- 1Center for Rock Instability and Seismicity Research, School of Resources and Civil Engineering, Northeastern University, Shenyang, China
- 2School of Mining Engineering, Anhui University of Science and Technology, Huainan, China
Landslides seriously threaten the safety of human life and property. In order to predict the on-set of potential landslides, this paper first characterizes the physical and mechanical parameters of rock mass on the basis of core rock quality designation identification, the geostatistics interpolation method, and the Hoek-Brown criterion. Then, the rock-mass physical and mechanical parameters characterization model was transformed to the slope numerical models, and the characteristics of potential landslides were predicted combined with the monitoring data and numerical simulation. Finally, on the basis of pairing between potential- and historical landslide cases, an early-warning indicator of a potential landslide was set as a posterior indicator of a historical landslide with the highest similarity. The results show that the potential landslide mode of the west and the southwest slope of the Wushan open-pit mine, the case study considered here, was a single-step wedge landslide and an integral-incline landslide. These two types of landslides were found to be most similar to those in the Anjialing open-pit mine and the Fushun West open-pit mine. Based on the posterior-warning indicator of the most similar landslide cases, the warning indicators of the west and southwest slope are set as 12.7 mm/d and 135.2 mm/d. The proposed method here provides a reference for the establishment of early-warning indicators for landslides in open-pit mines.
1 Introduction
In recent years, with the depletion of shallow resources, a large number of open-pit mines have begun to switch to deep-pit mining. The increase in the slope height of open-pit mines will undoubtedly increase the risk of landslides and the associated accidents. Therefore, it is of great practical significance to carry out research-based studies on developing warning methods that may assess the slides (landslides) of the slopes in open-pit mines to reduce the loss of personnel and property in the process of such mining activities.
Whilst considering the complexity of the properties of the rock and their respective geological environments, this warning on the failure of the rock slope that leads to landslides must be realized by combining monitoring such sites with the commonly used data-analysis and landslide-warning methods, such as the statistical induction method, the nonlinear theory method, and the artificial intelligence method.
The statistical-induction method assesses the future development trend of the slope based on the statistical law obtained from the historical landslide phenomenon. For example, Xu et al. (2011) proposed an improved displacement-time curve on the basis of the tangential angle of the slope, and used it to forecast slope failure time, and found that the rock landslides become irreversible once this angle exceeded 85. Loew et al. (2017) determined the early-warning threshold through the fitting of a creep-curve on the basis of recorded data on historical landslides in the Alps, and successfully predicted the onset of landslides in the same region in 2012. In addition, quite a few studies combined creep theory with numerical simulation to analyze and forecast the mechanical response behavior, and the time of the landslide and failure of the rock slope (Xu et al., 2014; Cui et al., 2021; Li et al., 2022.; Li et al., 2021a, b; He and Kusiak, 2017; Zhou et al., 2021).
With the development of system- and nonlinear-science, nonlinear theory was introduced to analyze landslide disasters, and many landslides forecast models were proposed based on the catastrophe-, chaos-, and the renormalization-group theory. Zhang et al. (2015) proposed an improved strain-energy catastrophe criterion by using the catastrophe theory, and concluded that the stability of the system under consideration could be determined by the catastrophe characteristic values after regularizing the potential function. Xue et al. (2013) established a deforming prediction model of chaotic neural network in the slope rock-mass, on which basis a high-accuracy prediction of slope deformation was developed. Due to the complexity of landslide evolution and the variability of external environment, however, it was still difficult to establish the nonlinear dynamic equation of the process that may have led to the formation of the landslide (Qin et al., 2006; Liu, 2013).
With the rapid development of artificial intelligence technology in recent years, its application has been conducted in early-warning systems related to landslides. For example, Niu (2020) took the lithology, the void ratio, the water content, the liquid index, the slope, and the slope-height factors of rock-soil contact zone in southern Shaanxi as inputs and established an early-warning safety model based on neural network. It was found that the prediction results of the model fit well with the actual results, and hence could be used to predict landslide-related geological disasters in the rock-soil contact zones in mountainous areas.
Creep model is suitable for medium and long-term forecasting, but its disadvantage is that it does not account for the trigger factors. The curve-fitting and the nonlinear-theory methods forecast the trend of future data according to the characteristic changes of the documented historical data, which are suitable for forecasting. For algorithms that may be considered intelligent or have a very good predictive power, not only a large number of training samples are needed, but also some input parameters should be determined according to the theory of rock mechanics, and the best possible experience(s) related to the engineering practices, which together may result in developing early-warning systems, that do not get severely compromised by gaps in theoretical calculations.
So far, a large number of landslide cases have occurred in open-pit mines, and it is important to fully analyze these cases. The engineering analogy method used in slope design is kind of a simple comparative analysis of cases, but there is still a lack of an in-depth analysis of such cases. Therefore, it may follow that landslide cases and engineering experience from such cases into knowledge, which may lead to development of models that have the capacity to predict the onset of landslides, is key to scientific and reasonable warning of landslides.
In this study, we have attempted to characterize the Wushan open-pit mine rock-mass mechanical parameters based on the RQD (rock quality designation) identification of the core of boreholes. Then, the potential landslide characteristics were predicted by numerical simulation. Based on the landslide case-matching, early-warning indicators of potential landslides in the Wushan open-pit Mine was established.
2 Project profile
The copper-molybdenum open-pit mine at Wushan is located at Xinbaerhu Right Banner, Inner Mongolia Autonomous Region, China. The mining area is 9.84 km2 in area, and the annual processing capacity of the mine is 2.475 million tons. The current height of the slope is more than 240 m, the overall slope angle is 43–45, and the step-slope angle is 65.
Under the influence of mining disturbance and rock-joints, the stability of the rock-mass has become very unstable, several local, failures of the slopes and landslides have occurred in the west and south slopes of the open-pit mine (Figure 1).
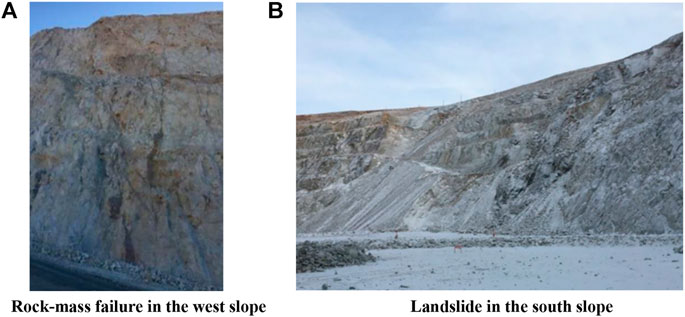
FIGURE 1. Rock mass failure and landslide in Wushan Open-pit Mine. (A) Rock-mass failure in the west slope; (B) Landslide in the south slope.
In order to monitor the stability of the west and south slope in real time, we established a ground-based radar (GBR), and its installation position is shown in Figure 2. The GBR is IBIS-Pover radar monitoring equipment produced by IDS GeoRadar.
3 Characterization of the rock-mass mechanical parameters
3.1 Identification and characterization and model-establishment of RQD
Cores of 160 geological survey boreholes (shown in Figure 2) with a total length of 64,000 m were photographed, and 9,605 core digital images were obtained. Automatic identification of RQD, on the basis of digital images of the core, were transformed into a deep-learning problem of case segmentation. Then the Mask R-CNN deep-learning network was used to identify the core segments with lengths greater than or equal to 10 cm from the core images (Liu et al., 2021), and calculate the RQD values of cores with different depths according to the following formula Eq. 1 and these formed the logging of the RQD borehole database. The RQD values of a few boreholes are shown in Figure 3.
where m is the number of core segments with a length ≥10 cm, li is the length of ith core segment with a length ≥10 cm, Lc is the total length of a single row of cores, ni is the number of pixels on the axis of ith core segment with a length ≥10 cm, Nc is the number of pixels on the axis of a single row of cores, and η is the core recovery rate, defined as the ratio of core length to the actual drilling footage, which is recorded during the construction of the drilling process.
The copper-molybdenum ore at Wushan is a hydrothermal-magmatic deposit, and the anisotropy of rock mass is not obvious; due to this, the anisotropy of the semivariogram of RQD was not considered. Since the exploration line spacing was 100 m, it was difficult to establish the omni-directional test semivariogram and, hence along the hole, a test semivariogram was used to characterize the spatial variability of RQD. The experimental and the semivariograms fitted by the spherical model are shown in Figure 4.
Based on the RQD borehole database, the RQD block model with uneven spatial distribution can be established by ordinary Kriging interpolation (Figure 5), in which the nugget value of the semivariogram was 5.5, the base value was 5.6, and the variation range was 250 m. The representative elementary volume scale of the jointed rock mass generally ranges between 7 and 10 m (Huang et al., 2020). Considering that the bench height of the Wushan open-pit mine is 15 m, the shape and scale of the block unit was set as cube and 15 m.
3.2 Establishment of rock mass mechanical parameter characterization model
According to Hoek et al. (2013), the relationship between the geological strength index (GSI) and RQD can be expressed as follows (Eq. 2):
where JCond89 is related to the grade conditions of the surface of the structural plane of the rock mass, which can be obtained by field observation of the outcrop joints of the rock mass and the quantitative method shown in Table 1.

TABLE 1. Definition of JCond89 after bieniawski (1989) and (Singh and Goel, 2011).
Based on the generalized Hoek-Brown criterion (Hoek and Brown, 2019), and GSI parameters, mb, s, α values, the mechanical parameters of rock mass can be obtained, which can all be expressed as in the following (Eqs 3–6):
where σ1 and σ3 are the major and minor principal stresses, respectively, σci is the uniaxial compressive strength of the intact rock that constitutes the rock mass, D is a factor which depends upon the degree of disturbance to which the rock mass has been subjected (for instance, during and after blast damage and stress relaxation), mi is a material constant for the intact rock that constitutes the rock mass.
The elastic modulus of rock mass can be calculated by Eq. 7:
If the Poisson’s ratio of the intact rock and the rock mass are assumed to be the same, then both the Lame constant and the bulk modulus can be obtained. The friction angle and the cohesion index of the rock mass can be obtained according to Equations 8-10:
where σ′3max is the upper-limit value of the limiting stress of the relationship between the Hoek-Brown criterion and the Mohr-Coulomb criterion, which is determined by Eq. 11:
where σ′cm, ρ, g, and H are the compressive strength of the rock mass, the density of rock mass, the acceleration of gravity, and height of the slope.
Combined with the actual engineering geological conditions and the mechanical parameters of the intact rock block (Table 2), RQD block model can be transformed into a rock-mass mechanical parameter block model (Figure 6) by Eqs 2–11. In addition, the block model can be directly transformed into a structured finite-element mesh for subsequent numerical simulations.
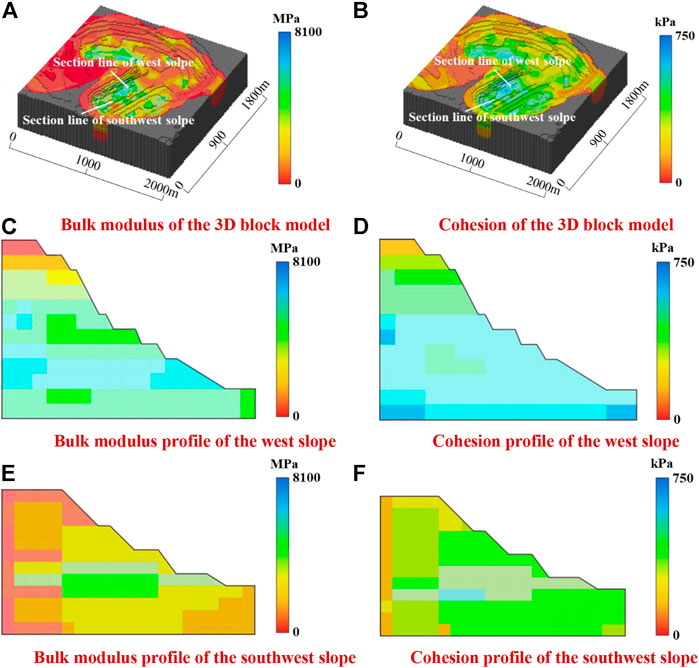
FIGURE 6. 3D block model of the rock-mass mechanical parameters. (A) Bulk modulus of the 3D block model; (B) Cohesion of the 3D block model; (C) Bulk modulus profile of the west slope; (D) Cohesion profile of the west slope; (E) Bulk modulus profile of the southwest slope; (F) Cohesion profile of the southwest slope.
As shown in Figures 6A,B, the bulk modulus and the cohesion of the rock mass in the open-pit mine at Wushan are distributed in the range of 0–8.1 GPa and 0–750 kPa, respectively, and the spatial distribution of the mechanical properties of the rock mass is very uneven. In general, the mechanical properties of the shallow parts are lower than those of the deeper parts. As shown in Figures 6C–F, the distribution of the mechanical properties inside the slope can be observed through the sections of the west and southwest slopes. Inside the west slope, the bulk modulus and the cohesion of the upper part is lower and increases with depth, while the same of the lower part is higher and also more random. Inside the southwest slope, the bulk modulus and the cohesion in the back side is lower than of the same in the front side.
4 Analysis of potential landslide characteristics combining monitoring and simulation
The 2D rock-mass mechanics parameters block models of the west and the southwest slopes was transformed into a structured 2D finite-element mesh, and the mesh was further refined for numerical simulations. Displacement constraints, perpendicular to the bottom and the side boundaries of the numerical model, were applied, respectively. The Mohr-Coulomb strength criterion was adopted in the numerical simulation.
Based on the slope displacement monitoring results by the ground-based radar, distance-power inverse-ratio method was used to calculate the displacement at each mesh-node of the numerical model, and then the displacement was added to each mesh-node as the initial displacement boundary condition. Using a method based on a reduction in strength, the potential landslide mode, the sliding surface angle, and the current safety coefficient were forecast (Figure 7), which provided the analogical factors and the constraint conditions for the establishment of landslide warning indicators.
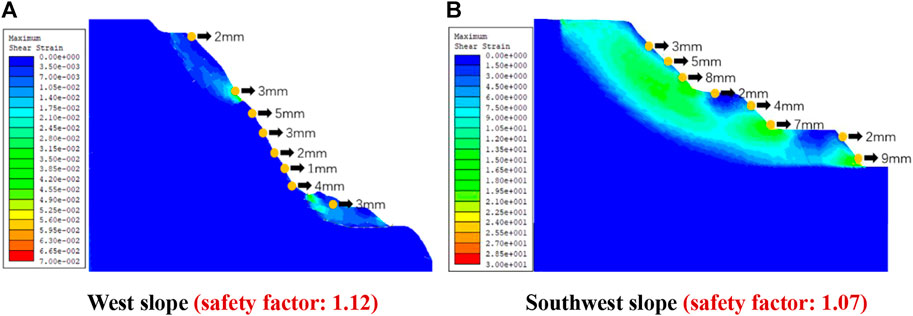
FIGURE 7. The potential landslide simulation. (A) West slope (safety factor: 1.12); (B) Southwest slope (safety factor: 1.07).
The depth of the sliding surface was determined by the average distance between each point on the sliding surface and its vertical upper slope. The simulation results show that single-step landslide with a 10 m depth-sliding surface may occur at the west slope (Figure 7A), and an overall landslide with more than a 60 m height slip-mass and more than a 15 m depth-sliding surface may occur at the southwest slope (Figure 7B). Combined with the historical landslide (Figure 1), and the simulation results, it may be inferred that the potential landslide mode of the west slope would be a single-step wedge landslide, whilst the potential landslide model of the southwest slope would be a large-scale incline landslide.
5 Construction of landslide warning indicators
5.1 The construction principle of landslide early-warning indicators based on case-matching
A total of 48 typical open pit landslide cases were collected and described quantitatively from three type features: mechanical mechanism, slope structure, and engineering geology (Table 3). The mechanical mechanism includes three secondary factors: landslide mode, angle of the sliding surface, and the condition of the sliding surface. The structure of the slope includes three secondary factors: the angle between the dominant structural plane and slope, rock-mass quality grade, and the slope angle. The engineering geology feature include three secondary factors: the thickness of the loose and weathered layer, the groundwater level, and the rainfall. Then, each landslide case can be represented as a score vector with nine features according to Table 3, Fi j = [fi1, fi2, fi3, fi4, fi5, fi6, fi7, fi8, fi9] and i = 1,2, … k, where i is the serial number of landslide cases in the database. The score vector, Gj, of a potential landslide (target landslide) of the open pit at Wushan can then be established.
Before case matching, the weight of each of the feature attribute was determined for the target landslide. The neighboring, comparative matching-method was used to determine the weight vector (Snyder, 2001). The weight-vector of the potential-wedge landslide in the west slope of the open-pit mine is [21.8%, 14.0%, 10.9%, 10.0%, 10.0%, 10.0%, 7.8%, 5.4%, 10.1%], and the integral-incline landslide in the southwest slope is [24.91%, 9.34%, 12.45%, 11%, 10%, 9%, 7.80%, 5.40%, 10.10%].
Each landslide case in the database was compared with the target landslide to find the most similar landslide cases. After getting the score vector, Fij, of each case in the database, and obtaining the score vector, Gj, and the weight vector, µ, of the target landslide, the matching vector, Di, was calculated (Eq. 12 as follows (Liu et al., 2021):
where i = 1,2, … k and j = 1,2, … 9.
The similarity, Si, between the target landslide and the slope landslide case was calculated as follows (Eq. 13):
The landslide case that is closest to the potential landslide of the open-pit mine can be obtained by a similar quantification. which can provide a basis for determining the warning threshold of a potential landslide at the mine at Wushan.
5.2 Construction of landslide early warning indicators of wushan open pit mine
Each index score for the west and the south slope is listed in Table 4. The type of the landslide and the sliding-surface angle were determined by simulations as described in Section 4. A least-square method was used to linearly fit the sliding surface, and the angle between the fitting line and the horizontal plane was the angle of the sliding surface. The sliding-surface condition and the structural-plane angle were obtained by field observations of the outcrop joints of the rock mass. The rock-mass quality was determined by Eq. 2 and the block model in Figure 5. The slope angle, the groundwater level, and the depth of the weathering zone were determined by the engineering-geological and the design data. The rainfall was determined by the on-site rain-gauge monitoring.
According to Table 4, the most similar cases with the potential landslide of the Wushan-type open-pit mine with the west and southwest slopes are Anjialing open-pit mine and Fushun-West open-pit mine, respectively; amongst the latter two open-pit mines, the similarity, with respect to Wushan open-pit mine, reached 77.39% and 80.90%, respectively. The deformation monitoring data of the surface of the slope of the whole landslide preparation and occurrence process in Anjialing open pit mine and Fushun West open pit mine are shown in Figure 8 (Nie et al., 2015). The maximum displacement rates of the surface of the slope surface prior to the landslide at Anjialing open-pit mine and Fushun-West open-pit mine reached 12.7 mm/day and 135.2 mm/day, respectively.
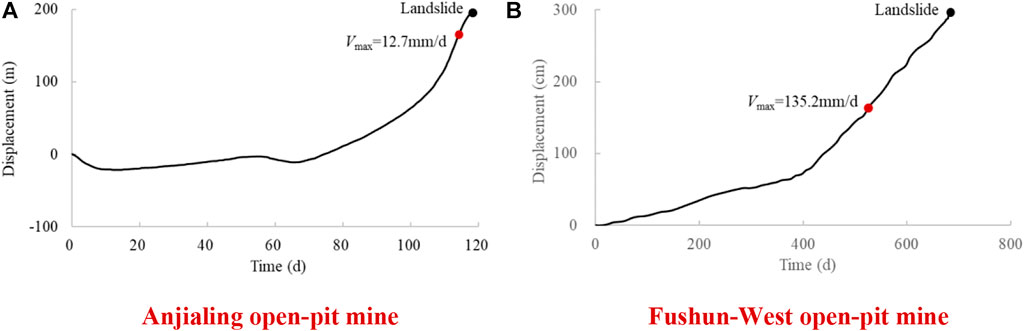
FIGURE 8. Displacement-time curves prior to the landslide at Wushan compared other similar landslide cases. (A) Anjialing open-pit mine; (B) Fushun-West open-pit mine.
The three-level landslide early-warning indicators was determined according to the principle of trisection, as shown in Table 5.
6 Conclusion
In this paper, the landslide warning indicators of Wushan Open-pit Mine were established combined with the characterization parameters of rock mechanics involving spatial distribution, numerical simulation, and matching of landslide cases. The main conclusions are as follows:
(1) The inhomogeneous distribution of the mechanical parameters of the rock mass were characterized based on core RQD automatic identification method and the ordinary Kriging interpolation and the Hoek-Brown criterion. Compared with the traditional method that assigns identical mechanical parameters to the same lithology in the study area, the characterization methods in this paper were found to be more reasonable.
(2) Using the monitoring data to modify the initial conditions of the slopes were found to be suitable to improve the reliability of the results obtained from numerical simulation results. The simulation results show that a local landslide with a 10 m depth sliding surface may occur at the west slope, and a large-scale landslide accompanied with a slip-mass may occur at the southwest slope when the height is more than 60 m.
(3) Based on the quantification of landslide features, the landslide early-warning indicator in this study was constructed by using similar cases from the landslide database. The displacement rates for the alarm level were found to be on the order of 12.7 mm/day and 135.2 mm/day for west slope and southwest slope, respectively. Finally, the methods outlined in this study may a provide a reference framework for the establishment of early-warning indicators of slope failures in other in open-pit mines.
Data availability statement
The raw data supporting the conclusion of this article will be made available by the authors, without undue reservation.
Author contributions
PZ contributed to the conception and design of the study. WD and FL made statistical analysis. PZ wrote the first draft of the manuscript. HW, XD and PN calculated and analyzed the data. All authors participated in the revision of the manuscript, read and approved the submitted version.
Acknowledgments
The work presented in this paper is financially supported by the National Natural Science Foundation of China (U1903216) and Fundamental Research Funds for the Central Universities of China (N2201015).
Conflict of interest
The authors declare that the research was conducted in the absence of any commercial or financial relationships that could be construed as a potential conflict of interest.
Publisher’s note
All claims expressed in this article are solely those of the authors and do not necessarily represent those of their affiliated organizations, or those of the publisher, the editors and the reviewers. Any product that may be evaluated in this article, or claim that may be made by its manufacturer, is not guaranteed or endorsed by the publisher.
References
Cui, S., Pei, X., Jiang, Y., Wang, G., Fan, X., Yang, Q., et al. (2021). Liquefaction within a bedding fault: Understanding the initiation and movement of the Daguangbao landslide triggered by the 2008 Wenchuan Earthquake (Ms= 8.0). Eng. Geol. 295, 106455. doi:10.1016/J.ENGGEO.2021.106455
He, Y., and Kusiak, A. (2017). Performance assessment of wind turbines: Data-derived quantitative metrics. IEEE Trans. Sustain. Energy 9 (1), 65–73. doi:10.1109/TSTE.2017.2715061
Hoek, E., and Brown, E. T. (2019). The Hoek–Brown failure criterion and GSI–2018 edition. J. Rock Mech. Geotechnical Eng. 11 (3), 445–463. doi:10.1016/j.jrmge.2018.08.001
Hoek, E., Carter, T. G., and Diederichs, M. S. (2013). “Quantification of the geological strength index chart,” in 47th US rock mechanics/geomechanics symposium. San Francisco, CA: OnePetro.
Huang, M., Liu, D., Hong, C., Du, S., Luo, Z., Li, C., et al. (2020). Representative sample sampling method for size effect experiment of jointed rock mass. Geofluids 2020 (10), 1–8. doi:10.1155/2020/8870387
Jixun, Z., Jiaqing, S., Haibo, Z., Xuhua, R., and Jiang, Q. (2015). Study on rock mass stability criterion based on catastrophe theory. Math. Problems Eng. 2015, 1–7. doi:10.1155/2015/850604
Li, H., Deng, J., Feng, P., Pu, C., Arachchige, D., and Cheng, Q. (2021a). Short-term nacelle orientation forecasting using bilinear transformation and ICEEMDAN framework. Front. Energy Res. 9, 780928. doi:10.3389/fenrg.2021.780928
Li, H., Deng, J., Yuan, S., Feng, P., and Arachchige, D. D. (2021b). Monitoring and identifying wind turbine generator bearing faults using deep belief network and EWMA control charts. Front. Energy Res. 770. doi:10.3389/fenrg.2021.799039
Li, H., He, Y., Xu, Q., Deng, J., Li, W., and Wei, Y. (2022). Detection and segmentation of loess landslides via satellite images: A two-phase framework. Landslides 19, 673–686. doi:10.1007/s10346-021-01789-0
Liu, H. D. (2013). Approach to forecasting occurrence of slope failure with nonlinear dynamical system. Appl. Mech. Mater. 438–439, 1597–1602. doi:10.4028/www.scientific
Fei-yue, L., Liu, Y., Zhang, P., Dong, X., and Zhang, H. (2021). Rock mass quality meticulous evaluation in mine engineering based on machine learning of core photo[J]. Chin. J. Geotechnical Eng. 43 (05), 968. doi:10.11779/CJGE202105023
Liu, F., Yang, Z., Deng, W., Yang, T., Zhou, J., Yu, Q., et al. (2021). Rock landslide early warning system combining slope stability analysis, two-stage monitoring, and case-based reasoning: A case study. Bull. Eng. Geol. Environ. 80 (11), 8433–8451. doi:10.1007/S10064-021-02461-6
Loew, S., Gschwind, S., Gischig, V., Keller-Signer, A., and Valenti, G. (2017). Monitoring and early warning of the 2012 Preonzo catastrophic rockslope failure. Landslides 14 (1), 141–154. doi:10.1007/s10346-016-0701-y
Nie, L., Li, Z., Zhang, M., and Xu, L. (2015). Deformation characteristics and mechanism of the landslide in west open-pit mine, fushun, China. Arab. J. Geosci. 8 (7), 4457–4468. doi:10.1007/s12517-014-1560-2
Niu, Hongtao (2020). Smart safety early warning model of landslide geological hazard based on BP neural network. Saf. Sci. 123, 104572. doi:10.1016/j.ssci.2019.104572
Qin, S. Q., Jiao, J. J., and Li, Z. G. (2006). Nonlinear evolutionary mechanisms of instability of plane-shear slope: Catastrophe, bifurcation, chaos and physical prediction. Rock Mech. Rock Eng. 39 (1), 59–76. doi:10.1007/s00603-005-0049-4
Singh, B., and Goel, R. K. (2011). Engineering rock mass classification. Boston: Butterworth-Heinemann, 45–142.
Snyder, R. (2001). Scaling down: The subnational comparative method. Stud. Comp. Int. Dev. 36 (1), 93–110. doi:10.1007/BF02687586
Xu, Q., Yuan, Y., Zeng, Y., and Hack, R. (2011). Some new pre-warning criteria for creep slope failure. Sci. China Technol. Sci. 54 (1), 210–220. doi:10.1007/s11431-011-4640-5
Xu, T., Xu, Q., Deng, M., Ma, T., Yang, T., and Tang, C. A. (2014). A numerical analysis of rock creep-induced slide: A case study from jiweishan mountain, China. Environ. Earth Sci. 72 (6), 2111–2128. doi:10.1007/s12665-014-3119-7
Xue, J., Li, X., and Liu, Z. (2013). Deformation laws of rock mass and safe alarm system of mine slope based on chaotic theory. J. Cent. South Univ. (Sci. Technol.) 44 (6), 2476–2481.
Zhang, J., Shu, J., Zhang, H., Ren, X., and Jiang, Q. (2015). Study on rock mass stability criterion based on catastrophe theory. Math. Prob. Eng. 8, 1–7.
Keywords: landslide warning, rock mechanics parameters, numerical simulation, case matching, open-pit mine
Citation: Zhang P, Deng W, Liu F, Wang H, Dong X and Niu P (2022) Establishment of landslide early-warning indicator using the combination of numerical simulations and case matching method in wushan open-pit mine. Front. Earth Sci. 10:960831. doi: 10.3389/feart.2022.960831
Received: 03 June 2022; Accepted: 18 July 2022;
Published: 07 September 2022.
Edited by:
Huajin Li, Chengdu University, ChinaReviewed by:
Baoxu Yan, Xi’an University of Science and Technology, ChinaChen Wang, Guizhou University, China
Copyright © 2022 Zhang, Deng, Liu, Wang, Dong and Niu. This is an open-access article distributed under the terms of the Creative Commons Attribution License (CC BY). The use, distribution or reproduction in other forums is permitted, provided the original author(s) and the copyright owner(s) are credited and that the original publication in this journal is cited, in accordance with accepted academic practice. No use, distribution or reproduction is permitted which does not comply with these terms.
*Correspondence: Wenxue Deng, dengwenxue@mail.neu.edu.cn