- National Meteorological Centre, Beijing, China
Tropical cyclones (TCs) can undergo offshore rapid intensification (RI) shortly before making landfall over the South China Sea (SCS). In this study, the inner rainbands distribution of both RI and non-RI landfall TCs (LTCs) in the SCS during 2015–2020 is examined based on a multi-source merged precipitation dataset. It is found that those RI LTCs exhibit a relatively higher averaged rain rate in the inner core region than that of non-RI LTCs. Both offshore RI cases and non-RI LTCs appear to have an increasing tendency of averaged rain rate after landfall, with the rain rate peak of the RI cases a few hours earlier than that of non-RI cases. By defining an axisymmetric index, the inner rainband evolution of both offshore RI cases and non-RI ones are further discussed. For both categories, most of the axisymmetric rainfall is concentric around the center and over 70% axisymmetric rainfall dominates the inner core region within three times of radius of maximum wind speed (RMW). It is shown that there is an obvious inwards shrinkage of axisymmetric rainfall for both offshore RI and non-RI cases. Analysis of typical RI and non-RI LTCs (1713 Hato and 1714 Pakhar) also shows an increasing rain rate of the inner rainbands soon after landfall, with larger amplitude for RI example than non-RI case. The inner rainbands of 1713 Hato show that a clockwise propagation with maximum enhancement happened at the down-shear left-hand side a few hours after landfall.
Introduction
Accompanied by gale winds, torrential rains, inland floods and mudslides in mountainous terrain, tropical cyclones (TCs) are one of the most devastating natural disasters in the world (Chen et al., 2010). The South China Sea (SCS) is affected by summer Monsoon, Madden-Julian Oscillation, and Subtropical High, and therefore TCs in this area can have more unpredictable tracks and intensity change (Liang et al., 2011; Molinari and Vollaro, 2012; Wu et al., 2013; Chen et al., 2015). Recently, many studies have shown that the onset of rapid intensification (RI) in the SCS occurred under favorable large-scale circulation, including weaker vertical wind shear (VWS), warmer ocean surface, and suitable low-level water vapor convergence (Leighton et al., 2018; Qiu et al., 2019; Cai et al., 2022; Zhao et al., 2022a). Both large-scale environment conditions and complex atmospheric interaction with land and ocean can induce asymmetric rainfall maximum distribution, especially during the landfall process. Forecasting and early warning of landfall TCs (LTCs) that undergo an offshore RI event before landfall is a considerable challenge. Recent LTCs such as Mujigae (1522), Mirinae (1603), Hato (1713), and Higos (2007) had all experienced RI shortly before landfall, which caused large forecasting errors and related disasters to the coastal area of the southern part of China. Consequently, offshore RI events and relevant heavy rainfall distribution are both crucial to disaster reduction.
Deep convection, in terms of rainbands within TCs, can be characterized by asymmetry affected by large-scale circulation (Corbosiero and Molinari, 2002; Chan et al., 2004; Lonfat et al., 2004; Chen et al., 2006; Cecil, 2007; Li and Dai, 2020). In addition to surface friction-induced low-level convergence caused by storm motion and uneven distribution of ocean thermal condition, environmental VWS in the surrounding areas of TC is a fundamental factor of asymmetric rainfall (Kaplan and Demaria, 2003; Kelvin and Johnny, 2011; Xu et al., 2014; Yu et al., 2015, 2017). The maximum convection in term of heavy rainfall occurs at the down-shear side and left-hand quadrant in the Northern Hemisphere, regardless of the storm’s motion (Jiang et al., 2008a; Yu et al., 2015). Actually, not all observed rain rate distribution can be exactly explained by the shear induced asymmetries. Other influential factors include the ocean’s thermal condition (Cecil, 2007, Jiang et al., 2008b), the surface land-sea contrast (Chen and Wu, 2016), and also interaction with other systems such as Monsoon Gyre (Qiu et al., 2020) or interaction between binary typhoon vortex (Xu et al., 2011) other large-scale atmospheric systems (Zhao et al., 2022b), and local topography (Liou et al., 2013).
During the landfall process, the rainfall distribution is very complicated when the development and collapse of the mesoscale circulation system of the inner TC circulation become dominant (Chen et al., 2004; Chen and Wu, 2016). Certain asymmetric rainfall or convective activities can be estimated through satellite image or radar rainfall estimation (Mueller et al., 2006; Reasor et al., 2013), or simulated by numerical models (Li and Wang, 2012). Recent studies have found that the size and structure change of TC inner rainbands is closely related to the rainfall distribution in landfall process (Dai et al., 2021; Yu et al., 2022). However, not enough observation analysis has been done, especially for the LTCs over the SCS. It is still unclear that whether the inner rainbands exhibit a similar structure change for both offshore RI LTCs and non-RI cases. Although the majority of previous studies have focused on the external factors caused by the large-scale environment, it is important to know the internal dynamical mechanism and mesoscale process to trigger the asymmetric distribution of rainfall during the landfall process. This study has used a newly developed multiple-source merged precipitation dataset to figure out the differences of inner rainbands between RI and non-RI LTCs during their landfall processes over the SCS.
Data and method
Data
The TC track that is used in this study is the best track dataset from the Shanghai Typhoon Institute (STI) of China Meteorological Administration (CMA). Using the maximum wind speed and minimum central sea level pressure to define the intensity, and the latitude and longitude to define the location, the STI best track provides TC information in the western North Pacific and SCS in 6h intervals (Ying et al., 2014). For offshore TCs (normally within 200 km from the coastal line), the STI best track dataset can additionally increase the time interval to 3 h because weather radar and coastal weather stations can capture their detailed structure. These multiple observations could be additionally beneficial to estimate the intensity and location of an offshore TC than other best track datasets in this region. To figure out the timely revolution of rainbands during the landfall process, linear interpolation was used to interpolate the every 6 h/3 h STI best track dataset to 1 h to specify the location migration during the landfall process. However, the size of TC cannot be directly obtained from STI best track dataset, and therefore we used the analysis from multi-platform tropical cyclone surface wind analysis (MTCSWA) from NOAA/NESDIS, in which the radius of maximum wind speed (RMW) is captured from satellite retrieved surface winds (Knaff et al., 2007). To specify the influence of VWS for the evolution of inner rain bands, ERA5 reanalysis from the European Centre for Medium-Range Weather Forecasts (ECMWF) is used to examining the large-scale conditions and circulation.
Given that satellite based rainfall estimation has a wide coverage and continuous monitoring, most previous research of TC rainfall characteristics have been based on satellite estimation datasets, such as the tropical rainfall measuring mission (TRMM; Chen and Wu et al., 2016; Yu et al., 2015; Yu et al., 2017), global precipitation measurement (GPM; Hou et al., 2014), or integrated multi-satellite retrievals of GPM (IMERG; Huffman et al., 2020; Guzman and Jiang, 2021). For TC overland, the precipitation retrieved from radar can also be used to estimate the TC rainfall in certain cases (Chan et al., 2004). Because few previous studies have used an in-situ rain gauge from an automatic weather station (AWS), we chose to use the CMA multi-source merged precipitation analysis system (CMPAS), which has includes more than 40,000 AWSs. Meanwhile, CMAPS has also included the satellite retrieved rainfall from climate precipitation centre morphing (CMORH) and radar estimation. Other than a single source of observation, the CMPAS merged three sources of precipitation observation with different temporal and spatial resolutions using Bayesian multi-model averaging and optimal interpolation. Independent verification has confirmed that the estimation root square mean errors of CMPAS are lower than those of a single source of estimation, such as CMOPH and radar retrievals (Shen et al. 2014). By combining geostationary satellite, radar observations, and especially rain gauge observation (including inland, offshore, and on-islands), this multiple-source fusion data could give a full picture of rainfall structure during their landfall process considering equivalent merging technique over land and sea.
To discuss the inner rainbands distribution of LTCs during the landfall process, three preliminary conditions were defined according to the research purpose. First, only the first landfall event for each LTC is included. In this way, those LTCs that made landfall on Hainan Island and then followed the second or third landfall on the mainland after passing over the ocean’s surface are excluded. Second, selected LTCs should maintain at least 12 h after making landfall on mainland and 6 h after making landfall over Hainan Island to ensure a sufficient analyzed period for the temporal and spatial evolution of the symmetric and asymmetric structure of rainfall. From the 24 h before landfall to the 12 h after landfall, all selected LTCs were captured in the same periods of time to examine the landfall process. Because the CMAPS dataset starts from 2015 with the domain of 5–60°N, 70–140°E, 10 LTCs from 2015 to 2020 over SCS were finally selected according to the previous two conditions. Detailed information of these LTCs will be given in third section.
Method
The asymmetric and symmetric components of precipitation are decomposed to study the relationship of asymmetric convection and other large-scale environmental factors (e.g., VWS) in previous studies (Lonfat et al., 2004; Uhlhorn et al., 2014; Yu et al., 2017). We used the same method to decompose precipitation distribution during a TC’s landfall process into axisymmetric and asymmetric components, as follows:
in which
Following the idea of defining the asymmetric convection in Miyamoto and Takemi (2003), we specified an axisymmetric index as a ratio of the axisymmetric precipitation to both axisymmetric and asymmetric components. Because the asymmetric component has both a positive and negative value, they were both squared, as follows:
where
Evolution of rain rate during the landfall process
Based on the criterion listed in the last section, 10 offshore LTCs in the SCS were selected during the period of 2015–2020 (see Table 1). Six of them made landfall on Guangdong Province and the other four made landfall on Hainan Island. The averaged landfall intensity is 36.9 m s−1, with a maximum of 48 m s−1 (Mujigae, 1522) and a minimum of 23 m s−1 (Son-Tinh,1809). The earliest landfall time of those 10 LTCs is in June (Kujira, 1508) and the last one is in October (Sarika, 1621).
In previous studies, the 95th percentile of all the samples of 24h intensity change of maximum sustained wind speed for TCs was normally settled as the threshold to define RI (Kaplan and DeMaria, 2003). We used the threshold of 15 m s−1 in 24h to define a RI event in the Northwestern Pacific basin followed previous works (Kaplan et al., 2010; Knaff et al., 2018). Second, to investigate the offshore intensity change before landfall over SCS, only the RI events that happened within 200 km from coastal line were defined as offshore RIs (i.e., those LTCs that experienced RI and landfall process at the same time when they are approaching the coastal line). The track segments of these LTCs during the landfall process, from 24h before landfall to 12 h after landfall, are given in Figure 1. Among these, four LTCs—Mujigae (1522), Mirinae (1603), Hato (1713) and Higos (2007)—had experienced offshore RI before landfall. Among these four offshore RI cases, three had an increase of 20 m s−1 during 24 h and Mirinae (1603) had 15 m s−1, respectively. The other six non-RI LTCs (presented in blue in Figure 1) had a more moderate intensity change during the landfall process. The landfall point spread from the east coast of Pearl River Estuary to the east of Hainan Island, covering the majority landfall places in this region. Further examination indicates that only Kujira (1508) was generated locally in the middle of SCS, while the others moved north-westward from the east of the Philippines.
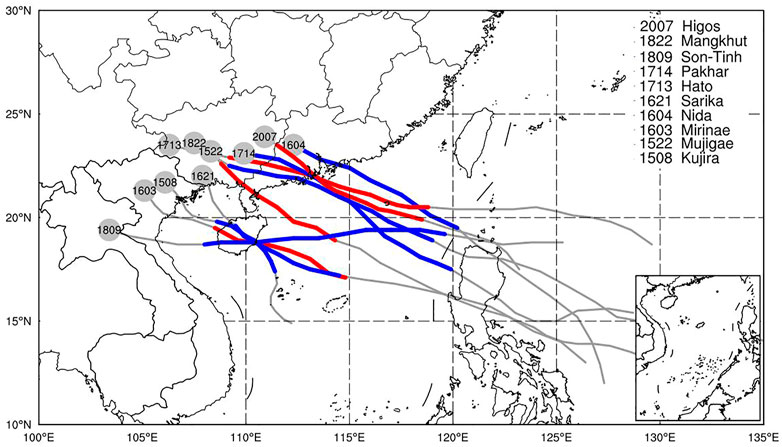
FIGURE 1. Track segments of 10 LTCs in SCS. The red (blue) lines represent RI (non-RI) in the period from 24 h before to 12 h after the landfall, respectively (the number and name of the LTCs are listed).
With a fully developed structure, over 80% of deep convection in TC is enveloped inside the inner core regions (Miyamoto and Nolan, 2018), which is normally defined as three times of RMW (Wang, 2009). The spiral rainbands could be concentrated within inner core regions, where the inner rainbands and distant rainbands could overlap or coincide to intensify the rain rate within inner core regions. For RI TCs, the convective bursts (CBs) within the inner core region could trigger the rainfall increase (Chen et al., 2004; Reasor et al., 2013). To figure out the rain rate distribution within the inner core region for LTCs in the SCS during the landfall process, we first composited the radial rain rate of the RI and non-RI LTCs to present rainfall evolution. Recent studies have investigated the possible relationship between the TC size change and the radial distribution of rainfall, and found that a large amount of rainfall occurs in low inertial stability regions when intense convection happened (Tsuji and Nakajima, 2019). To avoid the possible influence of rainfall distribution from TC size, we normalized the rain rate from distance to TC center to multiples of RMW, which is closely related to the TC eyewall.
As shown in Figure 2, the inner core averaged rain rate of RI LTCs is obviously higher than that of non-RIs during the entire landfall process. In agreement with other observation analyzes, there is an obvious inner core CB in the RI cases (Reasor et al., 2013). Given that offshore RI LTCs actually have higher landfall intensity (39.0 m s−1 on average) compared to that of non-RIs (35.5 m s−1 on average), the averaged rain rate within inner core region indeed depends on the landfall intensity. It is also noticed that both RI LTCs and non-RI cases experienced an outbreak of rain rate during the first 6h after landfall, when the peak rain rate shows up at the 2h after landfall for RI cases and around 4h for non-RI cases. This obvious intensification of rain rate within inner core region nearly doubled the rain rate (with a maximum rain rate over 10 mm/h) just a few hours after landfall, which might be related to the outbreaks of deep convection in the inner core regions after the landfall for both RI and non-RI LTCs. In the following 6–12 h after landfall, the rain rate of both the RI and non-RI cases decreased sharply to less than 5 mm/h. During that period, the difference between the two categories narrowed because the LTCs’ circulation were fully over land or island after the outbreak of rain rate within the first 6 h after landfall. The F-test is used to exam the statistical significance for the difference between RI and non-RI case during the landfall process. The yield results show that the difference of those two categories has passed 95% confidence level for the whole process.
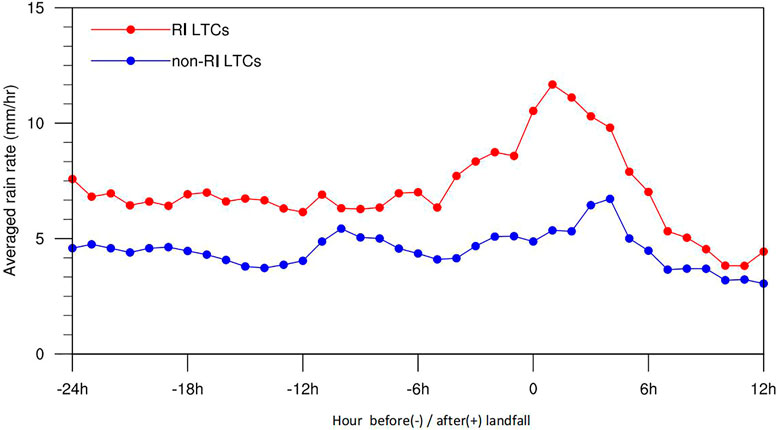
FIGURE 2. Comparison of averaged rain rate distribution within three RMW of four RI LTCs (indicated in red marked line) and six non-RI LTCs (indicated in blue) during the landfall process. The horizontal coordinate is the time before/after landfall.
Distribution of inner rainbands during the landfall process
We used an axisymmetric index defined in Section 2 to present the radial concentration of rainfall during the landfall process. The differences in axisymmetric evolution of rainfall between RI LTCs and non-RI LTCs are further examined as shown in Figure 3. The statistical significance of over 95% for the radial distribution is marked in shadows.
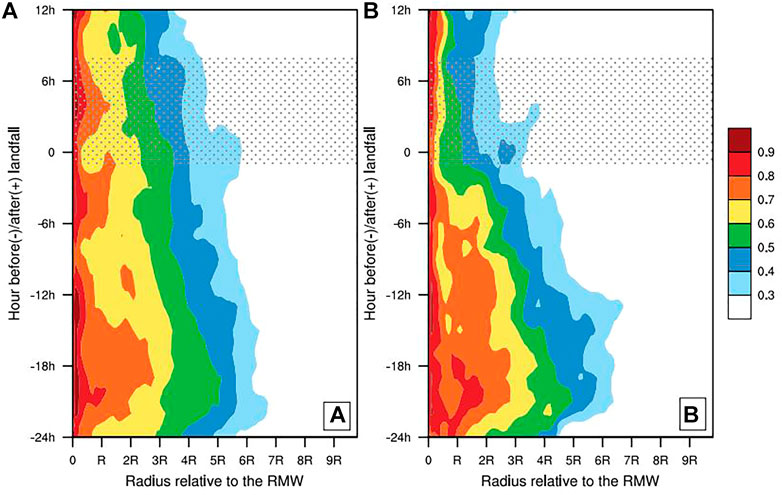
FIGURE 3. Composited radial-time distribution of asymmetric index of precipitation for (A) RI LTCs and (B) non-RI LTCs; the vertical coordinate is the hours before/after landfall and the horizontal coordinate is the multiple relative to RMW; area of significance over 95% is marked.
To avoid the possible influence of a TC’s size on its radial structure, the radial distance to TC center is at first converted into its relevant multiples to RMW. For both RI and non-RI LTCs, the axisymmetric rainfall shows a tendency of inwards concentration during the landfall process, with over 70% axisymmetric rainfall dominating the inner core region (here referred to 3RMW) 1 day before landfall. However, as the TC’s center approaches the coast line in the following hours, the inner core region is gradually dominated by asymmetric rainfall other than axisymmetric rainfall because only less than 50% total rainfall could be decomposed to azimuthal mean rainfall after landfall. This obvious inwards shrinkage of axisymmetric rainfall could also be found in the non-RI cases, while the differences appeared after the landfall. Compared to the steady asymmetric process, the non-RI LTCs show up the sharp collapse of axisymmetric rainfall soon after landfall, which means the asymmetric components of rainfall are eventually dominant outside the RMW for non-RI LTCs. It is found that the difference of axisymmetric rainfall after landfall for those two categories is of statistical significance when compared to the period before landfall.
To better distinguish the evolution of the inner rainbands’ evolution between RI LTCs and non-RIs, two typical LTCs 1713 Hato and 1714 Pakhar were selected to represent RI and non-RI cases respectively. Generated in August 2017, Hato and Pakhar successively made landfall over the western part of Guangdong Province. Other than similar prevailing tracks before landfall, the tracks after landfall also bear little difference. Given that 1713 Hato experienced RI shortly before landfall, the landfall intensity of 1713 Hato was higher than that of 1714 Pakhar. The Empirical Orthogonal Functions (EOF) analysis was used to simplify a spatial-temporal data set by transforming the rainfall to spatial patterns of variability and temporal projections of these patterns.
As in Figure 4A the radial coordinate is the radius relative to RMW, while only the inner rainbands distribution within 3R from the center was presented. From the typical EOF analysis, the first EOF pattern contributed over 40% variation of inner rainbands in the case of 1713 Hato. The majority of positive rainfall was concentric around the center within RMW, which means the inner rainbands were intensified during the landfall process, especially within the eyewall. The amplitude of EOF shows the time evolution of this pattern. As shown in Figure 4B, the inner core rainbands intensification exhibited an increase soon after its landfall, which means that the inner core rain rate actually double compared to the periods before landfall. The EOF analysis of a single example 1713 Hato has confirmed the previous composite analysis of inner core rainfall shown in Figure 2. We also examined the other three RI LTCs, 1522 Mujigae, 1603 Mirinae, and 2007 Higos using the same method. Although the first EOF pattern turned out to be distributed differently, the temporal series of amplitude for those patterns has all exhibited the same tendency of increasing after landfall.
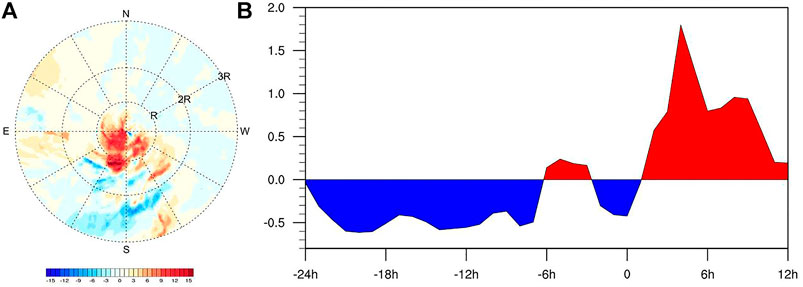
FIGURE 4. The first EOF pattern (A) (units: mm) and amplitude (B) of rainfall during the landfall process of 1713 Hato, R indicates the RMW. The horizontal coordinate is the time before/after landfall.
The spatial and temporal distribution of inner rainbands of 1714 Pakhar, which made landfall to the west of the Pearl River Estuary around 4 days after 1713 Hato, are given in Figure 5. The first EOF pattern of 1714 Pakhar has shown as a pattern of spiral rainbands, which starts from the southeast quadrant outside RMW and rotates anticlockwise into the TC center. Although the maximum value in the first EOF pattern of 1714 Pakhar was lower than that of 1713 Hato, this enhancement of inner rainbands has also exhibited an increase after landfall. Compared to the RI cases, this increase of inner rainbands did not last as long as 1713 Hato, and another decreasing tendency of inner rainbands was dominant only 6 h after landfall. Even though the EOF analysis of other non-RI LTCs presented in different spatial patterns, a similar increasing tendency of rainfall could be shown.
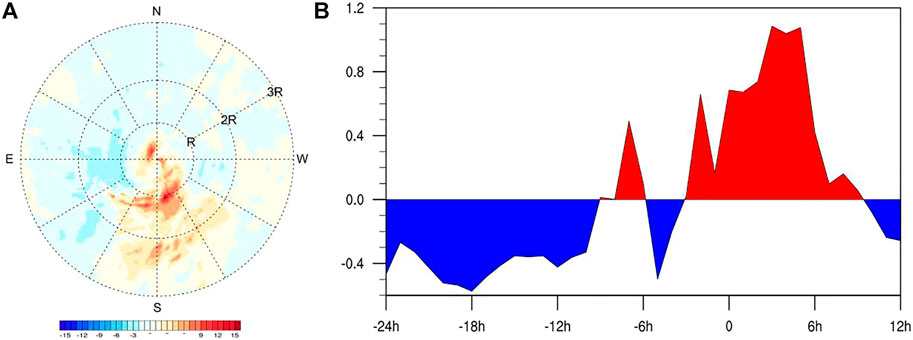
FIGURE 5. Same as in Figure 4, but for 1714 Pakhar.
As discussed in previous studies (Kaplan and Demaria, 2003; Kelvin and Johnny, 2011; Xu et al., 2014; Yu et al., 2015, 2017), environmental VWS is related to the asymmetric distribution of rainfall. The maximum rainfall often exhibit over the left-hand side along the direction of shear (Chen et al., 2006; Shimada et al., 2017; Yu et al., 2017). To figure out the inner rainbands’ evolution during the landfall process under different environmental VWS, the azimuthal-time distribution of inner rainbands of both 1713 Hato and 1714 Pakhar are given in Figure 6.
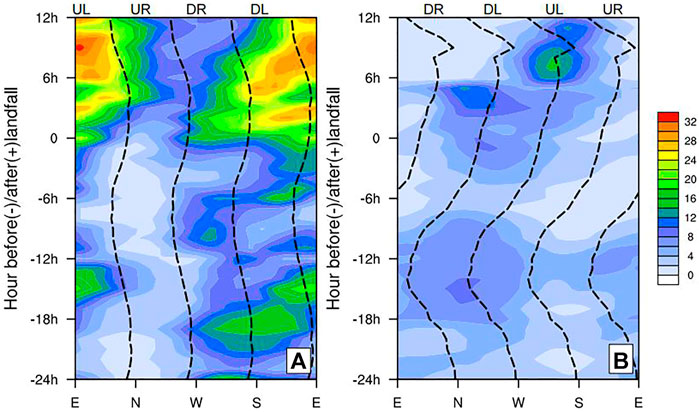
FIGURE 6. Azimuthal-time distribution of inner rain bands (units: mm) for (A) 1713 Hato and (B) 1714 Pakhar. The vertical coordinate is the hours before/after landfall and the horizontal coordinate is azimuth angle. Dashed lines indicate VWS; UL/UR represent up-shear left/right and DL/DR denote down-shear left/right.
As shown in Figure 6A, the rain rate of inner rainbands was averaged within the domain of three RMW from the TC center. The inner rainbands of 1713 Hato have shown up an obvious clockwise propagation during the landfall process. Meanwhile, the averaged rain rate within 3R intensified after landfall, which confirmed the previous analysis (as shown in Figure 3). The maximum rain rate doubled during the post-landfall period compared to before landfall. It is also noticed that the inner rain bands presented an obvious clockwise propagation, during which the spiral rain bands started from the Down-shear Right (DR) side and intensified as the Down-shear Left (DL). This kind propagation of spiral rainbands has also been discussed in numerical simulation (Li and Wang, 2012; Li and Dai, 2020). In the case of 1713 Hato, the inner rain bands intensified at the southern part after landfall, which might be related to the rainfall enhancement over the coastal line. As the non-RI case, 1714 Pakhar presented a much moderate intensification of inner rainbands after landfall (shown in Figure 6B). The maximum rain rate of inner rainbands could be found at DL side of TC at nearly 6 h after landfall. Given that the direction of VWS experienced a much wider change compared to that of 1713 Hato, the phenomenon of clock-wised propagation of inner rainbands was less obvious in this case. The maximum rain rate can be found in the southern semicircle after landfall, which is also related to the topographic influence.
Conclusion and discussion
The northern part of the SCS is one of most prevalent areas for offshore RIs. Therefore, the inner rainband distribution of both offshore RIs and non-RI TCs was examined from 24 h before to 12 h after landfall during 2015–2020. The relationship between TC inner rainbands and previous intensity change before landfall was studied in this work based on a multiple merged dataset CMPAS. Finally, 10 LTCs were selected to be discussed. All LTC samples were classified into two groups according to the definition of offshore RI events. It is found that the offshore RI LTCs exhibit a relatively higher than average rain rate in the inner core region compared to the non-RI LTCs. Due to possible impact from land-sea contrast and topography, the averaged inner rain rates for both offshore RI and non-RI cases appear to have a tendency to increase after landfall. Even though both composited temporal evolution of inner core rain rates have a peak within 6 h of landfall, the composited RI LTCs have a peak value a few hours earlier than that of non-RI cases. Because most of the offshore RI LTCs have indeed higher landfall intensities than those non-RI counterparts, stronger LTCs might have higher rain rate during their landfall process than weaker ones. From previous studies, it is known that the large-scale VWS, which favors the enhancement of TC intensity, might also an important environmental factor for the development of deep convection (Yu et al., 2017; Tsuji and Nakajima, 2019). The offshore RI LTCs have more favorable background conditions for the growth of both intensity and axisymmetric rainfall.
By defining an axisymmetric index, the axisymmetric rain rate evolution of both offshore RI and non-RI cases are further discussed. RMW is an important parameter in determining the inner core region. Before compositing the RI LTCs and non-RI cases, the radius change throughout the landfall process was carefully analyzed to avoid mismatching the size difference and their rainfall distributions. For both of the two composited azimuthal axisymmetric indices, most of axisymmetric rainfall is concentric around the center, with over 70% of axisymmetric rainfalls dominating the inner core region within 3RMW. When the land-sea contrast and topography have a gradual impact on the vertical structure of the LTCs, the inner core region with higher percentage of axisymmetric rainfall before landfall has lower azimuthal mean rainfall after landfall. It is noted that there is an obvious inwards shrinkage of axisymmetric rainfall for both offshore RI and non-RI cases, while the differences only appeared after landfall. The non-RI LTCs have a sharper collapse of axisymmetric rainfall soon after landfall, which means that for non-RI LTCs the asymmetric components of rainfall eventually dominate the distribution outside the RMW. This could explain why weaker LTCs that have experienced weakening before landfall will have much more unpredictable rainfall distributions than RI cases. In 1508 Kujira and 1809 Son-Tinh, the inner rainbands hardly disappeared after landfall. However, the distance rainbands caused by the Monsoon surge might trigger robust heavy rainfall inland. It is therefore important to further research the structure evolution and rainfall distribution during the landfall process for weaker or already weakened LTCs.
Two typical LTCs (1713 Hato and 1714 Pakhar) were selected to study the primary patterns of inner rainbands and its related temporal evolution using EOF analysis. For the RI case, 1713 Hato, the inner core rainband intensification exhibited an increase soon after landfall, while the inner core rain rate was nearly double that of the periods before landfall. The weaker LTC 1714 Pakhar shows a pattern of spiral rainbands, but its increase of inner rainbands lasted only 6h after landfall. Similar to previous numerical studies, the inner rainbands presented a clockwise propagation, and maximum intensification happened at the DL side in 1713 Hato. The enhancement of inner rainbands was observed at the southern part of TC after landfall for both two typical LTCs. This could be closely related to convective convergence and lifting along coastal line, which triggered the enhancement of inner rainbands a few hours after landfall.
It is suggested that the obvious outbreaks of rain rate can be related to land-sea contrast in most LTCs. More precise studies of the inner core structure during the landfall process might explain the dynamic mechanism other than rainfall distribution. In this article, we only discussed the relationship between previous intensity change and rainfall distribution of LTCs based on observations. The inner dynamic mechanism to explain the increase of inner rainbands within 6 h after landfall is hardly known. Numerical simulation or three-dimensional vertical structure analysis from other high-resolution observations (e.g., dual polarization radar) might help to answer the possible influence of topography and land-sea contrast, particularly during the landfall process.
Data availability statement
The datasets presented in this study can be found in online repositories. The names of the repository/repositories and accession number(s) can be found below: http://idata.cma/idata/web/data/index?dataCode=NAFP_NMIC_ART_CMPAS_0P05_CHN_DAY_PRE_RT
Author contributions
CX and YX contributed to main research part and design of the study. CX and SL organized the database. CX performed the statistical analysis and organization of manuscripts. LW provided suggestion and revision of this work.
Funding
This work is supported by The National Key Research and Development Program of China (2017YFC1501604).
Conflict of interest
The authors declare that the research was conducted in the absence of any commercial or financial relationships that could be construed as a potential conflict of interest.
Publisher’s note
All claims expressed in this article are solely those of the authors and do not necessarily represent those of their affiliated organizations, or those of the publisher, the editors and the reviewers. Any product that may be evaluated in this article, or claim that may be made by its manufacturer, is not guaranteed or endorsed by the publisher.
References
Cai, Y., Han, X., Zhao, H., and Klotzbach, P. J. (2022). Enhanced predictability of rapidly intensifying tropical cyclones over the western North Pacific associated with snow depth changes over the Tibetan Plateau. J. Clim. 35, 2093–2110.
Cecil, D. J. (2007). Satellite-derived rain rates in vertically sheared tropical cyclones. Geophys. Res. Lett. 34 (2), L02811. doi:10.1029/2006gl027942
Chan, J. C.-L., Liu, K. S., Ching, S. E., and Lai, E. S. T. (2004). Asymmetric distribution of convection associated with tropical cyclones making landfall along the south China coast. Mon. Weather Rev. 132 (10), 2410–2420. doi:10.1175/1520-0493(2004)132<2410:adocaw>2.0.co;2
Chen, H. D.-L., Zhang, J. Carton, and Atlas, R. (2011). On the Rapid intensification of hurricane wilma (2005). Part I: Model prediction and structural changes. Weather Forecast. 26, 885–901. doi:10.1175/waf-d-11-00001.1
Chen, L., Li, Y., and Cheng, Z. (2010). An overview of research and forecasting on rainfall associated with landfalling tropical cyclones. Adv. Atmos. Sci. 27 (5), 967–976. doi:10.1007/s00376-010-8171-y
Chen, L., Luo, Z., and Li, Y. (2004). Research advances on tropical cyclone landfall process. Acta. Meteor. Sin. 62, 541–549.
Chen, S. S., Knaff, J. A., and Marks, F. D., 2006: Effects of vertical wind shear and storm motion on tropical cyclone rainfall asymmetries deduced from TRMM. Monthly weather review, 134, 3190–3208, doi:10.1175/1520-0469(2003)060<2294:asiasl>2.0.co;2
Chen, X., and Wu, L. (2016). Topographic influence on the motion of tropical cyclones landfalling on the coast of China. Weather Forecast. 31 (5), 1615–1623. doi:10.1175/waf-d-16-0053.1
Chen, X., Wang, Y., and Zhao, K. (2015). Synoptic flow patterns and large-scale characteristics associated with rapidly intensifying tropical cyclones in the south China sea. Mon. Weather Rev. 143, 64–87. doi:10.1175/mwr-d-13-00338.1
Chen, Y., and Yau, M. K. (2003). Asymmetric structures in a simulated landfalling hurricane. J. Atmos. Sci. 60 (18), 2294–2312.
Corbosiero, K. L., and Molinari, J. (2002). The effects of vertical wind shear on the distribution of convection in tropical cyclones. Mon. Weather Rev. 130 (8), 2110–2123. doi:10.1175/1520-0493(2002)130<2110:teovws>2.0.co;2
Dai, H., Zhao, K., Li, Q., Lee, W. C., Ming, J., Zhou, A., et al. (2021). Quasi-periodic intensification of convective asymmetries in the outer eyewall of typhoon lekima(2019). Geophys. Res. Lett. 48. doi:10.1029/2020gl091633
Frank, W. W., and Ritchie, E. A. (2001). Effects of vertical wind shear on the intensity and structure of numerically simulated hurricanes. Mon. Weather Rev. 129, 2249–2269. doi:10.1175/1520-0493(2001)129<2249:eovwso>2.0.co;2
Guzman, O., and Jiang, H. (2021). Global increase in tropical cyclone rain rate. Nat. Commun. 12, 5344. doi:10.1038/s41467-021-25685-2
Hou, A. Y., Kakar, R. K., Neeck, S., Azarbarzin, A. A., Kummerow, C. D., Kojima, M., et al. (2014). The global precipitation measurement mission. Bull. Am. Meteorol. Soc. 95, 701–722. doi:10.1175/bams-d-13-00164.1
Huffman, G. J., Adler, R. F., Rudolf, B., Schneider, U., Keehn, P. R., Gu, G., et al. (2007). The TRMM multisatellite precipitation analysis (TMPA): Quasi-global, multiyear, combined sensor precipitation estimates at fine scales. J. Hydrometeorol. 8, 38–55. doi:10.1175/jhm560.1
Huffman, G. J., Bolvin, D. T., Nelkin, E. J., and Tan, J. (2020). Integrated multi-satellite retrievals for GPM (IMERG) technical documentation, 83.
Jiang, H., Halverson, J. B., and Simpson, J. (2008a). 2008a: On the differences in storm rainfall from hurricanes isidore and lili. Part i: Satellite observations and rain potential. Weather Forecast. 23 (1), 29–43. doi:10.1175/2007waf2005096.1
Jiang, H., Halverson, J. B., and Zipser, E. J. (2008b). 2008b: Influence of environmental moisture on TRMM-derived tropical cyclone precipitation over land and ocean. Geophys. Res. Lett. 35 (17), L17806. doi:10.1029/2008gl034658
Kaplan, J., and DeMaria, M. (2003). Large-scale characteristics of rapidly intensifying tropical cyclones in the North Atlantic basin. Weather Forecast. 18, 1093–1108. doi:10.1175/1520-0434(2003)018<1093:lcorit>2.0.co;2
Kaplan, J., DeMaria, M., and Knaff, J. A. (2010). A revised tropical cyclone rapid intensification index for the Atlantic and eastern North Pacific basins. Wea. Forecast. 25, 220–241. doi:10.1175/2009WAF2222280.1
Kelvin, T. F. C., and Johnny, C. L. C. (2011). Size and strength of tropical cyclones as inferred from QuikSCAT data. Mon. Weather Rev. 140, 811–824. doi:10.1175/mwr-d-10-05062.1
Knaff, J., Sampson, C., DeMaria, M., Marchok, T., Gross, J., and Mcdie, C. (2007). Statistical tropical cyclone wind radii prediction using climatology and persistence. Weather Forecast. 22 (4), 781–791. doi:10.1175/waf1026.1
Knaff, J. A., Sampson, C. R., and Musgrave, K. D. (2018). An operational rapid intensification prediction aid for the western North Pacific. Wea. Forecast. 33, 799–811. doi:10.1175/WAF-D-18-0012.1
Leighton, H., Gopalakrishnan, S., Zhang, J. A., Rogers, R. F., Zhang, Z., and Tallapragada, V. (2018). Azimuthal distribution of deep convection, environmental factors, and tropical cyclone Rapid intensification: A perspective from HWRF ensemble forecasts of hurricane Edouard (2014). J. Atmos. Sci. 75, 275–295. doi:10.1175/jas-d-17-0171.1
Li, Q., and Dai, Y. (2020). Revisiting azimuthally asymmetric moist instability in the outer core of sheared tropical cyclones. Mon. Weather Rev. 148, 1297–1319. doi:10.1175/mwr-d-19-0004.1
Li, Q., and Wang, Y. (2012). A comparison of inner and outer spiral rainbands in a numerically simulated tropical cyclone. Mon. Weather Rev. 140 (9), 2782–2805. doi:10.1175/mwr-d-11-00237.1
Liang, J., Wu, L., Ge, X., and Wu, C.-C. (2011). Monsoonal influence on typhoon Morakot (2009). Part II: Numerical study. J. Atmos. Sci. 68, 2222–2235. doi:10.1175/2011jas3731.1
Liou, Y. C., Wang, T. C. C., Tsai, Y. C., Tang, Y. S., Lin, P. L., and Lee, Y. A. (2013). Structure of precipitating systems over Taiwan’s complex terrain during Typhoon Morakot (2009) as revealed by weather radar and rain gauge observations. J. Hydrol. Atmosphere 506, 14–25.
Lonfat, M., Marks, F. D., and Chen, S. S. (2004). Precipitation distribution in tropical cyclones using the tropical rainfall measuring mission (TRMM) microwave imager: A global perspective. Mon. Weather Rev. 132, 1645–1660. doi:10.1175/1520-0493(2004)132<1645:pditcu>2.0.co;2
Miyamoto, Y., and Nolan, D. S. (2018). Structural changes preceding rapid intensification in tropical cyclones as shown in a large ensemble of idealized simulations. J. Atmos. Sci. 75, 555–569.
Miyamoto, Y., and Takemi, T. (2003). A transition mechanism for spontaneous axisymmetric intensification of tropical cyclone. J. Atmos. Sci. 70, 112–129.
Molinari, J., and Vollaro, D. (2012). A subtropical cyclonic gyre associated with interactions of the MJO and the midlatitude jet. Mon. Weather Rev. 140, 343–357. doi:10.1175/mwr-d-11-00049.1
Mueller, K. J. M. DeMaria, Knaff, J. A., Kossin, J. P., Vonder Haar, T. H., and Vonder Haar, T. H. (2006). Objective estimation of tropical cyclone wind structure from infrared satellite data. Weather Forecast. 21, 990–1005. doi:10.1175/waf955.1
Qiu, W., Ren, F., Wu, L., and Chen, L. (2019). Characteristics of tropical cyclone extreme precipitation and its preliminary causes in Southeast China. Meteorol. Atmospheric Phys. 131 (3), 613–626.
Qiu, W., Wu, L., and Ren, F. (2020). Monsoonal Influences on Offshore Rapid Intensification of Landfalling Typhoons in a Sheared Environment over the South China Sea. Wea. Forecast. 35 (2), 623–634.
Reasor, P. D., Rogers, R., and Lorsolo, S. (2013). Environmental flow impacts on tropical cyclone structure diagnosed from airborne Doppler radar composites. Mon. Weather Rev. 141 (9), 2949–2969. doi:10.1175/mwr-d-12-00334.1
Riemer, M., and Montgomery, M. T. (2001). Effects of vertical wind shear on the intensity and structure of numerically simulated hurricanes. Mon. Weather Rev. 129, 2249–2269. doi:10.1175/1520-0493(2001)129<2249:eovwso>2.0.co;2
Shen, Y. P., Zhao, Y., and Pan,, (2014). A high spatiotemporal gauge-satellite merged precipitation analysis over China. J. Geophys. Res. 119, 3063–3075.
Shimada, U., Aonashi, K., and Miyamoto, Y. (2017). Tropical cyclone intensity change and axisymmetricity deduced from GSMaP. Mon. Weather Rev. 145, 1003–1017. doi:10.1175/mwr-d-16-0244.1
Shu, S., Ming, J., and Chi, P. (2012). Large-scale characteristics and probability of rapidly intensifying tropical cyclones in the Western North Pacific basin. Weather Forecast. 27, 411–423. doi:10.1175/waf-d-11-00042.1
Tsuji, H., and Nakajima, K. (2019). Relationship between the change in size of tropical cyclones and spatial patterns of precipitation. JGR. Atmos. 124, 9948–9962. doi:10.1029/2019jd030404
Uhlhorn, E. W. B. W., Klotz, T., Vukicevic, P. D., Rogers, R. F., and Vukicevic, T. (2014). Observed hurricane wind speed asymmetries and relationships to motion and environmental shear. Mon. Weather Rev. 142, 1290–1311. doi:10.1175/mwr-d-13-00249.1
Wang, Y. (2009). How do outer spiral rainbands affect tropical cyclone structure and intensity? J. Atmos. Sci. 66, 1250–1273. doi:10.1175/2008jas2737.1
Wu, L., Ni, Z., Duan, J., and Zong, H. (2013). Sudden tropical cyclone track changes over the western north pacific: A composite study. Mon. Weather Rev. 141, 2597–2610. doi:10.1175/mwr-d-12-00224.1
Xu, W., Jiang, H., and Kang, X. (2014). 2014: Rainfall asymmetries of tropical cyclones prior to, during, and after making landfall in south China and southeast United States. Atmos. Res. 139 (3), 18–26. doi:10.1016/j.atmosres.2013.12.015
Ying, M., Zhang, W., Yu, H., Lu, X., Feng, J., Fan, Y., et al. (2014). An overview of the China meteorological administration tropical cyclone database. J. Atmos. Ocean. Technol. 31, 287–301. doi:10.1175/jtech-d-12-00119.1
Yu, Z., Wang, Y., Xu, H., Davidson, N., Chen, Y., ChenY, Yu H., et al. (2017). On the relationship between intensity and rainfall distribution in tropical cyclones making landfall over China. J. Appl. Meteorol. Climatol. 56 (10), 2883–2901. doi:10.1175/jamc-d-16-0334.1
Yu, Z., Wang, Y., Xu, H., and Duan, Y. (2022). The relationship between the inner-core size and the rainfall distribution in landfalling tropical cyclones over China. Geophys. Res. Lett. 49, e32021GL097576. doi:10.1029/2021gl097576
Yu, Z., Wang, Y., and Xu, H. (2015). Observed rainfall asymmetry in tropical cyclones making landfall over China. J. Appl. Meteorol. Climatol. 54 (1), 117–136. doi:10.1175/jamc-d-13-0359.1
Zhao, H. K., Zhao, X., Jiang, P. J., Klotzbach, , et al. (2022a). Interannual and interdecadal drivers of meridional migration of western north pacific tropical cyclone lifetime maximum intensity location. J. Climate 35, 2709–2722.
Keywords: landfall tropical cyclone, rapid intensification, inner rainbands, rain rate, landfalling process
Citation: Xiang C, Xu Y, Lin J and Liu S (2022) Analysis of the inner rainbands of tropical cyclones over the South China Sea during the landfall process. Front. Earth Sci. 10:956977. doi: 10.3389/feart.2022.956977
Received: 30 May 2022; Accepted: 26 July 2022;
Published: 09 September 2022.
Edited by:
Darko Koracin, University of Split, CroatiaReviewed by:
Boqi Liu, Chinese Academy of Meteorological Sciences, ChinaHaikun Zhao, Nanjing University of Information Science and Technology, China
Copyright © 2022 Xiang, Xu, Lin and Liu. This is an open-access article distributed under the terms of the Creative Commons Attribution License (CC BY). The use, distribution or reproduction in other forums is permitted, provided the original author(s) and the copyright owner(s) are credited and that the original publication in this journal is cited, in accordance with accepted academic practice. No use, distribution or reproduction is permitted which does not comply with these terms.
*Correspondence: Yinglong Xu, xuyl@cma.gov.cn