3.1 System resilience: hierarchy and assortativity
3.3.1.1 Hierarchy
Hierarchy represents the ranking of nodes in a network (Ye and Qian, 2021). Crespo (Crespo et al., 2013) proposed that the measure of hierarchy can be reflected by the network degree distribution exponent, and the greater the slope of the degree distribution, the more significant the degree of hierarchy between nodes. The degree distribution is the probability distribution of degrees, where K* is the probability that a node chosen randomly from the network has degree K. We calculated the probability of each degree and then draw the distribution on a log–log scale, such that:
with Ki* being the probability of random city i with degree K in the degree distribution, C being a constant, and a <0 the slope of the distribution.
3.3.1.2 Assortativity
The measure of assortativity can be reflected by the network degree correlation index (Crespo et al., 2013; Wei and Pan, 2021; Ye and Qian, 2021). The mean degree of the relevant neighborhood (Vj) for each node i can be calculated from following equations:
where Kj is the degree of node j belonging to the interaction neighborhood of node i.
Then, we estimate a linear relationship between ‾Ki and Ki:
with D as a constant and b as a coefficient capturing the degree correlation. If b>0, the network N exhibits assortativity with a positive-degree correlation, whereas if b<0, the network N is disassortative with a negative-degree correlation.
The “core/periphery and resilient network” exhibits another high-sloped degree distribution, but the degree correlation is now negative (b<0), so that the network presents a certain level of disassortativity. The larger |a| of the degree distribution coefficient, the higher its structural hierarchy, enabling it to be cohesive and competitive; the larger the |b| of the degree correlation coefficient, the more the disassortative patterns of relations increase regional resilience capabilities (Crespo et al., 2013). It can be used as the basis for judging the system resilience of the urban innovation network.
3.3.2 Node resilience: potential and connectivity
Network node resilience reflects the ability of a node to maintain its original innovation and connection strength when it is attacked (Wang et al., 2020), that is potential and connectivity.
3.3.2.1 Potential
Potential is measured by self-organization, which can be represented by the number of intracity patent co-inventions. In order to avoid the effect of numerical magnitude differences on the results, the data are normalized as:
xi is the number of intracity patent co-inventions in city i, min (xi) is the minimum number of intracity patent co-inventions, and max (xi) is the maximum number of intracity patent co-inventions.
3.3.2.2 Connectivity
We use two indicators—transitivity and diversity to measure the connectedness of nodes. Betweenness is used to quantitatively evaluate the transitivity of cities in the network, which is defined as the ratio of the number of shortest paths between any two cities in the network that pass through the city to the total number of shortest paths, representing the city’s mediation effect and connection function in the innovation network. The betweenness of cities are calculated by the following equations:
where Tmn is the number of shortest paths from city m to city n, and Pmni is the number of shortest paths from city m to city n passing through city i.
The diversity of the urban innovation network between two cities depends on whether there are other by-pass paths apart from the normal path. We draw on the concept of independent path proposed by Ip and Wang (2011) to measure diversity. If a path set includes the path-connected nodes i and j without any common edges with other paths between nodes i and j, the set is defined as an independent passageway set of nodes i and j. The element of the set is called as a path (Ip and Wang, 2011). Diversity is quantified by the average number of independent paths, which is defined as the ratio of the number of independent paths between any two cities in the network to the total number of possible paths between cities. Here, an interruption scenario is set up, assuming that the city is attacked, the change of the average number of independent paths in the network reflects the city’s diversity. The overall network diversity can be calculated as:
V(G) is the average number of independent paths. Nij represents the number of independent paths of cities i and j, which can be approximately replaced by the smaller of the two city degrees. Since the Nij +1 paths have no common edges, they certainly have Nij +1 edges to depart from city i or j. Nij +1 > Nij =Di or Dj, which conflicts with the definition of node degree. Nij is the upper bound of the passageway number of an independent passageway set between the city pair i and j (Ip and Wang, 2011).
The diversity for each city is calculated as:
where V(G)' represents the network diversity after removing the connection between city i and its neighboring cities.
4 Results and discussion
Using the network theory and adopting a network structural analysis, our study attempts to assess the resilience of the urban innovation network based on endogenous mechanisms. As a network can be represented by two basic elements including the nodes and the ties that connect the nodes (Crespo et al., 2013), the correlation between relational behavior and individual node features has to be captured in parallel. Next, we introduce system resilience and node resilience separately from different dimensions of network structural properties.
4.1 System resilience
System resilience is measured by the “Hierarchy” and the “Assortativity,” that by collaborating across different hierarchies and with complementary cities, scientists, and researchers can combine their techniques and complementary skills through perceptions and opinions in pursuit of corporate goals and providing better fertile ground for long-term knowledge creation. The hierarchy characteristic is quantitatively calculated using the index of degree distribution and degree correlation mirrors the assortativity characteristic of innovation network resilience.
4.1.1 Hierarchy
There are 10,178 edges in China’s urban innovation network, with an average degree of 30.11. Figure 2 shows the spatial distribution of 338 cities whose node degrees are divided into five levels. The number of the cities with the node degree at the first level is only 34 and the top nine cities are, respectively, Beijing, Shanghai, Shenzhen, Nanjing, Wuhan, Chengdu, Guangzhou, Suzhou, and Hangzhou. Among them, there are seven cities in the eastern region, one city in the central region, and one city in the western region, which shows the huge spatial differentiation at the national scale and reflects the simultaneous characteristics of innovation as unique and non-reproducibility. The cities at the second and third levels include sub-provincial cities, province capital cities, and cities within the innovation radiation sphere of the cities at the first level, such as Nantong and Luoyang, engaging with innovative cities actively by taking advantage of geographic proximity. Cities at the fourth and fifth levels are mostly distributed at the edge of the innovation network, with the maximum degree value being 14, only 1/23 of Beijing, indicates that the spatial differences of the degree distribution of innovation networks in Chinese cities are significant.
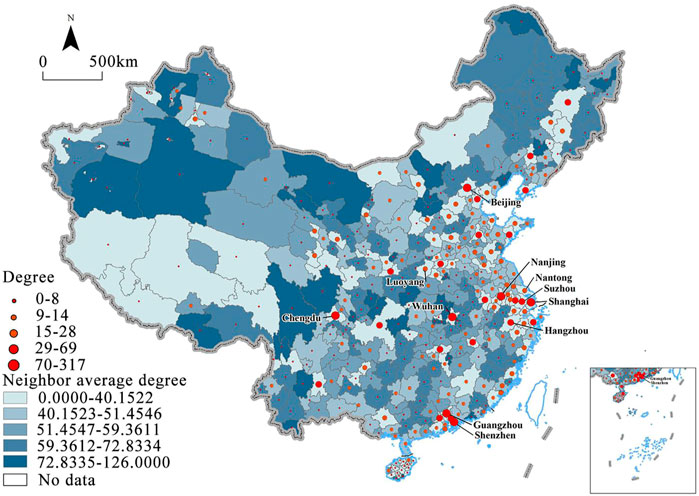
FIGURE 2. Spatial distribution of the degree and neighbor average degree of China’s urban innovation network (average value from 2017 to 2019).
We calculated the probability of each degree in the innovation network and drew the degree distribution on a log–log scale to analyze the overall hierarchy by using Matlab software (Figure 3). The fitted curve appears as a straight line with a negative slope of a power exponent on a log–log scale and the R2 of the degree distribution regression is 0.7237, indicating that the power–law distribution is suitable for fitting. We would expect that China’s urban innovation network presents a power–law degree distribution and is a scale-free network (Barabási and Albert, 1999; Barabasi et al., 2000), indicating that it has certain cohesion and competitiveness, providing resistance and robustness to resist scientific and technological risks and shocks. In theory, the power exponent of a scale-free network is usually between 2 and 3 (Barabási and Albert, 1999; Barabasi et al., 2000). The slope |a| of the curve is 0.8258, which is relatively low compared with the hierarchical coefficient of the resilience of information networks and economic networks studied by Wei and Pan (2021), respectively, 1.229 and 1.727, and the result of the resilient network studied by Crespo et al. (2013) which was 1.063. The low coefficient indicates that the innovation network may exhibit an insufficient level of cohesiveness into the core in some fields. In that case, leading cities may not succeed in maintaining a high level of knowledge integration. Thus, few but important fields should be focused on improving the cohesive force of the core to strengthen network resilience further.
4.1.2 Assortativity
The assortativity of the network reflects the preference attachment of cities. Using NAD, the degree correlation is measured based on average data from 2017 to 2019 and the coefficients are negative with a value of −0.2694 (Figure 4), indicating that the Chinese urban innovation network is disassortative. In Figure 2, we can find that cities in the innovation network with high-degree/low-degree values are usually neighbored by cities with low/high average values, which confirms the existence of disassortative innovation links in the urban innovation network. As if cities with high-degree values build innovative connections and cooperation with cities at the lower level, knowledge and technology can circulate through many structural bridges between the core and the periphery, making the structural resilience of the network become stronger.
Figure 5 displays the degree correlation, respectively, in 2017, 2018, and 2019. Specifically, the absolute values of the coefficient in 3 years are 0.3176, 0.2619, and 0.2454. The decreasing trend indicates that the network disassortativity is weakening year by year, as the assortative relationships are growing stronger and quicker than the disassortative relationships. The enhancement of assortative linkages may lead to potential crises such as path dependence and regional lock-in, reducing the probability of innovative activities and the infiltration of external information.
4.2 Node resilience
Node resilience consists of two parts: potential and connectivity. Potential is measured by the self-organization, which relates to the ability to learn, create, restructure, recombine, adopt various types of knowledge, and develop generalized responses to foreseen or unforeseen events (Walker et al., 2002); connectivity is measured by transitivity and diversity. The transitivity of the nodes concerns the closeness with which contributing actors in a region collaborate in ways that lead directly or indirectly to innovation-spawning activities; and a diverse regional innovation system structure often provides greater resistance to shocks since different cities have different sensitivities to external downturns.
4.2.1 Potential: Self-organization
Figure 6 displays the spatial patterns of a self-organization level in the urban innovation network. Specifically, cities with self-organization at the first level are mainly national central cities and national science and technology innovation cities, such as Beijing, Tianjin, Shanghai, Guangzhou, Nanjing, Hangzhou, Qingdao, and Suzhou, surrounded by cities at the lower level which are able to receive technological radiation. Cities at the fourth and fifth levels are mostly located in the northeast, northwest, and southwest areas of China. Due to single path dependence and weak innovation capabilities, they have a low status in the self-organization hierarchy and lack development momentum. This result is roughly consistent with the spatial distribution of the urban degree (Figure 2), indicating that cities with higher internal innovation potential also have higher external connection strength.
4.2.2 Connectivity: Transitivity and diversity
The spatial pattern of transitivity is that the southeast is strong and the northwest is weak (Figure 7). There is a strip-shaped spatial distribution pattern of cities with high transitivity along the eastern coast. Cities with high transitivity in the central area are distributed roughly consistently with the main railway trunk lines, for example, Zhengzhou, Wuhan, and Changsha connect the middle of the Beijing–Guangzhou Railway, showing that the transportation infrastructure brings convenience to the flow of innovation elements, and is beneficial to the diffusion and dissemination of knowledge and technology between cities. The high transitivity areas in the west are mainly the core and sub-core cities within the urban agglomerations, such as Lanzhou and Xining in the Lan–Xi urban agglomeration. Core cities such as Beijing, Tianjin, Shanghai, and Shenzhen have a good mediation effect and connection function in the network, acting as bridges to build connections with other cities through knowledge spillovers, and promote the rapid flow of technology. The speed and breadth of the transitivity of innovative elements should be strengthened in cities in the western areas to better buffer technological risks and impacts in the future.
When different cities are removed, the average number of independent paths in the network decreases to different degrees, which reflects the diversity of cities (Figure 8). Specifically, the eastern coastal cities, provincial capital cities in central and western areas have strong diversity and appropriate redundant paths, which has stronger resilience in the face of technological risks. The non-core cities have little impact on the resilience of the overall innovation network and the decrease of the network resilience can be ignored when they are attacked, such as Shiyan, Ankang, and Baiyin. However, considering the improvement of the overall network resilience, it is of great significance to increase the abundance of connections in non-core cities, which could limit serious downturns in patent productivity and ensure a speedy recovery from crises through a complex overlapping process between a mature market domain and a new emerging related one.
5 Conclusion
The concept of resilience originating from engineering mechanics is originally clear and unambiguous, but the expansion of the applications of resilience, especially in the socio-economic field, has increased the complexity of understanding resilience; of course, this complexity is also closely related to the complexity of the object of study, that is, the socio-economic system. The urban innovation network studied in this article is inherently characterized by complexity, making it difficult to provide a comprehensive and quantitative understanding of its resilience.
The main contribution of this article is to establish an analytical framework for exploring the innovative resilience of urban networks, which integrates systematic and nodal thinking on resilience research in network science (Wang et al., 2020) with the potential and connectivity concepts of resilience research in urban science (Philip Cooke et al., 2012), as well as the hierarchical and assortativity characteristics of recent research on urban network resilience (Wei and Pan, 2021). This framework contributes to a comprehensive perception of stability and adaptability issues in the development of innovation (characterized by uncertainty and sensitivity) and strives to promote the expanded application of the concept of resilience in the field of innovation.
Using this framework, this article analyses the resilience characteristics of Chinese urban technology cooperation innovation networks. From a systemic perspective, Chinese urban innovation networks exhibit relatively low hierarchical and yearly decreasing heterogeneity characteristics. Compared with intercity transportation network, information network, and economic network, the innovation network in China has a lower hierarchy (Wei and Pan, 2021), indicating that China still lacks cities with stronger innovation-leading ability. The disassortativity indicates that Chinese cities are characterized by “preferential attachment” in the innovation process (Wei and Pan, 2021), but the continuous decline in the past 3 years reflects that the cooperative innovation of cities at the same level has increased. From a nodal perspective, both the potential and connectivity of urban innovation show a spatial pattern of high in the southeast and low in the northwest, and cities with higher administrative level have higher innovation resilience. These patterns are basically consistent with other studies, confirming the spatial differentiation characteristics divided by the Hu line in China (Wei and Pan, 2021) and the spatial political bias of urban development. Although this article only explores the national innovation resilience from the perspective of urban networks of co-invention, it is also closely related to the national natural, cultural, and social landscape, which can be confirmed by the Hu Line reflecting China’s spatial differentiation due to its comprehensive nature. These results are more representative of the internal structure of the innovation resilience of urban networks than the resilience values calculated by a formula and contribute to a deeper understanding of the current status of innovation resilience in China.
Resilience has become a highly elusive concept (Veloz et al., 2022), and its application in the field of innovation is just beginning. There are also some shortcomings in the measurement of urban innovation network resilience, for example, what is the optimal interval range of hierarchy? And whether the assortativity is better or disassortativity is better, which have not been solved in this article. We have to say, these problems are difficult to solve in one article. This is a question we are committed to address in our future research. Due to practical difficulties such as data acquisition and processing, the impact of COVID-19 on the national innovation resilience network is not discussed in this article, which will be further explored in future research. Referring to the division of industrial space clusters (Li et al., 2019), we can further divide resilience space clusters on the basis of resilience measurement and propose more targeted policy suggestions to improve regional innovation resilience. This article is only an exploration of the innovation resilience at the national scale from the perspective of urban networks, while a true understanding of innovation resilience requires more extensive research on different scales, different subjects, and different knowledge flows. Only in this way can the concept of resilience be better applied to innovation development, both theoretically and practically.
Data availability statement
The original contributions presented in the study are included in the article/supplementary material; further inquiries can be directed to the corresponding author.
Author contributions
HM and XL contributed to the conception and design of the study. XL and JL organized the database. XL, JL, and HM performed the statistical analysis. HM, XX, and XL wrote the first draft of the manuscript. HM, XX, XL, and JL wrote sections of the manuscript. All authors contributed to manuscript revision, read, and approved the submitted version.
Funding
The author(s) disclosed receipt of the following financial support for the research, authorship and/or publication of this article: This work is supported by the National Natural Science Foundation of China (grant no. 41971209), the Third Xinjiang Scientific Expedition Program (grant no. 2021xjkk0900), and the Second Tibetan Plateau Scientific Expedition and Research Program (STEP) (grant no. 2019QZKK1005).
Conflict of interest
The authors declare that the research was conducted in the absence of any commercial or financial relationships that could be construed as a potential conflict of interest.
Publisher’s note
All claims expressed in this article are solely those of the authors and do not necessarily represent those of their affiliated organizations, or those of the publisher, the editors, and the reviewers. Any product that may be evaluated in this article, or claim that may be made by its manufacturer, is not guaranteed or endorsed by the publisher.
References
Albert, R., and Barabasi, A.-L. (2001). Statistical mechanics of complex networks. Rev. Mod. Phys. 74, 47–97. doi:10.1103/RevModPhys.74.47
Allenby, B., and Fink, J. (2005). Toward inherently secure and resilient societies. Science 309 (5737), 1034–1036. doi:10.1126/science.1111534
Amin, A., and Cohendet, P. (2004). Architectures of knowledge, companies, capabilities, and communities. Oxford: Oxford University Press.
Andergassen, R., Nardini, F., and Ricottilli, M. (2014). Emergence and resilience in a model of innovation and network formation. Netw. Spat. Econ. 15 (2), 293–311. doi:10.1007/s11067-014-9262-6
Asheim, B. T., and Isaksen, A. (2002). Regional innovation systems: The integration of local ‘sticky’ and global ‘ubiquitous’ knowledge. J. Technol. Transf. 27 (1), 77–86. doi:10.1023/a:1013100704794
Athey, G., Nathan, M., Webber, C., and Mahroum, S. (2008). Innovation and the city. Innov. (Camb). 10(2-3), 156–169. doi:10.5172/impp.453.10.2-3.156
Atwell, R. C., Schulte, L. A., and Westphal, L. M. (2008). Linking resilience theory and diffusion of innovations theory to understand the potential for perennials in the U.S. Corn belt. Ecol. Soc. 14 (1), 30. doi:10.5751/es-02787-140130
Balland, P. A., Rigby, D., and Boschma, R. (2015). The technological resilience of US cities. Camb. J. Regions Econ. Soc. 8 (2), 167–184. doi:10.1093/cjres/rsv007
Barabási, A.-L., and Albert, R. (1999). Emergence of scaling in random networks. Science 286 (5439), 509–512. doi:10.1126/science.286.5439.509
Barabasi, A. L., Albert, R., and Jeong, H. (2000). Scale-free characteristics of random networks: The topology of the world-wide web. Phys. A Stat. Mech. its Appl. 281 (1-4), 69–77. doi:10.1016/s0378-4371(00)00018-2
Bathelt, H., Malmberg, A., and Maskell, P. (2004). Clusters and knowledge: Local buzz, global pipelines and the process of knowledge creation. Prog. Hum. Geogr. 28 (1), 31–56. doi:10.1191/0309132504ph469oa
Berkes, F., Colding, J., and Folke, C. (2003). Navigating social-ecological systems: Building resilience for complexity and change. Cambridge, UK: Cambridge University Press.
Boschma, R. (2005). Proximity and innovation: A critical assessment. Reg. Stud. 39, 61–74. doi:10.1080/0034340052000320887
Boschma, R. (2015). Towards an evolutionary perspective on regional resilience. Reg. Stud. 49 (5), 733–751. doi:10.1080/00343404.2014.959481
Brede, M., and de Vries, B. J. M. (2009). in Networks that optimize a trade-off between efficiency and dynamical ResilienceComplex sciences. Editor J. Zhou (Berlin, Heidelberg: Springer Berlin Heidelberg).
Burnard, K., and Bhamra, R. (2011). Organisational resilience: Development of a conceptual framework for organisational responses. Int. J. Prod. Res. 49, 5581–5599. doi:10.1080/00207543.2011.563827
Carrington, P. J., Scott, J., and Wasserman, S. (2005). Models and methods in social network analysis. Cambridge: Cambridge University Press.
Cattani, G., and Ferriani, S. (2008). A core/periphery perspective on individual creative performance: Social networks and cinematic achievements in the hollywood film industry. Organ. Sci. 19 (6), 824–844. doi:10.1287/orsc.1070.0350
Christopherson, S., Michie, J., and Tyler, P. (2010). Regional resilience: Theoretical and empirical perspectives. Camb. J. Regions Econ. Soc. 3 (1), 3–10. doi:10.1093/cjres/rsq004
Clark, J., Huang, H. I., and Walsh, J. P. (2010). A typology of 'innovation districts': What it means for regional resilience. Camb. J. Regions, Econ. Soc. 3 (1), 121–137. doi:10.1093/cjres/rsp034
Clausen, T., Pohjola, M., Sapprasert, K., and Verspagen, B. (2012). Innovation strategies as a source of persistent innovation. Industrial Corp. Change 21 (3), 553–585. doi:10.1093/icc/dtr051
Cooke, P., Parrilli, M. D., and Curbelo, J. L. (2012). Innovation, global change and territorial resilience. Cheltenham: Edward Elgar.
Cooke, P. (2001). Regional innovation systems, clusters, and the knowledge economy. Industrial Corp. Change 10, 945–974. doi:10.1093/icc/10.4.945
Crespo, J., Suire, R., and Vicente, J. (2013). Lock-in or lock-out? How structural properties of knowledge networks affect regional resilience. J. Econ. Geogr. 14 (1), 199–219. doi:10.1093/jeg/lbt006
Dabson, B., Heflin, C., and Miller, K. (2012). Regional resilience. Research and policy brief, RUPRI, rural futures lab, harry S. Truman School of public affairs. Missouri: University of Missouri.
Davoudi, S., Brooks, E., and Mehmood, A. (2013). Evolutionary resilience and strategies for climate adaptation. Plan. Pract. Res. 28 (3), 307–322. doi:10.1080/02697459.2013.787695
Dorogovtsev, S. N., Goltsev, A. V., and Mendes, J. F. F. (2008). Critical phenomena in complex networks. Rev. Mod. Phys. 80 (4), 1275–1335. doi:10.1103/RevModPhys.80.1275
Dost, M., Pahi, M. H., Magsi, H. B., and Umrani, W. A. (2019). Effects of sources of knowledge on frugal innovation: Moderating role of environmental turbulence. J. Knowl. Manag. 23 (7), 1245–1259. doi:10.1108/JKM-01-2019-0035
Duguet, E., and Monjon, S. (2002). Creative destruction and the innovative core: Is innovation persistent at the firm level? Discussion paper. London: University College.
Eisenhardt, K. M., Furr, N. R., and Bingham, C. B. (2010). CROSSROADS—microfoundations of performance: Balancing efficiency and flexibility in dynamic environments. Organ. Sci. 21 (6), 1263–1273. doi:10.1287/orsc.1100.0564
Erhardt, G., Marsili, M., and Vega-Redondo, F. (2007). Emergence and resilience of social networks: A general theoretical framework. Switzerland: Annales d'Economie et de Statistique, 1–14.
Erol, O., Sauser, B. J., and Mansouri, M. (2010). A framework for investigation into extended enterprise resilience. Enterp. Inf. Syst. 4 (2), 111–136. doi:10.1080/17517570903474304
Euchner, J. (2019). Innovation and resilience. Research-Technology Manag. 62 (4), 10–11. doi:10.1080/08956308.2019.1613113
Farjoun, M. (2010). Beyond dualism: Stability and change as a duality. Acad. Manage. Rev. 35(2), 202–225. doi:10.5465/Amr.2010.48463331
Fastenrath, S., Coenen, L., and Davidson, K. (2019). Urban resilience in action: The resilient melbourne strategy as transformative urban innovation policy? Sustainability 11 (3), 693. doi:10.3390/su11030693
Fitjar, R. D., and Rodriguez-Pose, A. (2011). When local interaction does not suffice: Sources of firm innovation in urban Norway. Environ. Plan. A 43, 1248–1267. doi:10.1068/a43516
Fleming, L. (2007). Breakthroughs and the "Long tail" of innovation. Mit Sloan Manag. Rev. 49 (1), 69.
Folke, C. (2006). Resilience: The emergence of a perspective for social-ecological systems analyses. Glob. Environ. Change 16 (3), 253–267. doi:10.1016/j.gloenvcha.2006.04.002
Freeman, C., and Soete, L. (1997). The economics of industrial innovation. Cambridge: The MIT Press.
Gilly, J. P., Kechidi, M., and Talbot, D. (2014). Resilience of organisations and territories: The role of pivot firms. Eur. Manag. J. 32 (4), 596–602. doi:10.1016/j.emj.2013.09.004
Gunderson, L. H., and Holling, C. S. (2002). Panarchy: Understanding transformations in human and natural systems. Washington, D.C., USA: Island Press.
Hassink, R. (2010). Regional resilience: A promising concept to explain differences in regional economic adaptability? Camb. J. Regions Econ. Soc. 3 (1), 45–58. doi:10.1093/cjres/rsp033
Holling, C. S. (1973). Resilience and stability of ecological systems. Annu. Rev. Ecol. Syst. 4, 1–23. doi:10.1146/annurev.es.04.110173.000245
Hollnagel, E., Woods, D. D., and Leveson, N. G. (2006). Resilience engineering : Concepts and precepts.
Hosseini, S., Barker, K., and Ramirez-Marquez, J. E. (2016). A review of definitions and measures of system resilience. Reliab. Eng. Syst. Saf. 145, 47–61. doi:10.1016/j.ress.2015.08.006
Ip, W. H., and Wang, D. (2011). Resilience and friability of transportation networks: Evaluation, analysis and optimization. IEEE Syst. J. 5 (2), 189–198. doi:10.1109/jsyst.2010.2096670
Jackson, W. A. (1999). Dualism, duality and the complexity of economic institutions. Int. J. Soc. Econ. 26 (4), 545–558. doi:10.1108/03068299910215997
Janssen, M. A., Schoon, M. L., Ke, W. M., and Borner, K. (2006). Scholarly networks on resilience, vulnerability and adaptation within the human dimensions of global environmental change. Glob. Environ. Change 16 (3), 240–252. doi:10.1016/j.gloenvcha.2006.04.001
Kurth, M., Kozlowski, W., Ganin, A., Mersky, A., Leung, B., Dykes, J., et al. (2020). Lack of resilience in transportation networks: Economic implications. Transp. Res. Part D-Transport Environ. 86, 102419. doi:10.1016/j.trd.2020.102419
Lawson, C. (1999). Towards a competence theory of the region. Camb. J. Econ. 23, 151–166. doi:10.1093/cje/23.2.151
Lee, D.-S. (2018). Towards urban resilience through inter-city networks of Co-invention: A case study of U.S. Cities. Sustainability 10 (2), 289. doi:10.3390/su10020289
Lengnick-Hall, C. A., and Beck, T. E. (2005). Adaptive fit versus robust transformation: How organizations respond to environmental change. J. Manag. 31 (5), 738–757. doi:10.1177/0149206305279367
Lengnick-Hall, C. A., Beck, T. E., and Lengnick-Hall, M. L. (2011). Developing a capacity for organizational resilience through strategic human resource management. Hum. Resour. Manag. Rev. 21 (3), 243–255. doi:10.1016/j.hrmr.2010.07.001
Li, C., Wu, K., and Gao, X. (2019). Manufacturing industry agglomeration and spatial clustering: Evidence from Hebei Province, China. Environ. Dev. Sustain. 22 (4), 2941–2965. doi:10.1007/s10668-019-00328-1
Linnenluecke, M. K. (2017). Resilience in business and management research: A review of influential publications and a research agenda. Int. J. Manag. Rev. 19 (1), 4–30. doi:10.1111/ijmr.12076
Liu, J. G., Dietz, T., Carpenter, S. R., Alberti, M., Folke, C., Moran, E., et al. (2007). Complexity of coupled human and natural systems. Science 317 (5844), 1513–1516. doi:10.1126/science.1144004
Lundvall, B. Å. (1992). National systems of innovation: Toward a theory of innovation and interactive learning. London: Anthem Press.
Lv, W.-D., Tian, D., Wei, Y., and Xi, R.-X. (2018). Innovation resilience: A new approach for managing uncertainties concerned with sustainable innovation. Sustainability 10 (10), 3641. doi:10.3390/su10103641
Ma, H. T., Fang, C. L., Lin, S. N., Huang, X. D., and Xu, C. D. (2018). Hierarchy, clusters, and spatial differences in Chinese inter-city networks constructed by scientific collaborators. J. Geogr. Sci., 1793–1809. doi:10.1007/s11442-018-1579-5
Ma, H. T., Fang, C. L., Pang, B., and Wang, S. J. (2015). Structure of Chinese city network as driven by technological knowledge flows. Chin. Geogr. Sci. 25 (4), 498–510. doi:10.1007/s11769-014-0731-0
Ma, H. T., Li, Y. C., and Huang, X. D. (2021). Proximity and the evolving knowledge polycentricity of megalopolitan science: Evidence from China’s Guangdong-Hong Kong-Macao Greater Bay Area, 1990–2016. Urban Stud. 58 (12), 2405–2423. doi:10.1177/0042098020942665
Ma, H. T., and Xu, X. F. (2022). Knowledge polycentricity of China’s urban agglomerations. J. Urban Plan. Dev. 148 (2), 04022014. doi:10.1061/(ASCE)UP.1943-5444.0000828
McDonald, N. (2006). Organisational resilience and industrial risk. USA: Ashgate publishing company.
Modica, M., and Reggiani, A. (2015). Spatial economic resilience: Overview and perspectives. Netw. Spat. Econ. 15 (2), 211–233. doi:10.1007/s11067-014-9261-7
Moodysson, J. (2008). Principles and practices of knowledge creation: On the organization of "buzz" and "pipelines" in life science communities. Econ. Geogr. 84 (4), 449–469. doi:10.1111/j.1944-8287.2008.00004.x
Newman, M. E. J. (2003). Mixing patterns in networks. Phys. Rev. E 67 (2), 026126. doi:10.1103/physreve.67.026126
Nooteboom, B., and Gilsing, V. A. (2004). Density and strength of ties in innovation networks: A competence and governance view. Rotterdam: Erasmus Research Institute of Management (ERIM).
Ouyang, M., Dueñas-Osorio, L., and Min, X. (2012). A three-stage resilience analysis framework for urban infrastructure systems. Struct. Saf. 36-37, 23–31. doi:10.1016/j.strusafe.2011.12.004
Pike, A., Dawley, S., and Tomaney, J. (2010). Resilience, adaptation and adaptability. Camb. J. Regions Econ. Soc. 3 (1), 59–70. doi:10.1093/cjres/rsq001
Pinto, H., Noronha, T., and Vaz, E. (2018). Economic crisis, turbulence and the resilince in innovation: Insights from the atlantic martime cluster. Resil. Regional Dyn. Int. Approach a New Res. Agenda, E1. doi:10.1007/978-3-319-95135-5_14
Ponis, S. T., and Koronis, E. (2012). Supply chain resilience: Definition of concept and its formative elements. J. Appl. Bus. Res. 28 (5), 921. doi:10.19030/jabr.v28i5.7234
Popadiuk, S., and Choo, C. W. (2006). Innovation and knowledge creation: How are these concepts related? Int. J. Inf. Manag. 26 (4), 302–312. doi:10.1016/j.ijinfomgt.2006.03.011
Reggiani, A., De Graaff, T., and Nijkamp, P. (2002). Resilience: An evolutionary approach to spatial economic systems. Netw. Spat. Econ. 2 (2), 211–229. doi:10.1023/A:1015377515690
Reggiani, A. (2013). Network resilience for transport security: Some methodological considerations. Transp. Policy 28, 63–68. doi:10.1016/j.tranpol.2012.09.007
Reggiani, A., and Nijkamp, P. (2006). Spatial dynamics, networks and modelling. Cheltenham, UK: Edward Elgar Publishing.
Rose, A., and Krausmann, E. (2013). An economic framework for the development of a resilience index for business recovery. Int. J. Disaster Risk Reduct. 5, 73–83. doi:10.1016/j.ijdrr.2013.08.003
Salter, A., and Alexy, O. (2014). The nature of innovation. United Kingdom: Oxford University Clarendon street.
Sanchez, A. X., Osmond, P., and van der Heijden, J. (2017). Are some forms of resilience more sustainable than others? Procedia Eng. 180, 881–889. doi:10.1016/j.proeng.2017.04.249
Schumpeter, J. A. (1939). Business cycles: A theoretical, historical and statistical analysis of the capitalist process. New York: McGraw-Hill.
Schweitzer, F., Fagiolo, G., Sornette, D., Vega-Redondo, F., Vespignani, A., and White, D. R. (2009). Economic networks: The new challenges. Science 325 (1095-9203), 422–425. (Electronic)). doi:10.1126/science.1173644
Simmie, J., and Martin, R. (2010). The economic resilience of regions: Towards an evolutionary approach. Camb. J. Regions Econ. Soc. 3 (1), 27–43. doi:10.1093/cjres/rsp029
Sonenshein, S. (2016). Routines and creativity: From dualism to duality. Organ. Sci. 27 (3), 739–758. doi:10.1287/orsc.2016.1044
Suire, R., and Vicente, J. (2014). Clusters for life or life cycles of clusters: In search of the critical factors of clusters' resilience. Entrepreneursh. Regional Dev. 26 (1-2), 142–164. doi:10.1080/08985626.2013.877985
Sun, Y., and Luo, M. (2021). Development and enlightenment of American intelligent manufacturing. Bull. Chin. Acad. Sci. 36 (11), 1316–1325.
Sunley, P. (2013). Innovation, global change and territorial resilience. Reg. Stud. 47 (4), 644–645. doi:10.1080/00343404.2013.781754
Van Wellen, E. (2019). Global engineering congress (GEC): Notes and goals from the 2018 conference. J. Coast. Res. 35 (3), 485–488. doi:10.2112/JCOASTRES-D-19A-00004.1
Veloz, T., Maldonado, P., Bussseniers, E., Bassi, A., Beigi, S., Lenartowicz, M., et al. (2022). Towards an analytic framework for system resilience based on reaction networks. Complexity 2022, 1. doi:10.1155/2022/9944562
Vogus, T. J., and Sutcliffe, K. M. (2003). Organizing for resilience. San Francisco: Berrett-Koehler.
Walker, B., Carpenter, S., Anderies, J., Abel, N., Cumming, G., Janssen, M., et al. (2002). Resilience management in social-ecological systems: A working hypothesis for a participatory approach. Conserv. Ecol. 6 (1), art14. doi:10.5751/es-00356-060114
Wang, L., Xue, X., and Zhou, X. (2020). A new approach for measuring the resilience of transport infrastructure networks. Complexity 2020, 1–16. doi:10.1155/2020/7952309
Wang, Z. (2007). Technological innovation and market turbulence: The dot-com experience. Rev. Econ. Dyn. 10 (1), 78–105. doi:10.1016/j.red.2006.10.001
Watts, D. J., and Strogatz, S. H. (1998). Collective dynamics of ‘smallworld’networks. nature 393 (6684), 440–442. doi:10.1038/30918
Wei, S. M., and Pan, J. H. (2021). Resilience of urban network structure in China: The perspective of disruption. ISPRS Int. J. Geoinf. 10 (12), 796. doi:10.3390/ijgi10120796
Keywords: innovation resilience, network resilience, urban network, co-invention, patent, China
Citation: Ma H, Xu X, Li X and Liu J (2022) Towards innovation resilience through urban networks of co-invention: A case study of cities in China. Front. Earth Sci. 10:974219. doi: 10.3389/feart.2022.974219
Received: 21 June 2022; Accepted: 29 August 2022;
Published: 19 September 2022.
Edited by:
Bing Xue, Institute of Applied Ecology (CAS), ChinaReviewed by:
Chenxi Li, Xi’an University of Architecture and Technology, ChinaJun Yang, Northeastern University, China
Copyright © 2022 Ma, Xu, Li and Liu. This is an open-access article distributed under the terms of the Creative Commons Attribution License (CC BY). The use, distribution or reproduction in other forums is permitted, provided the original author(s) and the copyright owner(s) are credited and that the original publication in this journal is cited, in accordance with accepted academic practice. No use, distribution or reproduction is permitted which does not comply with these terms.
*Correspondence: Haitao Ma, bWFodEBpZ3NucnIuYWMuY24=