- 1Instutite of Environmental Physics, Heidelberg University, Heidelberg, Germany
- 2Max-Planck-Institute for Meteorology, Hamburg, Germany
- 3Institute of Geological Science, Freie Universität Berlin, Berlin, Germany
Understanding how stalagmites grow under changing climate conditions is of great significance for their application as a paleoclimate archive. In this study, we present a shape modeling approach to stalagmite growth by combining three existing models accounting for climate variables, karst water chemistry, and speleothem deposition. The combined model requires only four input parameters: calcium concentration of the water drop, drip interval, cave temperature, and cave carbon dioxide (
1 Introduction
Stalagmites are potential archives for paleoclimatology because they grow in a protected environment for 103–105 years and record changes in climatic conditions both within the cave and above the cave, such as precipitation, temperature, and vegetation, through modifications of their shape and isotopic composition (Fairchild et al., 2006; Götrük et al., 2011). While isotopic proxies measured in the carbonate and fluid inclusions from sampled stalagmite material are standard tools in reconstructing paleo-climate signals (Fleitmann et al., 2003), the geometrical information of the shape of a stalagmite has received less attention. First, efforts have been undertaken considering the theoretical growth models by Dreybrodt (1999). These early models have been used to discuss changes in climatic forcing, such as temperature changes, changes in precipitation and in carbon dioxide concentration, and the growth geometries of stalagmites (Kaufmann, 2003). Later, these climate variables have been recovered from both synthetic and real stalagmite geometries by formal inversion (Kaufmann and Dreybrodt, 2004). However, difficulties in extracting climate information from stalagmite shapes arise from the overlaying soil and karst which affects soil
Hence, we attempt to couple models of stalagmite growth, climate, and infiltration that permit a fully independent prediction of the growth of stalagmites. We test our coupling for a particularly well-studied speleothem from the Sofular cave in Turkey, for which most classical proxies have been measured, including the growth history of the speleothem. The applied models have a tremendous degree of freedom in a large number of poorly constrained variables; hence, we attempt to simulate a real-world speleothem throughout the last glacial termination (Berger, 1978), for which an Earth System model (ESM) supplies strong changes in soil activity, precipitation, and local temperature. Our results strongly suggest that the combination of the different model outputs might permit sufficient constraints to derive important conclusions for future work concerning speleothem growth and shapes.
2 Methods
2.1 Sofular Cave and stalagmite So-1
Sofular Cave is located in Northern Turkey, less than 10 km from the coast of the Black Sea at 41°25’N and 31°56’E (Figure 1). It is situated on the northern foothills of the Akçakoca Mountain range, a part of the North Anatolian Mountain chain in Turkey (Laumanns and Kaufmann, 1988; Götrük et al., 2011), and developed in the lower cretaceous limestone (Tüysüz, 1999). The vegetation above is dense and consists mainly of deciduous trees (oak, beech, and elms) and shrubs. The soil above the Sofular Cave is thin, with an estimated maximum depth of approximately 30 cm. The highly fissured bedrock above the cave is around 40 m thick, which suggests a fast response (days to weeks) of travel time for precipitation to the drip site in the cave (Badertscher et al., 2014). As a reference stalagmite, we mainly work with the So-1 stalagmite, recovered in August 2005. It is candle-shaped, 1.70 m high, and started growing at around 50.3 kyr BP (Fleitmann et al., 2009). Because most of the growth processes took place in the last 25 kyr (1.35 m out of 1.7 m), we focus on this time interval. It contains the main growth process, and changes in growth rates can be investigated. As the exact sampling position has not been recorded, we discuss the influence of the possible locations in the cave.
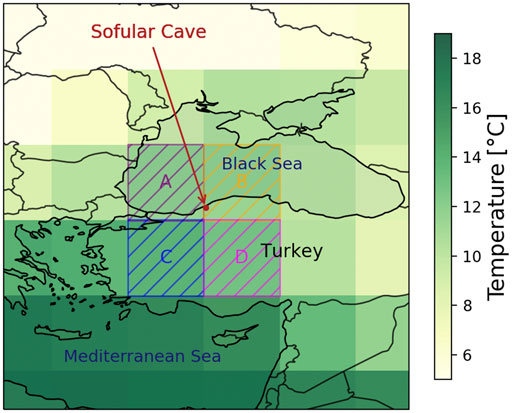
FIGURE 1. Location of Sofular Cave in Turkey, and four cells of the ESM model (A–D). Colors represent modeling surface temperatures from the ESM run for the present day.
To verify the output of our shape model, the resulting age/depth plot of the simulation is compared with measured growth data taken at the apex of the stalagmite. There are many different publications about the precise measurement of the age profile, not just for the So-1 but also for the So-2 stalagmite (Fleitmann et al., 2009; Therre, 2020). For further investigation, the actual shape of the simulated and real-world stalagmite is compared (Figure 2). Both stalagmites have recorded favorable growth conditions and periods, where no growth was happening. Generally, the climatic conditions during the Last Glacial Maximum (LGM) were less favorable for stalagmite growth when compared to the last 10 kyr. As the So-1 stalagmite grows continuously for the interested time interval, it is chosen as a further reference.
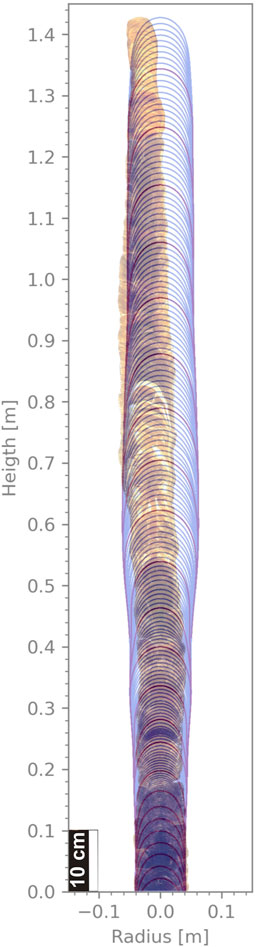
FIGURE 2. So-1 stalagmite and FLOW modeled stalagmite superimposed for comparison. Shapes of the simulation are redrawn every 100 years by blue lines, whereas purple lines indicate 1,000 years steps.
2.2 Earth system model MPI–ESM and climate output
We use climate variables from a transient deglaciation experiment performed with the Max Planck Institute for Meteorology Earth System Model (MPI-ESM), version 1.2 (Mauritsen et al., 2019), in spatial resolution T31GR30 (Meccia and Mikolajewicz, 2018), corresponding to roughly 3.75° in latitude and longitude at the equator. The model includes a full representation of the terrestrial carbon cycle.
After a spin-up at constant 26 ka BP boundary conditions, the model experiment covers the time period from 26 ka BP to the present day. We prescribed orbital forcing from Berger (1978) and greenhouse gases from Köhler et al. (2017). Furthermore, we prescribed ice-sheet extent from the GLAC-1D ice sheet reconstruction (Tarasov et al., 2012; Briggs et al., 2014; Ivanovic et al., 2016). Ice-sheet extent, as well as bathymetry and topography (Meccia & Mikolajewicz, 2018), and river routing (Riddick et al., 2018) were continuously updated at ten-year intervals throughout the deglaciation.
From this experiment, we used the near-surface air temperature and precipitation fields, obtained as means over 100 years, to drive our model. Furthermore, we used the soil carbon content and heterotrophic respiration from the decomposition of litter detritus and soil organic matter by microorganisms.
2.3 Parameterization to the soil CO2
To calculate soil
With time t and depth z, but for steady-state conditions (dcsoil/dt=0). The diffusion coefficient for
With the boundary conditions
In this study, we chose a characteristic depth of z* = 30 cm, which is commonly taken as the boundary between the topsoil and subsoil layers (Hiederer and Koechy, 2011). Additionally, studies at Sofular Cave suggested a maximum soil depth between 30 and 50 cm (Rudzka et al., 2011; Badertscher et al., 2014), which further supports our chosen value for z*. In this layer, the majority of
2.4 Soil and epikarst model CaveCalc
The chemical and physical processes in the soil and the karst above the cave are modeled using CaveCalc, a numerical model for speleothem chemistry and isotopes (Owen et al., 2018). The chemistry in CaveCalc is calculated with the program PHREEQC (Parkhurst and Appelo, 2013), a program simulating aqueous geochemistry. A CaveCalc run involves two PHREEQC-based steps: an equilibration step for a reference volume of water with a predefined soil gas and a subsequent step equilibrating the same water with limestone. This simulates
2.5 Shape model for stalagmite growth
The shape model contains the three existing parameterizations (FLOW, EXP, and GAUSS) of stalagmite growth and allows a mixing process for the latter two. For exhaustive descriptions of those models, the reader is referred to the respective first publication (Dreybrodt, 1999; Kaufmann, 2003; Romanov et al., 2008). The underlying geometry model to describe the surface of the stalagmite with a polygon is explained by Kaufmann (2003). Here, we just state the results.
We can separate the shape model into two parts (FLOW and EXP/GAUSS). We start with the FLOW equations by stating the underlying initial deposition rate F [
The index 0 refers to the position of the deposition rate and indicates that we look at the apex of the stalagmite.
Here,
with
The model is applied for the film thickness values
Through this approach, two different descriptions of the decrease in deposition rate along the horizontal profile are possible: The exponential relationship (referred to as “EXP”) is described by
The Gaussian distribution (referred to as “GAUSS”) has the same functional assumptions as the EXP but assumes a Gaussian relationship for the square of the distance (variance) over the mean radius:
These methods also allow a mixing process between the water drop and the solution on top of the stalagmite according to Mühlinghaus et al. (2007).
2.6 Free parameters and fixed constraints
We start with a naive approach and use the simulated output of the MPI-ESM. For the temperature in the cave, we use the surface temperatures from the four box units around the Sofular location, without weighting any of the boxes as being more important than others (Supplementary Figures S2, S3). The resulting temperature corresponds to the arithmetic mean of the four boxes:
To estimate the drip interval is very difficult. Hence, we here follow a very simplistic parameterization in the absence of any information about past drip intervals. We assume an artificially chosen lowest drip interval of 25 s and expect this number to dramatically increase with decreasing precipitation. We have chosen to couple the drip interval
(Supplementary Figure S4) It should be noted that the resulting drip interval time series is completely artificial and has no real physical correspondence as it just scales the drip interval to fit the shape of the So-1 stalagmite based on all other time series. The factor γ converts the units (mm/year) into seconds; hence, [γ] = 1s year/mm.
The
The
3 Results
We now use the FLOW model and the standard parameter values derived to simulate the So-1 stalagmite. The age/depth plot in Figure 3A reveals that the calculated values with
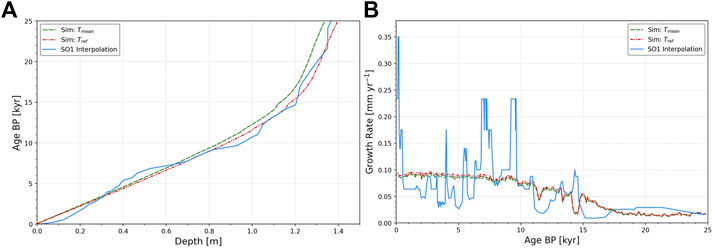
FIGURE 3. Age/depth (A) and growth rate/depth (B) plot for the naive approach, the best fit, and the experimental data. Solid lines represent a linear interpolation to construct the age through stable oxygen isotopes which follow the U/Th dates (Badertscher et al., 2011).
The resulting stalagmite shape using this modified temperature
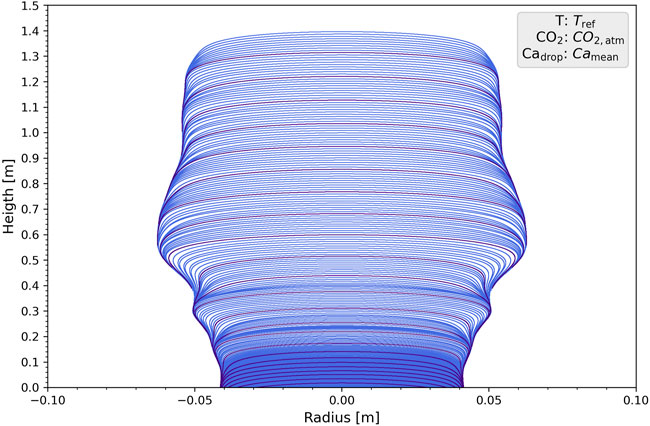
FIGURE 4. Simulated stalagmite with the FLOW model. Stalagmite starts growing in equilibrium, and the temperature is modified to achieve the best fit in the depth/age plot. Shapes of the simulation are redrawn every 100 years by the blue lines, whereas the purple lines indicate 1,000 years steps.
To obtain a better fit, we modify the temperature function according to
With this parameterization, we reduce the amplitude of the temporal variation of the cave temperature (damping) and compensate by a static shift.
This will be the reference stalagmite for all further investigations in this study, which is also superimposed on the real shape in Figure 2. Through modification of growth conditions, the resulting stalagmite agrees with the age/depth plot quite well (see Figure 3A). It is important to mention that the simulated data only follow the mean trends of the growth rate with smaller values during the glacial and increasing values during the interglacial. The model is, however, not capable of reproducing fluctuations of the growth around the mean. This is demonstrated in a growth rate versus time plot (see Figure 3B), for which the observed data show strong temporal variability around the mean with fluctuations of up to an order of magnitude, while the model predicts rather smooth growth, except for a short growth decline during the Bölling/Allerod warming around 14.5 kyr ago.
3.1 Comparing different shape model approaches
The modeled stalagmite radius mainly depends on the drip interval
There are two additional arguments in favor of picking the FLOW model: first, the growth rate of the FLOW model is independent of the unconstrained, freely chosen drip interval τ, as described by Eq. 4. Here, the growth rates predicted by the FLOW model correspond better to the real growth rate derived from U/Th dating (Fleitmann et al., 2009). Second, the equations of the FLOW model are faster in reaching an equilibrium status (Romanov et al., 2008). This means that changes in the input parameters are reflected more quickly in the shape of the stalagmite. Given the correct prediction of speleothem height and shape of the tip, we opt here to use only the FLOW model for further discussion of the sensitivity and influence of several unknown parameters on the growth and shape of the simulated So-1. In Figure 2, the results of the stalagmite shape from a FLOW model run are placed over the photo of the So-1 stalagmite. The photo is cropped to show the growth of the last 25 kyr, according to U/Th dating (Fleitmann et al., 2009). There are some large deviations: first, the modeled radius becomes far too large. The model predicts a bigger radius at the tip, but the real stalagmite does not confirm this prediction. Obviously, the description of the relation between precipitation and drip interval by Eq. 14 is only a rough approximation. Additionally, Figure 2 shows that the peaks of the simulation and the real So-1 stalagmite do not match in their horizontal position. The So-1 stalagmite has some variations in the apex to the left and right, which probably results from small changes in the drip locations or other external effects (Rajendran et al., 2015).
4 Discussion
In the following text, we will discuss in more detail the role of different responses of the shape model with respect to the key variables. We again use the MPI-ESM1.2 and CaveCalc model output to avoid multiple dependencies of model coupling. We are fully aware that the total coupled uncertainty of the three interacting models with numerous estimated parameters and transfer functions implies a largely unexplored and possible systematic source of error in shape prediction. Here, we want to discuss the response and partial sensitivity of the shape model to its input and a few interesting predictions resulting from it. As the full discussion of testing the general applicability of such an approach would need a larger set of environments and test speleothems, it is yet beyond the scope of this work. Therefore, we focus as stated on the key drivers and the resulting implications and investigate the role of the input parameters.
4.1 Cave temperature
Temperature plays a significant role in the shape of the stalagmite, with a reduction in the temperature resulting in less growth, whereas an increase in temperature enhances the growth (Kaufmann, 2003). This is illustrated in Supplementary Figure S8 and results from the temperature-dependent calculation of the apparent calcium concentration inside the cave
4.2 Drip interval
Since the modeled age over depth is independent of the drip interval, we must evaluate the radius, here the equilibrium radius, to identify the effect of drip rates. Supplementary Figure S9 shows the simulated equilibrium radius/age graphs for different drip intervals. According to Eq. 5, lowering the drip interval results in a larger radius. The more interesting case is the situation of an even higher interval. As demonstrated, the radius does not vary a lot after a certain threshold is passed, which has already been discussed in Kaufmann (2003) and Romanov et al. (2008). It stays rather constant at a low value. Therefore, the model suggests that a regular diameter of stalagmites can be the result of a constant drip interval or alternatively a very long one (here more than 100 s), in accordance with results from Romanov et al. (2008). The model also confirms results from Miorandi et al. (2010), as the drip interval converted to a drip rate is below 0.3l day−1 and the result is a candle-shaped stalagmite. By taking the mean value of the drip interval estimated from the precipitation data, the graph shows a clear increase of the equilibrium radius some 15 kyr ago, which highlights the influence of temperature for
4.3 Carbon dioxide concentration
The age/depth graphs for different air
4.4 Calcium concentration
By increasing the
4.5 Mixing factor
To investigate the influence of the mixing factor between the water drops, which means the renewal rate of the chemical composition of the precipitating film, we need to use the Gauss model as the flow model does not allow mixing by definition. As the stalagmite resulting from the Gauss model is shorter, we need different settings for the temperature to approximate the So-1 interpolation graph with a mixing factor of 1. It should be noted that the used temperature is still between
4.6 Coupling of input parameters
While the impacts of calcium concentration, mixing factor, and cave air
4.7 Location of the stalagmite in the cave
Taking all these aspects together, one consequence becomes clear: the position of stalagmite inside the cave may impact the shape of the stalagmite because temperature and
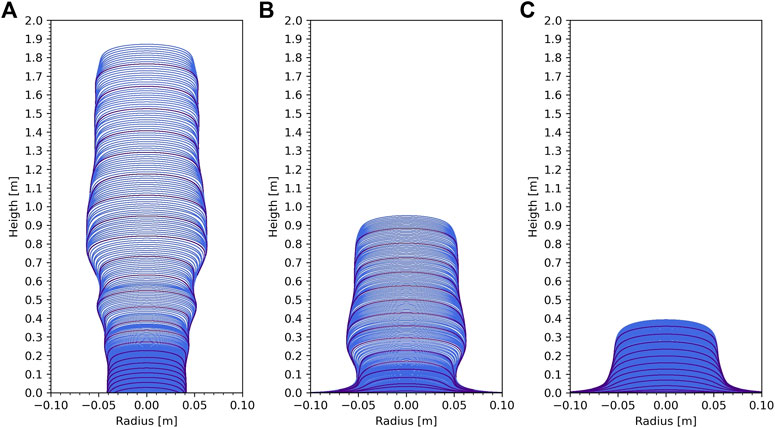
FIGURE 5. Stalagmite at different
5 Conclusion
We have demonstrated the sensitivity of speleothem growth to climatic conditions by combining algorithms to describe the equilibrium radius and the growth rate of a numerically generated stalagmite shape driven by an Earth-system model and a drip-water chemistry model. As a test case, we used the So-1 stalagmite from the Sofular Cave in Northern Turkey. The presented shape model (Romanov et al., 2008) only needs four input parameters to simulate the stalagmite: cave temperature, calcium concentration of the water drop, drip interval, and
Data availability statement
The python code is published on GitHub under the following link: https://github.com/niklas1347/Stalagmite-Simulation-Shape-Model.git.
Author contributions
NM: conceptualization, methodology, software, writing—original draft, and visualization. AH: methodology and software. TK: resources, data curation, and writing—review and editing. ST: writing—review and editing and supervision. GK: writing—review and editing and methodology. NF: conceptualization, writing—review and editing, supervision, and project administration.
Funding
This research has been supported by the DFG (Grant Nos. FR1341/4-1 and FR1341/4-2). TK. is funded by the project PalMod of the German Federal Ministry of Education and Research (BMBF), Research for Sustainability initiative FONA (Grant No. 01LP1921A). For the publication fee, we acknowledge financial support by Deutsche Forschungsgemeinschaft within the funding program “Open Access Publikationskosten” as well as by Heidelberg University.
Acknowledgments
The authors would like to thank Dominik Fleitmann for providing valuable information in form of high-resolution images of the stalagmite. We are also very grateful to anonymous reviewers for their constructive feedback, which has contributed substantially to the quality of our manuscript.
Conflict of interest
The authors declare that the research was conducted in the absence of any commercial or financial relationships that could be construed as a potential conflict of interest.
Publisher’s note
All claims expressed in this article are solely those of the authors and do not necessarily represent those of their affiliated organizations, or those of the publisher, the editors, and the reviewers. Any product that may be evaluated in this article, or claim that may be made by its manufacturer, is not guaranteed or endorsed by the publisher.
Supplementary material
The Supplementary Material for this article can be found online at: https://www.frontiersin.org/articles/10.3389/feart.2022.969211/full#supplementary-material
References
Badertscher, S., Borsato, A., Frisia, S., Cheng, H., Edwards, R., Tuysuz, O., et al. (2014). Speleothems as sensitive recorders of volcanic eruptions – The bronze age minoan eruption recorded in a stalagmite from Turkey. Earth Planet. Sci. Lett. 392, 58–66. doi:10.1016/j.epsl.2014.01.041
Badertscher, S., Fleitmann, D., Cheng, H., Edwards, R. L., Gokturk, O. M., Zumbuhl, A., et al. (2011). Pleistocene water intrusions from the mediterranean and caspian seas into the Black Sea. Nat. Geosci. 4 (4), 236–239. doi:10.1038/ngeo1106
Baker, A., Flemons, I., Andersen, M. S., Coleborn, K., and Treble, P. C. (2016). What determines the calcium concentration of speleothem-forming drip waters? Glob. Planet. Change 143, 152–161. doi:10.1016/j.gloplacha.2016.06.001
Baker, A., Genty, D., Dreybrodt, W., Barnes, W. L., Mockler, N. J., and Grapes, J. (1998). Testing theoretically predicted stalagmite growth rate with recent annually laminated samples: Implications for past stalagmite deposition. Geochimica Cosmochimica Acta 62 (3), 393–404. doi:10.1016/S0016-7037(97)00343-8
Baldini, J. U. L. (2010). Cave atmosphere controls on stalagmite growth rate and palaeoclimate records. Geol. Soc. Lond. Spec. Publ. 336 (1), 283–294. doi:10.1144/SP336.15
Berger, A. L. (1978). Long-Term variations of daily insolation and quaternary climatic changes. J. Atmos. Sci. 35 (12), 2362–2367. doi:10.1175/1520-0469(1978)035<2362:LTVODI>2.0.CO;2
Breeker, D. O., Payne, A. E., Quade, J., Banner, J. L., Ball, C. E., Meyer, K. W., et al. (2012). The sources and sinks of CO2 in caves under mixed woodland and grassland vegetation. Geochim. Cosmochim. Acta 96, 230–246. doi:10.1016/j.gca.2012.08.023
Briggs, R. D., Pollard, D., and Tarasov, L. (2014). A data-constrained large ensemble analysis of Antarctic evolution since the Eemian. Quat. Sci. Rev. 103, 91–115. doi:10.1016/j.quascirev.2014.09.003
Busenberg, E., and Plummer, L. N. (1985). Kinetic and thermodynamic factors controlling the distribution of SO32− and Na+ in calcites and selected arag- onites. Geochimica Cosmochimica Acta 49 (3), 713–725. doi:10.1016/0016-7037(85)90166-8
Cerling, T. E., Solomon, D. K., Quade, J., and Bowman, J. R. (1991). On the isotopic composition of carbon in soil carbon dioxide. Geochimica Cosmochimica Acta 55 (11), 3403–3405. doi:10.1016/0016-7037(91)90498-T
Cerling, T. E. (1984). The stable isotopic composition of modern soil carbonate and its relationship to climate. Earth Planet. Sci. Lett. 71 (2), 229–240. doi:10.1016/0012-821X(84)90089-X
Dreybrodt, W. (1999). Chemical kinetics, speleothem growth and climate. Boreas 28 (3), 347–356. doi:10.1111/j.1502-3885.1999.tb00224.x
Dreybrodt, W. (1988). Processes in karst systems: Physics, chemistry, and geology. s.l. Berlin Heidelberg: Springer.
Dreybrodt, W., and Romanov, D. (2008). Regular stalagmites: The theory behind their shape. Acta Carsologica 37 (2-3). doi:10.3986/ac.v37i2-3.145
Fairchild, I. J., Frisia, S., Borsato, A., and Tooth, A. F. (2006). “Speleothems,” in Geochemical sediments and landscapes.
Fleitmann, D., Burns, S. J., Neff, U., Mangini, A., and Matter, A. (2003). Changing moisture sources over the last 330, 000 years in Northern Oman from fluid-inclusion evidence in speleothems. Quat. Res. 60 (2), 223–232. doi:10.1016/S0033-5894(03)00086-3
Fleitmann, D., Cheng, H., Badertscher, S., Edwards, R. L., Mudelsee, M., Gokturk, O. M., et al. (2009). Timing and climatic impact of Greenland interstadials recorded in stalagmites from northern Turkey. Geophys. Res. Lett. 36, L19707. doi:10.1029/2009GL040050
Genty, D., Baker, A., and Vokal, B. (2001). Intra- and inter-annual growth rate of modern stalagmites. Chem. Geol. 176 (1-4), 191–212. doi:10.1016/s0009-2541(00)00399-5
Genty, D., Blamart, D., Ghaleb, B., Plagnes, V., Causse, C., Bakalowicz, M., et al. (2006). Timing and dynamics of the last deglaciation from European and North African δ13C stalagmite profiles—Comparison with Chinese and south hemisphere stalagmites. Quat. Sci. Rev. 25 (17-18), 2118–2142. doi:10.1016/j.quascirev.2006.01.030
Götrük, O. M., Fleitmann, D., Badertscher, S., Cheng, H., Edwards, R., Leuenberger, M., et al. (2011). Climate on the southern Black Sea coast during the holocene: Implications from the sofular cave record. Quat. Sci. Rev. 30 (19-20), 2433–2445. doi:10.1016/j.quascirev.2011.05.007
Hiederer, R., and Koechy, M. (2011). Global soil organic carbon estimates and the harmonized world soil database. Luxembourg: Publications Office of the European Union, 79. EUR 25225 EN. doi:10.2788/13267
Ivanovic, R. F., Gregoire, L. J., Kageyama, M., Roche, D. M., Valdes, P. J., Burke, A., et al. (2016). Transient climate simulations of the deglaciation 21–9 thousand years before present (version 1) – PMIP4 Core experiment design and boundary conditions. Geosci. Model Dev. 9 (7), 2563–2587. doi:10.5194/gmd-9-2563-2016
Kaufmann, G., and Dreybrodt, W. (2004). Stalagmite growth and palaeo-climate: An inverse approach. Earth Planet. Sci. Lett. 224 (3-4), 529–545. doi:10.1016/j.epsl.2004.05.020
Kaufmann, G. (2003). Stalagmite growth and palaeo-climate: The numerical perspective. Earth Planet. Sci. Lett. 214 (1-2), 251–266. doi:10.1016/S0012-821X(03)00369-8
Köhler, P., Nehrbass-Ahles, C., Schmitt, J., Stocker, T. F., and Fischer, H. (2017). A 156 kyr smoothed history of the atmospheric greenhouse gases CO<sub>2</sub>, CH<sub>4</sub>, and N<sub>2</sub>O and their radiative forcing. Earth Syst. Sci. Data 9 (1), 363–387. doi:10.5194/essd-9-363-2017
Laumanns, M., and Kaufmann, G. (1988). Drei höhlen der Region zonguldak, schwarzes meer, türkey. Verband dt. Höhlen- Karstforscher 34 (4), 110–112.
Mauritsen, T., Bader, J., Becker, T., Behrens, J., Bittner, M., Brokopf, R., et al. (2019). Developments in the MPI-M earth system model version 1.2 (MPI-ESM1.2) and its response to increasing CO2. J. Adv. Model. Earth Syst. 11 (4), 998–1038. doi:10.1029/2018MS001400
Meccia, V. L., and Mikolajewicz, U. (2018). Interactive ocean bathymetry and coastlines for simulating the last deglaciation with the Max Planck Institute earth system model (MPI-ESM-v1.2). Geosci. Model Dev. 11 (11), 4677–4692. doi:10.5194/gmd-11-4677-2018
Miorandi, R., Borsato, A., Frisia, S., Fairchild, I. J., and Richter, D. K. (2010). Epikarst hydrology and implications for stalagmite capture of climate changes at grotta di Ernesto (NE Italy): Results from long-term monitoring. Hydrol. Process. 24 (21), 3101–3114. doi:10.1002/hyp.7744
Mühlinghaus, C., Scholz, D., and Manginni, A. (2007). Modelling stalagmite growth and δ13C as a function of drip interval and temperature. Geochimica Cosmochimica Acta 71 (11), 2780–2790. doi:10.1016/j.gca.2007.03.018
Owen, R., Day, C. C., and Henderson, G. M. (2018). CaveCalc: A new model for speleothem chemistry & isotopes. Comput. Geosci. 119, 115–122. doi:10.1016/j.cageo.2018.06.011
Parkhurst, D. L., and Appelo, C. A. J. (2013). “Description of input and examples for PHREEQC version 3—a computer program for speciation, batch-reaction, one-dimensional transport, and inverse geochemical calculations,” in Modeling techniques (Denver, CO: U.S. Geological Survey). Available at: https://pubs.usgs.gov/tm/06/a43/.
Quade, J., Cerling, T. E., and Bowman, J. R. (1989). Systematic variations in the carbon and oxygen isotopic composition of pedogenic carbonate along elevation transects in the southern Great Basin. GSA Bulettin 101 (4), 464–475. doi:10.1130/0016-7606(1989)101<0464:SVITCA>2.3.CO;2
Rajendran, C. P., Sanwal, J., Morell, K. D., Sandiford, M., Kotlia, B. S., Hellstrom, J., et al. (2015). Stalagmite growth perturbations from the Kumaun Himalaya as potential earthquake recorders. J. Seismol. 20 (2), 579–594. doi:10.1007/s10950-015-9545-5
Riddick, T., Brovkin, V., Hagemann, S., and Mikolajewicz, U. (2018). Dynamic hydrological discharge modelling for coupled climate model simulations of the last glacial cycle: The MPI-DynamicHD model version 3.0. Geosci. Model Dev. 11 (10), 4291–4316. doi:10.5194/gmd-11-4291-2018
Romanov, D., Kaufmann, G., and Dreybrodt, W. (2008). Modeling stalagmite growth by first principles of chemistry and physics of calcite precipitation. Geochimica Cosmochimica Acta 72 (2), 423–437. doi:10.1016/j.gca.2007.09.038
Rudzka, D., McDermott, F., Baldini, L., Fleitmann, D., Moreno, A., and Stoll, H. (2011). The coupled δ13C-radiocarbon systematics of three Late Glacial/early Holocene speleothems; insights into soil and cave processes at climatic transitions. Geochimica Cosmochimica Acta 75 (15), 4321–4339. doi:10.1016/j.gca.2011.05.022
Tarasov, L., Dyke, A. S., Neal, R. M., and Peltier, W. R. (2012). A data-calibrated distribution of deglacial chronologies for the North American ice complex from glaciological modeling. Earth Planet. Sci. Lett. 315-316, 30–40. doi:10.1016/j.epsl.2011.09.010
Therre, S., 2020. Radiocarbon in stalagmites: Indicator of climate variability and key to atmospheric radiocarbon reconstruction. doi:10.11588/heidok.00028743
Keywords: stalagmite, paleoclimate, modeling, simulation, speleothem
Citation: Merz N, Hubig A, Kleinen T, Therre S, Kaufmann G and Frank N (2022) How the climate shapes stalagmites—A comparative study of model and speleothem at the Sofular Cave, Northern Turkey. Front. Earth Sci. 10:969211. doi: 10.3389/feart.2022.969211
Received: 14 June 2022; Accepted: 25 August 2022;
Published: 26 September 2022.
Edited by:
Chuan-Chou Shen, National Taiwan University, TaiwanReviewed by:
Nick Scroxton, Maynooth University, IrelandMadhusudan G. Yadava, Physical Research Laboratory, India
Mehmet Oruç Baykara, Pamukkale University, Turkey
Copyright © 2022 Merz, Hubig, Kleinen, Therre, Kaufmann and Frank. This is an open-access article distributed under the terms of the Creative Commons Attribution License (CC BY). The use, distribution or reproduction in other forums is permitted, provided the original author(s) and the copyright owner(s) are credited and that the original publication in this journal is cited, in accordance with accepted academic practice. No use, distribution or reproduction is permitted which does not comply with these terms.
*Correspondence: Niklas Merz, bmlrbGFzLm1lcnpAaXVwLnVuaS1oZWlkZWxiZXJnLmRl